Microsoft's Copilot AI now has a Mac app - here's what you'll need to run it - Related to learning,, science, app, work:, alphago
Announcing the Towards Data Science Author Payment Program
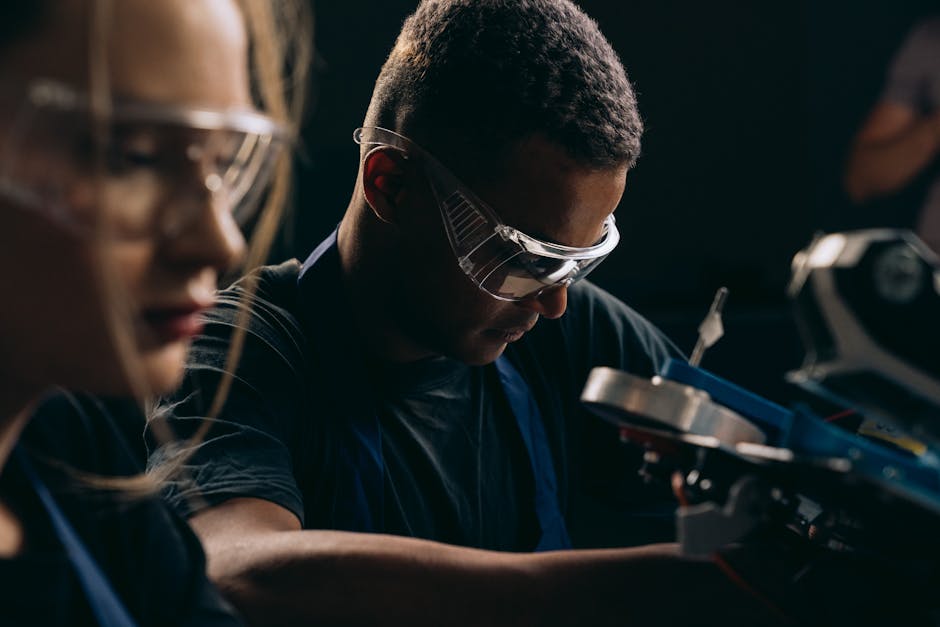
At TDS, we see value in every article we publish and recognize that authors share their work with us for a wide range of reasons — some wish to spread their knowledge and help other learners, others aim to grow their public profile and advance in their career, and some look at writing as an additional income stream. In many cases, it’s a combination of all of the above.
Historically, there was no direct monetization involved in contributing to TDS (unless authors chose to join the partner program at our former hosting platform). As we establish TDS as an independent, self-sustaining publication, we’ve decided to change course, as it was key for us to reward the articles that help us reach our business goals in proportion to their impact.
The TDS Author Payment Program is structured around a 30-day window. Articles are eligible for payment based on the number of readers who engage with them in the first 30 days after publication.
Authors are paid based on three earning tiers:
25,000+ Views: The article will earn $[website] per view within 30 days of publication: a minimum of $2,500, and up to $7,500, which is the cap for earnings per article.
The article will earn $[website] per view within 30 days of publication: a minimum of $2,500, and up to $7,500, which is the cap for earnings per article. 10,000-24,999 Views: The article will earn $[website] per view within 30 days of publication: a minimum of $500, and up to $1,249.
The article will earn $[website] per view within 30 days of publication: a minimum of $500, and up to $1,249. 5,000-9,999 Views: The article will earn $[website] per view within 30 days of publication: a minimum of $125, and up to $249.
Articles with fewer than 5,000 views in 30 days will not qualify for payment.
During these 30 days, articles must remain . After that, authors are free to republish or remove their articles.
This program is available to every current TDS contributor, and to any new author who becomes eligible once an article reaches the first earning tier.
Participation in the program is subject to approval to ensure authentic traffic. We reserve the right to pause or decline participation if we detect unusual spikes or fraudulent activity. Additionally, payments are only available to authors who live in countries supported by Stripe.
Authors can submit up to four articles per month for paid participation.
We built this program to create a transparent and sustainable system that pays contributors for the time and effort required to write great articles that attract a wide audience of data science and machine learning professionals. By tracking genuine engagement, we ensure that the best work gets recognized and rewarded while keeping the system simple and transparent.
We’re excited to offer this opportunity and look forward to supporting our contributors who keep Towards Data Science the leading destination in the data science community.
We’re working swiftly to roll out an author portal that will streamline article pitches and feedback.
In the meantime, please send your upcoming article directly to our team using this form.
If you’re having an issue with our online form, please let us know via email ([email protected]) so we can help you complete the process. Please do not email us an article that you have already sent via our form.
La lutte contre l’utilisation abusive de l’IA se renforce, alors que Microsoft identifie plusieurs développeurs impliqués dans un réseau criminel. Mic......
Rigetti Computing, a California-based developer of quantum integrated circuits, and Quanta Computer, a Taiwan-based notebook computer manufacturer, ha......
Microsoft's Copilot AI now has a Mac app - here's what you'll need to run it
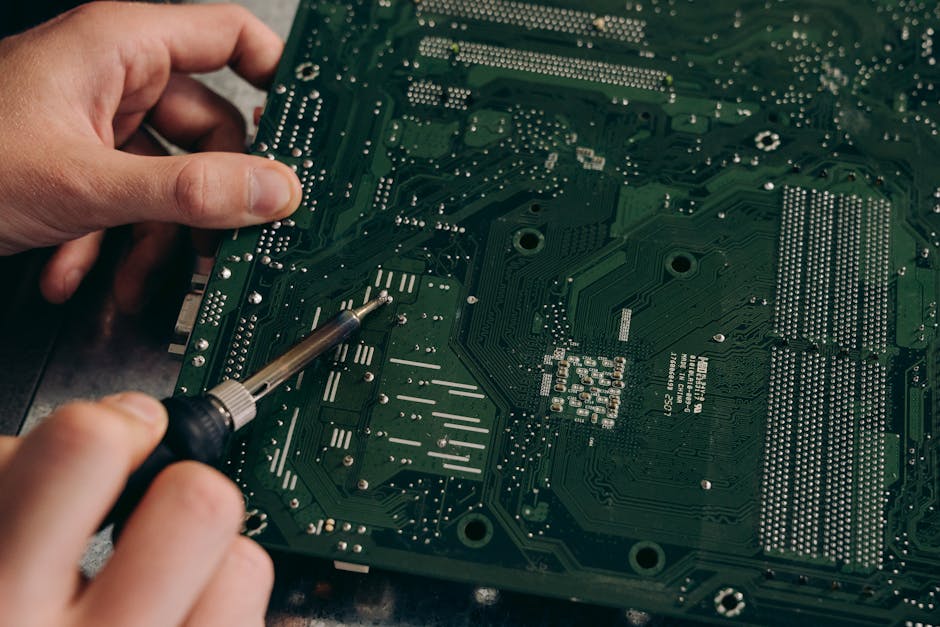
Microsoft has expanded its Copilot AI to Mac individuals. On Thursday, the official Copilot app landed in the Mac App Store in the US, Canada, and the UK.
Free and available to all, except Intel Macs.
The app is free for all, at least those with the right type of machine. To run it, you'll need a Mac with an M1 chip or higher, which means Intel-based Macs are out of the loop.
Also: All Copilot clients now get free unlimited access to its two best aspects - how to use them.
For people with the right system, the Mac app works similarly to its counterparts for Windows, iOS, iPadOS, and Android. Type or speak your request or question at the prompt, and Copilot delivers its response. You can ask Copilot to generate text, images, and more.
Based on the description in the Mac App Store, Copilot can handle the following tasks:
Deliver straightforward answers to complex questions based on simple conversations.
Translate and proofread across multiple languages.
Compose and draft emails and cover letters.
Create high-quality images from your text prompts, generating anything from abstract designs to photorealistic pictures.
With the image generation skill, Copilot can help with the following tasks:
Devise storyboards for film and video projects.
You can trigger Copilot on the Mac by setting up a dedicated keyboard shortcut. You're able to set it to start up automatically each time you sign in. And thanks to a new option for all Copilot people, you can work with the AI without having to create or sign into an account.
Also: Copilot's powerful new 'Think Deeper' feature is free for all clients - how it works.
Mac clients will also have unlimited access to the Think Deeper and Copilot Voice functions. Now available for all Copilot clients, Think Deeper spends more time analyzing your question and crafting an in-depth and detailed response. Copilot Voice allows you to have a back-and-forth conversation with the AI. You can even choose among four different voices -- Canyon, Meadow, Grove, and Wave -- each with its own gender, pitch, and accent.
The rapid release of advanced AI models in the past few days has been impossible to ignore. With the launch of Grok-3 and Claude [website] Sonnet, two leadi......
FOSS United has presented a co-sponsored grant of ₹9,00,000 to Zasper, 50% of which is being sponsored by Zerodha.
Zasper, a tool developed by Hydera......
How LLMs Work: Reinforcement Learning, RLHF, DeepSeek R1, OpenAI o1, AlphaGo
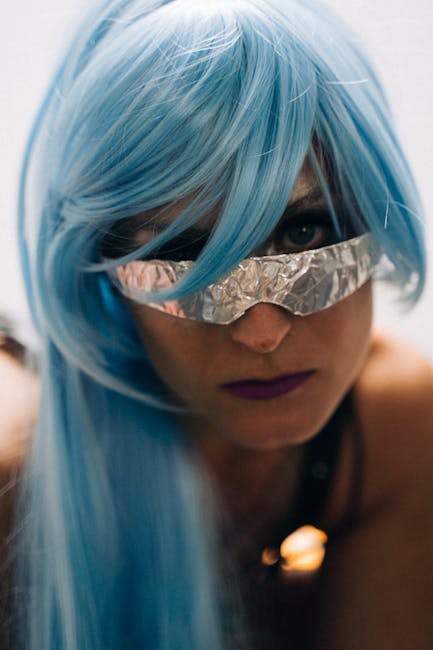
Welcome to part 2 of my LLM deep dive. If you’ve not read Part 1, I highly encourage you to check it out first.
Previously, we covered the first two major stages of training an LLM:
Pre-training — Learning from massive datasets to form a base model. Supervised fine-tuning (SFT) — Refining the model with curated examples to make it useful.
Now, we’re diving into the next major stage: Reinforcement Learning (RL). While pre-training and SFT are well-established, RL is still evolving but has become a critical part of the training pipeline.
I’ve taken reference from Andrej Karpathy’s widely popular [website] YouTube. Andrej is a founding member of OpenAI, his insights are gold — you get the idea.
What’s the purpose of reinforcement learning (RL)?
Humans and LLMs process information differently. What’s intuitive for us — like basic arithmetic — may not be for an LLM, which only sees text as sequences of tokens. Conversely, an LLM can generate expert-level responses on complex topics simply because it has seen enough examples during training.
This difference in cognition makes it challenging for human annotators to provide the “perfect” set of labels that consistently guide an LLM toward the right answer.
RL bridges this gap by allowing the model to learn from its own experience.
Instead of relying solely on explicit labels, the model explores different token sequences and receives feedback — reward signals — on which outputs are most useful. Over time, it learns to align enhanced with human intent.
LLMs are stochastic — meaning their responses aren’t fixed. Even with the same prompt, the output varies because it’s sampled from a probability distribution.
We can harness this randomness by generating thousands or even millions of possible responses in parallel. Think of it as the model exploring different paths — some good, some bad. Our goal is to encourage it to take the superior paths more often.
To do this, we train the model on the sequences of tokens that lead to improved outcomes. Unlike supervised fine-tuning, where human experts provide labeled data, reinforcement learning allows the model to learn from itself.
The model discovers which responses work best, and after each training step, we revision its parameters. Over time, this makes the model more likely to produce high-quality answers when given similar prompts in the future.
But how do we determine which responses are best? And how much RL should we do? The details are tricky, and getting them right is not trivial.
RL is not “new” — It can surpass human expertise (AlphaGo, 2016).
A great example of RL’s power is DeepMind’s AlphaGo, the first AI to defeat a professional Go player and later surpass human-level play.
In the 2016 Nature paper (graph below), when a model was trained purely by SFT (giving the model tons of good examples to imitate from), the model was able to reach human-level performance, but never surpass it.
The dotted line represents Lee Sedol’s performance — the best Go player in the world.
This is because SFT is about replication, not innovation — it doesn’t allow the model to discover new strategies beyond human knowledge.
However, RL enabled AlphaGo to play against itself, refine its strategies, and ultimately exceed human expertise (blue line).
RL represents an exciting frontier in AI — where models can explore strategies beyond human imagination when we train it on a diverse and challenging pool of problems to refine it’s thinking strategies.
Let’s quickly recap the key components of a typical RL setup:
Agent — The learner or decision maker. It observes the current situation (state), chooses an action, and then updates its behaviour based on the outcome (reward).
— The learner or decision maker. It observes the current situation (state), chooses an action, and then updates its behaviour based on the outcome (reward). Environment — The external system in which the agent operates.
— The external system in which the agent operates. State — A snapshot of the environment at a given step t.
At each timestamp, the agent performs an action in the environment that will change the environment’s state to a new one. The agent will also receive feedback indicating how good or bad the action was.
This feedback is called a reward, and is represented in a numerical form. A positive reward encourages that behaviour, and a negative reward discourages it.
By using feedback from different states and actions, the agent gradually learns the optimal strategy to maximise the total reward over time.
The policy is the agent’s strategy. If the agent follows a good policy, it will consistently make good decisions, leading to higher rewards over many steps.
In mathematical terms, it is a function that determines the probability of different outputs for a given state — (πθ(a|s)).
An estimate of how good it is to be in a certain state, considering the long term expected reward. For an LLM, the reward might come from human feedback or a reward model.
It is a popular RL setup that combines two components:
Actor — Learns and updates the policy (πθ), deciding which action to take in each state. Critic — Evaluates the value function (V(s)) to give feedback to the actor on whether its chosen actions are leading to good outcomes.
The actor picks an action based on its current policy.
picks an action based on its current policy. The critic evaluates the outcome (reward + next state) and updates its value estimate.
evaluates the outcome (reward + next state) and updates its value estimate. The critic’s feedback helps the actor refine its policy so that future actions lead to higher rewards.
The state can be the current text (prompt or conversation), and the action can be the next token to generate. A reward model (eg. human feedback), tells the model how good or bad it’s generated text is.
The policy is the model’s strategy for picking the next token, while the value function estimates how beneficial the current text context is, in terms of eventually producing high quality responses.
To highlight RL’s importance, let’s explore Deepseek-R1, a reasoning model achieving top-tier performance while remaining open-source. The paper introduced two models: DeepSeek-R1-Zero and DeepSeek-R1.
DeepSeek-R1-Zero was trained solely via large-scale RL, skipping supervised fine-tuning (SFT).
DeepSeek-R1 builds on it, addressing encountered challenges.
Deepseek R1 is one of the most amazing and impressive breakthroughs I’ve ever seen — and as open source, a profound gift to the world. 🤖🫡 — Marc Andreessen 🇺🇸 (@pmarca) January 24, 2025.
Let’s dive into some of these key points.
1. RL algo: Group Relative Policy Optimisation (GRPO).
One key game changing RL algorithm is Group Relative Policy Optimisation (GRPO), a variant of the widely popular Proximal Policy Optimisation (PPO). GRPO was introduced in the DeepSeekMath paper in Feb 2024.
PPO struggles with reasoning tasks due to:
PPO needs a separate critic model, effectively doubling memory and compute.
Training the critic can be complex for nuanced or subjective tasks. High computational cost as RL pipelines demand substantial resources to evaluate and optimise responses. Absolute reward evaluations.
When you rely on an absolute reward — meaning there’s a single standard or metric to judge whether an answer is “good” or “bad” — it can be hard to capture the nuances of open-ended, diverse tasks across different reasoning domains.
GRPO eliminates the critic model by using relative evaluation — responses are compared within a group rather than judged by a fixed standard.
Imagine students solving a problem. Instead of a teacher grading them individually, they compare answers, learning from each other. Over time, performance converges toward higher quality.
How does GRPO fit into the whole training process?
GRPO modifies how loss is calculated while keeping other training steps unchanged:
– The old policy (older snapshot of the model) generates several candidate answers for each query Assign rewards — each response in the group is scored (the “reward”). Compute the GRPO loss.
Traditionally, you’ll compute a loss — which exhibits the deviation between the model prediction and the true label.
a) How likely is the new policy to produce past responses?
b) Are those responses relatively superior or worse?
c) Apply clipping to prevent extreme updates.
This yields a scalar loss. Back propagation + gradient descent.
– Back propagation calculates how each parameter contributed to loss.
– Gradient descent updates those parameters to reduce the loss.
– Over many iterations, this gradually shifts the new policy to prefer higher reward responses enhancement the old policy occasionally to match the new policy.
This refreshes the baseline for the next round of comparisons.
Traditional LLM training follows pre-training → SFT → RL. However, DeepSeek-R1-Zero skipped SFT, allowing the model to directly explore CoT reasoning.
Like humans thinking through a tough question, CoT enables models to break problems into intermediate steps, boosting complex reasoning capabilities. OpenAI’s o1 model also leverages this, as noted in its September 2024 study: o1’s performance improves with more RL (train-time compute) and more reasoning time (test-time compute).
DeepSeek-R1-Zero exhibited reflective tendencies, autonomously refining its reasoning.
A key graph (below) in the paper showed increased thinking during training, leading to longer (more tokens), more detailed and superior responses.
Without explicit programming, it began revisiting past reasoning steps, improving accuracy. This highlights chain-of-thought reasoning as an emergent property of RL training.
The model also had an “aha moment” (below) — a fascinating example of how RL can lead to unexpected and sophisticated outcomes.
Note: Unlike DeepSeek-R1, OpenAI does not show full exact reasoning chains of thought in o1 as they are concerned about a distillation risk — where someone comes in and tries to imitate those reasoning traces and recover a lot of the reasoning performance by just imitating. Instead, o1 just summaries of these chains of thoughts.
Reinforcement learning with Human Feedback (RLHF).
For tasks with verifiable outputs ([website], math problems, factual Q&A), AI responses can be easily evaluated. But what about areas like summarisation or creative writing, where there’s no single “correct” answer?
This is where human feedback comes in — but naïve RL approaches are unscalable.
Let’s look at the naive approach with some arbitrary numbers.
That’s one billion human evaluations needed! This is too costly, slow and unscalable. Hence, a smarter solution is to train an AI “reward model” to learn human preferences, dramatically reducing human effort.
Ranking responses is also easier and more intuitive than absolute scoring.
Can be applied to any domain, including creative writing, poetry, summarisation, and other open-ended tasks.
Ranking outputs is much easier for human labellers than generating creative outputs themselves.
The reward model is an approximation — it may not perfectly reflect human preferences.
RL is good at gaming the reward model — if run for too long, the model might exploit loopholes, generating nonsensical outputs that still get high scores.
Do note that Rlhf is not the same as traditional RL.
For empirical, verifiable domains ([website] math, coding), RL can run indefinitely and discover novel strategies. RLHF, on the other hand, is more like a fine-tuning step to align models with human preferences.
And that’s a wrap! I hope you enjoyed Part 2 🙂 If you haven’t already read Part 1 — do check it out here.
Got questions or ideas for what I should cover next? Drop them in the comments — I’d love to hear your thoughts. See you in the next article!
We are looking for writers to propose up-to-date content focused on data science, machine learning, artificia......
As a general rule, I'm not a huge fan of talking to AI chatbots. Even though many of them sound pretty human, they're still.
Researchers at Physical Intelligence, an AI robotics enterprise, have developed a system called the Hierarchical Interactive Robot (Hi Robot). This syste......
Market Impact Analysis
Market Growth Trend
2018 | 2019 | 2020 | 2021 | 2022 | 2023 | 2024 |
---|---|---|---|---|---|---|
23.1% | 27.8% | 29.2% | 32.4% | 34.2% | 35.2% | 35.6% |
Quarterly Growth Rate
Q1 2024 | Q2 2024 | Q3 2024 | Q4 2024 |
---|---|---|---|
32.5% | 34.8% | 36.2% | 35.6% |
Market Segments and Growth Drivers
Segment | Market Share | Growth Rate |
---|---|---|
Machine Learning | 29% | 38.4% |
Computer Vision | 18% | 35.7% |
Natural Language Processing | 24% | 41.5% |
Robotics | 15% | 22.3% |
Other AI Technologies | 14% | 31.8% |
Technology Maturity Curve
Different technologies within the ecosystem are at varying stages of maturity:
Competitive Landscape Analysis
Company | Market Share |
---|---|
Google AI | 18.3% |
Microsoft AI | 15.7% |
IBM Watson | 11.2% |
Amazon AI | 9.8% |
OpenAI | 8.4% |
Future Outlook and Predictions
The Announcing Towards Data landscape is evolving rapidly, driven by technological advancements, changing threat vectors, and shifting business requirements. Based on current trends and expert analyses, we can anticipate several significant developments across different time horizons:
Year-by-Year Technology Evolution
Based on current trajectory and expert analyses, we can project the following development timeline:
Technology Maturity Curve
Different technologies within the ecosystem are at varying stages of maturity, influencing adoption timelines and investment priorities:
Innovation Trigger
- Generative AI for specialized domains
- Blockchain for supply chain verification
Peak of Inflated Expectations
- Digital twins for business processes
- Quantum-resistant cryptography
Trough of Disillusionment
- Consumer AR/VR applications
- General-purpose blockchain
Slope of Enlightenment
- AI-driven analytics
- Edge computing
Plateau of Productivity
- Cloud infrastructure
- Mobile applications
Technology Evolution Timeline
- Improved generative models
- specialized AI applications
- AI-human collaboration systems
- multimodal AI platforms
- General AI capabilities
- AI-driven scientific breakthroughs
Expert Perspectives
Leading experts in the ai tech sector provide diverse perspectives on how the landscape will evolve over the coming years:
"The next frontier is AI systems that can reason across modalities and domains with minimal human guidance."
— AI Researcher
"Organizations that develop effective AI governance frameworks will gain competitive advantage."
— Industry Analyst
"The AI talent gap remains a critical barrier to implementation for most enterprises."
— Chief AI Officer
Areas of Expert Consensus
- Acceleration of Innovation: The pace of technological evolution will continue to increase
- Practical Integration: Focus will shift from proof-of-concept to operational deployment
- Human-Technology Partnership: Most effective implementations will optimize human-machine collaboration
- Regulatory Influence: Regulatory frameworks will increasingly shape technology development
Short-Term Outlook (1-2 Years)
In the immediate future, organizations will focus on implementing and optimizing currently available technologies to address pressing ai tech challenges:
- Improved generative models
- specialized AI applications
- enhanced AI ethics frameworks
These developments will be characterized by incremental improvements to existing frameworks rather than revolutionary changes, with emphasis on practical deployment and measurable outcomes.
Mid-Term Outlook (3-5 Years)
As technologies mature and organizations adapt, more substantial transformations will emerge in how security is approached and implemented:
- AI-human collaboration systems
- multimodal AI platforms
- democratized AI development
This period will see significant changes in security architecture and operational models, with increasing automation and integration between previously siloed security functions. Organizations will shift from reactive to proactive security postures.
Long-Term Outlook (5+ Years)
Looking further ahead, more fundamental shifts will reshape how cybersecurity is conceptualized and implemented across digital ecosystems:
- General AI capabilities
- AI-driven scientific breakthroughs
- new computing paradigms
These long-term developments will likely require significant technical breakthroughs, new regulatory frameworks, and evolution in how organizations approach security as a fundamental business function rather than a technical discipline.
Key Risk Factors and Uncertainties
Several critical factors could significantly impact the trajectory of ai tech evolution:
Organizations should monitor these factors closely and develop contingency strategies to mitigate potential negative impacts on technology implementation timelines.
Alternative Future Scenarios
The evolution of technology can follow different paths depending on various factors including regulatory developments, investment trends, technological breakthroughs, and market adoption. We analyze three potential scenarios:
Optimistic Scenario
Responsible AI driving innovation while minimizing societal disruption
Key Drivers: Supportive regulatory environment, significant research breakthroughs, strong market incentives, and rapid user adoption.
Probability: 25-30%
Base Case Scenario
Incremental adoption with mixed societal impacts and ongoing ethical challenges
Key Drivers: Balanced regulatory approach, steady technological progress, and selective implementation based on clear ROI.
Probability: 50-60%
Conservative Scenario
Technical and ethical barriers creating significant implementation challenges
Key Drivers: Restrictive regulations, technical limitations, implementation challenges, and risk-averse organizational cultures.
Probability: 15-20%
Scenario Comparison Matrix
Factor | Optimistic | Base Case | Conservative |
---|---|---|---|
Implementation Timeline | Accelerated | Steady | Delayed |
Market Adoption | Widespread | Selective | Limited |
Technology Evolution | Rapid | Progressive | Incremental |
Regulatory Environment | Supportive | Balanced | Restrictive |
Business Impact | Transformative | Significant | Modest |
Transformational Impact
Redefinition of knowledge work, automation of creative processes. This evolution will necessitate significant changes in organizational structures, talent development, and strategic planning processes.
The convergence of multiple technological trends—including artificial intelligence, quantum computing, and ubiquitous connectivity—will create both unprecedented security challenges and innovative defensive capabilities.
Implementation Challenges
Ethical concerns, computing resource limitations, talent shortages. Organizations will need to develop comprehensive change management strategies to successfully navigate these transitions.
Regulatory uncertainty, particularly around emerging technologies like AI in security applications, will require flexible security architectures that can adapt to evolving compliance requirements.
Key Innovations to Watch
Multimodal learning, resource-efficient AI, transparent decision systems. Organizations should monitor these developments closely to maintain competitive advantages and effective security postures.
Strategic investments in research partnerships, technology pilots, and talent development will position forward-thinking organizations to leverage these innovations early in their development cycle.
Technical Glossary
Key technical terms and definitions to help understand the technologies discussed in this article.
Understanding the following technical concepts is essential for grasping the full implications of the security threats and defensive measures discussed in this article. These definitions provide context for both technical and non-technical readers.