Nanoprinter turns Meta’s AI predictions into potentially game-changing materials - Related to materials, training, game-changing, predictions, ai
Advances in private training for production on-device language models
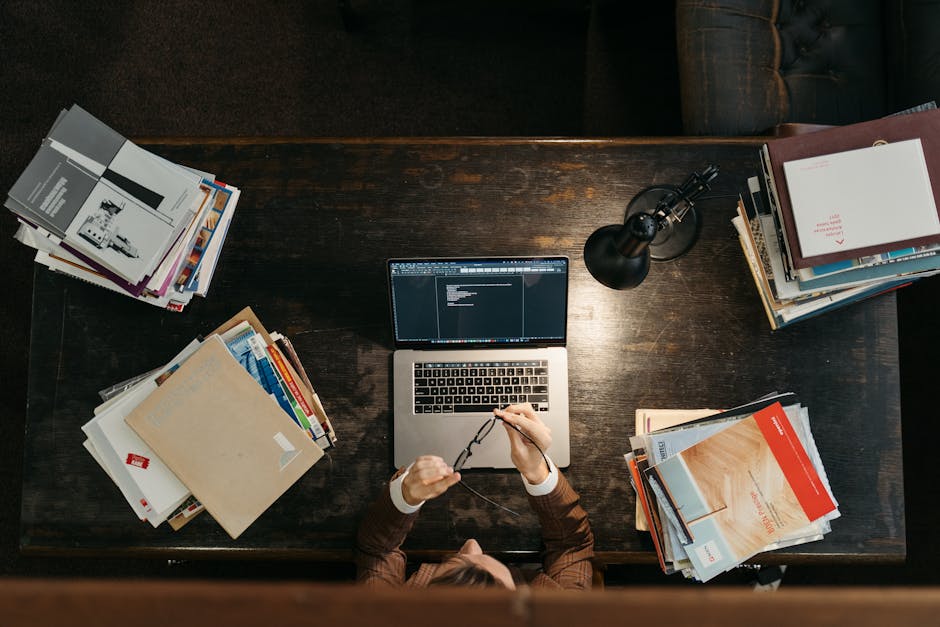
Language models (LMs) trained to predict the next word given input text are the key technology for many applications [1, 2]. In Gboard, LMs are used to improve customers’ typing experience by supporting functions like next word prediction (NWP), Smart Compose, smart completion and suggestion. Slide to type, and proofread. Deploying models on customers’ devices rather than enterprise servers has advantages like lower latency and superior privacy for model usage. While training on-device models directly from user data effectively improves the utility performance for applications such as NWP and smart text selection, protecting the privacy of user data for model training is critical.
Gboard capabilities powered by on-device language models.
Additionally, in this blog we discuss how years of research advances now power the private training of Gboard LMs, since the proof-of-concept development of federated learning (FL) in 2017 and. Formal differential privacy (DP) guarantees in 2022. FL enables mobile phones to collaboratively learn a model while keeping all the training data on device, and. DP provides a quantifiable measure of data anonymization. Formally, DP is often characterized by (ε, δ) with smaller values representing stronger guarantees. Machine learning (ML) models are considered to have reasonable DP guarantees for ε=10 and strong DP guarantees for ε=1 when δ is small.
As of today, all NWP neural network LMs in Gboard are trained with FL with formal DP guarantees. And all future launches of Gboard LMs trained on user data require DP. These 30+ Gboard on-device LMs are launched in 7+ languages and 15+ countries, and satisfy (ɛ, δ)-DP guarantees of small δ of 10-10 and ɛ between and To the best of our knowledge, this is the largest known deployment of user-level DP in production at Google or anywhere, and the first time a strong DP guarantee of ɛ < 1 is announced for models trained directly on user data.
Privacy principles and practices in Gboard.
In “Private Federated Learning in Gboard”, we discussed how different privacy principles are currently reflected in production models, including:
Transparency and user control: We provide disclosure of what data is used. What purpose it is used for, how it is processed in various channels, and how Gboard clients can easily configure the data usage in learning models.
Data minimization: FL immediately aggregates only focused updates that improve a specific model. Secure aggregation (SecAgg) is an encryption method to further guarantee that only aggregated results of the ephemeral updates can be accessed.
Data anonymization: DP is applied by the server to prevent models from memorizing the unique information in individual user’s training data.
Auditability and verifiability: We have made public the key algorithmic approaches and privacy accounting in open-sourced code (TFF aggregator, TFP DPQuery. DP accounting, and FL system).
In recent years, FL has become the default method for training Gboard on-device LMs from user data. In 2020, a DP mechanism that clips and adds noise to model updates was used to prevent memorization for training the Spanish LM in Spain. Which satisfies finite DP guarantees (Tier 3 described in “How to DP-fy ML“ guide). In 2022, with the help of the DP-Follow-The-Regularized-Leader (DP-FTRL) algorithm, the Spanish LM became the first production neural network trained directly on user data revealed with a formal DP guarantee of (ε, δ=10-10)-DP (equivalent to the reported ρ zero-Concentrated-Differential-Privacy), and therefore satisfies reasonable privacy guarantees (Tier 2).
Differential privacy by default in federated learning.
In “Federated Learning of Gboard Language Models with Differential Privacy”. We showcased that all the NWP neural network LMs in Gboard have DP guarantees, and all future launches of Gboard LMs trained on user data require DP guarantees. DP is enabled in FL by applying the following practices:
Pre-train the model with the multilingual C4 dataset.
Via simulation experiments on public datasets. Find a large DP-noise-to-signal ratio that allows for high utility. Increasing the number of clients contributing to one round of model modification improves privacy while keeping the noise ratio fixed for good utility, up to the point the DP target is met, or the maximum allowed by the system and the size of the population.
Configure the parameter to restrict the frequency each client can contribute (, once every few days) based on computation budget and estimated population in the FL system.
Run DP-FTRL training with limits on the magnitude of per-device updates chosen either via adaptive clipping, or fixed based on experience.
SecAgg can be additionally applied by adopting the advances in improving computation and. Communication for scales and sensitivity.
Federated learning with differential privacy and (SecAgg).
In relation to this, the DP guarantees of launched Gboard NWP LMs are visualized in the barplot below. The x-axis reveals LMs labeled by language-locale and trained on corresponding populations; the y-axis reveals the ε value when δ is fixed to a small value of 10-10 for (ε. Δ)-DP (lower is superior). The utility of these models are either significantly superior than previous non-neural models in production, or comparable with previous LMs without DP. Measured based on user-interactions metrics during A/B testing. For example, by applying the best practices, the DP guarantee of the Spanish model in Spain is improved from ε to ε SecAgg is additionally used for training the Spanish model in Spain and. English model in the US. More details of the DP guarantees are reported in the appendix following the guidelines outlined in “How to DP-fy ML”.
The ε~10 DP guarantees of many launched LMs are already considered reasonable for ML models in practice. While the journey of DP FL in Gboard continues for improving user typing experience while protecting data privacy. We are excited to announce that, for the first time, production LMs of Portuguese in Brazil and Spanish in Latin America are trained and. Launched with a DP guarantee of ε ≤ 1, which satisfies Tier 1 strong privacy guarantees. Specifically, the (ε, δ=10-10)-DP guarantee is achieved by running the advanced Matrix Factorization DP-FTRL (MF-DP-FTRL) algorithm, with 12,000+ devices participating in every training round of server model improvement larger than the common setting of 6500+ devices, and. A carefully configured policy to restrict each client to at most participate twice in the total 2000 rounds of training in 14 days in the large Portuguese user population of Brazil. Using a similar setting, the es-US Spanish LM was trained in a large population combining multiple countries in Latin America to achieve (ε, δ=10-10)-DP. The ε ≤ 1 es-US model significantly improved the utility in many countries, and launched in Colombia, Ecuador, Guatemala, Mexico, and Venezuela. For the smaller population in Spain, the DP guarantee of es-ES LM is improved from ε to ε by only replacing DP-FTRL with MF-DP-FTRL without increasing the number of devices participating every round. More technical details are disclosed in the colab for privacy accounting.
DP guarantees for Gboard NWP LMs (the purple bar represents the first es-ES launch of ε; cyan bars represent privacy improvements for models trained with MF-DP-FTRL; tiers are from “How to DP-fy ML“ guide; en-US* and es-ES* are additionally trained with SecAgg).
Our experience implies that DP can be achieved in practice through system algorithm co-design on client participation, and. That both privacy and utility can be strong when populations are large and a large number of devices' contributions are aggregated. Privacy-utility-computation trade-offs can be improved by using public data, the new MF-DP-FTRL algorithm, and tightening accounting. With these techniques, a strong DP guarantee of ε ≤ 1 is possible but still challenging. Active research on empirical privacy auditing [1, 2] implies that DP models are potentially more private than the worst-case DP guarantees imply. While we keep pushing the frontier of algorithms, which dimension of privacy-utility-computation should be prioritized?
We are actively working on all privacy aspects of ML, including extending DP-FTRL to distributed DP and. Improving auditability and verifiability. Trusted Execution Environment opens the opportunity for substantially increasing the model size with verifiable privacy. The recent breakthrough in large LMs (LLMs) motivates us to rethink the usage of public information in private training and more future interactions between LLMs, on-device LMs, and Gboard production.
The authors would like to thank Peter Kairouz, Brendan McMahan, and Daniel Ramage for their early feedback on the blog post itself, Shaofeng Li and. Tom Small for helping with the animated figures, and the teams at Google that helped with algorithm design, infrastructure implementation, and production maintenance. The collaborators below directly contribute to the presented results:
Research and algorithm development: Galen Andrew, Stanislav Chiknavaryan, Christopher A. Choquette-Choo, Arun Ganesh, Peter Kairouz, Ryan McKenna, H. Brendan McMahan, Jesse Rosenstock, Timon Van Overveldt, Keith Rush, Shuang Song, Thomas Steinke, Abhradeep Guha Thakurta, Om Thakkar, and Yuanbo Zhang.
Infrastructure, production and leadership support: Mingqing Chen, Stefan Dierauf, Billy Dou, Hubert Eichner, Zachary Garrett, Jeremy Gillula, Jianpeng Hou, Hui Li, Xu Liu, Wenzhi Mao, Brett McLarnon, Mengchen Pei, Daniel Ramage, Swaroop Ramaswamy, Haicheng Sun, Andreas Terzis, Yun Wang, Shanshan Wu. Yu Xiao, and Shumin Zhai.
In “Domain-specific optimization and diverse evaluation of self-supervised models for histopathology”, we showed that self-supervised...
AI-driven technologies are weaving themselves into the fabric of our daily routines, with the potential to enhance our access to knowledge and boost o...
It’s been a great year for the Dutch startup ecosystem.
Venture capitalists have, so far, invested $ into Netherlands-based early-stage companie...
Dr. Rob’s new AI model promises to cut aircraft design time from months to days
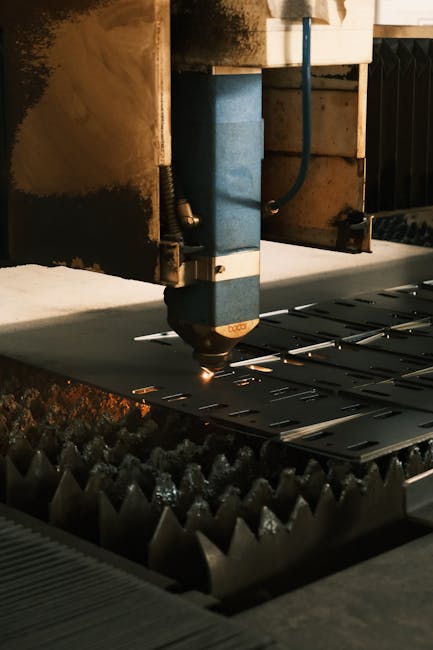
UK startup PhysicsX, founded by former Formula 1 engineering whizz Robin “Dr. Rob” Tuluie, has unveiled an AI tool that could fast-track the time it takes to design a new aircraft from months to just a few days.
Dubbed LGM-Aero. The software creates new designs for aeroplanes. Using advanced algorithms trained on more than 25 million geometries, the model predicts lift, drag, stability, structural stress and other attributes for each shape. It then tailors the design .
PhysicsX stated the AI is the first-ever Large Geometry Model (LGM) for aerospace engineering. A barebones version of the model, , is also accessible free of charge.
“This is a first step in transforming the way engineering is practised in advanced industries [like automotive, aerospace, and manufacturing],” mentioned Tuluie, founder and chairman of PhysicsX.
“Over time. We will bring new capabilities to LGM-Aero and , allowing clients to select powertrains, add controls and further content to reach mature designs in days rather than months or years,” he presented.
Tuluie wasn’t always an entrepreneur. For the first half of his life, he worked alongside Nobel Prize winners as an astrophysicist. Then, at 41, he entered the F1 scene where he devised designs that helped Renault, and later Mercedes, win four Formula One world championships between them.
In 2019. Tuluie founded PhysicsX alongside Jacomo Corbo, a Harvard-educated engineer who ran McKinsey’s AI lab. Together, the duo have assembled a 50-strong team of some of the world’s top minds in data science, AI, and. Machine learning.
PhysicsX, based in London, emerged from stealth in November 2023 with €30mn in funding. The corporation is on a mission to reimagine simulation for science and engineering using AI in sectors such as automotive, aerospace, and manufacturing.
PhysicsX says it is looking to help engineers enhanced anticipate design bottlenecks. Such as the drag of a new aeroplane or car design before they set out on building a physical prototype — saving them time and money. Its software acts like a supercharged wind tunnel for ideas.
“In the same way that large language models understand text, has a vast knowledge of the shapes and structures that are crucial to aerospace engineering,” explained Corbo.
“The technology can optimise across multiple types of physics in seconds, many orders of magnitude faster than numerical simulation. And at the same level of accuracy.”.
Corbo called LGM-Aero “an key stepping stone” towards developing physics foundation models. These are AI systems designed to simulate and solve complex physical problems by learning patterns from data and. Physical laws.
Applying AI to complex scientific problems is gaining traction. In 2020, Google Deepmind’s Alphafold model famously cracked a puzzle in protein biology that had confounded scientists for centuries. The discovery has accelerated research in drug discovery, molecular biology, and bioengineering.
Other companies, like Dutch scaleup VSParticle, are using algorithms to fastrack the discovery and synthesis of potentially game-changing materials.
While the applications of AI in science may differ from discipline to discipline, the benefits are shared: artificial intelligence can supercharge scientific discovery by analysing data, simulating complex systems. And uncovering insights faster than humans ever could.
So AI isn’t all about asking ChatGPT what to eat for dinner? No, dear reader, it’s a actually pretty big deal.
Amsterdam-headquartered Nebius, which builds full-stack AI infrastructure for tech firms, has secured $700mn in a private equity deal led by Nvidia, A...
Time-series forecasting is ubiquitous in various domains, such as retail. Finance, manufacturing, healthcare and natural sciences. In retail use cases...
Akool, a startup doing AI-driven avatar content creation, revealed enhancements to Akool Streaming Avatars that connect avatars with AI models.
Nanoprinter turns Meta’s AI predictions into potentially game-changing materials
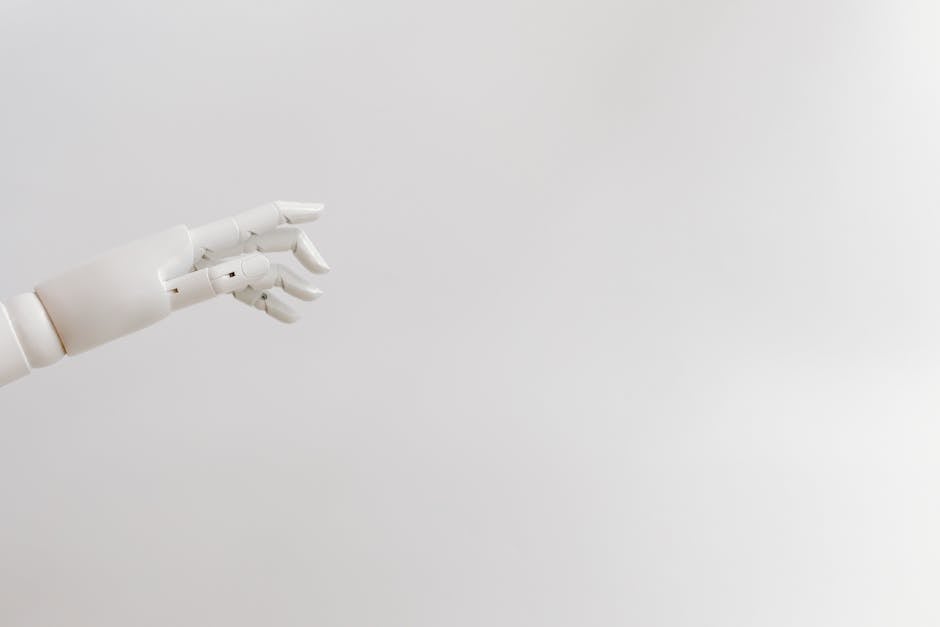
For the past few months, Meta has been sending recipes to a Dutch scaleup called VSParticle (VSP). These are not food recipes — they’re AI-generated instructions for how to make new nanoporous materials that could potentially supercharge the green transition.
VSP has so far taken 525 of these recipes and. Synthesised them into nanomaterials called electrocatalysts. Meta’s algorithms predicted these electrocatalysts would be ideal for breaking down CO2 into useful products like methane or ethanol. VSP brought the AI predictions to life using a nanoprinter, a machine which vaporises materials and then deposits them as thin nanoporous films.
Electrocatalysts speed up chemical reactions that involve electricity, such as splitting water into hydrogen and oxygen. Converting CO2 into fuels, or generating power in fuel cells. They make these processes more efficient, reducing the energy required and enabling clean energy technologies like hydrogen production and advanced batteries.
The problem is that it typically takes scientists up to 15 years just to create one new nanomaterial — until now.
“We’ve synthesised, tested, and validated hundreds of nanomaterials at a scale and. Speed never seen before,” Aaike van Vugt, co-founder and CEO of VSP, told TNW. “This rapid prototyping gives researchers a quick way to validate AI predictions and discover low-cost electrocatalysts that might have taken years or even decades to find using traditional methods.”.
VSP put each batch of the new materials in an envelope and. Shipped it to a lab at the University of Toronto for testing. The findings were then integrated into an open-source experimental database, which can now be used to train AI models to become more more effective at predicting new material combinations.
Larry Zitnick, Research Director at Meta AI. expressed the research is “breaking new ground” in material discovery. “It marks a significant leap in our ability to predict and validate materials that are critical for clean energy solutions,” he expressed.
The Alphafold of nanomaterial discovery?
But to really crack the code for material discovery. AI models need to be trained on much larger datasets. Not hundreds but tens or even hundreds of thousands of tested materials.
Van Vugt expressed that VSP’s machine is the only technology available today that could synthesize such a large number of thin-film nanoporous materials in a reasonable time frame — about two to three years, expressed the founder.
“This could create an AI that is the equivalent of Google Deepmind’s Alphafold. But for nanoporous materials,” presented Van Vugt. He’s referring, of course, to the breakthrough algorithm that cracked a puzzle in protein biology that had confounded scientists for centuries.
If that’s true. Then it puts the enterprise in a pretty sweet position. The world’s tech giants — think Google, Microsoft, Meta — are all racing to build bigger, superior forms of artificial intelligence in a bid to find solutions to some of the world’s greatest challenges. Including climate change. Ironically, these models could also think up solutions for their endless appetite for energy. For companies like Meta, investing in material discovery using AI is a win-win.
VSP is working with many other organisations to build out its dataset and. Mature its technology. These include the Sorbonne University Abu Dhabi, the San Francisco-based Lawrence Livermore National Laboratory, the Materials Discovery Research Institute (MDRI) in the Chicago area, and. The Dutch Institute for Fundamental Energy Research (DIFFER).
The Dutch firm is also fine-tuning its nanoprinters to be faster and more efficient. The current machines are powered by 300 sparks per second, but the team is working on a new printer that would increase this output time to 20,000 sparks per second. This could supercharge material discovery even further.
UK startup Surf Security has launched a beta version of what it asserts is the world’s first browser with a built-in feature designed to spot AI-genera...
As an illustrative case study, we applied the framework to a dermatology model. Which utilizes a convolutional neural network similar to that describe...
UK startup PhysicsX, founded by former Formula 1 engineering whizz Robin “Dr. Rob” Tuluie, has unveiled an AI tool that could fast-track the time it t...
Market Impact Analysis
Market Growth Trend
2018 | 2019 | 2020 | 2021 | 2022 | 2023 | 2024 |
---|---|---|---|---|---|---|
23.1% | 27.8% | 29.2% | 32.4% | 34.2% | 35.2% | 35.6% |
Quarterly Growth Rate
Q1 2024 | Q2 2024 | Q3 2024 | Q4 2024 |
---|---|---|---|
32.5% | 34.8% | 36.2% | 35.6% |
Market Segments and Growth Drivers
Segment | Market Share | Growth Rate |
---|---|---|
Machine Learning | 29% | 38.4% |
Computer Vision | 18% | 35.7% |
Natural Language Processing | 24% | 41.5% |
Robotics | 15% | 22.3% |
Other AI Technologies | 14% | 31.8% |
Technology Maturity Curve
Different technologies within the ecosystem are at varying stages of maturity:
Competitive Landscape Analysis
Company | Market Share |
---|---|
Google AI | 18.3% |
Microsoft AI | 15.7% |
IBM Watson | 11.2% |
Amazon AI | 9.8% |
OpenAI | 8.4% |
Future Outlook and Predictions
The Advances Private Training landscape is evolving rapidly, driven by technological advancements, changing threat vectors, and shifting business requirements. Based on current trends and expert analyses, we can anticipate several significant developments across different time horizons:
Year-by-Year Technology Evolution
Based on current trajectory and expert analyses, we can project the following development timeline:
Technology Maturity Curve
Different technologies within the ecosystem are at varying stages of maturity, influencing adoption timelines and investment priorities:
Innovation Trigger
- Generative AI for specialized domains
- Blockchain for supply chain verification
Peak of Inflated Expectations
- Digital twins for business processes
- Quantum-resistant cryptography
Trough of Disillusionment
- Consumer AR/VR applications
- General-purpose blockchain
Slope of Enlightenment
- AI-driven analytics
- Edge computing
Plateau of Productivity
- Cloud infrastructure
- Mobile applications
Technology Evolution Timeline
- Improved generative models
- specialized AI applications
- AI-human collaboration systems
- multimodal AI platforms
- General AI capabilities
- AI-driven scientific breakthroughs
Expert Perspectives
Leading experts in the ai tech sector provide diverse perspectives on how the landscape will evolve over the coming years:
"The next frontier is AI systems that can reason across modalities and domains with minimal human guidance."
— AI Researcher
"Organizations that develop effective AI governance frameworks will gain competitive advantage."
— Industry Analyst
"The AI talent gap remains a critical barrier to implementation for most enterprises."
— Chief AI Officer
Areas of Expert Consensus
- Acceleration of Innovation: The pace of technological evolution will continue to increase
- Practical Integration: Focus will shift from proof-of-concept to operational deployment
- Human-Technology Partnership: Most effective implementations will optimize human-machine collaboration
- Regulatory Influence: Regulatory frameworks will increasingly shape technology development
Short-Term Outlook (1-2 Years)
In the immediate future, organizations will focus on implementing and optimizing currently available technologies to address pressing ai tech challenges:
- Improved generative models
- specialized AI applications
- enhanced AI ethics frameworks
These developments will be characterized by incremental improvements to existing frameworks rather than revolutionary changes, with emphasis on practical deployment and measurable outcomes.
Mid-Term Outlook (3-5 Years)
As technologies mature and organizations adapt, more substantial transformations will emerge in how security is approached and implemented:
- AI-human collaboration systems
- multimodal AI platforms
- democratized AI development
This period will see significant changes in security architecture and operational models, with increasing automation and integration between previously siloed security functions. Organizations will shift from reactive to proactive security postures.
Long-Term Outlook (5+ Years)
Looking further ahead, more fundamental shifts will reshape how cybersecurity is conceptualized and implemented across digital ecosystems:
- General AI capabilities
- AI-driven scientific breakthroughs
- new computing paradigms
These long-term developments will likely require significant technical breakthroughs, new regulatory frameworks, and evolution in how organizations approach security as a fundamental business function rather than a technical discipline.
Key Risk Factors and Uncertainties
Several critical factors could significantly impact the trajectory of ai tech evolution:
Organizations should monitor these factors closely and develop contingency strategies to mitigate potential negative impacts on technology implementation timelines.
Alternative Future Scenarios
The evolution of technology can follow different paths depending on various factors including regulatory developments, investment trends, technological breakthroughs, and market adoption. We analyze three potential scenarios:
Optimistic Scenario
Responsible AI driving innovation while minimizing societal disruption
Key Drivers: Supportive regulatory environment, significant research breakthroughs, strong market incentives, and rapid user adoption.
Probability: 25-30%
Base Case Scenario
Incremental adoption with mixed societal impacts and ongoing ethical challenges
Key Drivers: Balanced regulatory approach, steady technological progress, and selective implementation based on clear ROI.
Probability: 50-60%
Conservative Scenario
Technical and ethical barriers creating significant implementation challenges
Key Drivers: Restrictive regulations, technical limitations, implementation challenges, and risk-averse organizational cultures.
Probability: 15-20%
Scenario Comparison Matrix
Factor | Optimistic | Base Case | Conservative |
---|---|---|---|
Implementation Timeline | Accelerated | Steady | Delayed |
Market Adoption | Widespread | Selective | Limited |
Technology Evolution | Rapid | Progressive | Incremental |
Regulatory Environment | Supportive | Balanced | Restrictive |
Business Impact | Transformative | Significant | Modest |
Transformational Impact
Redefinition of knowledge work, automation of creative processes. This evolution will necessitate significant changes in organizational structures, talent development, and strategic planning processes.
The convergence of multiple technological trends—including artificial intelligence, quantum computing, and ubiquitous connectivity—will create both unprecedented security challenges and innovative defensive capabilities.
Implementation Challenges
Ethical concerns, computing resource limitations, talent shortages. Organizations will need to develop comprehensive change management strategies to successfully navigate these transitions.
Regulatory uncertainty, particularly around emerging technologies like AI in security applications, will require flexible security architectures that can adapt to evolving compliance requirements.
Key Innovations to Watch
Multimodal learning, resource-efficient AI, transparent decision systems. Organizations should monitor these developments closely to maintain competitive advantages and effective security postures.
Strategic investments in research partnerships, technology pilots, and talent development will position forward-thinking organizations to leverage these innovations early in their development cycle.
Technical Glossary
Key technical terms and definitions to help understand the technologies discussed in this article.
Understanding the following technical concepts is essential for grasping the full implications of the security threats and defensive measures discussed in this article. These definitions provide context for both technical and non-technical readers.