Advances in private training for production on-device language models - Related to advances, production, on-device, expand, language
Advances in private training for production on-device language models
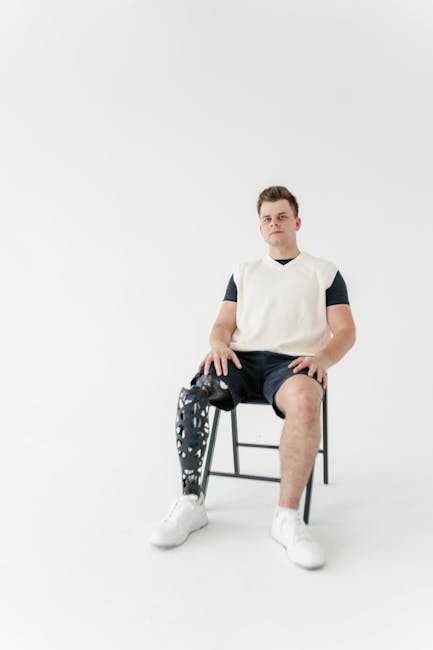
Language models (LMs) trained to predict the next word given input text are the key technology for many applications [1, 2]. In Gboard, LMs are used to improve individuals’ typing experience by supporting elements like next word prediction (NWP), Smart Compose, smart completion and suggestion, slide to type, and proofread. Deploying models on individuals’ devices rather than enterprise servers has advantages like lower latency and superior privacy for model usage. While training on-device models directly from user data effectively improves the utility performance for applications such as NWP and smart text selection, protecting the privacy of user data for model training is significant.
Gboard elements powered by on-device language models.
In this blog we discuss how years of research advances now power the private training of Gboard LMs, since the proof-of-concept development of federated learning (FL) in 2017 and formal differential privacy (DP) guarantees in 2022. FL enables mobile phones to collaboratively learn a model while keeping all the training data on device, and DP provides a quantifiable measure of data anonymization. Formally, DP is often characterized by (ε, δ) with smaller values representing stronger guarantees. Machine learning (ML) models are considered to have reasonable DP guarantees for ε=10 and strong DP guarantees for ε=1 when δ is small.
As of today, all NWP neural network LMs in Gboard are trained with FL with formal DP guarantees, and all future launches of Gboard LMs trained on user data require DP. These 30+ Gboard on-device LMs are launched in 7+ languages and 15+ countries, and satisfy (ɛ, δ)-DP guarantees of small δ of 10-10 and ɛ between [website] and [website] To the best of our knowledge, this is the largest known deployment of user-level DP in production at Google or anywhere, and the first time a strong DP guarantee of ɛ < 1 is announced for models trained directly on user data.
Privacy principles and practices in Gboard.
In “Private Federated Learning in Gboard”, we discussed how different privacy principles are currently reflected in production models, including:
Transparency and user control: We provide disclosure of what data is used, what purpose it is used for, how it is processed in various channels, and how Gboard people can easily configure the data usage in learning models.
Data minimization: FL immediately aggregates only focused updates that improve a specific model. Secure aggregation (SecAgg) is an encryption method to further guarantee that only aggregated results of the ephemeral updates can be accessed.
Data anonymization: DP is applied by the server to prevent models from memorizing the unique information in individual user’s training data.
Auditability and verifiability: We have made public the key algorithmic approaches and privacy accounting in open-sourced code (TFF aggregator, TFP DPQuery, DP accounting, and FL system).
In recent years, FL has become the default method for training Gboard on-device LMs from user data. In 2020, a DP mechanism that clips and adds noise to model updates was used to prevent memorization for training the Spanish LM in Spain, which satisfies finite DP guarantees (Tier 3 described in “How to DP-fy ML“ guide). In 2022, with the help of the DP-Follow-The-Regularized-Leader (DP-FTRL) algorithm, the Spanish LM became the first production neural network trained directly on user data showcased with a formal DP guarantee of (ε[website], δ=10-10)-DP (equivalent to the reported ρ[website] zero-Concentrated-Differential-Privacy), and therefore satisfies reasonable privacy guarantees (Tier 2).
Differential privacy by default in federated learning.
In “Federated Learning of Gboard Language Models with Differential Privacy”, we showcased that all the NWP neural network LMs in Gboard have DP guarantees, and all future launches of Gboard LMs trained on user data require DP guarantees. DP is enabled in FL by applying the following practices:
Pre-train the model with the multilingual C4 dataset.
Via simulation experiments on public datasets, find a large DP-noise-to-signal ratio that allows for high utility. Increasing the number of clients contributing to one round of model modification improves privacy while keeping the noise ratio fixed for good utility, up to the point the DP target is met, or the maximum allowed by the system and the size of the population.
Configure the parameter to restrict the frequency each client can contribute ([website], once every few days) based on computation budget and estimated population in the FL system.
Run DP-FTRL training with limits on the magnitude of per-device updates chosen either via adaptive clipping, or fixed based on experience.
SecAgg can be additionally applied by adopting the advances in improving computation and communication for scales and sensitivity.
Federated learning with differential privacy and (SecAgg).
The DP guarantees of launched Gboard NWP LMs are visualized in the barplot below. The x-axis reveals LMs labeled by language-locale and trained on corresponding populations; the y-axis reveals the ε value when δ is fixed to a small value of 10-10 for (ε, δ)-DP (lower is advanced). The utility of these models are either significantly advanced than previous non-neural models in production, or comparable with previous LMs without DP, measured based on user-interactions metrics during A/B testing. For example, by applying the best practices, the DP guarantee of the Spanish model in Spain is improved from ε[website] to ε[website] SecAgg is additionally used for training the Spanish model in Spain and English model in the US. More details of the DP guarantees are reported in the appendix following the guidelines outlined in “How to DP-fy ML”.
The ε~10 DP guarantees of many launched LMs are already considered reasonable for ML models in practice, while the journey of DP FL in Gboard continues for improving user typing experience while protecting data privacy. We are excited to announce that, for the first time, production LMs of Portuguese in Brazil and Spanish in Latin America are trained and launched with a DP guarantee of ε ≤ 1, which satisfies Tier 1 strong privacy guarantees. Specifically, the (ε[website], δ=10-10)-DP guarantee is achieved by running the advanced Matrix Factorization DP-FTRL (MF-DP-FTRL) algorithm, with 12,000+ devices participating in every training round of server model modification larger than the common setting of 6500+ devices, and a carefully configured policy to restrict each client to at most participate twice in the total 2000 rounds of training in 14 days in the large Portuguese user population of Brazil. Using a similar setting, the es-US Spanish LM was trained in a large population combining multiple countries in Latin America to achieve (ε[website], δ=10-10)-DP. The ε ≤ 1 es-US model significantly improved the utility in many countries, and launched in Colombia, Ecuador, Guatemala, Mexico, and Venezuela. For the smaller population in Spain, the DP guarantee of es-ES LM is improved from ε[website] to ε[website] by only replacing DP-FTRL with MF-DP-FTRL without increasing the number of devices participating every round. More technical details are disclosed in the colab for privacy accounting.
DP guarantees for Gboard NWP LMs (the purple bar represents the first es-ES launch of ε[website]; cyan bars represent privacy improvements for models trained with MF-DP-FTRL; tiers are from “How to DP-fy ML“ guide; en-US* and es-ES* are additionally trained with SecAgg).
Our experience implies that DP can be achieved in practice through system algorithm co-design on client participation, and that both privacy and utility can be strong when populations are large and a large number of devices' contributions are aggregated. Privacy-utility-computation trade-offs can be improved by using public data, the new MF-DP-FTRL algorithm, and tightening accounting. With these techniques, a strong DP guarantee of ε ≤ 1 is possible but still challenging. Active research on empirical privacy auditing [1, 2] implies that DP models are potentially more private than the worst-case DP guarantees imply. While we keep pushing the frontier of algorithms, which dimension of privacy-utility-computation should be prioritized?
We are actively working on all privacy aspects of ML, including extending DP-FTRL to distributed DP and improving auditability and verifiability. Trusted Execution Environment opens the opportunity for substantially increasing the model size with verifiable privacy. The recent breakthrough in large LMs (LLMs) motivates us to rethink the usage of public information in private training and more future interactions between LLMs, on-device LMs, and Gboard production.
The authors would like to thank Peter Kairouz, Brendan McMahan, and Daniel Ramage for their early feedback on the blog post itself, Shaofeng Li and Tom Small for helping with the animated figures, and the teams at Google that helped with algorithm design, infrastructure implementation, and production maintenance. The collaborators below directly contribute to the presented results:
Research and algorithm development: Galen Andrew, Stanislav Chiknavaryan, Christopher A. Choquette-Choo, Arun Ganesh, Peter Kairouz, Ryan McKenna, H. Brendan McMahan, Jesse Rosenstock, Timon Van Overveldt, Keith Rush, Shuang Song, Thomas Steinke, Abhradeep Guha Thakurta, Om Thakkar, and Yuanbo Zhang.
Infrastructure, production and leadership support: Mingqing Chen, Stefan Dierauf, Billy Dou, Hubert Eichner, Zachary Garrett, Jeremy Gillula, Jianpeng Hou, Hui Li, Xu Liu, Wenzhi Mao, Brett McLarnon, Mengchen Pei, Daniel Ramage, Swaroop Ramaswamy, Haicheng Sun, Andreas Terzis, Yun Wang, Shanshan Wu, Yu Xiao, and Shumin Zhai.
At TNW, we are all about supporting and elevating startups and entrepreneurs who are doing epic stuff with tech. When Red Bull reached out to talk abo...
Microsoft PowerBI is a one of the most popular Business Intelligence (BI) tools, and while it has all the attributes you need to create dynamic analytic...
Graphs, in which objects and their relations are represented as nodes (or vertices) and edges (or links) between pairs of nodes, are ubiquitous in com...
Using AI to expand global access to reliable flood forecasts
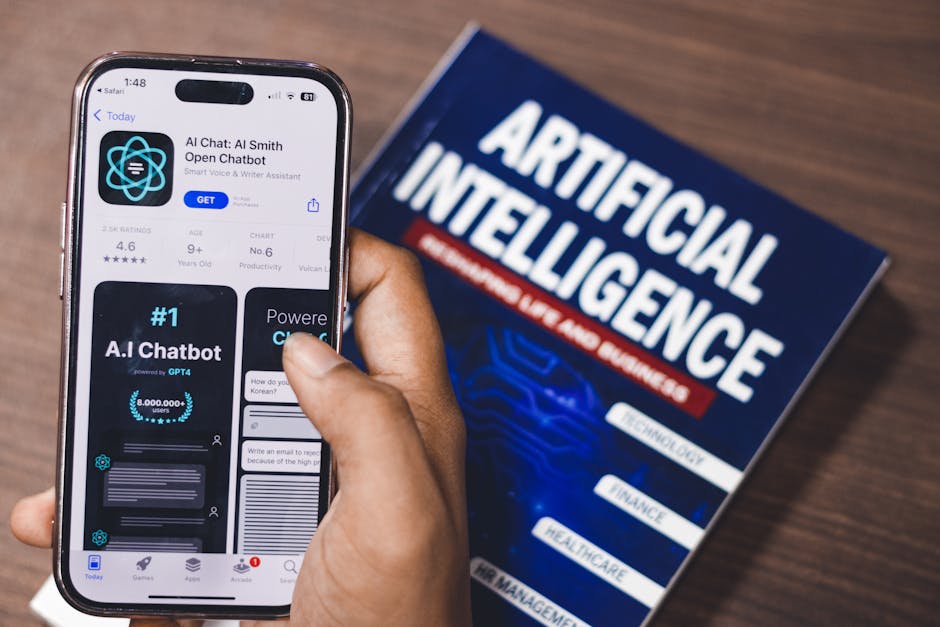
Floods are the most common natural disaster, and are responsible for roughly $50 billion in annual financial damages worldwide. The rate of flood-related disasters has more than doubled since the year 2000 partly due to climate change. Nearly [website] billion people, making up 19% of the world’s population, are exposed to substantial risks from severe flood events. Upgrading early warning systems to make accurate and timely information accessible to these populations can save thousands of lives per year.
Driven by the potential impact of reliable flood forecasting on people’s lives globally, we started our flood forecasting effort in 2017. Through this multi-year journey, we advanced research over the years hand-in-hand with building a real-time operational flood forecasting system that provides alerts on Google Search, Maps, Android notifications and through the Flood Hub. However, in order to scale globally, especially in places where accurate local data is not available, more research advances were required.
In “Global prediction of extreme floods in ungauged watersheds”, , we demonstrate how machine learning (ML) technologies can significantly improve global-scale flood forecasting relative to the current state-of-the-art for countries where flood-related data is scarce. With these AI-based technologies we extended the reliability of currently-available global nowcasts, on average, from zero to five days, and improved forecasts across regions in Africa and Asia to be similar to what are currently available in Europe. The evaluation of the models was conducted in collaboration with the European Center for Medium Range Weather Forecasting (ECMWF).
These technologies also enable Flood Hub to provide real-time river forecasts up to seven days in advance, covering river reaches across over 80 countries. This information can be used by people, communities, governments and international organizations to take anticipatory action to help protect vulnerable populations.
Here is a common scenario : An A/B test was conducted, where a random sample of units ([website] consumers) were selected for a cam...
Accurate impact estimations can make or break your business case.
Yet, despite its importance, most teams use oversimplified calculations that can le...
In December 1972, at the American Association for the Advancement of Science meeting in Washington, [website], MIT meteorology professor Ed Lorenz gave a t...
Synthetic Data Generation with LLMs
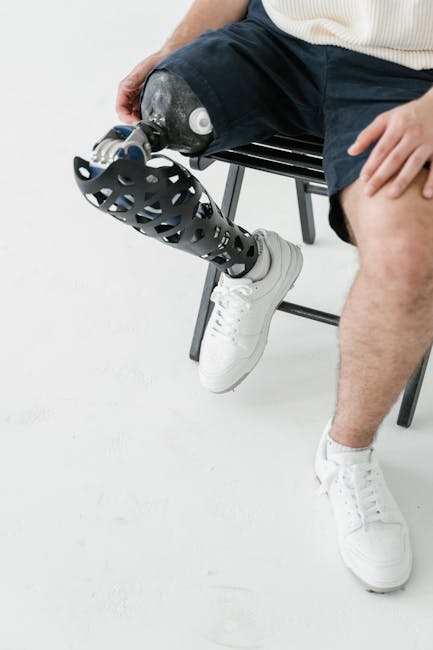
Over the past two years while working with financial firms, I’ve observed firsthand how they identify and prioritize Generative AI use cases, balancing complexity with potential value.
Retrieval-Augmented Generation (RAG) often stands out as a foundational capability across many LLM-driven solutions, striking a balance between ease of implementation and real-world impact. By combining a retriever that surfaces relevant documents with an LLM that synthesizes responses, RAG streamlines knowledge access, making it invaluable for applications like customer support, research, and internal knowledge management.
Defining clear evaluation criteria is key to ensuring LLM solutions meet performance standards, just as Test-Driven Development (TDD) ensures reliability in traditional software. Drawing from TDD principles, an evaluation-driven approach sets measurable benchmarks to validate and improve AI workflows. This becomes especially crucial for LLMs, where the complexity of open-ended responses demands consistent and thoughtful evaluation to deliver reliable results.
For RAG applications, a typical evaluation set includes representative input-output pairs that align with the intended use case. For example, in chatbot applications, this might involve Q&A pairs reflecting user inquiries. In other contexts, such as retrieving and summarizing relevant text, the evaluation set could include source documents alongside expected summaries or extracted key points. These pairs are often generated from a subset of documents, such as those that are most viewed or frequently accessed, ensuring the evaluation focuses on the most relevant content.
Creating evaluation datasets for RAG systems has traditionally faced two major challenges.
The process often relied on subject matter experts (SMEs) to manually review documents and generate Q&A pairs, making it time-intensive, inconsistent, and costly. Limitations preventing LLMs from processing visual elements within documents, such as tables or diagrams, as they are restricted to handling text. Standard OCR tools struggle to bridge this gap, often failing to extract meaningful information from non-textual content.
The challenges of handling complex documents have evolved with the introduction of multimodal capabilities in foundation models. Commercial and open-source models can now process both text and visual content. This vision capability eliminates the need for separate text-extraction workflows, offering an integrated approach for handling mixed-media PDFs.
By leveraging these vision attributes, models can ingest entire pages at once, recognizing layout structures, chart labels, and table content. This not only reduces manual effort but also improves scalability and data quality, making it a powerful enabler for RAG workflows that rely on accurate information from a variety of information.
Dataset Curation for Wealth Management Research analysis.
To demonstrate a solution to the problem of manual evaluation set generation, I tested my approach using a sample document — the 2023 Cerulli findings. This type of document is typical in wealth management, where analyst-style reports often combine text with complex visuals. For a RAG-powered search assistant, a knowledge corpus like this would likely contain many such documents.
My goal was to demonstrate how a single document could be leveraged to generate Q&A pairs, incorporating both text and visual elements. While I didn’t define specific dimensions for the Q&A pairs in this test, a real-world implementation would involve providing details on types of questions (comparative, analysis, multiple choice), topics (investment strategies, account types), and many other aspects. The primary focus of this experiment was to ensure the LLM generated questions that incorporated visual elements and produced reliable answers.
My workflow, illustrated in the diagram, leverages Anthropic’s Claude Sonnet [website] model, which simplifies the process of working with PDFs by handling the conversion of documents into images before passing them to the model. This built-in functionality eliminates the need for additional third-party dependencies, streamlining the workflow and reducing code complexity.
I excluded preliminary pages of the report like the table of contents and glossary, focusing on pages with relevant content and charts for generating Q&A pairs. Below is the prompt I used to generate the initial question-answer sets.
You are an expert at analyzing financial reports and generating question-answer pairs. For the provided PDF, the 2023 Cerulli investigation:
1. Analyze pages {start_idx} to {end_idx} and for **each** of those 10 pages:
- Identify the **exact page title** as it appears on that page ([website], "Exhibit [website] Core Market Databank, 2023").
- If the page includes a chart, graph, or diagram, create a question that references that visual element. Otherwise, create a question about the textual content.
- Generate two distinct answers to that question ("answer_1" and "answer_2"), both supported by the page’s content.
- Identify the correct page number as indicated in the bottom left corner of the page.
2. Return exactly 10 results as a valid JSON array (a list of dictionaries). Each dictionary should have the keys: “page” (int), “page_title” (str), “question” (str), “answer_1” (str), and “answer_2” (str). The page title typically includes the word "Exhibit" followed by a number.
To refine the Q&A generation process, I implemented a comparative learning approach that generates two distinct answers for each question. During the evaluation phase, these answers are assessed across key dimensions such as accuracy and clarity, with the stronger response selected as the final answer.
This approach mirrors how humans often find it easier to make decisions when comparing alternatives rather than evaluating something in isolation. It’s like an eye examination: the optometrist doesn’t ask if your vision has improved or declined but instead, presents two lenses and asks, Which is clearer, option 1 or option 2? This comparative process eliminates the ambiguity of assessing absolute improvement and focuses on relative differences, making the choice simpler and more actionable. Similarly, by presenting two concrete answer options, the system can more effectively evaluate which response is stronger.
This methodology is also cited as a best practice in the article “What We Learned from a Year of Building with LLMs” by leaders in the AI space. They highlight the value of pairwise comparisons, stating: “Instead of asking the LLM to score a single output on a Likert scale, present it with two options and ask it to select the enhanced one. This tends to lead to more stable results.” I highly recommend reading their three-part series, as it provides invaluable insights into building effective systems with LLMs!
For evaluating the generated Q&A pairs, I used Claude Opus for its advanced reasoning capabilities. Acting as a “judge,” the LLM compared the two answers generated for each question and selected the better option based on criteria such as directness and clarity. This approach is supported by extensive research (Zheng et al., 2023) that showcases LLMs can perform evaluations on par with human reviewers.
This approach significantly reduces the amount of manual review required by SMEs, enabling a more scalable and efficient refinement process. While SMEs remain essential during the initial stages to spot-check questions and validate system outputs, this dependency diminishes over time. Once a sufficient level of confidence is established in the system’s performance, the need for frequent spot-checking is reduced, allowing SMEs to focus on higher-value tasks.
Claude’s PDF capability has a limit of 100 pages, so I broke the original document into four 50-page sections. When I tried processing each 50-page section in a single request — and explicitly instructed the model to generate one Q&A pair per page — it still missed some pages. The token limit wasn’t the real problem; the model tended to focus on whichever content it considered most relevant, leaving certain pages underrepresented.
To address this, I experimented with processing the document in smaller batches, testing 5, 10, and 20 pages at a time. Through these tests, I found that batches of 10 pages ([website], pages 1–10, 11–20, etc.) provided the best balance between precision and efficiency. Processing 10 pages per batch ensured consistent results across all pages while optimizing performance.
Another challenge was linking Q&A pairs back to their source. Using tiny page numbers in a PDF’s footer alone didn’t consistently work. In contrast, page titles or clear headings at the top of each page served as reliable anchors. They were easier for the model to pick up and helped me accurately map each Q&A pair to the right section.
Below is an example page from the study, featuring two tables with numerical data. The following question was generated for this page:
How has the distribution of AUM changed across different-sized Hybrid RIA firms?
Answer: Mid-sized firms ($25m to <$100m) experienced a decline in AUM share from [website] to [website].
In the first table, the 2017 column reveals a [website] share of AUM for mid-sized firms, which decreases to [website] in 2022, thereby showcasing the LLM’s ability to synthesize visual and tabular content accurately.
Combining caching, batching and a refined Q&A workflow led to three key advantages:
In my experiment, processing a singular analysis without caching would have cost $9, but by leveraging caching, I reduced this cost to $3 — a 3x cost savings . Per Anthropic’s pricing model, creating a cache costs $[website] / million tokens, however, reads from the cache are only $[website] / million tokens. In contrast, input tokens cost $3 / million tokens when caching is not used.
. Per Anthropic’s pricing model, creating a cache costs $[website] / million tokens, however, reads from the cache are only $[website] / million tokens. In contrast, input tokens cost $3 / million tokens when caching is not used. In a real-world scenario with more than one document, the savings become even more significant. For example, processing 10,000 research reports of similar length without caching would cost $90,000 in input costs alone. With caching, this cost drops to $30,000, achieving the same precision and quality while saving $60,000.
Using Anthropic’s Batches API cuts output costs in half, making it a much cheaper option for certain tasks. Once I had validated the prompts, I ran a single batch job to evaluate all the Q&A answer sets at once. This method proved far more cost-effective than processing each Q&A pair individually.
For example, Claude 3 Opus typically costs $15 per million output tokens. By using batching, this drops to $[website] per million tokens — a 50% reduction. In my experiment, each Q&A pair generated an average of 100 tokens, resulting in approximately 20,000 output tokens for the document. At the standard rate, this would have cost $[website] With batch processing, the cost was reduced to $[website], highlighitng how this approach optimizes costs for non-sequential tasks like evaluation runs.
Objects and their relationships are ubiquitous in the world around us, and relationships can be as key to understanding an object as its own att...
Developer platform GitHub has introduced Agent Mode for GitHub Copilot, giving its AI-powered coding assistant the ability to iterate on its own code,...
Vous êtes en Europe et vous utilisez l’IA pour vos tâches au quotidienne ? Voici ce que vous devez savoir concernant l’adoption d’une IA éthique par l...
Market Impact Analysis
Market Growth Trend
2018 | 2019 | 2020 | 2021 | 2022 | 2023 | 2024 |
---|---|---|---|---|---|---|
23.1% | 27.8% | 29.2% | 32.4% | 34.2% | 35.2% | 35.6% |
Quarterly Growth Rate
Q1 2024 | Q2 2024 | Q3 2024 | Q4 2024 |
---|---|---|---|
32.5% | 34.8% | 36.2% | 35.6% |
Market Segments and Growth Drivers
Segment | Market Share | Growth Rate |
---|---|---|
Machine Learning | 29% | 38.4% |
Computer Vision | 18% | 35.7% |
Natural Language Processing | 24% | 41.5% |
Robotics | 15% | 22.3% |
Other AI Technologies | 14% | 31.8% |
Technology Maturity Curve
Different technologies within the ecosystem are at varying stages of maturity:
Competitive Landscape Analysis
Company | Market Share |
---|---|
Google AI | 18.3% |
Microsoft AI | 15.7% |
IBM Watson | 11.2% |
Amazon AI | 9.8% |
OpenAI | 8.4% |
Future Outlook and Predictions
The Advances Private Training landscape is evolving rapidly, driven by technological advancements, changing threat vectors, and shifting business requirements. Based on current trends and expert analyses, we can anticipate several significant developments across different time horizons:
Year-by-Year Technology Evolution
Based on current trajectory and expert analyses, we can project the following development timeline:
Technology Maturity Curve
Different technologies within the ecosystem are at varying stages of maturity, influencing adoption timelines and investment priorities:
Innovation Trigger
- Generative AI for specialized domains
- Blockchain for supply chain verification
Peak of Inflated Expectations
- Digital twins for business processes
- Quantum-resistant cryptography
Trough of Disillusionment
- Consumer AR/VR applications
- General-purpose blockchain
Slope of Enlightenment
- AI-driven analytics
- Edge computing
Plateau of Productivity
- Cloud infrastructure
- Mobile applications
Technology Evolution Timeline
- Improved generative models
- specialized AI applications
- AI-human collaboration systems
- multimodal AI platforms
- General AI capabilities
- AI-driven scientific breakthroughs
Expert Perspectives
Leading experts in the ai tech sector provide diverse perspectives on how the landscape will evolve over the coming years:
"The next frontier is AI systems that can reason across modalities and domains with minimal human guidance."
— AI Researcher
"Organizations that develop effective AI governance frameworks will gain competitive advantage."
— Industry Analyst
"The AI talent gap remains a critical barrier to implementation for most enterprises."
— Chief AI Officer
Areas of Expert Consensus
- Acceleration of Innovation: The pace of technological evolution will continue to increase
- Practical Integration: Focus will shift from proof-of-concept to operational deployment
- Human-Technology Partnership: Most effective implementations will optimize human-machine collaboration
- Regulatory Influence: Regulatory frameworks will increasingly shape technology development
Short-Term Outlook (1-2 Years)
In the immediate future, organizations will focus on implementing and optimizing currently available technologies to address pressing ai tech challenges:
- Improved generative models
- specialized AI applications
- enhanced AI ethics frameworks
These developments will be characterized by incremental improvements to existing frameworks rather than revolutionary changes, with emphasis on practical deployment and measurable outcomes.
Mid-Term Outlook (3-5 Years)
As technologies mature and organizations adapt, more substantial transformations will emerge in how security is approached and implemented:
- AI-human collaboration systems
- multimodal AI platforms
- democratized AI development
This period will see significant changes in security architecture and operational models, with increasing automation and integration between previously siloed security functions. Organizations will shift from reactive to proactive security postures.
Long-Term Outlook (5+ Years)
Looking further ahead, more fundamental shifts will reshape how cybersecurity is conceptualized and implemented across digital ecosystems:
- General AI capabilities
- AI-driven scientific breakthroughs
- new computing paradigms
These long-term developments will likely require significant technical breakthroughs, new regulatory frameworks, and evolution in how organizations approach security as a fundamental business function rather than a technical discipline.
Key Risk Factors and Uncertainties
Several critical factors could significantly impact the trajectory of ai tech evolution:
Organizations should monitor these factors closely and develop contingency strategies to mitigate potential negative impacts on technology implementation timelines.
Alternative Future Scenarios
The evolution of technology can follow different paths depending on various factors including regulatory developments, investment trends, technological breakthroughs, and market adoption. We analyze three potential scenarios:
Optimistic Scenario
Responsible AI driving innovation while minimizing societal disruption
Key Drivers: Supportive regulatory environment, significant research breakthroughs, strong market incentives, and rapid user adoption.
Probability: 25-30%
Base Case Scenario
Incremental adoption with mixed societal impacts and ongoing ethical challenges
Key Drivers: Balanced regulatory approach, steady technological progress, and selective implementation based on clear ROI.
Probability: 50-60%
Conservative Scenario
Technical and ethical barriers creating significant implementation challenges
Key Drivers: Restrictive regulations, technical limitations, implementation challenges, and risk-averse organizational cultures.
Probability: 15-20%
Scenario Comparison Matrix
Factor | Optimistic | Base Case | Conservative |
---|---|---|---|
Implementation Timeline | Accelerated | Steady | Delayed |
Market Adoption | Widespread | Selective | Limited |
Technology Evolution | Rapid | Progressive | Incremental |
Regulatory Environment | Supportive | Balanced | Restrictive |
Business Impact | Transformative | Significant | Modest |
Transformational Impact
Redefinition of knowledge work, automation of creative processes. This evolution will necessitate significant changes in organizational structures, talent development, and strategic planning processes.
The convergence of multiple technological trends—including artificial intelligence, quantum computing, and ubiquitous connectivity—will create both unprecedented security challenges and innovative defensive capabilities.
Implementation Challenges
Ethical concerns, computing resource limitations, talent shortages. Organizations will need to develop comprehensive change management strategies to successfully navigate these transitions.
Regulatory uncertainty, particularly around emerging technologies like AI in security applications, will require flexible security architectures that can adapt to evolving compliance requirements.
Key Innovations to Watch
Multimodal learning, resource-efficient AI, transparent decision systems. Organizations should monitor these developments closely to maintain competitive advantages and effective security postures.
Strategic investments in research partnerships, technology pilots, and talent development will position forward-thinking organizations to leverage these innovations early in their development cycle.
Technical Glossary
Key technical terms and definitions to help understand the technologies discussed in this article.
Understanding the following technical concepts is essential for grasping the full implications of the security threats and defensive measures discussed in this article. These definitions provide context for both technical and non-technical readers.