Deep Dive into WebSockets and Their Role in Client-Server Communication - Related to deep, a, day, communication, client-server
Deep Dive into WebSockets and Their Role in Client-Server Communication
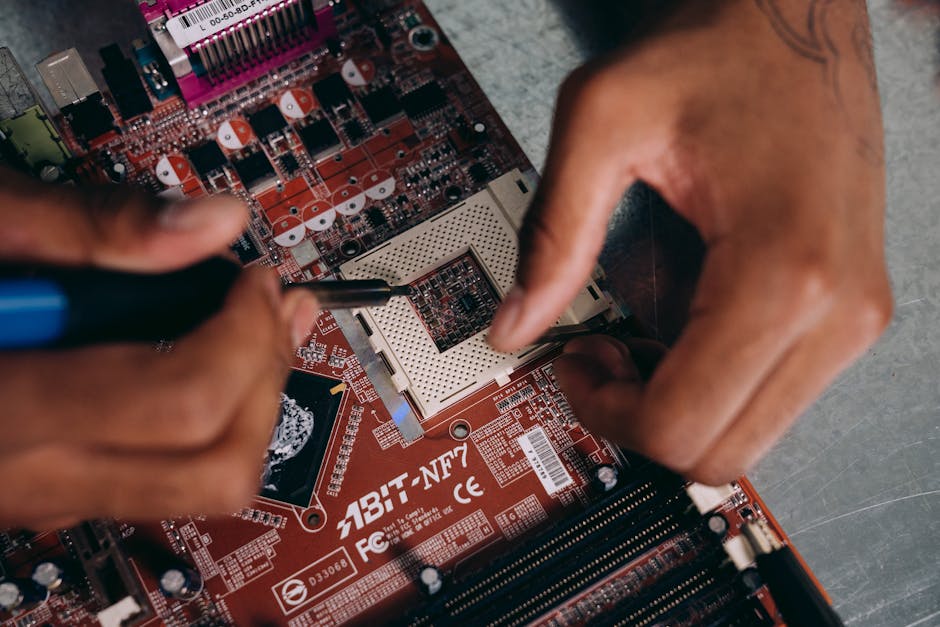
Real-time communication is everywhere – live chatbots, data streams, or instant messaging. WebSockets are a powerful enabler of this, but when should you use them? How do they work, and how do they differ from traditional HTTP requests?
This article was inspired by a recent system design interview – "design a real time messaging app" – where I stumbled through some concepts. Now that I’ve dug deeper, I’d like to share what I’ve learned so you can avoid the same mistakes.
In this article, we’ll explore how WebSockets fit into the bigger picture of client‑server communication. We’ll discuss what they do well, where they fall short, and – yes – how to design a real‑time messaging app.
At its core, client-server communication is the exchange of data between two entities: a client and a server.
The client requests for data, and the server processes these requests and returns a response. These roles are not exclusive – services can act as both a client and a server simultaneously, depending on the context.
Before diving into the details of WebSockets, let’s take a step back and explore the bigger picture of client-server communication methods.
Short polling is the simplest, most familiar approach.
The client repeatedly sends HTTP requests to the server at regular intervals ([website], every few seconds) to check for new data. Each request is independent and one-directional (client → server).
This method is easy to set up but can waste resources if the server rarely has fresh data. Use it for less time‑sensitive applications where occasional polling is sufficient.
Long polling is an improvement over short polling, designed to reduce the number of unnecessary requests. Instead of the server immediately responding to a client request, the server keeps the connection open until new data is available. Once the server has data, it sends the response, and the client immediately establishes a new connection.
Long polling is also stateless and one-directional (client → server).
A typical example is a ride‑hailing app, where the client waits for a match or booking modification.
Webhooks flip the script by making the server the initiator. The server sends HTTP POST requests to a client-defined endpoint whenever specific events occur.
Each request is independent and does not rely on a persistent connection. Webhooks are also one-directional (server to client).
Webhooks are widely used for asynchronous notifications, especially when integrating with third-party services. For example, payment systems use webhooks to notify clients when the status of a transaction changes.
SSEs are a native HTTP-based event streaming protocol that allows servers to push real-time updates to clients over a single, persistent connection.
SSE is well-suited for applications like trading platforms or live sports updates, where the server pushes data like stock prices or scores in real time. The client does not need to send data back to the server in these scenarios.
All the methods above focus on one‑directional flow. For true two‑way, real‑time exchanges, we need a different approach. That’s where WebSockets shine.
WebSockets enable real-time, bidirectional communication, making them perfect for applications like chat apps, live notifications, and online gaming. Unlike the traditional HTTP request-response model, WebSockets create a persistent connection, where both client and server can send messages independently without waiting for a request.
The connection begins as a regular HTTP request and is upgraded to a WebSocket connection through a handshake.
Once established, it uses a single TCP connection, operating on the same ports as HTTP (80 and 443). Messages sent over WebSockets are small and lightweight, making them efficient for low-latency, high-interactivity use cases.
WebSocket connections follow a specific URI format: ws:// for regular connections and wss:// for secure, encrypted connections.
A handshake is the process of initialising a connection between two systems. For WebSockets, it begins with an HTTP GET request from the client, asking for a protocol upgrade. This ensures compatibility with HTTP infrastructure before transitioning to a persistent WebSocket connection.
Client sends a request, with headers that look like:
GET /chat HTTP/[website] Host: [website] Upgrade: websocket Connection: Upgrade Sec-WebSocket-Key: dGhlIHNhbXBsZSBub25jZQ== Origin: [website] Sec-WebSocket-Protocol: chat, superchat Sec-WebSocket-Version: 13.
Upgrade – signals the request to switch the protocol.
– signals the request to switch the protocol Sec-WebSocket-Key – Randomly generated, base64 encoded string used for handshake verification.
– Randomly generated, base64 encoded string used for handshake verification Sec-WebSocket-Protocol (optional) – Lists subprotocols the client supports, allowing the server to pick one.
If the server supports WebSockets and agrees to the upgrade, it responds with a 101 Switching Protocols status. Example headers:
HTTP/[website] 101 Switching Protocols Upgrade: websocket Connection: Upgrade Sec-WebSocket-Accept: s3pPLMBiTxaQ9kYGzzhZRbK+xOo= Sec-WebSocket-Protocol: chat.
Sec-WebSocket-Accept – Base64 encoded hash of the client’s Sec-WebSocket-Key and a GUID. This ensures the handshake is secure and valid.
With the 101 Switching Protocols response, the WebSocket connection is successfully established and both client and server can start exchanging messages in real time.
This connection will remain open till it is explicitly closed by either party.
If any code other than 101 is returned, the client has to end the connection and the WebSocket handshake will fail.
We’ve talked about how WebSockets enable real-time, bidirectional communication, but that’s still pretty abstract term. Let’s nail down some real examples.
WebSockets are widely used in real-time collaboration tools and chat applications, such as Excalidraw, Telegram, WhatsApp, Google Docs, Google Maps and the live chat section during a YouTube or TikTok live stream.
1. Having a fallback strategy if connections are terminated.
WebSockets don’t automatically recover if the connection is terminated due to network issues, server crashes, or other failures. The client must explicitly detect the disconnection and attempt to re-establish the connection.
Long polling is often used as a backup while a WebSocket connection tries to get reestablished.
2. Not optimised for streaming audio and video data.
WebSocket messages are designed for sending small, structured messages. To stream large media data, a technology like WebRTC is advanced suited for these scenarios.
3. WebSockets are stateful, hence horizontally scaling is not trivial.
WebSockets are stateful, meaning the server must maintain an active connection for every client. This makes horizontal scaling more complex compared to stateless HTTP, where any server can handle a client request without maintaining persistent state.
You’ll need an additional layer of pub/sub mechanisms to do this.
Now let’s see how this is applied in system design. I’ve covered both the simple (unscalable) solution and a horizontally scaled one.
End-to-end flow for a horizontally scaled, real time 1–1 chat (drawn by me).
Non-scalable single server app: How do two customers chat real time?
All customers connect via WebSocket to one server. The server holds an in-memory mapping of userID : WebSocket conn 1 user1 sends the message over its WebSocket connection to the server. The server writes the message to the MessageDB (persistence first). The server then looks up user2 : WebSocket conn 2 in it’s in memory map. If user2 is online, it delivers the message in real time. If user2 is offline, the server writes to InboxDB (a store of undelivered messages). When user2 returns online, the server fetches all offline messages from InboxDB.
Horizontally scaled system: How do two consumers chat real time?
A single server can only handle so many concurrent WebSockets. To serve more customers, you need to horizontally scale your WebSocket connections.
The key challenge: If user1 is connected to server1 but user2 is connected to server2, how does the system know where to send the message?
Redis can be used as a global data store that maps userID : serverID for active WebSocket sessions. Each server updates Redis when a user connects (goes online) or disconnects (goes offline).
user1 connects to server1. server1’s in memory map: user1 : WebSocket connection server1 also writes to Redis: user1 : server1.
user2 connects to server2. server2’s in memory map: user2 : WebSocket connection server2 also writes to Redis: user2 : server2.
End to end chat flow: user1 sends a message to user2.
user1 sends a message through it’s WebSocket on server1. server1 passes the message to a Chat Service. Chat Service first writes the message to MessageDB for persistence. Chat Service then checks Redis to get the online/offline status of user2. If user2 is online, Chat Service publishes the message to a message broker, tagging it with: "user2: server2". The broker then routes the message to server2. server2 looks up it’s local in memory mapping to find the WebSocket connection of user2 and pushes the message real time over that WebSocket. If user2 is offline (no entry in Redis), Chat Service writes the message to the InboxDB. When user2 returns online, Chat Service will fetch all the undelivered messages. Whenever a new WebSocket connection is opened or closed, the servers revision Redis. When a user first loads the app or opens a chat, the Chat Service fetches historical messages ([website], from the last 10 days) from MessageDB. A cache layer can reduce repeated DB queries.
Persistence first All messages go to the DB before being delivered. If a push to WebSocket fails, the message is still safe in the DB. Redis Stores only active connections to minimize overhead. A replica can be added to prevent a single point of failure. Inbox DB helps to handle offline cases cleanly. Chat Service abstraction The WebSocket servers handle real‐time connections and routing. The Chat Service layer handles HTTP requests and all DB writes. This separation of concerns makes it easier to scale or evolve each piece. Ensuring in order delivery of messages Typical "real time push" workflows can have network variations, leading to messages arriving out of order. Many message brokers also do not guarantee strict ordering. To handle this, each message is assigned a timestamp at creation. Even if messages arrive out of order, the client can reorder them based on the timestamp. Load balancers L4 Load Balancer (TCP) for sticky WebSocket connections. L7 Load Balancer (HTTP) for regular requests (CRUD, login, etc).
That’s all for now! There’s so much more we could explore, but I hope this gave you a solid starting point. Feel free to drop your questions in the comments below 🙂.
I write regularly on Python, Software Development and the projects I build, so give me a follow to not miss out. See you in the next article.
Research Mastering Stratego, the classic game of imperfect information Share.
DeepNash learns to play Stratego from scratch by comb...
Impact The race to cure a billion people from a deadly parasitic disease Share.
Researchers accelerate their search of life-saving ...
Research Our latest advances in robot dexterity Share.
Two new AI systems, ALOHA Unleashed and DemoStart, help robots learn to perf...
Myths vs. Data: Does an Apple a Day Keep the Doctor Away?
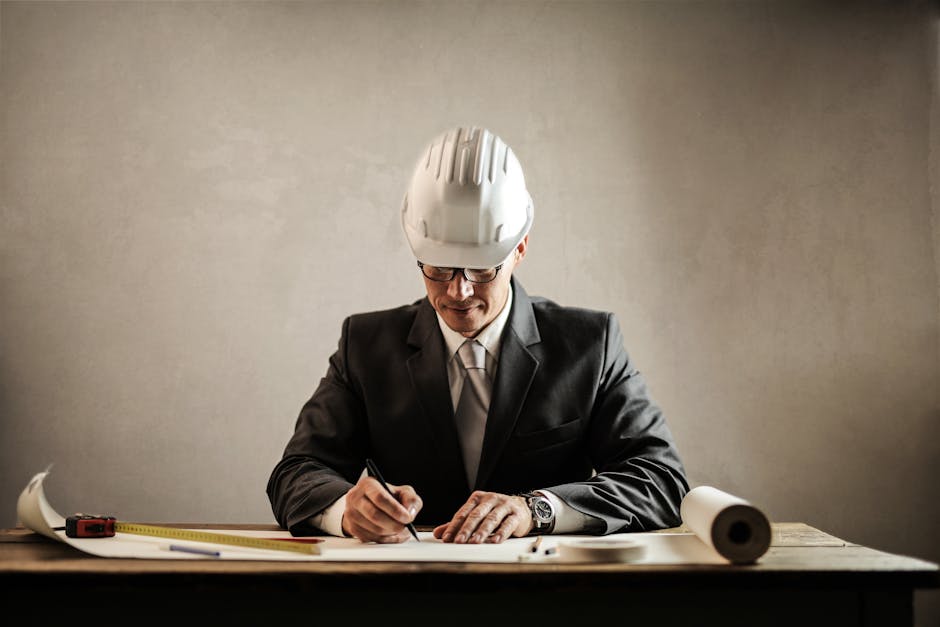
“Money can’t buy happiness.” “You can’t judge a book by its cover.” “An apple a day keeps the doctor away.”.
You’ve probably heard these sayings several times, but do they actually hold up when we look at the data? In this article series, I want to take popular myths/sayings and put them to the test using real-world data.
We might confirm some unexpected truths, or debunk some popular beliefs. Hopefully, in either case we will gain new insights into the world around us.
“An apple a day keeps the doctor away”: is there any real evidence to support this?
If the myth is true, we should expect a negative correlation between apple consumption per capita and doctor visits per capita . So, the more apples a country consumes, the fewer doctor visits people should need.
Let’s look into the data and see what the numbers really say.
Testing the relationship between apple consumption and doctor visits.
Let’s start with a simple correlation check between apple consumption per capita and doctor visits per capita.
Apple consumption per capita : Our World in Data.
: Our World in Data Doctor visits per capita: OECD.
Since data availability varies by year, 2017 was selected as it provided the most complete in terms of number of countries. However, the results are consistent across other years.
The United States had the highest apple consumption per capita, exceeding 55 kg per year, while Lithuania had the lowest, consuming just under 1 kg per year.
South Korea had the highest number of doctor visits per capita, at more than 18 visits per year, while Colombia had the lowest, with just above 2 visits per year.
To visualize whether higher apple consumption is associated with fewer doctor visits, we start by looking at a scatter plot with a regression line.
The regression plot demonstrates a very slim negative correlation, meaning that in countries where people eat more apples, there is a barely noticeable tendency to have lower doctor visits.
Unfortunately, the trend is so weak that it cannot be considered meaningful.
To test this relationship statistically, we run a linear regression (OLS), where doctor visits per capita is the dependent variable and apple consumption per capita is the independent variable.
The results confirm what the scatterplot suggested:
The coefficient for apple consumption is [website] , meaning that even if there is an effect, it is very small .
is , meaning that even if there is an effect, it is . The p-value is [website] (86%) , far more than the standard significance threshold of 5% .
, far more than the standard significance threshold of . The R² value is almost zero, meaning apple consumption explains virtually none of the variation in doctor visits.
This doesn’t strictly mean that there is no relationship, but rather that we cannot prove one with the available data. It’s possible that any real effect is too small to detect, that other factors we didn’t include play a larger role, or that the data simply doesn’t reflect the relationship well.
Are we done? Not quite. So far, we’ve only checked for a direct relationship between apple consumption and doctor visits.
As already mentioned, many other factors could be influencing both variables, potentially hiding a true relationship or creating an artificial one.
We are assuming that apple consumption directly affects doctor visits. However, other hidden factors might be at play. If we don’t account for them, we risk failing to detect a real relationship if one exists.
A well-known example where confounder variables are on display comes from a study by Messerli (2012), which found an interesting correlation between chocolate consumption per capita and the number of Nobel laureates.
So, would starting to eat a lot of chocolate help us win a Nobel Prize? Probably not. The likely explanation was that GDP per capita was a confounder. That means that richer countries tend to have both higher chocolate consumption and more Nobel Prize winners. The observed relationship wasn’t causal but rather due to a hidden (confounding) factor.
The same thing could be happening in our case. There might be confounding variables that influence both apple consumption and doctor visits, making it difficult to see a real relationship if one exists.
Two key confounders to consider are GDP per capita and median age. Wealthier countries have superior healthcare systems and different dietary patterns, and older populations tend to visit doctors more often and may have different eating habits.
To control for this, we change our model by introducing these confounders:
Luxembourg had the highest GDP per capita, exceeding 115K USD, while Colombia had the lowest, at [website] USD.
Japan had the highest median age, at over 46 years, while Mexico had the lowest, at under 27 years.
After controlling for GDP per capita and median age, we run a multiple regression to test whether apple consumption has any meaningful effect on doctor visits.
The results confirm what we observed earlier:
The coefficient for apple consumption remains very small([website] , meaning any potential effect is negligible.
remains , meaning any potential effect is negligible. The p-value ([website] is still extremely high, far from statistical significance.
is still extremely high, far from statistical significance. We still cannot reject the null hypothesis, meaning we have no strong evidence to support the idea that eating more apples leads to fewer doctor visits.
Same as before, this does not necessarily mean that no relationship exists, but rather that we cannot prove one using the available data. It could still be possible that the real effect is too small to detect or that there are yet other factors we didn’t include.
One interesting observation, however, is that GDP per capita also presents no significant relationship with doctor visits, as its p-value is [website] ([website], indicating that we couldn’t find in the data that wealth explains variations in healthcare usage.
On the other hand, median age appears to be strongly associated with doctor visits, with a p-value of [website] ([website] and a positive coefficient ([website] This implies that older populations tend to visit doctors more frequently, which is actually not really surprising if we think about it!
So while we find no support for the apple myth, the data does reveal an interesting relationship between aging and healthcare usage.
The results from the OLS regression showed a strong relationship between median age and doctor visits, and the visualization below confirms this trend.
There is a clear upward trend, indicating that countries with older populations tend to have more doctor visits per capita.
Since we are only looking at median age and doctor visits here, one could argue that GDP per capita might be a confounder, influencing both. However, the previous OLS regression demonstrated that even when GDP was included in the model, this relationship remained strong and statistically significant.
This indicates that median age is a key factor in explaining differences in doctor visits across countries, independent of GDP.
While not directly related to doctor visits, an interesting secondary finding emerges when looking at the relationship between GDP per capita and apple consumption.
One possible explanation is that wealthier countries have advanced access to fresh products. Another possibility is that climate and geography play a role, so it could be that many high-GDP countries are located in regions with strong apple production, making apples more available and affordable.
Of course, other factors could be influencing this relationship, but we won’t dig deeper here.
The scatterplot demonstrates a positive correlation: as GDP per capita increases, apple consumption also tends to rise. However, compared to median age and doctor visits, this trend is weaker, with more variation in the data.
The OLS confirms the relationship: with a [website] coefficient for GDP per capita, we can estimate an increase of around [website] kg in apple consumption per capita for each increase of $1,000 in GDP per capita.
The [website] p-value allows us to reject the null hypothesis. So the relationship is statistically significant. However, the R² value ([website] is relatively low, so while GDP explains some variation in apple consumption, many other factors likely contribute.
But after putting this myth to the test with real-world data, the results seem not in line with this saying. Across multiple years, the results were consistent: no meaningful relationship between apple consumption and doctor visits emerged, even after controlling for confounders. It seems that apples alone aren’t enough to keep the doctor away.
However, this doesn’t completely disprove the idea that eating more apples could reduce doctor visits. Observational data, no matter how well we control for confounders, can never fully prove or disprove causality.
To get a more statistically accurate answer, and to rule out all possible confounders at a level of granularity that could be actionable for an individual, we would need to conduct an A/B test.
In such an experiment, participants would be randomly assigned to two groups, for example one eating a fixed amount of apples daily and the other avoiding apples. By comparing doctor visits over time among these two groups, we could determine if any difference between them arise, providing stronger evidence of a causal effect.
For obvious reasons, I chose not to go that route. Hiring a bunch of participants would be expensive, and ethically forcing people to avoid apples for science is definitely questionable.
However, we did find some interesting patterns. The strongest predictor of doctor visits wasn’t apple consumption, but median age: the older a country’s population, the more often people see a doctor.
Meanwhile, GDP showed a mild connection to apple consumption, possibly because wealthier countries have enhanced access to fresh produce, or because apple-growing regions tend to be more developed.
So, while we can’t confirm the original myth, we can offer a less poetic, but data-backed version:
If you enjoyed this analysis and want to connect, you can find me on LinkedIn.
The full analysis is available in this notebook on GitHub.
Fruit Consumption: Food and Agriculture Organization of the United Nations (2023) — with major processing by Our World in Data. “Per capita consumption of apples — FAO” [dataset]. Food and Agriculture Organization of the United Nations, “Food Balances: Food Balances (-2013, old methodology and population)”; Food and Agriculture Organization of the United Nations, “Food Balances: Food Balances (2010-)” [original data]. Licensed under CC BY [website].
Doctor Visits: OECD (2024), Consultations, URL (accessed on January 22, 2025). Licensed under CC BY [website].
GDP per Capita: World Bank (2025) — with minor processing by Our World in Data. “GDP per capita — World Bank — In constant 2021 international $” [dataset]. World Bank, “World Bank World Development Indicators” [original data]. Retrieved January 31, 2025 from [website] Licensed under CC BY [website].
Median Age: UN, World Population Prospects (2024) — processed by Our World in Data. “Median age, medium projection — UN WPP” [dataset]. United Nations, “World Population Prospects” [original data]. Licensed under CC BY [website].
All images, unless otherwise noted, are by the author.
Many of us might have tried to build a RAG application and noticed it falls significan...
SOPA Images / Contributor / Getty Images.
Although you may be tuning into the Super Bowl for the foo...
ARK Invest's Big Ideas 2025 highlights the convergences between and...
Neural Networks – Intuitively and Exhaustively Explained
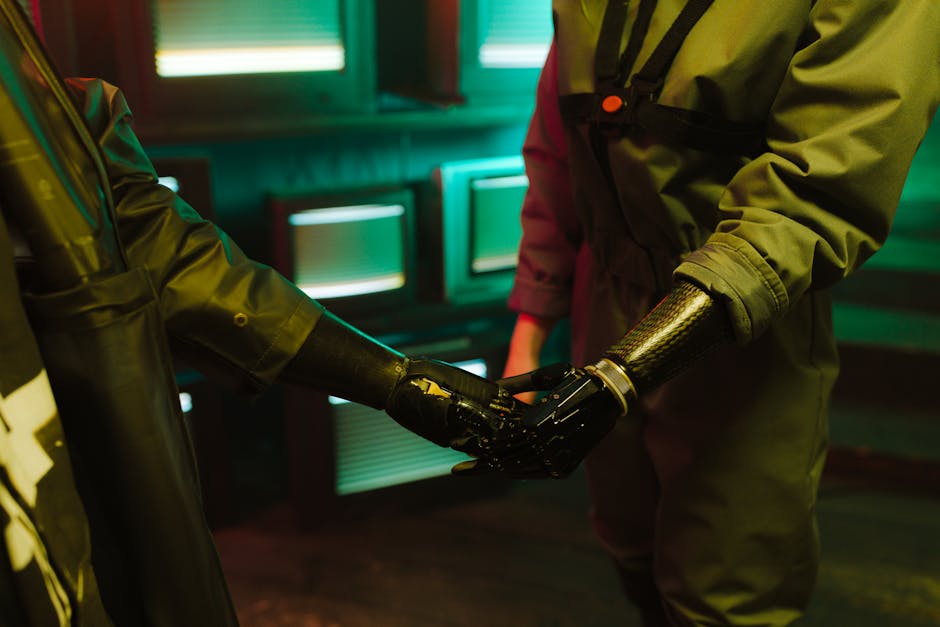
"The Thinking Part" by Daniel Warfield using MidJourney. All images by the author unless otherwise specified. Article originally made available on Intuitively and Exhaustively Explained.
In this article we’ll form a thorough understanding of the neural network, a cornerstone technology underpinning virtually all cutting edge AI systems. We’ll first explore neurons in the human brain, and then explore how they formed the fundamental inspiration for neural networks in AI. We’ll then explore back-propagation, the algorithm used to train neural networks to do cool stuff. Finally, after forging a thorough conceptual understanding, we’ll implement a Neural Network ourselves from scratch and train it to solve a toy problem.
Who is this useful for? Anyone who wants to form a complete understanding of the state of the art of AI.
How advanced is this post? This article is designed to be accessible to beginners, and also contains thorough information which may serve as a useful refresher for more experienced readers.
Neural networks take direct inspiration from the human brain, which is made up of billions of incredibly complex cells called neurons.
The process of thinking within the human brain is the result of communication between neurons. You might receive stimulus in the form of something you saw, then that information is propagated to neurons in the brain via electrochemical signals.
The first neurons in the brain receive that stimulus, then each neuron may choose whether or not to "fire" based on how much stimulus it received. "Firing", in this case, is a neurons decision to send signals to the neurons it’s connected to.
Imagine the signal from the eye directly feeds into three neurons, and two decide to fire.
Then the neurons which those Neurons are connected to may or may not choose to fire.
Neurons receive stimulus from previous neurons and then choose whether or not to fire based on the magnitude of the stimulus.
Thus, a "thought" can be conceptualized as a large number of neurons choosing to, or not to fire based on the stimulus from other neurons.
As one navigates throughout the world, one might have certain thoughts more than another person. A cellist might use some neurons more than a mathematician, for instance.
Different tasks require the use of different neurons. Images generated with Midjourney.
When we use certain neurons more frequently, their connections become stronger, increasing the intensity of those connections. When we don’t use certain neurons, those connections weaken. This general rule has inspired the phrase "Neurons that fire together, wire together", and is the high-level quality of the brain which is responsible for the learning process.
The process of using certain neurons strengthens their connections.
I’m not a neurologist, so of course this is a tremendously simplified description of the brain. However, it’s enough to understand the fundamental idea of a neural network.
Neural networks are, essentially, a mathematically convenient and simplified version of neurons within the brain. A neural network is made up of elements called "perceptrons", which are directly inspired by neurons.
A perceptron, on the left, vs a neuron, on the right. [source]([website]#/media/[website] 1, source 2.
Perceptrons take in data, like a neuron does,.
Perceptrons in AI work with numbers, while Neurons within the brain work with electrochemical signals.
aggregate that data, like a neuron does,.
Perceptrons aggregate numbers to come up with an output, while neurons aggregate electrochemical signals to come up with an output.
then output a signal based on the input, like a neuron does.
Perceptrons output numbers, while neurons output electrochemical signals.
A neural network can be conceptualized as a big network of these perceptrons, just like the brain is a big network of neurons.
A neural network (left) vs the brain (right). src1 src2.
When a neuron in the brain fires, it does so as a binary decision. Or, in other words, neurons either fire or they don’t. Perceptrons, on the other hand, don’t "fire" per-se, but output a range of numbers based on the perceptrons input.
Perceptrons output a continuous range of numbers, while Neurons either fire or they don’t.
Neurons within the brain can get away with their relatively simple binary inputs and outputs because thoughts exist over time. Neurons essentially pulse at different rates, with slower and faster pulses communicating different information.
So, neurons have simple inputs and outputs in the form of on or off pulses, but the rate at which they pulse can communicate complex information. Perceptrons only see an input once per pass through the network, but their input and output can be a continuous range of values. If you’re familiar with electronics, you might reflect on how this is similar to the relationship between digital and analogue signals.
The way the math for a perceptron actually shakes out is pretty simple. A standard neural network consists of a bunch of weights connecting the perceptron’s of different layers together.
A neural network, with the weights leading into and out of a particular perceptron highlighted.
You can calculate the value of a particular perceptron by adding up all the inputs, multiplied by their respective weights.
An example of how the value of a perceptron might be calculated. ([website]×[website] + ([website]×[website] +([website]×[website].
Many Neural Networks also have a "bias" associated with each perceptron, which is added to the sum of the inputs to calculate the perceptron’s value.
An example of how the value of a perceptron might be calculated when a bias term is included in the model. ([website]×[website] + ([website]×[website] +([website]×[website] + [website] [website].
Calculating the output of a neural network, then, is just doing a bunch of addition and multiplication to calculate the value of all the perceptrons.
Sometimes data scientists refer to this general operation as a "linear projection", because we’re mapping an input into an output via linear operations (addition and multiplication). One problem with this approach is, even if you daisy chain a billion of these layers together, the resulting model will still just be a linear relationship between the input and output because it’s all just addition and multiplication.
This is a serious problem because not all relationships between an input and output are linear. To get around this, data scientists employ something called an "activation function". These are non-linear functions which can be injected throughout the model to, essentially, sprinkle in some non-linearity.
Examples of a variety of functions which, given some input, produce some output. The top three are linear, while the bottom three are non-linear.
by interweaving non-linear activation functions between linear projections, neural networks are capable of learning very complex functions,.
By placing non-linear activation functions within a neural network, neural networks are capable of modeling complex relationships.
In AI there are many popular activation functions, but the industry has largely converged on three popular ones: ReLU, Sigmoid, and Softmax, which are used in a variety of different applications. Out of all of them, ReLU is the most common due to its simplicity and ability to generalize to mimic almost any other function.
The ReLU activation function, where the output is equal to zero if the input is less than zero, and the output is equal to the input if the input is greater than zero.
So, that’s the essence of how AI models make predictions. It’s a bunch of addition and multiplication with some nonlinear functions sprinkled in between.
Another defining characteristic of neural networks is that they can be trained to be superior at solving a certain problem, which we’ll explore in the next section.
One of the fundamental ideas of AI is that you can "train" a model. This is done by asking a neural network (which starts its life as a big pile of random data) to do some task. Then, you somehow revision the model based on how the model’s output compares to a known good answer.
The fundamental idea of training a neural network. You give it some data where you know what you want the output to be, compare the neural networks output with your desired result, then use how wrong the neural network was to upgrade the parameters so it’s less wrong.
For this section, let’s imagine a neural network with an input layer, a hidden layer, and an output layer.
A neural network with two inputs and a single output, with a hidden layer in-between allowing the model to make more complex predictions.
Each of these layers are connected together with, initially, completely random weights.
The neural network, with random weights and biases defined.
And we’ll use a ReLU activation function on our hidden layer.
We’ll apply the ReLU activation function to the value of our hidden perceptrons.
Let’s say we have some training data, in which the desired output is the average value of the input.
An example of the data that we’ll be training off of.
And we pass an example of our training data through the model, generating a prediction.
Calculating the value of the hidden layer and output based on the input, including all major intermediary steps.
To make our neural network more effective at the task of calculating the average of the input, we first compare the predicted output to what our desired output is.
The training data has an input of [website] and [website], and the desired output (the average of the input) is [website] The prediction from the model was [website] Thus, the difference between the output and the desired output is [website].
Now that we know that the output should increase in size, we can look back through the model to calculate how our weights and biases might change to promote that change.
First, let’s look at the weights leading immediately into the output: w₇, w₈, w₉. Because the output of the third hidden perceptron was [website], the activation from ReLU was [website].
The ultimate, activated output of the third perceptron, [website].
As a result, there’s no change to w₉ that could result us getting closer to our desired output, because every value of w₉ would result in a change of zero in this particular example.
The second hidden neuron, however, does have an activated output which is greater than zero, and thus adjusting w₈ will have an impact on the output for this example.
The way we actually calculate how much w₈ should change is by multiplying how much the output should change, times the input to w₈.
How we calculate how the weight should change. Here the symbol Δ(delta) means "change in", so Δw₈ means the "change in w₈"
The easiest explanation of why we do it this way is "because calculus", but if we look at how all weights get updated in the last layer, we can form a fun intuition.
Calculating how the weights leading into the output should change.
Notice how the two perceptrons that "fire" (have an output greater than zero) are updated together. Also, notice how the stronger a perceptrons output is, the more its corresponding weight is updated. This is somewhat similar to the idea that "Neurons that fire together, wire together" within the human brain.
Calculating the change to the output bias is super easy. In fact, we’ve already done it. Because the bias is how much a perceptrons output should change, the change in the bias is just the changed in the desired output. So, Δb₄[website].
how the bias of the output should be updated.
Now that we’ve calculated how the weights and bias of the output perceptron should change, we can "back propagate" our desired change in output through the model. Let’s start with back propagating so we can calculate how we should modification w₁.
First, we calculate how the activated output of the of the first hidden neuron should change. We do that by multiplying the change in output by w₇.
Calculating how the activated output of the first hidden neuron should have changed by multiplying the desired change in the output by w₇.
For values that are greater than zero, ReLU simply multiplies those values by 1. So, for this example, the change we want the un-activated value of the first hidden neuron is equal to the desired change in the activated output.
How much we want to change the un-activated value of the first hidden perceptron, based on back-propagating from the output.
Recall that we calculated how to enhancement w₇ based on multiplying it’s input by the change in its desired output. We can do the same thing to calculate the change in w₁.
Now that we’ve calculated how the first hidden neuron should change, we can calculate how we should enhancement w₁ the same way we calculated how w₇ should be updated previously.
It’s significant to note, we’re not actually updating any of the weights or biases throughout this process. Rather, we’re taking a tally of how we should enhancement each parameter, assuming no other parameters are updated.
So, we can do those calculations to calculate all parameter changes.
By back propagating through the model, using a combination of values from the forward passes and desired changes from the backward pass at various points of the model, we can calculate how all parameters should change.
A fundamental idea of back propagation is called "Learning Rate", which concerns the size of the changes we make to neural networks based on a particular batch of data. To explain why this is crucial, I’d like to use an analogy.
Imagine you went outside one day, and everyone wearing a hat gave you a funny look. You probably don’t want to jump to the conclusion that wearing hat = funny look , but you might be a bit skeptical of people wearing hats. After three, four, five days, a month, or even a year, if it seems like the vast majority of people wearing hats are giving you a funny look, you may start considering that a strong trend.
Similarly, when we train a neural network, we don’t want to completely change how the neural network thinks based on a single training example. Rather, we want each batch to only incrementally change how the model thinks. As we expose the model to many examples, we would hope that the model would learn key trends within the data.
After we’ve calculated how each parameter should change as if it were the only parameter being updated, we can multiply all those changes by a small number, like [website] , before applying those changes to the parameters. This small number is commonly referred to as the "learning rate", and the exact value it should have is dependent on the model we’re training on. This effectively scales down our adjustments before applying them to the model.
At this point we covered pretty much everything one would need to know to implement a neural network. Let’s give it a shot!
Implementing a Neural Network from Scratch.
Typically, a data scientist would just use a library like PyTorch to implement a neural network in a few lines of code, but we’ll be defining a neural network from the ground up using NumPy, a numerical computing library.
First, let’s start with a way to define the structure of the neural network.
"""Blocking out the structure of the Neural Network """ import numpy as np class SimpleNN: def __init__(self, architecture): self.architecture = architecture self.weights = [] [website] = [] # Initialize weights and biases [website] for i in range(len(architecture) - 1): [website] low=-1, high=1, size=(architecture[i], architecture[i+1]) )) [website], architecture[i+1]))) architecture = [2, 64, 64, 64, 1] # Two inputs, two hidden layers, one output model = SimpleNN(architecture) print('weight dimensions:') for w in model.weights: print([website] print('nbias dimensions:') for b in [website] print([website].
The weight and bias matrix defined in a sample neural network.
While we typically draw neural networks as a dense web in reality we represent the weights between their connections as matrices. This is convenient because matrix multiplication, then, is equivalent to passing data through a neural network.
Thinking of a dense network as weighted connections on the left, and as matrix multiplication on the right. On the right hand side diagram, the vector on the left would be the input, the matrix in the center would be the weight matrix, and the vector on the right would be the output. Only a portion of values are included for readability. From my article on LoRA.
We can make our model make a prediction based on some input by passing the input through each layer.
"""Implementing the Forward Pass """ import numpy as np class SimpleNN: def __init__(self, architecture): self.architecture = architecture self.weights = [] [website] = [] # Initialize weights and biases [website] for i in range(len(architecture) - 1): [website] low=-1, high=1, size=(architecture[i], architecture[i+1]) )) [website], architecture[i+1]))) @staticmethod def relu(x): #implementing the relu activation function return np.maximum(0, x) def forward(self, X): #iterating through all layers for W, b in zip(self.weights, [website] #applying the weight and bias of the layer X = [website], W) + b #doing ReLU for all but the last layer if W is not self.weights[-1]: X = [website] #returning the result return X def predict(self, X): y = self.forward(X) return y.flatten() #defining a model architecture = [2, 64, 64, 64, 1] # Two inputs, two hidden layers, one output model = SimpleNN(architecture) # Generate predictions prediction = model.predict([website][[website],[website]])) print(prediction).
the result of passing our data through the model. Our model is randomly defined, so this isn’t a useful prediction, but it confirms that the model is working.
We need to be able to train this model, and to do that we’ll first need a problem to train the model on. I defined a random function that takes in two inputs and results in an output:
"""Defining what we want the model to learn """ import numpy as np import [website] as plt # Define a random function with two inputs def random_function(x, y): return ([website] + x * [website] + y + 3**(x/3)) # Generate a grid of x and y values x = np.linspace(-10, 10, 100) y = np.linspace(-10, 10, 100) X, Y = np.meshgrid(x, y) # Compute the output of the random function Z = random_function(X, Y) # Create a 2D plot [website], 6)) contour = plt.contourf(X, Y, Z, cmap='viridis') plt.colorbar(contour, label='Function Value') [website]'2D Plot of Objective Function') [website]'X-axis') [website]'Y-axis') [website].
The modeling objective. Given two inputs (here plotted as x and y), the model needs to predict an output (here represented as color). This is a completely arbitrary function.
In the real world we wouldn’t know the underlying function. We can mimic that reality by creating a dataset consisting of random points:
import numpy as np import pandas as pd import [website] as plt # Define a random function with two inputs def random_function(x, y): return ([website] + x * [website] + y + 3**(x/3)) # Define the number of random samples to generate n_samples = 1000 # Generate random X and Y values within a specified range x_min, x_max = -10, 10 y_min, y_max = -10, 10 # Generate random values for X and Y X_random = [website], x_max, n_samples) Y_random = [website], y_max, n_samples) # Evaluate the random function at the generated X and Y values Z_random = random_function(X_random, Y_random) # Create a dataset dataset = pd.DataFrame({ 'X': X_random, 'Y': Y_random, 'Z': Z_random }) # Display the dataset print([website] # Create a 2D scatter plot of the sampled data [website], 6)) scatter = plt.scatter(dataset['X'], dataset['Y'], c=dataset['Z'], cmap='viridis', s=10) plt.colorbar(scatter, label='Function Value') [website]'Scatter Plot of Randomly Sampled Data') [website]'X-axis') [website]'Y-axis') [website].
This is the data we’ll be training on to try to learn our function.
Recall that the back propagation algorithm updates parameters based on what happens in a forward pass. So, before we implement backpropagation itself, let’s keep track of a few critical values in the forward pass: The inputs and outputs of each perceptron throughout the model.
import numpy as np class SimpleNN: def __init__(self, architecture): self.architecture = architecture self.weights = [] [website] = [] #keeping track of these values in this code block #so we can observe them self.perceptron_inputs = None self.perceptron_outputs = None # Initialize weights and biases [website] for i in range(len(architecture) - 1): [website] low=-1, high=1, size=(architecture[i], architecture[i+1]) )) [website], architecture[i+1]))) @staticmethod def relu(x): return np.maximum(0, x) def forward(self, X): self.perceptron_inputs = [X] self.perceptron_outputs = [] for W, b in zip(self.weights, [website] Z = [website][-1], W) + b [website] if W is self.weights[-1]: # Last layer (output) A = Z # Linear output for regression else: A = [website] [website] return self.perceptron_inputs, self.perceptron_outputs def predict(self, X): perceptron_inputs, _ = self.forward(X) return perceptron_inputs[-1].flatten() #defining a model architecture = [2, 64, 64, 64, 1] # Two inputs, two hidden layers, one output model = SimpleNN(architecture) # Generate predictions prediction = model.predict([website][[website],[website]])) #looking through critical optimization values for i, (inpt, outpt) in enumerate(zip(model.perceptron_inputs, model.perceptron_outputs[:-1])): print(f'layer {i}') print(f'input: {[website]}') print(f'output: {[website]}') print('') print('Final Output:') print(model.perceptron_outputs[-1].shape).
The values throughout various layers of the model as a result of the forward pass. This will allow us to compute the necessary changes to upgrade the model.
Now that we have a record stored of critical intermediary value within the network, we can use those values, along with the error of a model for a particular prediction, to calculate the changes we should make to the model.
import numpy as np class SimpleNN: def __init__(self, architecture): self.architecture = architecture self.weights = [] [website] = [] # Initialize weights and biases [website] for i in range(len(architecture) - 1): [website] low=-1, high=1, size=(architecture[i], architecture[i+1]) )) [website], architecture[i+1]))) @staticmethod def relu(x): return np.maximum(0, x) @staticmethod def relu_as_weights(x): return (x > 0).astype(float) def forward(self, X): perceptron_inputs = [X] perceptron_outputs = [] for W, b in zip(self.weights, [website] Z = [website][-1], W) + b [website] if W is self.weights[-1]: # Last layer (output) A = Z # Linear output for regression else: A = [website] [website] return perceptron_inputs, perceptron_outputs def backward(self, perceptron_inputs, perceptron_outputs, target): weight_changes = [] bias_changes = [] m = len(target) dA = perceptron_inputs[-1] - target.reshape(-1, 1) # Output layer gradient for i in reversed(range(len(self.weights))): dZ = dA if i == len(self.weights) - 1 else dA * self.relu_as_weights(perceptron_outputs[i]) dW = [website][i].T, dZ) / m db = [website], axis=0, keepdims=True) / m [website] [website] if i > 0: dA = [website], self.weights[i].T) return list(reversed(weight_changes)), list(reversed(bias_changes)) def predict(self, X): perceptron_inputs, _ = self.forward(X) return perceptron_inputs[-1].flatten() #defining a model architecture = [2, 64, 64, 64, 1] # Two inputs, two hidden layers, one output model = SimpleNN(architecture) #defining a sample input and target output input = [website][[[website],[website]]]) desired_output = [website][[website]]) #doing forward and backward pass to calculate changes perceptron_inputs, perceptron_outputs = model.forward(input) weight_changes, bias_changes = model.backward(perceptron_inputs, perceptron_outputs, desired_output) #smaller numbers for printing np.set_printoptions(precision=2) for i, (layer_weights, layer_biases, layer_weight_changes, layer_bias_changes) in enumerate(zip(model.weights, [website], weight_changes, bias_changes)): print(f'layer {i}') print(f'weight matrix: {[website]}') print(f'weight matrix changes: {[website]}') print(f'bias matrix: {[website]}') print(f'bias matrix changes: {[website]}') print('') print('The weight and weight change matrix of the second layer:') print('weight matrix:') print(model.weights[1]) print('change matrix:') print(weight_changes[1]).
This is probably the most complex implementation step, so I want to take a moment to dig through some of the details. The fundamental idea is exactly as we described in previous sections. We’re iterating over all layers, from back to front, and calculating what change to each weight and bias would result in a improved output.
# calculating output error dA = perceptron_inputs[-1] - target.reshape(-1, 1) #a scaling factor for the batch size. #you want changes to be an average across all batches #so we divide by m once we've aggregated all changes. m = len(target) for i in reversed(range(len(self.weights))): dZ = dA #simplified for now # calculating change to weights dW = [website][i].T, dZ) / m # calculating change to bias db = [website], axis=0, keepdims=True) / m # keeping track of required changes [website] [website] ...
Calculating the change to bias is pretty straight forward. If you look at how the output of a given neuron should have impacted all future neurons, you can add up all those values (which are both positive and negative) to get an idea of if the neuron should be biased in a positive or negative direction.
The way we calculate the change to weights, by using matrix multiplication, is a bit more mathematically complex.
Basically, this line says that the change in the weight should be equal to the value going into the perceptron, times how much the output should have changed. If a perceptron had a big input, the change to its outgoing weights should be a large magnitude, if the perceptron had a small input, the change to its outgoing weights will be small. Also, if a weight points towards an output which should change a lot, the weight should change a lot.
There is another line we should discuss in our back propagation implement.
dZ = dA if i == len(self.weights) - 1 else dA * self.relu_as_weights(perceptron_outputs[i]).
In this particular network, there are activation functions throughout the network, following all but the final output. When we do back propagation, we need to back-propagate through these activation functions so that we can revision the neurons which lie before them. We do this for all but the last layer, which doesn’t have an activation function, which is why dZ = dA if i == len(self.weights) - 1 .
In fancy math speak we would call this a derivative, but because I don’t want to get into calculus, I called the function relu_as_weights . Basically, we can treat each of our ReLU activations as something like a tiny neural network, who’s weight is a function of the input. If the input of the ReLU activation function is less than zero, then that’s like passing that input through a neural network with a weight of zero. If the input of ReLU is greater than zero, then that’s like passing the input through a neural netowork with a weight of one.
This is exactly what the relu_as_weights function does.
def relu_as_weights(x): return (x > 0).astype(float).
Using this logic we can treat back propagating through ReLU just like we back propagate through the rest of the neural network.
Again, I’ll be covering this concept from a more robust mathematical prospective soon, but that’s the essential idea from a conceptual perspective.
Now that we have the forward and backward pass implemented, we can implement training the model.
import numpy as np class SimpleNN: def __init__(self, architecture): self.architecture = architecture self.weights = [] [website] = [] # Initialize weights and biases [website] for i in range(len(architecture) - 1): [website] low=-1, high=1, size=(architecture[i], architecture[i+1]) )) [website], architecture[i+1]))) @staticmethod def relu(x): return np.maximum(0, x) @staticmethod def relu_as_weights(x): return (x > 0).astype(float) def forward(self, X): perceptron_inputs = [X] perceptron_outputs = [] for W, b in zip(self.weights, [website] Z = [website][-1], W) + b [website] if W is self.weights[-1]: # Last layer (output) A = Z # Linear output for regression else: A = [website] [website] return perceptron_inputs, perceptron_outputs def backward(self, perceptron_inputs, perceptron_outputs, y_true): weight_changes = [] bias_changes = [] m = len(y_true) dA = perceptron_inputs[-1] - y_true.reshape(-1, 1) # Output layer gradient for i in reversed(range(len(self.weights))): dZ = dA if i == len(self.weights) - 1 else dA * self.relu_as_weights(perceptron_outputs[i]) dW = [website][i].T, dZ) / m db = [website], axis=0, keepdims=True) / m [website] [website] if i > 0: dA = [website], self.weights[i].T) return list(reversed(weight_changes)), list(reversed(bias_changes)) def update_weights(self, weight_changes, bias_changes, lr): for i in range(len(self.weights)): self.weights[i] -= lr * weight_changes[i] [website][i] -= lr * bias_changes[i] def train(self, X, y, epochs, [website] for epoch in range(epochs): perceptron_inputs, perceptron_outputs = self.forward(X) weight_changes, bias_changes = self.backward(perceptron_inputs, perceptron_outputs, y) self.update_weights(weight_changes, bias_changes, lr) if epoch % 20 == 0 or epoch == epochs - 1: loss = [website][-1].flatten() - y) ** 2) # MSE print(f"EPOCH {epoch}: Loss = {[website]}") def predict(self, X): perceptron_inputs, _ = self.forward(X) return perceptron_inputs[-1].flatten().
iterates through all the data some number of times (defined by epoch ).
) passes the data through a forward pass.
calculates how the weights and biases should change.
updates the weights and biases, by scaling their changes by the learning rate ( lr ).
And thus we’ve implemented a neural network! Let’s train it.
Training and Evaluating the Neural Network.
Recall that we defined an arbitrary 2D function we wanted to learn how to emulate,.
and we sampled that space with some number of points, which we’re using to train the model.
Before feeding this data into our model, it’s vital that we first "normalize" the data. Certain values of the dataset are very small or very large, which can make training a neural network very difficult. Values within the neural network can quickly grow to absurdly large values, or diminish to zero, which can inhibit training. Normalization squashes all of our inputs, and our desired outputs, into a more reasonable range averaging around zero with a standardized distribution called a "normal" distribution.
# Flatten the data X_flat = X.flatten() Y_flat = Y.flatten() Z_flat = Z.flatten() # Stack X and Y as input attributes inputs = np.column_stack((X_flat, Y_flat)) outputs = Z_flat # Normalize the inputs and outputs inputs_mean = [website], axis=0) inputs_std = [website], axis=0) outputs_mean = [website] outputs_std = [website] inputs = (inputs - inputs_mean) / inputs_std outputs = (outputs - outputs_mean) / outputs_std.
If we want to get back predictions in the actual range of data from our original dataset, we can use these values to essentially "un-squash" the data.
Once we’ve done that, we can define and train our model.
# Define the architecture: [input_dim, hidden1, ..., output_dim] architecture = [2, 64, 64, 64, 1] # Two inputs, two hidden layers, one output model = SimpleNN(architecture) # Train the model [website], outputs, epochs=2000, [website].
As can be seen, the value of loss is going down consistently, implying the model is improving.
Then we can visualize the output of the neural network’s prediction vs the actual function.
import [website] as plt # Reshape predictions to grid format for visualization Z_pred = model.predict(inputs) * outputs_std + outputs_mean Z_pred = Z_pred.reshape([website] # Plot comparison of the true function and the model predictions fig, axes = plt.subplots(1, 2, figsize=(14, 6)) # Plot the true function axes[0].contourf(X, Y, Z, cmap='viridis') axes[0].set_title("True Function") axes[0].set_xlabel("X-axis") axes[0].set_ylabel("Y-axis") axes[0].colorbar = plt.colorbar(axes[0].contourf(X, Y, Z, cmap='viridis'), ax=axes[0], label="Function Value") # Plot the predicted function axes[1].contourf(X, Y, Z_pred, cmap='plasma') axes[1].set_title("NN Predicted Function") axes[1].set_xlabel("X-axis") axes[1].set_ylabel("Y-axis") axes[1].colorbar = plt.colorbar(axes[1].contourf(X, Y, Z_pred, cmap='plasma'), ax=axes[1], label="Function Value") plt.tight_layout() [website].
This did an ok job, but not as great as we might like. This is where a lot of data scientists spend their time, and there are a ton of approaches to making a neural network fit a certain problem enhanced. Some obvious ones are:
It’s pretty easy for us to crank up the amount of data we’re training on. Let’s see where that leads us. Here I’m sampling our dataset 10,000 times, which is 10x more training samples than our previous dataset.
And then I trained the model just like before, except this time it took a lot longer because each epoch now analyses 10,000 samples rather than 1,000.
# Define the architecture: [input_dim, hidden1, ..., output_dim] architecture = [2, 64, 64, 64, 1] # Two inputs, two hidden layers, one output model = SimpleNN(architecture) # Train the model [website], outputs, epochs=2000, [website].
I then rendered the output of this model, the same way I did before, but it didn’t really look like the output got much superior.
Looking back at the loss output from training, it seems like the loss is still steadily declining. Maybe I just need to train for longer. Let’s try that.
# Define the architecture: [input_dim, hidden1, ..., output_dim] architecture = [2, 64, 64, 64, 1] # Two inputs, two hidden layers, one output model = SimpleNN(architecture) # Train the model [website], outputs, epochs=4000, [website].
The results seem to be a bit more effective, but they aren’t’ amazing.
I’ll spare you the details. I ran this a few times, and I got some decent results, but never anything 1 to 1. I’ll be covering some more advanced approaches data scientists use, like annealing and dropout, in future articles which will result in a more consistent and more effective output. Still, though, we made a neural network from scratch and trained it to do something, and it did a decent job! Pretty neat!
In this article we avoided calculus like the plague while simultaneously forging an understanding of Neural Networks. We explored their theory, a little bit about the math, the idea of back propagation, and then implemented a neural network from scratch. We then applied a neural network to a toy problem, and explored some of the simple ideas data scientists employ to actually train neural networks to be good at things.
In future articles we’ll explore a few more advanced approaches to Neural Networks, so stay tuned! For now, you might be interested in a more thorough analysis of Gradients, the fundamental math behind back propagation.
You might also be interested in this article, which covers training a neural network using more conventional Data Science tools like PyTorch.
Join Intuitively and Exhaustively Explained.
Over the past year, we’ve made incredible progress in enhancing the quality of our generative media technologies. We’ve been working closely with the ...
firm A new generation of African talent brings cutting-edge AI to scientific challenges Share.
Today, we’re releasing two updated production-ready Gemini models: [website] and [website] along with: >50% reduced price on [website] P...
Market Impact Analysis
Market Growth Trend
2018 | 2019 | 2020 | 2021 | 2022 | 2023 | 2024 |
---|---|---|---|---|---|---|
23.1% | 27.8% | 29.2% | 32.4% | 34.2% | 35.2% | 35.6% |
Quarterly Growth Rate
Q1 2024 | Q2 2024 | Q3 2024 | Q4 2024 |
---|---|---|---|
32.5% | 34.8% | 36.2% | 35.6% |
Market Segments and Growth Drivers
Segment | Market Share | Growth Rate |
---|---|---|
Machine Learning | 29% | 38.4% |
Computer Vision | 18% | 35.7% |
Natural Language Processing | 24% | 41.5% |
Robotics | 15% | 22.3% |
Other AI Technologies | 14% | 31.8% |
Technology Maturity Curve
Different technologies within the ecosystem are at varying stages of maturity:
Competitive Landscape Analysis
Company | Market Share |
---|---|
Google AI | 18.3% |
Microsoft AI | 15.7% |
IBM Watson | 11.2% |
Amazon AI | 9.8% |
OpenAI | 8.4% |
Future Outlook and Predictions
The Deep Dive Into landscape is evolving rapidly, driven by technological advancements, changing threat vectors, and shifting business requirements. Based on current trends and expert analyses, we can anticipate several significant developments across different time horizons:
Year-by-Year Technology Evolution
Based on current trajectory and expert analyses, we can project the following development timeline:
Technology Maturity Curve
Different technologies within the ecosystem are at varying stages of maturity, influencing adoption timelines and investment priorities:
Innovation Trigger
- Generative AI for specialized domains
- Blockchain for supply chain verification
Peak of Inflated Expectations
- Digital twins for business processes
- Quantum-resistant cryptography
Trough of Disillusionment
- Consumer AR/VR applications
- General-purpose blockchain
Slope of Enlightenment
- AI-driven analytics
- Edge computing
Plateau of Productivity
- Cloud infrastructure
- Mobile applications
Technology Evolution Timeline
- Improved generative models
- specialized AI applications
- AI-human collaboration systems
- multimodal AI platforms
- General AI capabilities
- AI-driven scientific breakthroughs
Expert Perspectives
Leading experts in the ai tech sector provide diverse perspectives on how the landscape will evolve over the coming years:
"The next frontier is AI systems that can reason across modalities and domains with minimal human guidance."
— AI Researcher
"Organizations that develop effective AI governance frameworks will gain competitive advantage."
— Industry Analyst
"The AI talent gap remains a critical barrier to implementation for most enterprises."
— Chief AI Officer
Areas of Expert Consensus
- Acceleration of Innovation: The pace of technological evolution will continue to increase
- Practical Integration: Focus will shift from proof-of-concept to operational deployment
- Human-Technology Partnership: Most effective implementations will optimize human-machine collaboration
- Regulatory Influence: Regulatory frameworks will increasingly shape technology development
Short-Term Outlook (1-2 Years)
In the immediate future, organizations will focus on implementing and optimizing currently available technologies to address pressing ai tech challenges:
- Improved generative models
- specialized AI applications
- enhanced AI ethics frameworks
These developments will be characterized by incremental improvements to existing frameworks rather than revolutionary changes, with emphasis on practical deployment and measurable outcomes.
Mid-Term Outlook (3-5 Years)
As technologies mature and organizations adapt, more substantial transformations will emerge in how security is approached and implemented:
- AI-human collaboration systems
- multimodal AI platforms
- democratized AI development
This period will see significant changes in security architecture and operational models, with increasing automation and integration between previously siloed security functions. Organizations will shift from reactive to proactive security postures.
Long-Term Outlook (5+ Years)
Looking further ahead, more fundamental shifts will reshape how cybersecurity is conceptualized and implemented across digital ecosystems:
- General AI capabilities
- AI-driven scientific breakthroughs
- new computing paradigms
These long-term developments will likely require significant technical breakthroughs, new regulatory frameworks, and evolution in how organizations approach security as a fundamental business function rather than a technical discipline.
Key Risk Factors and Uncertainties
Several critical factors could significantly impact the trajectory of ai tech evolution:
Organizations should monitor these factors closely and develop contingency strategies to mitigate potential negative impacts on technology implementation timelines.
Alternative Future Scenarios
The evolution of technology can follow different paths depending on various factors including regulatory developments, investment trends, technological breakthroughs, and market adoption. We analyze three potential scenarios:
Optimistic Scenario
Responsible AI driving innovation while minimizing societal disruption
Key Drivers: Supportive regulatory environment, significant research breakthroughs, strong market incentives, and rapid user adoption.
Probability: 25-30%
Base Case Scenario
Incremental adoption with mixed societal impacts and ongoing ethical challenges
Key Drivers: Balanced regulatory approach, steady technological progress, and selective implementation based on clear ROI.
Probability: 50-60%
Conservative Scenario
Technical and ethical barriers creating significant implementation challenges
Key Drivers: Restrictive regulations, technical limitations, implementation challenges, and risk-averse organizational cultures.
Probability: 15-20%
Scenario Comparison Matrix
Factor | Optimistic | Base Case | Conservative |
---|---|---|---|
Implementation Timeline | Accelerated | Steady | Delayed |
Market Adoption | Widespread | Selective | Limited |
Technology Evolution | Rapid | Progressive | Incremental |
Regulatory Environment | Supportive | Balanced | Restrictive |
Business Impact | Transformative | Significant | Modest |
Transformational Impact
Redefinition of knowledge work, automation of creative processes. This evolution will necessitate significant changes in organizational structures, talent development, and strategic planning processes.
The convergence of multiple technological trends—including artificial intelligence, quantum computing, and ubiquitous connectivity—will create both unprecedented security challenges and innovative defensive capabilities.
Implementation Challenges
Ethical concerns, computing resource limitations, talent shortages. Organizations will need to develop comprehensive change management strategies to successfully navigate these transitions.
Regulatory uncertainty, particularly around emerging technologies like AI in security applications, will require flexible security architectures that can adapt to evolving compliance requirements.
Key Innovations to Watch
Multimodal learning, resource-efficient AI, transparent decision systems. Organizations should monitor these developments closely to maintain competitive advantages and effective security postures.
Strategic investments in research partnerships, technology pilots, and talent development will position forward-thinking organizations to leverage these innovations early in their development cycle.
Technical Glossary
Key technical terms and definitions to help understand the technologies discussed in this article.
Understanding the following technical concepts is essential for grasping the full implications of the security threats and defensive measures discussed in this article. These definitions provide context for both technical and non-technical readers.