AlphaFold unlocks one of the greatest puzzles in biology - Related to parkinson’s, unlocks, biology, tracks, malaria
AlphaFold unlocks one of the greatest puzzles in biology
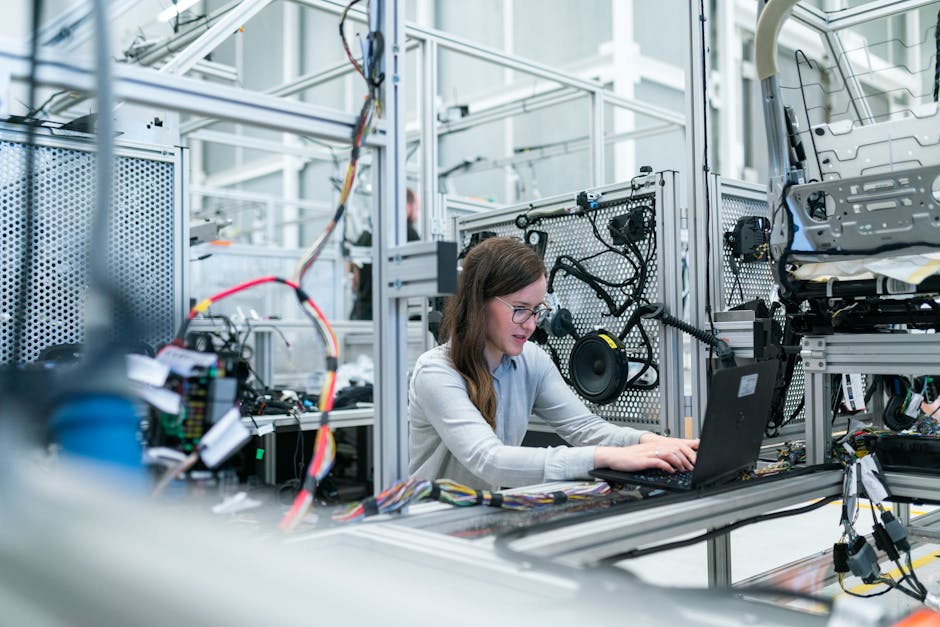
Impact AlphaFold unlocks one of the greatest puzzles in biology Share.
AI system helps researchers piece together one of the largest molecular structures in human cells When Pietro Fontana joined the Wu Lab at Harvard Medical School and Boston Children’s Hospital in May 2019, he had before him what has been called one of the world’s hardest, giant jigsaw puzzles. It was the task of piecing together a model of the nuclear pore complex, one of the largest molecular machines in human cells. “It was very challenging from the start,” he explains. The complex has been called a behemoth for good reason: it is made up of more than 30 different protein subunits, termed nucleoporins, and in total contains more than 1,000 of them, intricately weaved together.
“ I think AlphaFold has completely changed the idea of structural biology Pietro Fontana, Postdoctoral Fellow.
So when he sat down to use AlphaFold in his work for the first time two years later – together with Alexander Tong of University of California, Berkeley, who was more familiar with the AI system – he was uncertain if it would help. But what followed in the summer of 2021 was a somewhat unexpected breakthrough moment. AlphaFold predicted the structures of nucleoporins that had not been previously determined, unveiling more of the nuclear pore complex in the process. Thanks to the AI they could generate a near-complete model of the complex’s cytoplasmic ring.
Model of the nuclear pore complex cytoplasmic ring. Credit: Fontana et al. Science 2022.
“Many components were already well known, but with AlphaFold, we also built the ones that were structurally unknown,” he says. “I started to realize how it’s actually a big and useful tool for us. I think AlphaFold has completely changed the idea of structural biology.” Molecular scientists like Fontana have dedicated themselves to deciphering the nuclear pore complex for decades. It is key because it is a gatekeeper for everything that goes in and out of the nucleus and is thought to hold answers to a growing number of serious human diseases, including amyotrophic lateral sclerosis (ALS) and other neurodegenerative illnesses. Knowing how the complex is assembled could open the door to other groundbreaking, even life-saving, discoveries.
The sheer size complex alone is challenging enough, but its many varied parts represent an added complication. “That’s one major difficulty in achieving a resolution [clear enough] that we can interpret the sequence and structure of the complex,” says Hao Wu, the lab’s principal investigator. Even with a lot of data, the team had previously only managed structural images of medium resolution. Missing pieces of the puzzle also stymied progress. Without the full set, it is hard to tell how the jigsaw fits, says Wu. “To figure out how the different protein subunits come together, you really need to have some assistance on their individual structures,” Wu explains. This is where AlphaFold changed the game for the Wu Lab which also included Ying Dong and Xiong Pi. Running it on proteins found in the eggs of the African clawed frog (Xenopus laevis) – used as a model system – the team, managed to map all the different subunit structures, which were unknown up until then. “When we started trying, we didn’t really know if the predictions would fit the map nicely,” Wu recalls. “But that’s what happened. That was pretty remarkable.” Of course, science is a collaborative effort. When it comes to solving a riddle as intricate as the nuclear pore complex, it’s not just teamwork, but the culmination of the diligence and tenacity of many teams around the world. Across the Atlantic, scientists from Max Planck Institute of Biophysics (MPIBP) and European Molecular Biology Laboratory (EMBL) in Germany have used AlphaFold in combination with cryo-electron tomography to model the human NPC. What they have achieved so far is a new model twice as complete as the old one. Now covering two-thirds of the NPC, a huge part of the puzzle has been solved, and a big step has been taken towards understanding how it controls what goes in and out of the cell nucleus.
The model of the human nuclear pore complex by scientists from MPIBP and EMBL in Germany. Credit: Agnieszka Obarska-Kosinska.
Impact Google Cloud: Driving digital transformation Share.
Google Cloud empowers organizations to digitally transform themselves in...
Some time ago, I mentioned in an article how I believed AI could replace a traditional search engine, and the reasons behind that were focused ...
Note: This blog was first . Following the paper’s publication in Science on 8 Dec 2022, we’ve made minor updates to the text to...
Targeting early-onset Parkinson’s with AI
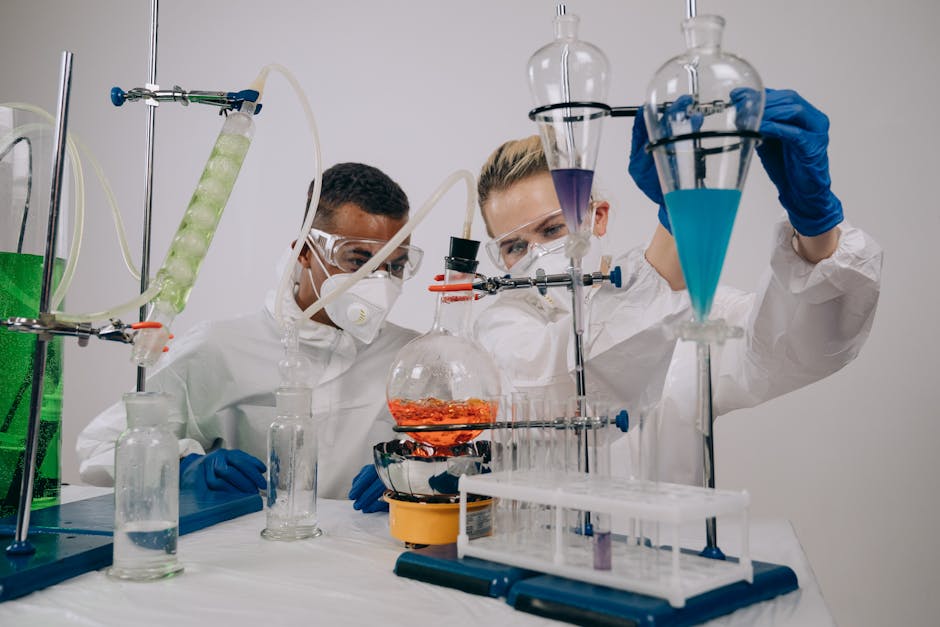
Impact Targeting early-onset Parkinson’s with AI Share.
AlphaFold predictions are paving the way towards new treatments that can impact over 10 million people worldwide It was a source of hard-earned satisfaction after what had often felt like an uphill battle. David Komander and his colleagues had finally -sought structure of PINK1. Mutations in the gene that encodes this protein cause early-onset Parkinson’s, a neurodegenerative disease with a wide range of progressive symptoms – particularly body tremors and difficulty in moving. But when other scientific teams , it became clear that something was amiss. “The other two structures that came out looked very different to the structure that had been done by our group,” says Zhong Yan Gan, a PhD student in Komander’s lab, co-supervised by Associate Professor Grant Dewson, at WEHI (the Walter and Eliza Hall Institute of Medical Research) in Melbourne, Australia. Theirs was the odd one out, with unique capabilities that didn’t appear to exist in the others. The stakes were high: understanding PINK1 could help to unlock new treatments addressing the fundamental cause of Parkinson’s, which affects more than 10 million people worldwide. While Komander’s team had confidence in their own findings, the contrasting results raised some big questions. And in a competitive research field, they knew they wouldn’t be alone in hunting for answers. “Not only were these really difficult nuts to crack, but, once they were cracked, you suddenly open this entire realm of everybody doing very similar things,” says Komander.
The team eventually unraveled the mystery, but it took several more years of research, one chance discovery, and a helping hand from DeepMind’s protein-structure prediction system, AlphaFold. The symptoms of Parkinson’s develop when someone’s brain can no longer make enough of the chemical dopamine. Most people who get Parkinson’s won’t know the specific cause, but around 10% of patients can point to a particular genetic mutation. In these cases, Parkinson’s tends to develop early, affecting people before they reach the age of 50. One of those genetic mutations is in the gene that encodes the PINK1 protein. PINK1 plays a key role in the breakdown and removal of mitochondria, often referred to as the powerhouses inside our cells. “As you age, mitochondria can become old and damaged,” says Gan. “PINK1 is part of the body’s mechanism to recycle old mitochondria to make way for new ones.” When this mechanism falters, the damaged mitochondria build up, leading to the loss of dopamine-producing nerve cells, and eventually to Parkinson’s. So one avenue to finding improved ways to treat the condition is to improved understand PINK1 and its role.
When researchers discovered that PINK1 could cause Parkinson’s disease in 2004, finding its structure became a key goal – but it was not forthcoming, in part because human PINK1 was too unstable to produce in the lab. Pushed to cast their net wider, scientists discovered that insect versions of PINK1 – such as that from human body lice – were stable enough to produce and study in the lab. Which brings us back to our story’s start. Komander’s team . But when other researchers (flour beetles), they knew they only had part of the story. It wasn’t entirely surprising. After all, proteins are dynamic molecules. “They're like machines, and they can take different shapes,” says Gan. What if the – a snapshot of PINK1 during a single stage of a longer process?
“ We had these new structures and, at the time, we were the only people on the planet to know what PINK1 looks like during activation David Komander, biochemist.
Gan took on the ambitious task of figuring out what PINK1 looks like during every step of its activation process as his PhD project. It was during this work that he spotted something odd: a molecule that looked far too big to be his target. “Normally you would disregard it as something that has just clumped together, like a scrambled egg white kind-of-thing,” says Komander. But Gan had a hunch that this clump was worth investigating in greater detail, and decided, with the help of Dr Alisa Glukhova, to probe the molecule at the atomic scale using cryo-electron microscopy (cryo-EM), whereby a frozen sample is examined using a beam of electrons. “I remember saying to Zhong, ‘Yeah, you can try it, but that's never gonna work’,” Komander admits. Gan’s persistence paid off in spades. What he discovered was the very molecule the researchers were looking for: PINK1. But why so big? It turned out that PINK1 likes business. Instead of a single protein, it was grouped together into pairs of molecules known as dimers, which had arranged themselves into still larger formations. “Six dimers of PINK1 were assembling into large, bagel-shaped structures,” says Gan.
This chance discovery meant he could use cryo-EM, which wouldn’t work for a molecule as small as a single PINK1, to solve the protein’s physical structure. The team had their answer. The previously – they were different forms that the protein takes at various stages of its activation process. But there was a catch. All of this experimental work had been done using PINK1 derived from insects. To understand the implications of their findings for humans with Parkinson’s, they would have to investigate whether their findings extended to the human version of the protein. Komander and his team turned to AlphaFold. “We had these new structures and, at the time, we were the only people on the planet to know what PINK1 looks like during activation,” says Komander. So they used AlphaFold to call up its prediction for the structure of human-sourced PINK1, and moments later there it was on the screen. It was “completely shocking” how accurate the AlphaFold predictions were, he says. Later, when Gan put two protein sequences into AlphaFold to predict the structure of a PINK1 dimer in humans, the result was almost indistinguishable from his experimental work with the insect protein. “That dimer was basically showing exactly how these two proteins interact so that they can act and work together to form some of these complexes that we had seen,” says Komander.
“ We can start to think about, 'What kind of drugs do we have to develop to fix the protein, rather than just deal with the fact that it's broken' David Komander.
This close alignment between several experimental results and AlphaFold’s predicted structures gave the team confidence that the AI system could deliver meaningful knowledge beyond their empirical work. They went on to use AlphaFold to model what effect certain mutations would have on the formation of the dimer – to explore how those mutations might lead to Parkinson’s, and their suspicions were confirmed. “We were able to immediately generate some real insights for people who have these particular mutations,” says Komander. These insights could ultimately lead to new treatments. "We can start to think about, 'What kind of drugs do we have to develop to fix the protein, rather than just deal with the fact that it's broken,'" says Komander. They submitted their findings on the activation mechanism of PINK1 to the journal Nature in August 2021 and the paper was accepted in early December 2021. It turned out that researchers at the Trempe Lab in Montreal, Canada, had arrived at similar conclusions, and when that team's paper was , the WEHI authors had to fast-track final revisions. “We were told to finish the paper three days before Christmas, so that it could be ,” says Komander. “It was a brutal timeline.”.
Looking hundreds of millions of years into a protein’s past with AlphaFold to learn about the beginnings of life itself.
New research drawing upon pragmatics and philosophy proposes ways to align conversational agents with human values.
Responsibility & Safety Updating the Frontier Safety Framework Share.
Our next iteration of the FSF sets out stronger security prot...
Stopping malaria in its tracks
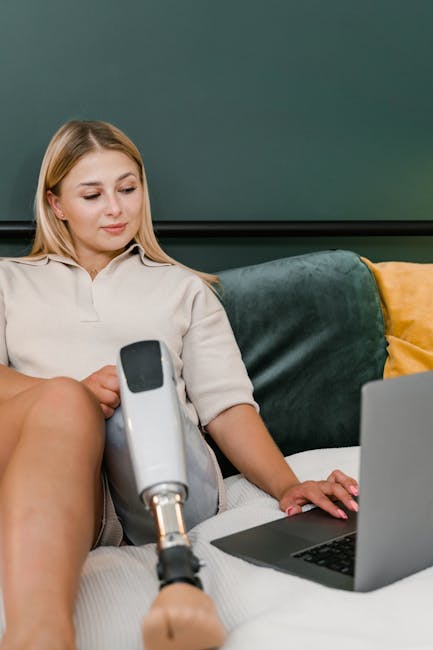
Impact Stopping malaria in its tracks Share.
Developing a advanced malaria vaccine with the help of AI that could save hundreds of thousands of lives every year When biochemist Matthew Higgins established his research group in 2006, he had malaria firmly in his sights. The mosquito-borne disease is second only to tuberculosis in terms of its devastating global impact. Malaria killed an estimated 627,000 people in 2020, mostly children under five, and almost half of the world’s population is within its reach, though Africa is by far the hardest hit. Symptoms of infection can begin with just a fever and a headache, making it easily missed or misdiagnosed – and therefore left untreated. Preventing malaria is therefore the priority, which is why Higgins, a professor of molecular parasitology at the University of Oxford, has been working tirelessly with his team to understand how the malaria parasite interacts with human-host proteins. Their aim is to use these insights to design improved therapies, including a vaccine that will be much more effective than what is currently available.
When a human is bitten by an infected female mosquito, one of five types of malaria parasite may enter the bloodstream. These single-celled parasites are typically carried to the liver, where they mature and multiply, releasing more into the bloodstream. Symptoms such as fever, chills, fatigue, and sickness might not appear until 10 days to four weeks after infection occurs, yet the speed of diagnosis is critical. Of the five parasite species that cause malaria in humans, two are particularly dangerous. For example, an infection by Plasmodium falciparum can, if untreated, suddenly escalate to severe illness and death inside a day. The key challenge for Higgins is the shapeshifting nature of malaria parasites. Their ability to constantly alter their appearance as well as that of their host (red blood) cells allows them to evade the human immune system. “In terms of drug, or vaccine, discovery, that makes it hard to pin it down and decide what to target,” he says. The possibility of a fully effective vaccine – the only way to stop malaria in its tracks – seemed remote.
The urgency of the race to develop an effective vaccine is underlined by the number of teams working towards that goal. Currently, RTS,S, widely known by its brand name Mosquirix, is the only approved inoculation. It was designed for children and in October 2021. Its arrival was a “huge advancement” and “very good news”, says Higgins. Because RTS,S targets only the first step of an infection, in which the malaria parasite is carried to the liver, it only has about a 30% efficacy rate. “30% is a big deal. It means a lot of lives saved,” he says. “But it’s a long way short of the 100% we want.”.
“ When we combined our model with Alphafold’s predicted structure, we could suddenly see how the whole system worked. Matthew Higgins, biochemist.
More in the recent past, another team at the University of Oxford – the Jenner Institute – reported promising results of another similar vaccine. Its approach, which consists of three doses followed by a booster one year later, has an efficacy rate of 77%. However, like Mosquirix, this vaccine intercepts at the first, pre-liver stage of the malaria parasite’s life cycle. In contrast, Higgins - along with his Oxford-based collaborators Simon Draper and Sumi Biswas - is developing vaccine immunogens for a multi-stage vaccine that can simultaneously work at every phase of the infection cycle. Beyond the parasite’s initial entry into human liver cells, the lab’s ultimate goal is a vaccine that can not only target the blood-cell invasion that follows infection, but also the final reproductive stage of the parasite’s life cycle, which involves the fusion of its male and female gametes. It’s critical to tackle this stage, because infected humans can otherwise transmit the parasite to previously uninfected mosquitoes if bitten again, continuing the cycle.
Progress has been hard-fought and slow. To illustrate why, consider the COVID-19 virus. This type of coronavirus has just one spike protein on its surface that a vaccine needs to target. The malaria parasites, on the other hand, have hundreds or even thousands of surface proteins, . And it’s a slippery shapeshifter. Crucially, developing a vaccine that contains a critical infection-disrupting component requires knowing the molecular structure of one gamete surface protein – Pfs48/45 – essential to the development of the parasite in the mosquito midgut. This is where Higgins and his team got derailed. For years they tried to decipher the protein’s shape, with limited success. Even using two of the best experimental techniques available to discern a protein’s structure – X-ray crystallography and cryo-electron microscopy – the researchers could obtain only fuzzy, low-resolution images. As a result, their structural models of Pfs48/45 were necessarily imperfect and incomplete. That was, until AlphaFold arrived. “We’d been battling with this problem for years, trying to get the details we needed,” says Higgins. “Then we added AlphaFold into the mix. And when we combined our model with Alphafold’s predicted structure, we could suddenly see how the whole system worked.” Higgins recalls the exciting moment that his PhD student Kuang-Ting Ko – “who had been trying all sorts of different things to improve the experimental images” – burst into the office with the news.
“ AlphaFold has allowed us to take our project to the next level, from a fundamental science stage to the preclinical and clinical development stage. - Matthew Higgins.
“It was a great relief,” says Higgins, and a turning point for the project. The combination of laborious experimental work and AI prediction quickly resulted in a sharp view of Pfs48/45. “The crucial AlphaFold information enabled us to decide which bits of the protein we want to put in a vaccine and how we want to organize those proteins,” says Higgins. “AlphaFold has allowed us to take our project to the next level, from a fundamental science stage to the preclinical and clinical development stage.” AlphaFold is not without its flaws, of course. Higgins noted that while the AI system worked well in predicting how each module within a protein adopts its structure, there were instances when its 3D visualizations were a little off. To get the most accurate and confident results, AlphaFold is best used alongside more traditional tools such as cryo-electron microscopy, he says. “I’m sure AlphaFold’s predictions will get enhanced and enhanced. But for now, combining experimental knowledge with AlphaFold models is the optimal approach, because it allows us to piece everything together. This is the approach which we are taking for many of our projects.”.
In my professional life as a data scientist, I have encountered time series multiple times. Most of my knowledge comes from my academic experience, sp...
Exploring AGI, the challenges of scaling and the future of multimodal generative AI.
Next week the artificial intelligence (AI) community will come to...
Research Building interactive agents in video game worlds Share.
Introducing a framework to create AI agents that can understand hu...
Market Impact Analysis
Market Growth Trend
2018 | 2019 | 2020 | 2021 | 2022 | 2023 | 2024 |
---|---|---|---|---|---|---|
23.1% | 27.8% | 29.2% | 32.4% | 34.2% | 35.2% | 35.6% |
Quarterly Growth Rate
Q1 2024 | Q2 2024 | Q3 2024 | Q4 2024 |
---|---|---|---|
32.5% | 34.8% | 36.2% | 35.6% |
Market Segments and Growth Drivers
Segment | Market Share | Growth Rate |
---|---|---|
Machine Learning | 29% | 38.4% |
Computer Vision | 18% | 35.7% |
Natural Language Processing | 24% | 41.5% |
Robotics | 15% | 22.3% |
Other AI Technologies | 14% | 31.8% |
Technology Maturity Curve
Different technologies within the ecosystem are at varying stages of maturity:
Competitive Landscape Analysis
Company | Market Share |
---|---|
Google AI | 18.3% |
Microsoft AI | 15.7% |
IBM Watson | 11.2% |
Amazon AI | 9.8% |
OpenAI | 8.4% |
Future Outlook and Predictions
The Alphafold Unlocks Greatest landscape is evolving rapidly, driven by technological advancements, changing threat vectors, and shifting business requirements. Based on current trends and expert analyses, we can anticipate several significant developments across different time horizons:
Year-by-Year Technology Evolution
Based on current trajectory and expert analyses, we can project the following development timeline:
Technology Maturity Curve
Different technologies within the ecosystem are at varying stages of maturity, influencing adoption timelines and investment priorities:
Innovation Trigger
- Generative AI for specialized domains
- Blockchain for supply chain verification
Peak of Inflated Expectations
- Digital twins for business processes
- Quantum-resistant cryptography
Trough of Disillusionment
- Consumer AR/VR applications
- General-purpose blockchain
Slope of Enlightenment
- AI-driven analytics
- Edge computing
Plateau of Productivity
- Cloud infrastructure
- Mobile applications
Technology Evolution Timeline
- Improved generative models
- specialized AI applications
- AI-human collaboration systems
- multimodal AI platforms
- General AI capabilities
- AI-driven scientific breakthroughs
Expert Perspectives
Leading experts in the ai tech sector provide diverse perspectives on how the landscape will evolve over the coming years:
"The next frontier is AI systems that can reason across modalities and domains with minimal human guidance."
— AI Researcher
"Organizations that develop effective AI governance frameworks will gain competitive advantage."
— Industry Analyst
"The AI talent gap remains a critical barrier to implementation for most enterprises."
— Chief AI Officer
Areas of Expert Consensus
- Acceleration of Innovation: The pace of technological evolution will continue to increase
- Practical Integration: Focus will shift from proof-of-concept to operational deployment
- Human-Technology Partnership: Most effective implementations will optimize human-machine collaboration
- Regulatory Influence: Regulatory frameworks will increasingly shape technology development
Short-Term Outlook (1-2 Years)
In the immediate future, organizations will focus on implementing and optimizing currently available technologies to address pressing ai tech challenges:
- Improved generative models
- specialized AI applications
- enhanced AI ethics frameworks
These developments will be characterized by incremental improvements to existing frameworks rather than revolutionary changes, with emphasis on practical deployment and measurable outcomes.
Mid-Term Outlook (3-5 Years)
As technologies mature and organizations adapt, more substantial transformations will emerge in how security is approached and implemented:
- AI-human collaboration systems
- multimodal AI platforms
- democratized AI development
This period will see significant changes in security architecture and operational models, with increasing automation and integration between previously siloed security functions. Organizations will shift from reactive to proactive security postures.
Long-Term Outlook (5+ Years)
Looking further ahead, more fundamental shifts will reshape how cybersecurity is conceptualized and implemented across digital ecosystems:
- General AI capabilities
- AI-driven scientific breakthroughs
- new computing paradigms
These long-term developments will likely require significant technical breakthroughs, new regulatory frameworks, and evolution in how organizations approach security as a fundamental business function rather than a technical discipline.
Key Risk Factors and Uncertainties
Several critical factors could significantly impact the trajectory of ai tech evolution:
Organizations should monitor these factors closely and develop contingency strategies to mitigate potential negative impacts on technology implementation timelines.
Alternative Future Scenarios
The evolution of technology can follow different paths depending on various factors including regulatory developments, investment trends, technological breakthroughs, and market adoption. We analyze three potential scenarios:
Optimistic Scenario
Responsible AI driving innovation while minimizing societal disruption
Key Drivers: Supportive regulatory environment, significant research breakthroughs, strong market incentives, and rapid user adoption.
Probability: 25-30%
Base Case Scenario
Incremental adoption with mixed societal impacts and ongoing ethical challenges
Key Drivers: Balanced regulatory approach, steady technological progress, and selective implementation based on clear ROI.
Probability: 50-60%
Conservative Scenario
Technical and ethical barriers creating significant implementation challenges
Key Drivers: Restrictive regulations, technical limitations, implementation challenges, and risk-averse organizational cultures.
Probability: 15-20%
Scenario Comparison Matrix
Factor | Optimistic | Base Case | Conservative |
---|---|---|---|
Implementation Timeline | Accelerated | Steady | Delayed |
Market Adoption | Widespread | Selective | Limited |
Technology Evolution | Rapid | Progressive | Incremental |
Regulatory Environment | Supportive | Balanced | Restrictive |
Business Impact | Transformative | Significant | Modest |
Transformational Impact
Redefinition of knowledge work, automation of creative processes. This evolution will necessitate significant changes in organizational structures, talent development, and strategic planning processes.
The convergence of multiple technological trends—including artificial intelligence, quantum computing, and ubiquitous connectivity—will create both unprecedented security challenges and innovative defensive capabilities.
Implementation Challenges
Ethical concerns, computing resource limitations, talent shortages. Organizations will need to develop comprehensive change management strategies to successfully navigate these transitions.
Regulatory uncertainty, particularly around emerging technologies like AI in security applications, will require flexible security architectures that can adapt to evolving compliance requirements.
Key Innovations to Watch
Multimodal learning, resource-efficient AI, transparent decision systems. Organizations should monitor these developments closely to maintain competitive advantages and effective security postures.
Strategic investments in research partnerships, technology pilots, and talent development will position forward-thinking organizations to leverage these innovations early in their development cycle.
Technical Glossary
Key technical terms and definitions to help understand the technologies discussed in this article.
Understanding the following technical concepts is essential for grasping the full implications of the security threats and defensive measures discussed in this article. These definitions provide context for both technical and non-technical readers.