Benchmarking the next generation of never-ending learners - Related to 2022, benchmarking, latest, perception, learners
Benchmarking the next generation of never-ending learners
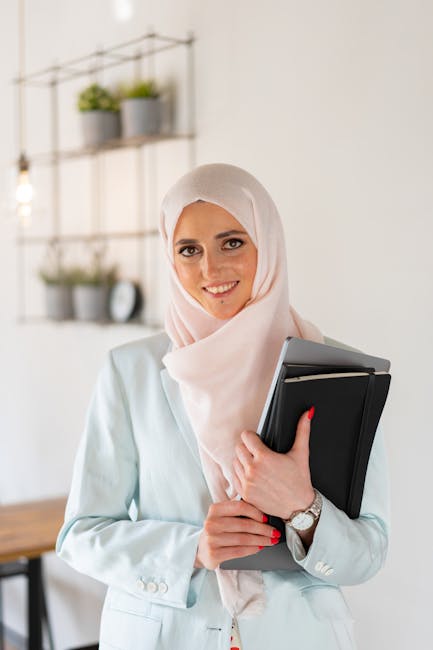
NEVIS’22 is actually composed of 106 tasks extracted from publications randomly sampled from the online proceedings of major computer vision conferences over the past three decades. Each task is a supervised classification task, the best understood approach in machine learning. And crucially, the tasks are arranged chronologically, and so, become more challenging and expansive, providing increasing opportunities to transfer knowledge from a growing set of related tasks. The challenge is how to automatically transfer useful knowledge from one task to the next to achieve a more effective or more efficient performance.
Our new paper, NEVIS’22: A Stream of 100 Tasks Sampled From 30 Years of Computer Vision Research , proposes a playground to study the question of efficient knowledge transfer in a controlled and reproducible setting. The Never-Ending Visual classification Stream (NEVIS’22) is a benchmark stream in addition to an evaluation protocol, a set of initial baselines, and an open-source codebase. This package provides an opportunity for researchers to explore how models can continually build on their knowledge to learn future tasks more efficiently.
This raises the question of whether we could improve the trade-off between the efficiency and performance of these large models, making them faster and more sustainable while also preserving their outstanding capabilities. One answer to this is to encourage the development of models that accrue knowledge over time, and that can therefore enhanced adapt more efficiently to new situations and novel tasks.
Scaling up has resulted in fantastic capabilities, but also means that DL models can be resource intensive. For example, when large models are deployed, whatever they have learned on one task is seldom harnessed to facilitate their learning of the next task. What’s more, once new data or more compute become available, large models are typically retrained from scratch – a costly, time-consuming process.
In just a few years, large-scale deep learning (DL) models have achieved unprecedented success in a variety of domains, from predicting protein structures to natural language processing and vision[1, 2, 3]. Machine learning engineers and researchers have delivered these successes for the most part thanks to powerful new hardware that has enabled their models to scale up and be trained with more data.
NEVIS’22 is reproducible and sufficiently scaled to test state-of-the-art learning algorithms. The stream includes a rich diversity of tasks, from optical character recognition and texture analysis to crowd counting and scene recognition. The task-selection process, being randomly sampled, did not favour any particular approach, but merely reflects what the computer vision community has deemed interesting over time.
NEVIS’22 is not only about data, but also about the methodology used to train and evaluate learning models. We evaluate learners , as measured by their trade-off between error rate and compute (the latter measured by the number of floating-point operations). So, for example, achieving a lower error rate in NEVIS’22 is not sufficient if this comes at an unreasonable computational cost. Instead, we incentivise models to be both accurate and efficient.
Our initial experiments show that the models that achieve a enhanced trade-off are those that leverage the structure shared across tasks and employ some form of transfer learning. In particular, clever fine-tuning approaches can be rather competitive, even when combined with large pre-trained models. This latter finding highlights the possibility to further improve upon the general representations of large-scale models, opening up an entirely new avenue of research. We believe that NEVIS’22 presents an exciting new challenge for our community as we strive to develop more efficient and effective never-ending learning models.
Discover more about NEVIS’22 by reading our paper and downloading our code.
Today, we’re releasing two updated production-ready Gemini models: [website] and [website] along with: >50% reduced price on [website] P...
Note: This blog was first . Following the paper’s publication in Science on 8 Dec 2022, we’ve made minor updates to the text to...
New research drawing upon pragmatics and philosophy proposes ways to align conversational agents with human values.
DeepMind’s latest research at NeurIPS 2022
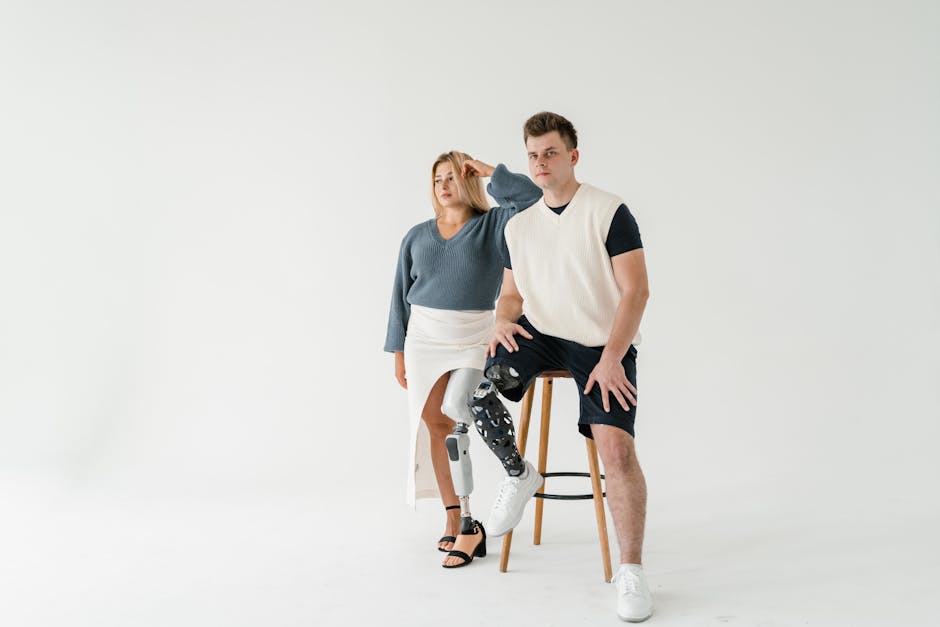
Advancing best-in-class large models, compute-optimal RL agents, and more transparent, ethical, and fair AI systems.
The thirty-sixth International Conference on Neural Information Processing Systems (NeurIPS 2022) is taking place from 28 November - 9 December 2022, as a hybrid event, based in New Orleans, USA.
NeurIPS is the world’s largest conference in artificial intelligence (AI) and machine learning (ML), and we’re proud to support the event as Diamond sponsors, helping foster the exchange of research advances in the AI and ML community.
Teams from across DeepMind are presenting 47 papers, including 35 external collaborations in virtual panels and poster sessions. Here’s a brief introduction to some of the research we’re presenting:
Large models (LMs) – generative AI systems trained on huge amounts of data – have resulted in incredible performances in areas including language, text, audio, and image generation. Part of their success is down to their sheer scale.
However, in Chinchilla, we have created a 70 billion parameter language model that outperforms many larger models, including Gopher. We updated the scaling laws of large models, showing how previously trained models were too large for the amount of training performed. This work already shaped other models that follow these updated rules, creating leaner, superior models, and has won an Outstanding Main Track Paper award at the conference.
Building upon Chinchilla and our multimodal models NFNets and Perceiver, we also present Flamingo, a family of few-shot learning visual language models. Handling images, videos and textual data, Flamingo represents a bridge between vision-only and language-only models. A single Flamingo model sets a new state of the art in few-shot learning on a wide range of open-ended multimodal tasks.
And yet, scale and architecture aren’t the only factors that are crucial for the power of transformer-based models. Data properties also play a significant role, which we discuss in a presentation on data properties that promote in-context learning in transformer models.
Reinforcement learning (RL) has shown great promise as an approach to creating generalised AI systems that can address a wide range of complex tasks. It has led to breakthroughs in many domains from Go to mathematics, and we’re always looking for ways to make RL agents smarter and leaner.
We introduce a new approach that boosts the decision-making abilities of RL agents in a compute-efficient way by drastically expanding the scale of information available for their retrieval.
We’ll also showcase a conceptually simple yet general approach for curiosity-driven exploration in visually complex environments – an RL agent called BYOL-Explore. It achieves superhuman performance while being robust to noise and being much simpler than prior work.
From compressing data to running simulations for predicting the weather, algorithms are a fundamental part of modern computing. And so, incremental improvements can have an enormous impact when working at scale, helping save energy, time, and money.
We share a radically new and highly scalable method for the automatic configuration of computer networks, based on neural algorithmic reasoning, showing that our highly flexible approach is up to 490 times faster than the current state of the art, while satisfying the majority of the input constraints.
During the same session, we also present a rigorous exploration of the previously theoretical notion of “algorithmic alignment”, highlighting the nuanced relationship between graph neural networks and dynamic programming, and how best to combine them for optimising out-of-distribution performance.
At the heart of DeepMind’s mission is our commitment to act as responsible pioneers in the field of AI. We’re committed to developing AI systems that are transparent, ethical, and fair.
Explaining and understanding the behaviour of complex AI systems is an essential part of creating fair, transparent, and accurate systems. We offer a set of desiderata that capture those ambitions, and describe a practical way to meet them, which involves training an AI system to build a causal model of itself, enabling it to explain its own behaviour in a meaningful way.
To act safely and ethically in the world, AI agents must be able to reason about harm and avoid harmful actions. We’ll introduce collaborative work on a novel statistical measure called counterfactual harm, and demonstrate how it overcomes problems with standard approaches to avoid pursuing harmful policies.
Finally, we're presenting our new paper which proposes ways to diagnose and mitigate failures in model fairness caused by distribution shifts, showing how key these issues are for the deployment of safe ML technologies in healthcare settings.
See the full range of our work at NeurIPS 2022 here.
Building a responsible approach to data collection with the Partnership on AI.
At DeepMind, our goal is to make sure everything we do meets the highes...
Experience AI's course and resources are expanding on a global scale.
AI has the potential to drive one of the greatest social, economic and scientifi...
Technologies Gemma Scope: helping the safety community shed light on the inner workings of language models Share.
Measuring perception in AI models
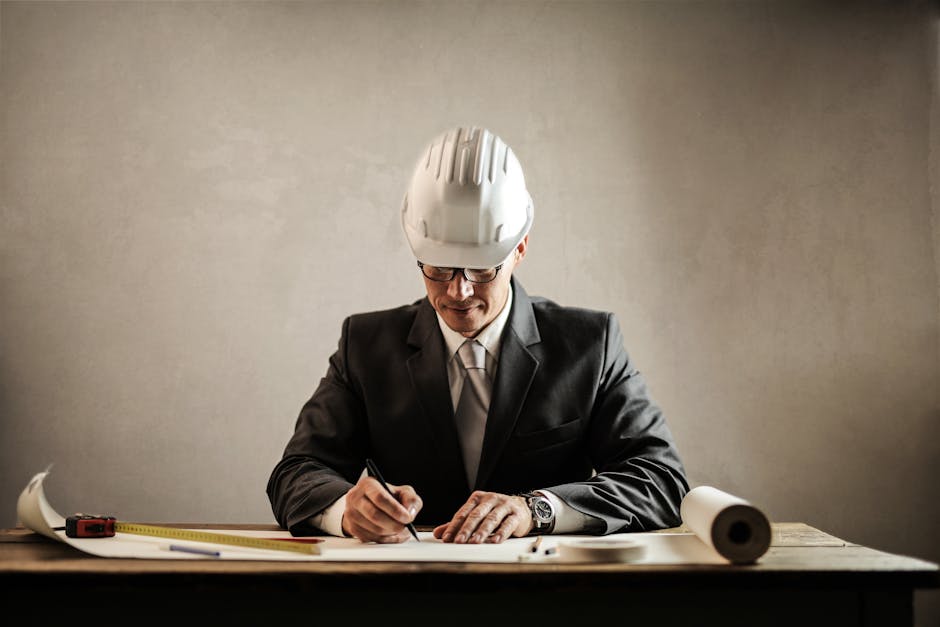
New benchmark for evaluating multimodal systems based on real-world video, audio, and text data.
From the Turing test to ImageNet, benchmarks have played an instrumental role in shaping artificial intelligence (AI) by helping define research goals and allowing researchers to measure progress towards those goals. Incredible breakthroughs in the past 10 years, such as AlexNet in computer vision and AlphaFold in protein folding, have been closely linked to using benchmark datasets, allowing researchers to rank model design and training choices, and iterate to improve their models. As we work towards the goal of building artificial general intelligence (AGI), developing robust and effective benchmarks that expand AI models’ capabilities is as significant as developing the models themselves.
Perception – the process of experiencing the world through senses – is a significant part of intelligence. And building agents with human-level perceptual understanding of the world is a central but challenging task, which is becoming increasingly key in robotics, self-driving cars, personal assistants, medical imaging, and more. So today, we’re introducing the Perception Test, a multimodal benchmark using real-world videos to help evaluate the perception capabilities of a model.
Many perception-related benchmarks are currently being used across AI research, like Kinetics for video action recognition, Audioset for audio event classification, MOT for object tracking, or VQA for image question-answering. These benchmarks have led to amazing progress in how AI model architectures and training methods are built and developed, but each one only targets restricted aspects of perception: image benchmarks exclude temporal aspects; visual question-answering tends to focus on high-level semantic scene understanding; object tracking tasks generally capture lower-level appearance of individual objects, like colour or texture. And very few benchmarks define tasks over both audio and visual modalities.
Multimodal models, such as Perceiver, Flamingo, or BEiT-3, aim to be more general models of perception. But their evaluations were based on multiple specialised datasets because no dedicated benchmark was available. This process is slow, expensive, and provides incomplete coverage of general perception abilities like memory, making it difficult for researchers to compare methods.
To address many of these issues, we created a dataset of purposefully designed videos of real-world activities, labelled :
Research A catalogue of genetic mutations to help pinpoint the cause of diseases Share.
New AI tool classifies the effects of 71 mi...
During the telecommunication boom, Claude Shannon, in his seminal 1948 paper¹, posed a question that would revoluti...
Research A glimpse of the next generation of AlphaFold Share.
Progress upgrade: Our latest AlphaFold model exhibits significantly impro...
Market Impact Analysis
Market Growth Trend
2018 | 2019 | 2020 | 2021 | 2022 | 2023 | 2024 |
---|---|---|---|---|---|---|
23.1% | 27.8% | 29.2% | 32.4% | 34.2% | 35.2% | 35.6% |
Quarterly Growth Rate
Q1 2024 | Q2 2024 | Q3 2024 | Q4 2024 |
---|---|---|---|
32.5% | 34.8% | 36.2% | 35.6% |
Market Segments and Growth Drivers
Segment | Market Share | Growth Rate |
---|---|---|
Machine Learning | 29% | 38.4% |
Computer Vision | 18% | 35.7% |
Natural Language Processing | 24% | 41.5% |
Robotics | 15% | 22.3% |
Other AI Technologies | 14% | 31.8% |
Technology Maturity Curve
Different technologies within the ecosystem are at varying stages of maturity:
Competitive Landscape Analysis
Company | Market Share |
---|---|
Google AI | 18.3% |
Microsoft AI | 15.7% |
IBM Watson | 11.2% |
Amazon AI | 9.8% |
OpenAI | 8.4% |
Future Outlook and Predictions
The Benchmarking Next Generation landscape is evolving rapidly, driven by technological advancements, changing threat vectors, and shifting business requirements. Based on current trends and expert analyses, we can anticipate several significant developments across different time horizons:
Year-by-Year Technology Evolution
Based on current trajectory and expert analyses, we can project the following development timeline:
Technology Maturity Curve
Different technologies within the ecosystem are at varying stages of maturity, influencing adoption timelines and investment priorities:
Innovation Trigger
- Generative AI for specialized domains
- Blockchain for supply chain verification
Peak of Inflated Expectations
- Digital twins for business processes
- Quantum-resistant cryptography
Trough of Disillusionment
- Consumer AR/VR applications
- General-purpose blockchain
Slope of Enlightenment
- AI-driven analytics
- Edge computing
Plateau of Productivity
- Cloud infrastructure
- Mobile applications
Technology Evolution Timeline
- Improved generative models
- specialized AI applications
- AI-human collaboration systems
- multimodal AI platforms
- General AI capabilities
- AI-driven scientific breakthroughs
Expert Perspectives
Leading experts in the ai tech sector provide diverse perspectives on how the landscape will evolve over the coming years:
"The next frontier is AI systems that can reason across modalities and domains with minimal human guidance."
— AI Researcher
"Organizations that develop effective AI governance frameworks will gain competitive advantage."
— Industry Analyst
"The AI talent gap remains a critical barrier to implementation for most enterprises."
— Chief AI Officer
Areas of Expert Consensus
- Acceleration of Innovation: The pace of technological evolution will continue to increase
- Practical Integration: Focus will shift from proof-of-concept to operational deployment
- Human-Technology Partnership: Most effective implementations will optimize human-machine collaboration
- Regulatory Influence: Regulatory frameworks will increasingly shape technology development
Short-Term Outlook (1-2 Years)
In the immediate future, organizations will focus on implementing and optimizing currently available technologies to address pressing ai tech challenges:
- Improved generative models
- specialized AI applications
- enhanced AI ethics frameworks
These developments will be characterized by incremental improvements to existing frameworks rather than revolutionary changes, with emphasis on practical deployment and measurable outcomes.
Mid-Term Outlook (3-5 Years)
As technologies mature and organizations adapt, more substantial transformations will emerge in how security is approached and implemented:
- AI-human collaboration systems
- multimodal AI platforms
- democratized AI development
This period will see significant changes in security architecture and operational models, with increasing automation and integration between previously siloed security functions. Organizations will shift from reactive to proactive security postures.
Long-Term Outlook (5+ Years)
Looking further ahead, more fundamental shifts will reshape how cybersecurity is conceptualized and implemented across digital ecosystems:
- General AI capabilities
- AI-driven scientific breakthroughs
- new computing paradigms
These long-term developments will likely require significant technical breakthroughs, new regulatory frameworks, and evolution in how organizations approach security as a fundamental business function rather than a technical discipline.
Key Risk Factors and Uncertainties
Several critical factors could significantly impact the trajectory of ai tech evolution:
Organizations should monitor these factors closely and develop contingency strategies to mitigate potential negative impacts on technology implementation timelines.
Alternative Future Scenarios
The evolution of technology can follow different paths depending on various factors including regulatory developments, investment trends, technological breakthroughs, and market adoption. We analyze three potential scenarios:
Optimistic Scenario
Responsible AI driving innovation while minimizing societal disruption
Key Drivers: Supportive regulatory environment, significant research breakthroughs, strong market incentives, and rapid user adoption.
Probability: 25-30%
Base Case Scenario
Incremental adoption with mixed societal impacts and ongoing ethical challenges
Key Drivers: Balanced regulatory approach, steady technological progress, and selective implementation based on clear ROI.
Probability: 50-60%
Conservative Scenario
Technical and ethical barriers creating significant implementation challenges
Key Drivers: Restrictive regulations, technical limitations, implementation challenges, and risk-averse organizational cultures.
Probability: 15-20%
Scenario Comparison Matrix
Factor | Optimistic | Base Case | Conservative |
---|---|---|---|
Implementation Timeline | Accelerated | Steady | Delayed |
Market Adoption | Widespread | Selective | Limited |
Technology Evolution | Rapid | Progressive | Incremental |
Regulatory Environment | Supportive | Balanced | Restrictive |
Business Impact | Transformative | Significant | Modest |
Transformational Impact
Redefinition of knowledge work, automation of creative processes. This evolution will necessitate significant changes in organizational structures, talent development, and strategic planning processes.
The convergence of multiple technological trends—including artificial intelligence, quantum computing, and ubiquitous connectivity—will create both unprecedented security challenges and innovative defensive capabilities.
Implementation Challenges
Ethical concerns, computing resource limitations, talent shortages. Organizations will need to develop comprehensive change management strategies to successfully navigate these transitions.
Regulatory uncertainty, particularly around emerging technologies like AI in security applications, will require flexible security architectures that can adapt to evolving compliance requirements.
Key Innovations to Watch
Multimodal learning, resource-efficient AI, transparent decision systems. Organizations should monitor these developments closely to maintain competitive advantages and effective security postures.
Strategic investments in research partnerships, technology pilots, and talent development will position forward-thinking organizations to leverage these innovations early in their development cycle.
Technical Glossary
Key technical terms and definitions to help understand the technologies discussed in this article.
Understanding the following technical concepts is essential for grasping the full implications of the security threats and defensive measures discussed in this article. These definitions provide context for both technical and non-technical readers.