From Zero Millions: Latest Updates and Analysis
From zero to millions? How regular people are cashing in on AI
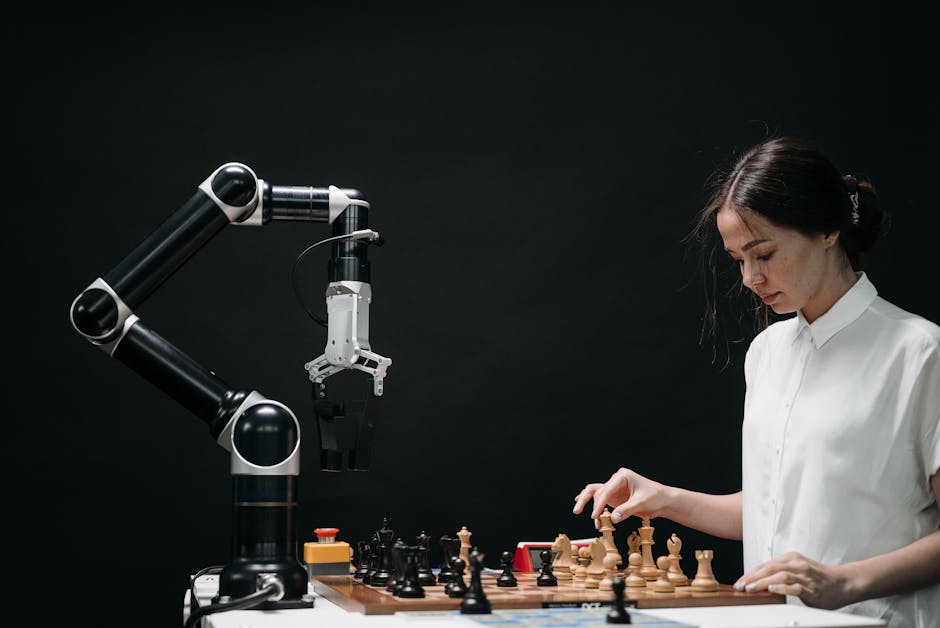
There I was, yet again, scrolling on TikTok before it was banned and then unbanned, and I came across this insane caption.
"Meet The 17-Year-Old CEO Behind A $12 Million AI-Powered Nutrition App."
The article asserts that Cal AI, a GPT-powered nutrition app, makes calorie tracking easier with AI aspects like image recognition and barcode scanning.
Also: AI isn't the next big thing - here's what is.
The app reportedly has over 1 million downloads, $12 million in annual recurring revenue, and a team of 17 full-time employees.
It's led by 17-year-olds Zach Yadegari and Henry Langmack.
Needless to say, my flabbers were gasted! 🤧.
But it got me thinking: if I wanted to start an AI side hustle, how would I do so?
So, if you've ever wanted a side hustle using AI, I got you!
What is a GPT wrapper, and why it might be the easiest way to start building an AI-powered product.
What ideas should you consider, and why they could lead to your next big opportunity.
How to build and scale your app, and what is the best way to get consumers?
If you're new to my work, I'm Lester Mapp, but my friends call me Les. 👋.
I'm a founder with a successful exit and currently the executive chairman of a group of DTC brands. At my core, I'm an award-winning performance marketer.
Coming up with ideas and getting them to market is my thing.
Also: 3 lucrative side hustles you can start right now with OpenAI's Sora video generator.
Now that we have the pleasantries out of the way, kick up your feet and get comfortable.
We're about to dive into a mini AI Shark Tank and say yes or no to some business ideas. 👨🏻💻.
Before we jump into it, I need to bring you up to speed on what GPT wrappers are and how they can help you build a tech empire.
I must explain what a GPT wrapper is and how it works. This context is essential to help you see the possibilities.
A "GPT wrapper" is a software application, like a web app or mobile app, that acts as a user interface built on top of a GPT (Generative Pre-trained Transformer). It provides additional aspects and functionality to make it easier for consumers to interact with the underlying AI.
This includes adding context, refining prompts, and presenting results in a more accessible format. Essentially, it "wraps" around the GPT model to enhance usability.
Also: Your end-clients don't want more AI - here's what to invest in instead.
A real-world example? Think of when General Motors tells us the Tahoe, Yukon, and Escalade are different cars.
Hey GM, are the different cars in the room with us right now? 🤭.
Trolling aside, a GPT wrapper is very similar. The attributes, price points, and target audiences might differ, but the product's core is GPT.
Some real-world examples of GPT wrappers include but are not limited to.
AI-powered writing assistant that helps create blog posts or marketing copy.
Language learning app that generates personalized practice exercises.
Customer service chatbot that handles complex queries.
From a technical standpoint, GPT wrappers handle API calls to the language model, implement prompt engineering techniques, and manage conversation context for more coherent interactions.
Also: Could your job be at risk due to AI? Do this before it's too late.
While GPT wrappers are usually built using OpenAI's API, other options include Anthropic's Claude, Google's PaLM, and several emerging players in the AI space.
All that noted, AI language models are evolving fast, opening up new ways to build cool stuff. 😮💨.
Opportunities and pitfalls of building a GPT wrapper.
Building a GPT wrapper comes with its fair share of pros and cons.
Built-in infrastructure : OpenAI's backend handles all the heavy lifting. You're saving time and skipping the headaches of developing your own AI model.
: OpenAI's backend handles all the heavy lifting. You're saving time and skipping the headaches of developing your own AI model. Speed to market : Wrapping an existing GPT lets you launch faster. Why reinvent the wheel when you can improve the ride?
: Wrapping an existing GPT lets you launch faster. Why reinvent the wheel when you can improve the ride? Cost efficiency: Developing a proprietary AI model costs a ton. Using GPT as your foundation keeps those development costs way down.
Performance limitations : GPT can only work with what it knows, which means it might fall short on domain-specific accuracy.
: GPT can only work with what it knows, which means it might fall short on domain-specific accuracy. Scaling struggles : API throttling and rising costs can be a real hurdle when your app grows fast.
: API throttling and rising costs can be a real hurdle when your app grows fast. Limited differentiation: At the end of the day, you're still building on GPT, so standing out from the crowd might take extra effort.
Wrapping GPT can open doors, but knowing where those doors might slam shut is just as essential.
The things that frustrate you about AI, like being wrong, getting stuck in loops, or showing bias, will likely appear in your app.
This is something to remember, not a reason not to do it.
I'm sure you're wondering, what tools can I use to create a GPT wrapper?
Grab a pen and paper because one of the best tools you can use to make a GPT wrapper is, ironically, ChatGPT.
That mentioned, there are a few other tools worth mentioning. 🛠️.
Bubble: A no-code platform that makes building web apps simple.
FlutterFlow: Great for creating mobile apps with a no-code approach.
LangChain: A popular framework for developers building with LLMs (Large Language Models).
An honorable mention goes to Replit. I've written a detailed article about it; check it out.
If coding isn't your thing, you can hire someone on Upwork or another freelancing platform to help you.
Also: The best AI for coding in 2025 (and what not to use).
While building your app is crucial, the real focus should be on what to build and how to get clients. 📌.
Without a solid idea and a plan to attract consumers, even the best-built tool won't get off the ground.
I have so many ideas, but I don't want to tell you exactly what to do. Instead, I want to teach you how to think about the problem you should be solving.
You must solve a problem for which people are willing to pay top dollar. 💡.
In this case, following your passion isn't great advice. That's more suitable for a hobby, not a sustainable business.
Also: No, AI won't revolutionize shopping - but this will.
That mentioned, the bigger your ambitions are, the bigger the problem you need to solve. Think painkillers, not vitamins.
Before you start building anything, talk to potential customers. Ask questions like:
What solution are you using to solve it now?
Would you pay for a enhanced solution? If so, how much?
Your job is to bridge the gap between your solution's value and the amount your clients are willing to pay. 💰.
The biggest mistake founders make is assuming that if they build it, people will come, which isn't true. Talking to potential consumers gives you insight into what they actually want. Too many founders create solutions looking for a problem.
My advice? Whatever you build, care enough to stay disciplined through the good and bad days. As my mentor would say:
"You won't always be motivated. That's why you need to be disciplined."
Whatever you decide to build, you need to solve a problem for someone willing to pay for it.
The more pressing the problem, the greater your level of success. 💪.
How to market and grow a GPT wrapper business.
10 times out of 10, if you find the right problem, there's already a community.
These communities exist everywhere. They attend conferences, host meetups, and hang out online. 📱.
Moms hang out on social platforms, sharing tips and tricks and offering emotional support.
Formula One fans gather online, discussing the next race or debating who performed best.
This also applies in the professional world: CFOs, CMOs, and CEOs all connect in their own spaces, talking about shared problems. Right now, they're discussing a problem you could solve.
Also: Could AI make you a billionaire in 2025?
Once you've figured out the problem you're solving, start building a community. Ideally, you should build your app and community simultaneously. 👌.
Some call this "building in public," but the real magic is bringing potential people along for the ride.
Take Harrison Hide, for example. He's building Long Lane, a wellness hotel and member club that gives professionals a personalized, all-in-one wellness experience. It focuses on health, nutrition, and mental performance, all in a curated, alcohol-free environment.
Harrison isn't creating a tech solution, but he's proving that building a community first works with any idea. 🎯.
I'm not suggesting you ignore platforms like TikTok and Instagram entirely. You can expand to them later, but it's key to choose your priorities in the early stages. You can't do everything alone, so staying focused is crucial.
Also: ChatGPT Search could destroy online businesses - how you can stay ahead.
This approach is like running a media enterprise first and monetizing through a software solution. 🔎.
And let me tell you, after spending tens of millions of dollars on marketing campaigns, this is by far the best approach.
, Airbnb produced [website] times more revenue for its member hosts by using its community to drive innovation and product development. This statistic demonstrates the significant impact that a well-managed online community can have on a corporation's revenue growth. 🤯.
I know I came at you with a lot today, so here's a quick recap: 😊.
Do the research : Don't build ish without talking to the people you expect to give you money.
: Start building your app after you fully grasp the problem and who you're serving. Keep the community engaged : Share updates, "Today we built this," "What do you think of this?" and so on.
: Share updates, "Today we built this," "What do you think of this?" and so on. Stay consistent : Tough times don't last, but tough people do.
: Tough times don't last, but tough people do. Launch your app: Keep engaging your audience even after launch.
When you become a gazillionaire, let me use your yacht when you're out of town!
😇 Hope this article helps; I'm rooting for you!
'ZDNET Recommends': What exactly does it mean?
ZDNET's recommendations are based on many hours of testing, research, and comparison shopping. We gath......
Can you jailbreak Anthropic's latest AI safety measure? Researchers want you to try -- and are offering up to $20,000 if you suc......
Every year, the spring season brings a series of launch events from the major tech companies, including Microsoft. The software g......
How to use GitHub's AI coding assistant for free - and why it's worth a try
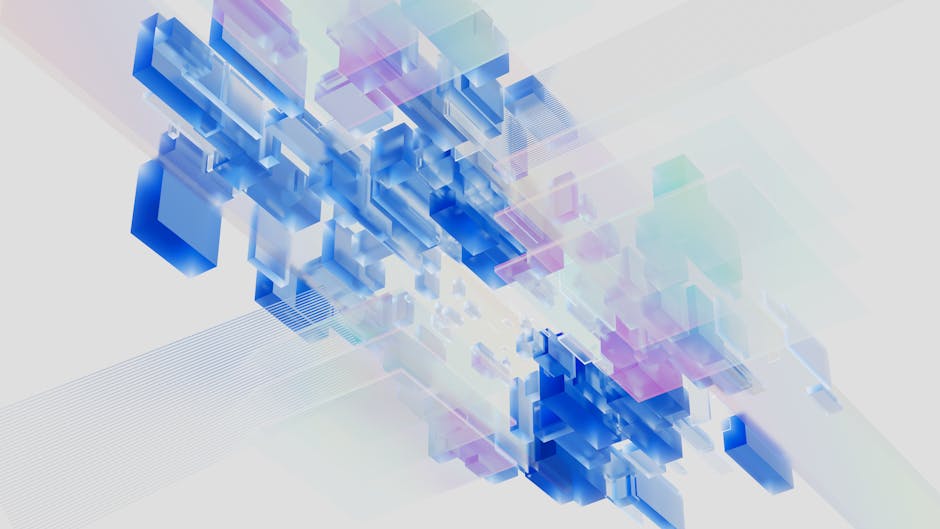
GitHub Copilot is GitHub's AI coding assistant. In December, GitHub presented that the previously paid-only helper now has a free tier. In this article, we'll take you step-by-step through the process of setting up and using this helpful tool in VS Code.
Also: The best AI for coding (and what not to use - including DeepSeek R1).
Before we begin, I will share one cautionary note. In my testing of the free GitHub Copilot's programming prowess, the AI failed half of my tests. That's not great. Paid subscriptions offer access to OpenAI's higher-level LLMs, so if you pay for the service, you may get more effective results.
Even with the AI missteps, I still think it's worth trying out the free tier. The free version gives you 50 full queries a month, which should be more than enough to give you a solid feel for how the product performs and integrates into your VS Code workflow.
Let's get started. As prerequisites for this guided tour, please install VS Code, upgrade it to the current version, and make sure you have a GitHub account.
Now, open VS Code. You'll see a prompt inviting you to get started using GitHub Copilot for free.
There's a two-step authorization process. First, link your account.
Once that's done, you'll be dropped into a start screen where you can begin interacting with GitHub Copilot in either chat mode or in-line with your code.
This will bring you to a main settings screen. Here there are three options I recommend you turn off.
The first option allows or disallows Copilot from using public code as part of its suggestions. I, personally, don't want GitHub Copilot to incorporate public code snippets into my code. I'd rather it build those snippets on its own, rather than run the risk of pulling in someone else's code. Yes, all these AIs are trained on public code, but where I have the option of turning off blatant code grabs, I will.
The second option allows GitHub to use my code for improvements. No. Just no. I do release most of my code as open source, but I still don't want GitHub incorporating it into their data.
Also: I tested DeepSeek's R1 and V3 coding skills - and we're not all doomed (yet).
The third option is similar. I don't want GitHub using my code for training. Period.
Once you've made these settings changes (or whichever ones you want to make), close the web page and return to VS Code. GitHub Copilot is installed as an extension to VS Code. If you open up your extensions list, you'll see GitHub Copilot's two extensions installed and activated.
Go ahead and close the extensions pane. Now it's time to actually use the AI.
In my first example, I opened a block of code in the editor. In the chat box, I asked GitHub Copilot to find any logic errors. As you can see, it scanned the code in the editor window and gave me some suggestions.
The arrow points to a logic error that could have been fatal. That's a very valuable observation that I might not have found during initial testing.
What's interesting is that GitHub Copilot also points to fixes, which you can apply simply by pressing the "Apply" button. Assuming Copilot's code works, that can be a real time-saver.
You can also access the AI right from the code editor itself by pressing Control-I. Here, I've pressed Control-I, which opens a small chat pane. I then asked Copilot to write code that highlights duplicated lines.
As you can see, it wrote the code inline, right where I asked for it. Hitting Accept drops the AI-written code directly into the editor.
That's it. That's all there is to using GitHub Copilot. With 50 free queries a month, you should be able to get a very good idea of whether or not it's a useful service. Why not give it a try?
Also: OpenAI launches new o3-mini model - here's how free ChatGPT customers can try it.
Have you tried GitHub Copilot's free tier yet? Do you think AI-powered coding assistants like Copilot genuinely improve productivity, or do they introduce more problems than they solve? What elements do you find most useful or most frustrating? Are you comfortable with Copilot's data policies, or do you have concerns about code privacy? Let us know in the comments below.
When you think of headphones, AR/VR headsets, and smart jewelry companies OpenAI may not come to mind. However, a new tra......
There I was, yet again, scrolling on TikTok before it was banned and then unbanned, and I came across this insane caption.
Remember Nokia? Back before smartphones, many of us carried Nokia's nearly indestructible cell phones. They no longer make p......
How to Create Network Graph Visualizations in Microsoft PowerBI
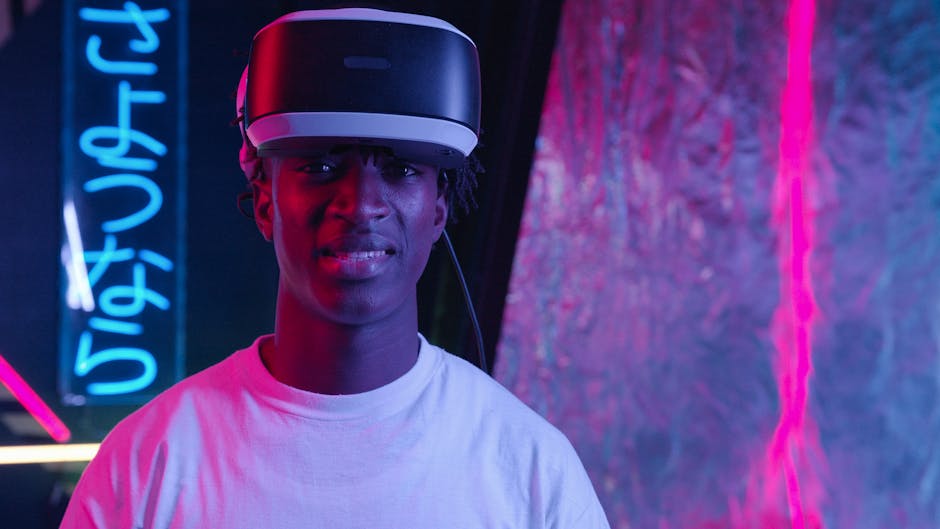
Microsoft PowerBI is a one of the most popular Business Intelligence (BI) tools, and while it has all the elements you need to create dynamic analytic reporting for stakeholders across the business, creating some advanced data visualizations is more challenging.
This article will walk through how to create large network graph visualizations in Microsoft PowerBI to enable dynamic and interactive exploration of interconnected datasets such as supply chain networks, financial transactions, and much more.
But before we do that, let’s take a look at some quick foundations of network graphs.
Data for network graphs, referred to as “graph data” is data formatted in node and edge format. Nodes represent discrete things and edges represent the relationships between nodes.
Let’s take a simple example of an online social network, which can be represented in graph format.
Nodes refer to profiles, while edges refer to following status.
A simple network of 3 profiles might end up looking like this:
When visualizing network graphs, we can embed additional information about nodes and edges in various ways, such as but not limited to:
Node size Edge size Node color Edge color Labels.
So now that you know the basic building blocks of a network graph, how do you structure and transform your dataset?
While you might be thinking, “we only have relational data where I’m at”, that’s often not the case. In fact, lots of relational datasets can be visualized as a network graph.
Let’s take a simple sales table as an example with columns for product name, customer name, and quantity.
We can represent this same sales table as a network graph by representing both product name as the node type “product”, customer name as the node type “customer”, and each row as the edge “Purchased”.
Visualized as a network graph, this might look something like:
There are a few ways this data is structured, such as but not limited to:
Node & Edge Lists (Often in .csv format) Graph Databases (Such as Neo4j) Graph Files (such as GraphML or GEXF).
But in this article, we will be using a combined node and edge list into a single tabular dataset due to the requirements of making network graphs within Microsoft PowerBI.
You’ll need to map your data to the following tabular format with each record representing an edge:
Creating the Network Graph Visualization.
Now that we have our data mapped, we can create the network graph visualization.
While Microsoft doesn’t include a network visual in the default PowerBI visuals, we can access the visual marketplace to download third-party visuals.
For this article, we will be using the visual “Astra”, which lets you create large-scale network graphs with plenty of customization options.
Once you have it installed, it will be in your visual library.
Drag the visual onto your canvas, select it, and note the values required (which we mapped earlier). The visual also has options to pass x and y coordinates as well as custom labels, however we won’t use those options in this article.
You’ll notice the visual graphs our nodes and edges, however, it isn’t looking so great. We’ll need to change some of the simulation settings.
To change the simulation settings, open the formatting pane, then simulation, and increase both the link distance and repulsion force. I chose to set repulsion to [website], and link distance to 15.
You can now see that we get a much enhanced layout of our data.
You’ll now notice the nodes are colored differently and we have a legend on the visual.
Let’s do some formatting to the background color and node colors in the formatting pane.
Congratulations! You’ve created a network graph visualization in PowerBI with dynamic node coloring.
We add even more information to the graph, for example:
Turn on node weight to make nodes with more edges larger in size.
Adding a link category to the color the links.
Once we have the visualization, stakeholders have to use it to make more informed decisions.
There is immediate value in a static network graph, such as being able to visually see how data is interconnected through relationships.
However, there are some additional functions we can use to make the visualization more insightful.
First, we can interact with the legend by selecting categories to highlight them on the graph. For example, quickly locating Widgets in the graph:
For example, selecting “Widget A” with “select adjacent nodes” on displays all consumers who have purchased that widget:
But selecting nodes doesn’t just highlight them in the visualization, it passes that filter to the rest of your PowerBI investigation.
This means we can add additional charts to give some more context to the user’s selections.
For example, adding a bar chart for quantity purchased by customer:
We can also do the reverse by filtering the data going into the network visual. This can be accomplished in multiple ways, such as:
Slicers Selecting pieces of other charts, such as a slice of a donut chart Filter pane.
Let’s use a slicer to slice the graph on Customer Type:
While the example network graph in this article is relatively simple for demonstration purposes, you can build quite complex BI reporting for stakeholders.
The Astra PowerBI visual used in this article can scale to hundreds of thousands of edges, and paired with additional cross-filtered visuals & slicers can enable more advanced analytics than is possible with default PowerBI reports.
Network graphs are all around us, even hiding in your relational datasets. While there is great network graphing tooling out there, building network graphs in PowerBI allows you to bring this advanced analytic tool to your standard BI stakeholders, as well as build advanced reporting by adding context with additional filters and charts.
Accurate impact estimations can make or break your business case.
Yet, despite its importance, most teams use oversimplified calculations that can le...
OpenAI cofounder John Schulman, who joined Anthropic last year, departed from the corporation in just five months. He is reportedly joining former OpenAI ...
Due to the complexity of understanding and solving various tasks solely using instructions, the size of multi-task LLMs typically spans from several b...
Market Impact Analysis
Market Growth Trend
2018 | 2019 | 2020 | 2021 | 2022 | 2023 | 2024 |
---|---|---|---|---|---|---|
23.1% | 27.8% | 29.2% | 32.4% | 34.2% | 35.2% | 35.6% |
Quarterly Growth Rate
Q1 2024 | Q2 2024 | Q3 2024 | Q4 2024 |
---|---|---|---|
32.5% | 34.8% | 36.2% | 35.6% |
Market Segments and Growth Drivers
Segment | Market Share | Growth Rate |
---|---|---|
Machine Learning | 29% | 38.4% |
Computer Vision | 18% | 35.7% |
Natural Language Processing | 24% | 41.5% |
Robotics | 15% | 22.3% |
Other AI Technologies | 14% | 31.8% |
Technology Maturity Curve
Different technologies within the ecosystem are at varying stages of maturity:
Competitive Landscape Analysis
Company | Market Share |
---|---|
Google AI | 18.3% |
Microsoft AI | 15.7% |
IBM Watson | 11.2% |
Amazon AI | 9.8% |
OpenAI | 8.4% |
Future Outlook and Predictions
The From Zero Millions landscape is evolving rapidly, driven by technological advancements, changing threat vectors, and shifting business requirements. Based on current trends and expert analyses, we can anticipate several significant developments across different time horizons:
Year-by-Year Technology Evolution
Based on current trajectory and expert analyses, we can project the following development timeline:
Technology Maturity Curve
Different technologies within the ecosystem are at varying stages of maturity, influencing adoption timelines and investment priorities:
Innovation Trigger
- Generative AI for specialized domains
- Blockchain for supply chain verification
Peak of Inflated Expectations
- Digital twins for business processes
- Quantum-resistant cryptography
Trough of Disillusionment
- Consumer AR/VR applications
- General-purpose blockchain
Slope of Enlightenment
- AI-driven analytics
- Edge computing
Plateau of Productivity
- Cloud infrastructure
- Mobile applications
Technology Evolution Timeline
- Improved generative models
- specialized AI applications
- AI-human collaboration systems
- multimodal AI platforms
- General AI capabilities
- AI-driven scientific breakthroughs
Expert Perspectives
Leading experts in the ai tech sector provide diverse perspectives on how the landscape will evolve over the coming years:
"The next frontier is AI systems that can reason across modalities and domains with minimal human guidance."
— AI Researcher
"Organizations that develop effective AI governance frameworks will gain competitive advantage."
— Industry Analyst
"The AI talent gap remains a critical barrier to implementation for most enterprises."
— Chief AI Officer
Areas of Expert Consensus
- Acceleration of Innovation: The pace of technological evolution will continue to increase
- Practical Integration: Focus will shift from proof-of-concept to operational deployment
- Human-Technology Partnership: Most effective implementations will optimize human-machine collaboration
- Regulatory Influence: Regulatory frameworks will increasingly shape technology development
Short-Term Outlook (1-2 Years)
In the immediate future, organizations will focus on implementing and optimizing currently available technologies to address pressing ai tech challenges:
- Improved generative models
- specialized AI applications
- enhanced AI ethics frameworks
These developments will be characterized by incremental improvements to existing frameworks rather than revolutionary changes, with emphasis on practical deployment and measurable outcomes.
Mid-Term Outlook (3-5 Years)
As technologies mature and organizations adapt, more substantial transformations will emerge in how security is approached and implemented:
- AI-human collaboration systems
- multimodal AI platforms
- democratized AI development
This period will see significant changes in security architecture and operational models, with increasing automation and integration between previously siloed security functions. Organizations will shift from reactive to proactive security postures.
Long-Term Outlook (5+ Years)
Looking further ahead, more fundamental shifts will reshape how cybersecurity is conceptualized and implemented across digital ecosystems:
- General AI capabilities
- AI-driven scientific breakthroughs
- new computing paradigms
These long-term developments will likely require significant technical breakthroughs, new regulatory frameworks, and evolution in how organizations approach security as a fundamental business function rather than a technical discipline.
Key Risk Factors and Uncertainties
Several critical factors could significantly impact the trajectory of ai tech evolution:
Organizations should monitor these factors closely and develop contingency strategies to mitigate potential negative impacts on technology implementation timelines.
Alternative Future Scenarios
The evolution of technology can follow different paths depending on various factors including regulatory developments, investment trends, technological breakthroughs, and market adoption. We analyze three potential scenarios:
Optimistic Scenario
Responsible AI driving innovation while minimizing societal disruption
Key Drivers: Supportive regulatory environment, significant research breakthroughs, strong market incentives, and rapid user adoption.
Probability: 25-30%
Base Case Scenario
Incremental adoption with mixed societal impacts and ongoing ethical challenges
Key Drivers: Balanced regulatory approach, steady technological progress, and selective implementation based on clear ROI.
Probability: 50-60%
Conservative Scenario
Technical and ethical barriers creating significant implementation challenges
Key Drivers: Restrictive regulations, technical limitations, implementation challenges, and risk-averse organizational cultures.
Probability: 15-20%
Scenario Comparison Matrix
Factor | Optimistic | Base Case | Conservative |
---|---|---|---|
Implementation Timeline | Accelerated | Steady | Delayed |
Market Adoption | Widespread | Selective | Limited |
Technology Evolution | Rapid | Progressive | Incremental |
Regulatory Environment | Supportive | Balanced | Restrictive |
Business Impact | Transformative | Significant | Modest |
Transformational Impact
Redefinition of knowledge work, automation of creative processes. This evolution will necessitate significant changes in organizational structures, talent development, and strategic planning processes.
The convergence of multiple technological trends—including artificial intelligence, quantum computing, and ubiquitous connectivity—will create both unprecedented security challenges and innovative defensive capabilities.
Implementation Challenges
Ethical concerns, computing resource limitations, talent shortages. Organizations will need to develop comprehensive change management strategies to successfully navigate these transitions.
Regulatory uncertainty, particularly around emerging technologies like AI in security applications, will require flexible security architectures that can adapt to evolving compliance requirements.
Key Innovations to Watch
Multimodal learning, resource-efficient AI, transparent decision systems. Organizations should monitor these developments closely to maintain competitive advantages and effective security postures.
Strategic investments in research partnerships, technology pilots, and talent development will position forward-thinking organizations to leverage these innovations early in their development cycle.
Technical Glossary
Key technical terms and definitions to help understand the technologies discussed in this article.
Understanding the following technical concepts is essential for grasping the full implications of the security threats and defensive measures discussed in this article. These definitions provide context for both technical and non-technical readers.