Adobe Acrobat's AI Assistant can now decipher complex contracts for you - Related to icml, complex, towards, now, 2023
Adobe Acrobat's AI Assistant can now decipher complex contracts for you
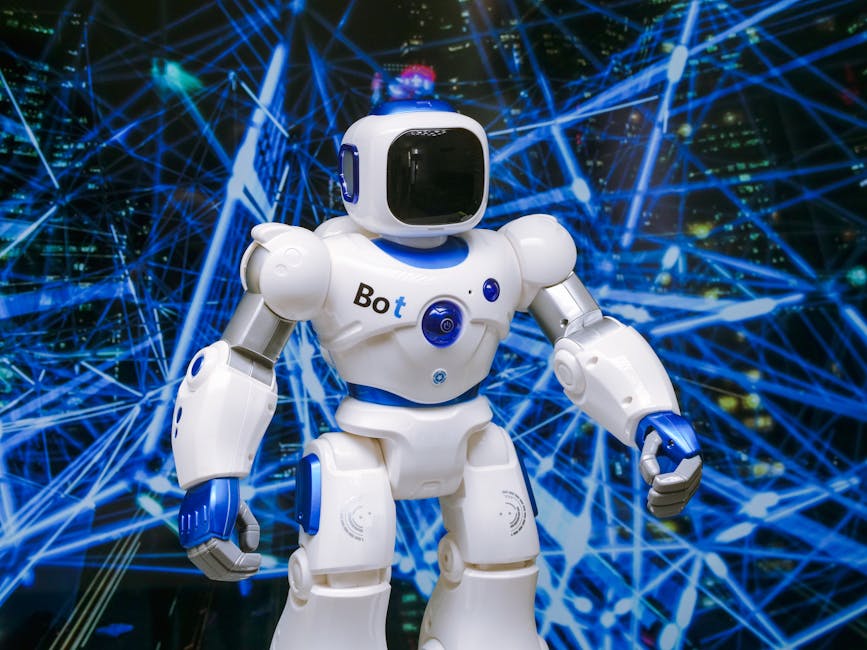
We all have to deal with contracts and agreements as consumers or business professionals. However, as most contracts are written in legalese, understanding them can be challenging. To ease the pain, Adobe has added a new skill to its Acrobat AI that tries to interpret complex contracts for the average person.
Automatically detecting when a PDF is a contract, the AI can summarize the information, suggest questions for you to ask, and even find differences between multiple versions of a contract. To access the new contract-reading superpower, you'll need to purchase Adobe's AI Assistant for Acrobat, a $5 per month add-on for the paid Adobe Acrobat and the free Acrobat Reader.
Also: Business leaders are embracing AI, but their employees are not so sure.
Recognizing regular PDFs and scanned documents set up as contracts, the AI will generate an overview and highlight keywords and terms for you. After analyzing the document, the AI provides a brief summary of the details with cleaner, clearer language and citations. Selecting a specific citation takes you to the source document for review.
The AI will also help if you have to deal with multiple versions of the same contract. Here, it can scan for differences among them and find inconsistencies and discrepancies across as many as 10 versions. When you're done, you can review the contract with other people and parties involved and request e-signatures directly from Adobe Acrobat or Reader.
You can use the new skill for a variety of contract types, including credit card and vendor agreements, loyalty programs, purchase orders, and business agreements. For example, a business owner could use the AI to review a partnership agreement with their attorney. A financial analyst can review sales contracts. Consumers could use it to analyze changes in everything from apartment leases to mobile phone plans.
Sounds helpful. But what are the potential pitfalls? Well, AI is far from perfect. How do you know that Adobe's AI is analyzing and interpreting the contract correctly?
To address that question, Adobe says that its AI assistant combines its large language models (LLMs) with the same artificial intelligence and machine learning models behind its Liquid Mode technology. The goal is to offer a more accurate analysis of the document's structure and content to improve the quality and reliability of the output.
Also: Perplexity lets you try DeepSeek R1 - without the security risk.
AI is also infamous for training itself on user data. Will your contracts be on the training list? To address this concern, Adobe says that the AI is governed by data security protocols and developed in line with its AI ethics processes. The enterprise promises that it will never train its generative AI models on customer data and restricts third-party LLMs from similar training.
"clients open billions of contracts in Adobe Acrobat each month and AI can be a game changer in helping simplify their experience," Abhigyan Modi, senior vice president of Adobe Document Cloud, mentioned in a news release. "We are introducing new capabilities to deliver contract intelligence in Adobe AI Assistant, making it easier for clients to understand and compare these complex documents and providing citations to help them verify responses, all while keeping their data safe."
In the world of machine learning, we obsess over model architectures, training pipelines, and hyper-...
One of the biggest complaints about ChatGPT is that it provi...
Deepseek has in the recent past made quite a buzz in the AI community, thanks to its impressive performance at...
Towards Data Science is Launching as an Independent Publication
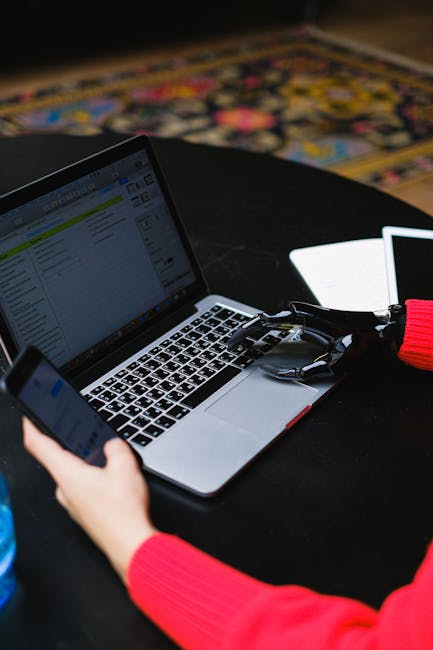
Since founding Towards Data Science in 2016, we’ve built the largest publication on Medium with a dedicated community of readers and contributors focused on data science, machine learning, and AI. Medium built a fantastic platform, and we wouldn’t have been able to reach our audience without its help.
As of Monday, February 3, 2025, Towards Data Science will become an independent publication. While Medium has been instrumental in our growth, our priorities have diverged, and we believe this transition will help us superior serve our contributors and readers.
A recent Medium policy change caused us to accelerate a plan to go independent. By moving off Medium we can maintain complete control over our editorial vision, protect the domain authority we’ve built, and guide our future growth on our own terms.
Today, we’re launching our new WordPress site, though some parts of the migration are still a work in progress. We’re in close communication with our contributors to ensure a smooth transition. Despite the move, our mission remains unchanged: We’ll continue to provide the same in-depth coverage of data science, machine learning, and AI – only now on a platform where we can further support authors by utilizing exciting new promotional tools, advanced analytics, and dynamic community elements that open up fresh opportunities for engagement and growth.
We’ve long recognized that Medium provides valuable hosting, distribution and monetization tools – resources that have helped many writers and publishers (like us!) reach a wide audience. However, as Medium continues to evolve, we believe our long-term goals – as well as those of our readers and contributors – are best supported on an independent platform.
Here’s why we believe an independent platform is the right move:
Improved Accessibility for Readers – We believe in open access to all articles, not in paywalls. Moving to our own platform ensures that all of our content is available without charge to readers.
Ongoing Support for Authors – Our independent platform gives authors greater flexibility to promote their work. This means authors can amplify their voices and engage with new audiences.
Ownership of our Domain Authority – Operating independently allows us to preserve and grow Towards Data Science by not only retaining our search visibility, but also allowing us to experiment with novel content formats and create deeper connections with readers.
For current TDS authors, expect an email soon with details on how to join the new platform.
For new contributors, we’re opening up submissions and would love to feature your work on our new site. More details will follow in the coming days.
in the recent past, Medium started updating its SEO and domain authority structure to consolidate it under the [website] domain. Starting on January 31, 2025, articles that were once on [website] now redirect to Medium URLs.
While this change fits within Medium’s broader strategy, it significantly affects how independent publications like TDS manage brand visibility and long-term search presence. Since our domain structure is key to discoverability, we saw this as the right moment to launch our independent publication.
Medium remains a valuable platform for many writers, but our ambitions extend far outside of its paywall. For us at Towards Data Science, moving to an independent platform isn’t just about preserving editorial control – it’s a strategic leap towards growing the publication we’ve spent the last eight years building.
If you have any questions or concerns about how your content will be handled during this migration, please don’t hesitate to reach out to our editorial team – you can email us here: [email protected]. We’re here to provide any additional clarification you might need and to address any issues that arise.
Join Us for the Next Chapter of Towards Data Science.
To our readers and contributors: thank you for being a part of Towards Data Science. With our new home, we’re set to deliver quality, open-access content on data science, machine learning, and AI and gaining access to a full suite of publishing and marketing tools.
We hope you’ll join us in TDS’s exciting new chapter. Check out the new site, share your feedback, and help us spread the word as we continue to launch the new site. Your support means everything to us, and we’re excited to show you the future of Towards Data Science.
Research AI achieves silver-medal standard solving International Mathematical Olympiad problems Share.
Technologies Transforming the future of music creation Share.
Announcing our most advanced music generation model, and two new AI e...
Responsibility & Safety How can we build human values into AI? Share.
Drawing from philosophy to identify fair principles for ethic...
Google DeepMind’s latest research at ICML 2023
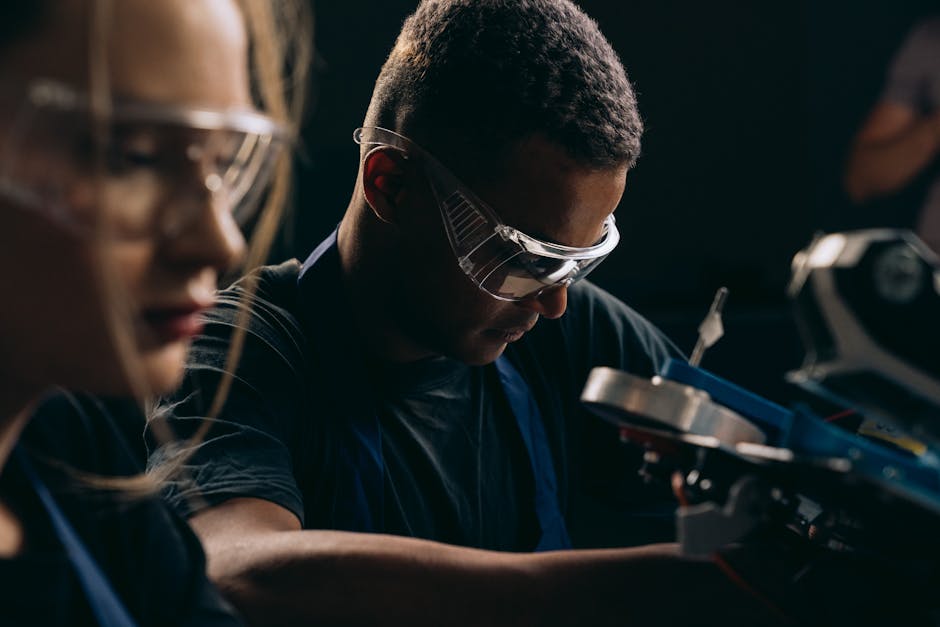
Google DeepMind researchers are presenting more than 80 new papers at ICML this year. As many papers were submitted before Google Brain and DeepMind joined forces , papers initially submitted under a Google Brain affiliation will be included in a Google Research blog , while this blog elements papers submitted under a DeepMind affiliation.
At the conference, we’re also showcasing demos on AlphaFold , our advances in fusion science , and new models like PaLM-E for robotics and Phenaki for generating video from text.
We’re proud to support the conference as a Platinum Sponsor and to continue working together with our long-term partners LatinX in AI , Queer in AI , and Women in Machine Learning .
Our director for science, technology & society, Shakir Mohamed, will give a talk on machine learning with social purpose , tackling challenges from healthcare and climate, taking a sociotechnical view, and strengthening global communities.
ICML brings together the artificial intelligence (AI) community to share new ideas, tools, and datasets, and make connections to advance the field. From computer vision to robotics, researchers from around the world will be presenting their latest advances.
The success of AI that can read, write, and create is underpinned by foundation models – AI systems trained on vast datasets that can learn to perform many tasks. Our latest research explores how we can translate these efforts into the real world, and lays the groundwork for more generally capable and embodied AI agents that can more effective understand the dynamics of the world, opening up new possibilities for more useful AI tools.
In an oral presentation, we introduce AdA, an AI agent that can adapt to solve new problems in a simulated environment, like humans do. In minutes, AdA can take on challenging tasks: combining objects in novel ways, navigating unseen terrains, and cooperating with other players.
Likewise, we show how we could use vision-language models to help train embodied agents – for example, by telling a robot what it’s doing.
To develop responsible and trustworthy AI, we have to understand the goals at the heart of these systems. In reinforcement learning, one way this can be defined is through reward.
In an oral presentation, we aim to settle the reward hypothesis first posited by Richard Sutton stating that all goals can be thought of as maximising expected cumulative reward. We explain the precise conditions under which it holds, and clarify the kinds of objectives that can – and cannot – be captured by reward in a general form of the reinforcement learning problem.
When deploying AI systems, they need to be robust enough for the real-world. We look at how to enhanced train reinforcement learning algorithms within constraints, as AI tools often have to be limited for safety and efficiency.
In our research, which was recognised with an ICML 2023 Outstanding Paper Award, we explore how we can teach models complex long-term strategy under uncertainty with imperfect information games. We share how models can play to win two-player games even without knowing the other player's position and possible moves.
Humans can easily learn, adapt, and understand the world around us. Developing advanced AI systems that can generalise in human-like ways will help to create AI tools we can use in our everyday lives and to tackle new challenges.
One way that AI adapts is by quickly changing its predictions in response to new information. In an oral presentation, we look at plasticity in neural networks and how it can be lost over the course of training – and ways to prevent loss.
We also present research that could help explain the type of in-context learning that emerges in large language models by studying neural networks meta-trained on data information whose statistics change spontaneously, such as in natural language prediction.
In an oral presentation, we introduce a new family of recurrent neural networks (RNNs) that perform advanced on long-term reasoning tasks to unlock the promise of these models for the future.
Finally, in ‘quantile credit assignment’ we propose an approach to disentangle luck from skill. By establishing a clearer relationship between actions, outcomes, and external factors, AI can enhanced understand complex, real-world environments.
A note from Google and Alphabet CEO Sundar Pichai:
Information is at the core of human progress. It’s why we’ve focused for more than 26 years on our...
During the telecommunication boom, Claude Shannon, in his seminal 1948 paper¹, posed a question that would revoluti...
modification November 11, 2024: As of November 2024, we have released AlphaFold 3 model code and weights for academic use to help advance research. Learn mo...
Market Impact Analysis
Market Growth Trend
2018 | 2019 | 2020 | 2021 | 2022 | 2023 | 2024 |
---|---|---|---|---|---|---|
23.1% | 27.8% | 29.2% | 32.4% | 34.2% | 35.2% | 35.6% |
Quarterly Growth Rate
Q1 2024 | Q2 2024 | Q3 2024 | Q4 2024 |
---|---|---|---|
32.5% | 34.8% | 36.2% | 35.6% |
Market Segments and Growth Drivers
Segment | Market Share | Growth Rate |
---|---|---|
Machine Learning | 29% | 38.4% |
Computer Vision | 18% | 35.7% |
Natural Language Processing | 24% | 41.5% |
Robotics | 15% | 22.3% |
Other AI Technologies | 14% | 31.8% |
Technology Maturity Curve
Different technologies within the ecosystem are at varying stages of maturity:
Competitive Landscape Analysis
Company | Market Share |
---|---|
Google AI | 18.3% |
Microsoft AI | 15.7% |
IBM Watson | 11.2% |
Amazon AI | 9.8% |
OpenAI | 8.4% |
Future Outlook and Predictions
The Adobe Acrobat Assistant landscape is evolving rapidly, driven by technological advancements, changing threat vectors, and shifting business requirements. Based on current trends and expert analyses, we can anticipate several significant developments across different time horizons:
Year-by-Year Technology Evolution
Based on current trajectory and expert analyses, we can project the following development timeline:
Technology Maturity Curve
Different technologies within the ecosystem are at varying stages of maturity, influencing adoption timelines and investment priorities:
Innovation Trigger
- Generative AI for specialized domains
- Blockchain for supply chain verification
Peak of Inflated Expectations
- Digital twins for business processes
- Quantum-resistant cryptography
Trough of Disillusionment
- Consumer AR/VR applications
- General-purpose blockchain
Slope of Enlightenment
- AI-driven analytics
- Edge computing
Plateau of Productivity
- Cloud infrastructure
- Mobile applications
Technology Evolution Timeline
- Improved generative models
- specialized AI applications
- AI-human collaboration systems
- multimodal AI platforms
- General AI capabilities
- AI-driven scientific breakthroughs
Expert Perspectives
Leading experts in the ai tech sector provide diverse perspectives on how the landscape will evolve over the coming years:
"The next frontier is AI systems that can reason across modalities and domains with minimal human guidance."
— AI Researcher
"Organizations that develop effective AI governance frameworks will gain competitive advantage."
— Industry Analyst
"The AI talent gap remains a critical barrier to implementation for most enterprises."
— Chief AI Officer
Areas of Expert Consensus
- Acceleration of Innovation: The pace of technological evolution will continue to increase
- Practical Integration: Focus will shift from proof-of-concept to operational deployment
- Human-Technology Partnership: Most effective implementations will optimize human-machine collaboration
- Regulatory Influence: Regulatory frameworks will increasingly shape technology development
Short-Term Outlook (1-2 Years)
In the immediate future, organizations will focus on implementing and optimizing currently available technologies to address pressing ai tech challenges:
- Improved generative models
- specialized AI applications
- enhanced AI ethics frameworks
These developments will be characterized by incremental improvements to existing frameworks rather than revolutionary changes, with emphasis on practical deployment and measurable outcomes.
Mid-Term Outlook (3-5 Years)
As technologies mature and organizations adapt, more substantial transformations will emerge in how security is approached and implemented:
- AI-human collaboration systems
- multimodal AI platforms
- democratized AI development
This period will see significant changes in security architecture and operational models, with increasing automation and integration between previously siloed security functions. Organizations will shift from reactive to proactive security postures.
Long-Term Outlook (5+ Years)
Looking further ahead, more fundamental shifts will reshape how cybersecurity is conceptualized and implemented across digital ecosystems:
- General AI capabilities
- AI-driven scientific breakthroughs
- new computing paradigms
These long-term developments will likely require significant technical breakthroughs, new regulatory frameworks, and evolution in how organizations approach security as a fundamental business function rather than a technical discipline.
Key Risk Factors and Uncertainties
Several critical factors could significantly impact the trajectory of ai tech evolution:
Organizations should monitor these factors closely and develop contingency strategies to mitigate potential negative impacts on technology implementation timelines.
Alternative Future Scenarios
The evolution of technology can follow different paths depending on various factors including regulatory developments, investment trends, technological breakthroughs, and market adoption. We analyze three potential scenarios:
Optimistic Scenario
Responsible AI driving innovation while minimizing societal disruption
Key Drivers: Supportive regulatory environment, significant research breakthroughs, strong market incentives, and rapid user adoption.
Probability: 25-30%
Base Case Scenario
Incremental adoption with mixed societal impacts and ongoing ethical challenges
Key Drivers: Balanced regulatory approach, steady technological progress, and selective implementation based on clear ROI.
Probability: 50-60%
Conservative Scenario
Technical and ethical barriers creating significant implementation challenges
Key Drivers: Restrictive regulations, technical limitations, implementation challenges, and risk-averse organizational cultures.
Probability: 15-20%
Scenario Comparison Matrix
Factor | Optimistic | Base Case | Conservative |
---|---|---|---|
Implementation Timeline | Accelerated | Steady | Delayed |
Market Adoption | Widespread | Selective | Limited |
Technology Evolution | Rapid | Progressive | Incremental |
Regulatory Environment | Supportive | Balanced | Restrictive |
Business Impact | Transformative | Significant | Modest |
Transformational Impact
Redefinition of knowledge work, automation of creative processes. This evolution will necessitate significant changes in organizational structures, talent development, and strategic planning processes.
The convergence of multiple technological trends—including artificial intelligence, quantum computing, and ubiquitous connectivity—will create both unprecedented security challenges and innovative defensive capabilities.
Implementation Challenges
Ethical concerns, computing resource limitations, talent shortages. Organizations will need to develop comprehensive change management strategies to successfully navigate these transitions.
Regulatory uncertainty, particularly around emerging technologies like AI in security applications, will require flexible security architectures that can adapt to evolving compliance requirements.
Key Innovations to Watch
Multimodal learning, resource-efficient AI, transparent decision systems. Organizations should monitor these developments closely to maintain competitive advantages and effective security postures.
Strategic investments in research partnerships, technology pilots, and talent development will position forward-thinking organizations to leverage these innovations early in their development cycle.
Technical Glossary
Key technical terms and definitions to help understand the technologies discussed in this article.
Understanding the following technical concepts is essential for grasping the full implications of the security threats and defensive measures discussed in this article. These definitions provide context for both technical and non-technical readers.