In conversation with AI: building better language models - Related to scientist, a, data, breakthroughs, our
How to Get Promoted as a Data Scientist
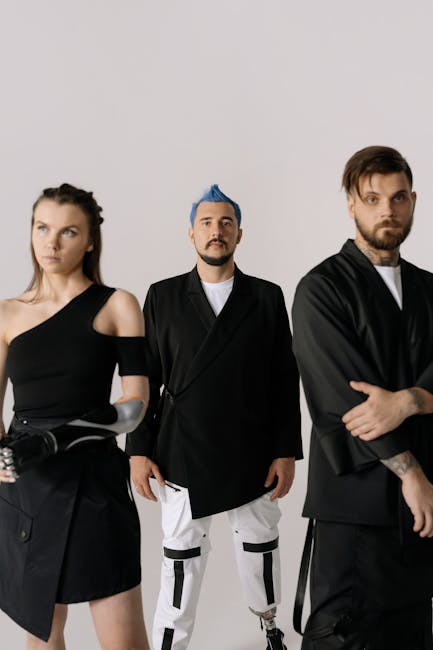
Image artificially generated using Grok 2.
I have been working as a Data Scientist since 2017, and during that time I have been promoted from a junior/mid-level to a senior, and most lately to a Lead Data Scientist.
There is a lot of content online regarding landing your first Data Scientist position, but what I find is lacking is the amount of knowledge being shared regarding how to progress your Data Scientist career after you have already landed your first position.
In this article, I will share my experiences over the past 8 years and offer advice to those looking to take the leap and get promoted in 2025.
I have always been a believer in earning your promotion through hard work rather than receiving it under alternative circumstances. It might seem like an obvious suggestion but simply demonstrating what you’re capable of and that you’re ready to step up and take on more responsibility is key to landing a promotion.
Take advantage of opportunities to showcase your work and vocalise what you have achieved. Many companies host internal show-and-tell sessions where individuals are given the opportunity to present their work to the wider organisation, take advantage of these opportunities! During these interactions, don’t just focus on the technical elements of your work, provide insights into how your work has directly contributed to business goals ([website] increasing ROI, reducing costs, or improving efficiency).
With that mentioned, it is not only about showcasing your work once complete. You also need to show that you are ready to take the lead on projects and that you understand how project success directly impacts business goals. Start to take more ownership of your work, when speaking to stakeholders, demonstrate that you are willing to take on more responsibility for your tasks and their outcomes.
Always remember, actions speak louder than words!
Most of the companies I have worked for have their employees fill out annual or quarterly personal development plans (PDPs) as a way to set goals and track progress over time. If your corporation does not do this, I highly recommend that you set these goals yourself as a benchmark of what you want to achieve in the short/mid-term. Having a PDP in place provides you with a formal future checkpoint which you can discuss with your line manager. Individuals who are overachieving in their PDP goals are much more likely to gain promotion or receive a salary increase.
I also recommend keeping an updated personal list of all of your main achievements broken down by quarter, therefore you have solid evidence to back up your asserts that you are ready to step up and be promoted. Always ask for feedback from senior colleagues when completing projects and document this, a strong candidate for promotion should be able to demonstrate their ability to implement feedback and improve their performance over time.
Don’t just focus on technical achievements; also focus on non-technical achievements, such as leading a workshop, mentoring a junior team member, or temporarily taking on more responsibility due to an annual leave or absence.
In my experience, those who approach the conversation of promotion with factual documented achievements have a much enhanced chance of being promoted, and preparation is key.
Throughout my 8 years as a Data Scientist, I have witnessed individuals less qualified get promoted over others simply because they were more visible and had established a presence within the business.
There is the perception that Data Scientists are promoted primarily based on their technical experience, but this is not the case. Having a broader presence within your corporation is a great asset when looking to land a promotion. I believe the higher the promotion, the more visible you should be to both technical and non-technical colleagues.
To become more visible in your firm, you should:
Speak up during group discussions, vocalise your ideas, and do not be afraid to challenge other people’s opinions, regardless of job titles. Spend time getting to know others within the business, and engage with product managers, stakeholders, and broader technical teams [website] software developers. Present your work at technical talks or write about your achievements via corporation blogs. Spend time at lunch and after work with colleagues, and get to know them personally outside of a work setting.
These actions can be challenging, especially for more introverted people. To simplify, just start communicating with others outside of your immediate team.
Every organization is different. What you first need to do is understand how your organization handles promotions. Some companies will batch promotions together, others will prefer to recruit externally, and others will promote ad-hoc throughout the year.
You will find in larger companies there are more hoops to jump through, more people are involved, and there is a higher level of sign-off before a promotion is granted. Smaller companies and start-ups are often able to promote at a much faster pace.
My advice would be to look at existing employees who have been promoted during the time you have worked at the organization. Analyse their path and understand whether or not this is something you are able to replicate. It wouldn’t be a bad idea to approach them and ask them about their own journey. Once you understand this process, it gives you more information to roadmap your own progression.
Unfortunately, some things can arise that are out of your control, such as restructures, funding, and people’s opinions that you are not quite at the level they expect for the position you are hoping to be promoted to.
To be successful as a Data Scientist you must have a passion for continuous learning. New research is being , but we need to try!
An often disregarded learning resource is actually the people around you who you work with day-to-day. Keep an interest in their projects, ask them what they are working on, and learn from them.
The best place to get advice on getting promoted is to ask those who have not long ago experienced this themselves (that is why you are reading this, right?).
Sometimes you believe you are ready for a promotion when in fact you are not. In these circumstances, it is great to have a mentor or senior colleague who can provide you with advice and recommend areas for you to develop further before pursuing a conversation regarding promotion.
For instances where you are ready, you want to set yourself up with the best pitch possible to deliver to your line manager. Although I am providing you with my personal advice in this article, you must understand that all companies are different. I strongly advise you to reach out to your senior colleagues and ask them about what makes a good senior in your firm. Take their advice, build your case, work on developing any areas they believe are essential, and arrange the conversation to pitch yourself as a strong candidate for the newly available senior position at your firm.
Your tenure at a firm alone does not warrant you to be next in line for promotion, neither does your technical ability alone or your relationship with your stakeholders.
From my experience, those who demonstrate what they are capable of, keep a log of all their achievements, enhance their visibility, understand the business structure, continue to expand their knowledge, and take advice from others have a much higher likelihood of getting promoted.
Don’t get me wrong, there are instances where promotions are not handed to the best-suited candidate, but by following the six points in this article, you will be maximising your chances of being successfully promoted in 2025.
Disclaimer: Unless stated otherwise, all images included in this article are owned by the author.
If you enjoyed reading this article, please follow me on Medium, X, and GitHub for similar content relating to Data Science, Artificial Intelligence, and Engineering.
Impact Stopping malaria in its tracks Share.
Developing a more effective malaria vaccine with the help of AI that could save hundreds of th...
AlphaFold is helping researchers uncover how protein-mutations cause disease, and how to prevent them.
Luigi Vitagliano is a Research Director at the ...
business 2023: A Year of Groundbreaking Advances in AI and Computing Share.
This has been a year of incredible progress in the field...
Maximising the impact of our breakthroughs
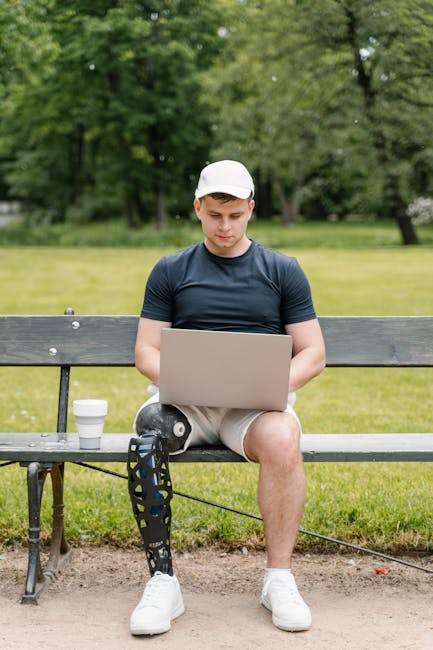
Applying our AI research to help enrich the lives of billions of people around the world.
Building useful products with new technologies has always been one of my greatest joys. As a boy, I’d spend hours connecting resistors, capacitors, and other electronic components with wires. First I’d assemble a Morse code circuit. Then dissemble the circuit, reusing its wires and components to build a timer. And then take the timer apart and build an amplifier. Analog electronic components provided the perfect toolkit for young minds, like mine, to build and create.
Soon I was designing processing chips at my first summer job at Marconi, the telecoms giant. And this led to studying electronics and software at university, where, on lab days, we’d build circuit boards for radio receivers. Now, we have pocket-sized computers and colossal data centres – all connected by light-speed communications – running powerful software for the benefit of businesses, communities, and consumers across the globe.
But it’s artificial intelligence (AI) that holds the greatest potential for humanity. This technology learns and iterates, with an ability to solve problems at every turn. When combined with human ingenuity and direction, AI could discover new solutions to humanity’s greatest challenges, at a speed and a scale that was previously unimaginable.
AI isn’t hype – it’s perhaps the ultimate general-purpose tool. As chief business officer (CBO) of one of the world’s leading AI companies, I see and feel, every day, how this technology can enrich the lives of billions of people around the world.
Finding the best ways to use AI for building helpful products is a key focus of my work and life today. It’s frequently a topic of conversation at my external engagements with business leaders, product people, and engineers – like my upcoming luminary keynote at this week’s AI Hardware Summit.
My main focus as CBO is on taking our cutting-edge research breakthroughs and matching our technologies to solving everyday business problems. This intersection is phenomenally exciting since in many cases, we’re working in uncharted territory, introducing tools that hold the promise of solving problems for billions of people around the world.
I’m often asked, as a future-facing research organisation, why it’s crucial to work on global challenges that impact people every day? One of the things (but not the only thing!) that makes DeepMind so special is our ability to bridge leading AI research to hundreds, if not thousands, of AI-ready problems that impact billions of people.
We’ve been creating one of the largest libraries of AI solutions in the world, and our parent business, Alphabet, has an amazing marketplace of problems to solve. Together, we’re able to focus on the hardest technical challenges with the biggest pay-offs, creating useful products that help billions of people in the moments that matter.
For instance, we’ve helped extend the battery life of phones for Android’s operating systems, used by over a billion people every day. This can be a lifesaver, especially in times of need. It’s been one of the most universally requested problems to solve, and will become increasingly key as we move to a cleaner, greener world.
One of the greatest challenges and opportunities of working in this space is finding ways to leverage AI’s potential while ensuring that our work is safe, ethical, and inclusive at every stage, from research and development through to application and impact.
In recent years, we’ve gone from talking about the potential of our work to actually benefiting billions of people on a daily basis. And now we’re at the stage where we’re applying AI to Nobel Prize-level problems in science and society.
But with so much potential, where does one start? To make sure our work is being applied in the most effective ways possible, we start by searching for core, transformational challenges that, if solved, could help tackle many other efficiencies across a wide array of problems.
One of our most prominent examples of this is AlphaFold, our AI system that can accurately predict protein structures, the building blocks of life. With this system, we helped solve this 50-year-old grand challenge in biology, which is now helping scientists around the world advance their work.
We’ve also been working in areas like sustainability, particularly looking at the ways AI can help optimise the production and consumption of energy. For instance, we’ve created an AI system that can control plasma in the nuclear fusion process, which could lead to safer, cleaner energy production. We’ve also helped reduce energy consumption in Google’s enormous data centres, improving energy efficiency and reducing emissions. These advances are game changers for how energy can be managed and used in society.
Similarly, MuZero has shown so much potential for saving time and energy at scale. It was initially built to progress gaming intelligence and is now helping enhance the YouTube experience. Nearly seven hundred thousand hours of content are viewed on YouTube every minute. This is an astonishing amount of web traffic. By optimising the ways videos are compressed, for example, we’ve reduced data and energy use and helped increase access to video content across the world.
AI is undoubtedly the most transformative technology of our time. This exceptional promise also requires exceptional care. Anticipating potential impacts of a technology as general and transformative as AI is really difficult, and so careful consideration is essential.
For this reason, we’ve devised a long-term scientific roadmap to help guide our research. And as we go along this journey, we’re continuously assessing the long-term impact of our work to make sure it’s being deployed in a safe and responsible way.
We need to have the smartest minds working on things carefully, step by step. This is too big, too critical to move fast and break things, which is why pioneering responsibly is at the heart of everything that we do.
It’s incredibly inspiring to be able to really connect with the vast range of problems that our work helps to solve, and the potential to have enormous benefits for humanity, with our access to billions of people around the world. For me, that combination is truly extraordinary.
Introducing a context-based framework for comprehensively evaluating the social and ethical risks of AI systems.
Generative AI systems are already bei...
During the telecommunication boom, Claude Shannon, in his seminal 1948 paper¹, posed a question that would revoluti...
Some time ago, I mentioned in an article how I believed AI could replace a traditional search engine, and the reasons behind that were focused ...
In conversation with AI: building better language models
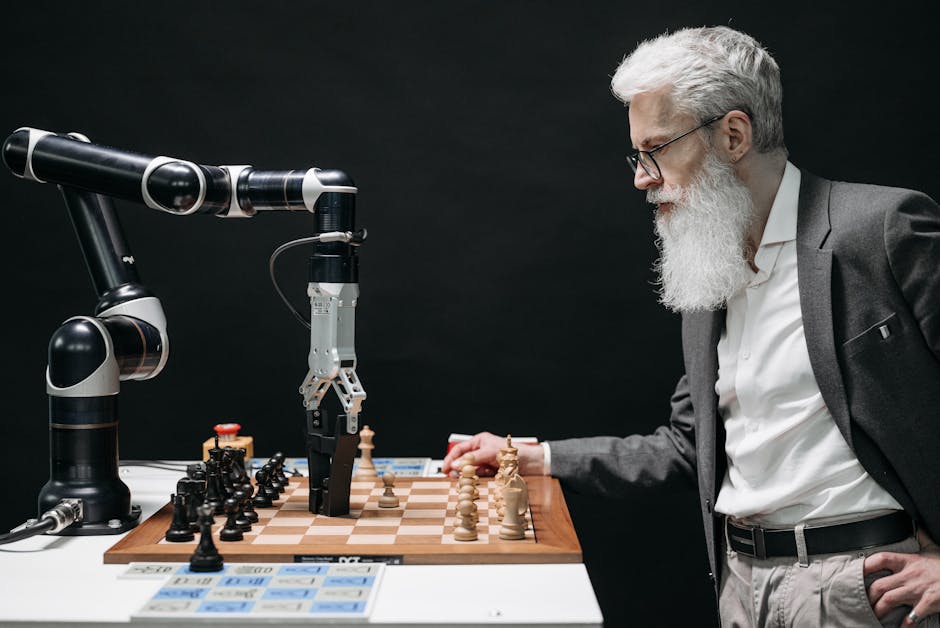
New research drawing upon pragmatics and philosophy proposes ways to align conversational agents with human values.
Language is an essential human trait and the primary means by which we communicate information including thoughts, intentions, and feelings. Recent breakthroughs in AI research have led to the creation of conversational agents that are able to communicate with humans in nuanced ways. These agents are powered by large language models – computational systems trained on vast corpora of text-based materials to predict and produce text using advanced statistical techniques.
Yet, while language models such as InstructGPT, Gopher, and LaMDA have achieved record levels of performance across tasks such as translation, question-answering, and reading comprehension, these models have also been shown to exhibit a number of potential risks and failure modes. These include the production of toxic or discriminatory language and false or misleading information [1, 2, 3].
These shortcomings limit the productive use of conversational agents in applied settings and draw attention to the way in which they fall short of certain communicative ideals. To date, most approaches on the alignment of conversational agents have focused on anticipating and reducing the risks of harms [4].
Our new paper, In conversation with AI: aligning language models with human values, adopts a different approach, exploring what successful communication between a human and an artificial conversational agent might look like, and what values should guide these interactions across different conversational domains.
To address these issues, the paper draws upon pragmatics, a tradition in linguistics and philosophy, which holds that the purpose of a conversation, its context, and a set of related norms, all form an essential part of sound conversational practice.
Modelling conversation as a cooperative endeavour between two or more parties, the linguist and philosopher, Paul Grice, held that participants ought to:
However, our paper demonstrates that further refinement of these maxims is needed before they can be used to evaluate conversational agents, given variation in the goals and values embedded across different conversational domains.
By way of illustration, scientific investigation and communication is geared primarily toward understanding or predicting empirical phenomena. Given these goals, a conversational agent designed to assist scientific investigation would ideally only make statements whose veracity is confirmed by sufficient empirical evidence, or otherwise qualify its positions .
For example, an agent reporting that, “At a distance of [website] light years, Proxima Centauri is the closest star to earth,” should do so only after the model underlying it has checked that the statement corresponds with the facts.
Yet, a conversational agent playing the role of a moderator in public political discourse may need to demonstrate quite different virtues. In this context, the goal is primarily to manage differences and enable productive cooperation in the life of a community. Therefore, the agent will need to foreground the democratic values of toleration, civility, and respect [5].
Moreover, these values explain why the generation of toxic or prejudicial speech by language models is often so problematic: the offending language fails to communicate equal respect for participants to the conversation, something that is a key value for the context in which the models are deployed. At the same time, scientific virtues, such as the comprehensive presentation of empirical data, may be less key in the context of public deliberation.
Finally, in the domain of creative storytelling, communicative exchange aims at novelty and originality, values that again differ significantly from those outlined above. In this context, greater latitude with make-believe may be appropriate, although it remains essential to safeguard communities against malicious content produced under the guise of ‘creative uses’.
This research has a number of practical implications for the development of aligned conversational AI agents. To begin with, they will need to embody different traits depending on the contexts in which they are deployed: there is no one-size-fits-all account of language-model alignment. Instead, the appropriate mode and evaluative standards for an agent – including standards of truthfulness – will vary .
Additionally, conversational agents may also have the potential to cultivate more robust and respectful conversations over time, via a process that we refer to as context construction and elucidation. Even when a person is not aware of the values that govern a given conversational practice, the agent may still help the human understand these values by prefiguring them in conversation, making the course of communication deeper and more fruitful for the human speaker.
Life is like a box of chocolate. Generated using DALL-E.
My momma always mentioned "Life was like a box of chocolates. You never know what you’re gonna get...
Impact The race to cure a billion people from a deadly parasitic disease Share.
Researchers accelerate their search of life-saving ...
One of the biggest drawbacks to using Gemini as your default Android assistant is going away.
Market Impact Analysis
Market Growth Trend
2018 | 2019 | 2020 | 2021 | 2022 | 2023 | 2024 |
---|---|---|---|---|---|---|
23.1% | 27.8% | 29.2% | 32.4% | 34.2% | 35.2% | 35.6% |
Quarterly Growth Rate
Q1 2024 | Q2 2024 | Q3 2024 | Q4 2024 |
---|---|---|---|
32.5% | 34.8% | 36.2% | 35.6% |
Market Segments and Growth Drivers
Segment | Market Share | Growth Rate |
---|---|---|
Machine Learning | 29% | 38.4% |
Computer Vision | 18% | 35.7% |
Natural Language Processing | 24% | 41.5% |
Robotics | 15% | 22.3% |
Other AI Technologies | 14% | 31.8% |
Technology Maturity Curve
Different technologies within the ecosystem are at varying stages of maturity:
Competitive Landscape Analysis
Company | Market Share |
---|---|
Google AI | 18.3% |
Microsoft AI | 15.7% |
IBM Watson | 11.2% |
Amazon AI | 9.8% |
OpenAI | 8.4% |
Future Outlook and Predictions
The Promoted Data Scientist landscape is evolving rapidly, driven by technological advancements, changing threat vectors, and shifting business requirements. Based on current trends and expert analyses, we can anticipate several significant developments across different time horizons:
Year-by-Year Technology Evolution
Based on current trajectory and expert analyses, we can project the following development timeline:
Technology Maturity Curve
Different technologies within the ecosystem are at varying stages of maturity, influencing adoption timelines and investment priorities:
Innovation Trigger
- Generative AI for specialized domains
- Blockchain for supply chain verification
Peak of Inflated Expectations
- Digital twins for business processes
- Quantum-resistant cryptography
Trough of Disillusionment
- Consumer AR/VR applications
- General-purpose blockchain
Slope of Enlightenment
- AI-driven analytics
- Edge computing
Plateau of Productivity
- Cloud infrastructure
- Mobile applications
Technology Evolution Timeline
- Improved generative models
- specialized AI applications
- AI-human collaboration systems
- multimodal AI platforms
- General AI capabilities
- AI-driven scientific breakthroughs
Expert Perspectives
Leading experts in the ai tech sector provide diverse perspectives on how the landscape will evolve over the coming years:
"The next frontier is AI systems that can reason across modalities and domains with minimal human guidance."
— AI Researcher
"Organizations that develop effective AI governance frameworks will gain competitive advantage."
— Industry Analyst
"The AI talent gap remains a critical barrier to implementation for most enterprises."
— Chief AI Officer
Areas of Expert Consensus
- Acceleration of Innovation: The pace of technological evolution will continue to increase
- Practical Integration: Focus will shift from proof-of-concept to operational deployment
- Human-Technology Partnership: Most effective implementations will optimize human-machine collaboration
- Regulatory Influence: Regulatory frameworks will increasingly shape technology development
Short-Term Outlook (1-2 Years)
In the immediate future, organizations will focus on implementing and optimizing currently available technologies to address pressing ai tech challenges:
- Improved generative models
- specialized AI applications
- enhanced AI ethics frameworks
These developments will be characterized by incremental improvements to existing frameworks rather than revolutionary changes, with emphasis on practical deployment and measurable outcomes.
Mid-Term Outlook (3-5 Years)
As technologies mature and organizations adapt, more substantial transformations will emerge in how security is approached and implemented:
- AI-human collaboration systems
- multimodal AI platforms
- democratized AI development
This period will see significant changes in security architecture and operational models, with increasing automation and integration between previously siloed security functions. Organizations will shift from reactive to proactive security postures.
Long-Term Outlook (5+ Years)
Looking further ahead, more fundamental shifts will reshape how cybersecurity is conceptualized and implemented across digital ecosystems:
- General AI capabilities
- AI-driven scientific breakthroughs
- new computing paradigms
These long-term developments will likely require significant technical breakthroughs, new regulatory frameworks, and evolution in how organizations approach security as a fundamental business function rather than a technical discipline.
Key Risk Factors and Uncertainties
Several critical factors could significantly impact the trajectory of ai tech evolution:
Organizations should monitor these factors closely and develop contingency strategies to mitigate potential negative impacts on technology implementation timelines.
Alternative Future Scenarios
The evolution of technology can follow different paths depending on various factors including regulatory developments, investment trends, technological breakthroughs, and market adoption. We analyze three potential scenarios:
Optimistic Scenario
Responsible AI driving innovation while minimizing societal disruption
Key Drivers: Supportive regulatory environment, significant research breakthroughs, strong market incentives, and rapid user adoption.
Probability: 25-30%
Base Case Scenario
Incremental adoption with mixed societal impacts and ongoing ethical challenges
Key Drivers: Balanced regulatory approach, steady technological progress, and selective implementation based on clear ROI.
Probability: 50-60%
Conservative Scenario
Technical and ethical barriers creating significant implementation challenges
Key Drivers: Restrictive regulations, technical limitations, implementation challenges, and risk-averse organizational cultures.
Probability: 15-20%
Scenario Comparison Matrix
Factor | Optimistic | Base Case | Conservative |
---|---|---|---|
Implementation Timeline | Accelerated | Steady | Delayed |
Market Adoption | Widespread | Selective | Limited |
Technology Evolution | Rapid | Progressive | Incremental |
Regulatory Environment | Supportive | Balanced | Restrictive |
Business Impact | Transformative | Significant | Modest |
Transformational Impact
Redefinition of knowledge work, automation of creative processes. This evolution will necessitate significant changes in organizational structures, talent development, and strategic planning processes.
The convergence of multiple technological trends—including artificial intelligence, quantum computing, and ubiquitous connectivity—will create both unprecedented security challenges and innovative defensive capabilities.
Implementation Challenges
Ethical concerns, computing resource limitations, talent shortages. Organizations will need to develop comprehensive change management strategies to successfully navigate these transitions.
Regulatory uncertainty, particularly around emerging technologies like AI in security applications, will require flexible security architectures that can adapt to evolving compliance requirements.
Key Innovations to Watch
Multimodal learning, resource-efficient AI, transparent decision systems. Organizations should monitor these developments closely to maintain competitive advantages and effective security postures.
Strategic investments in research partnerships, technology pilots, and talent development will position forward-thinking organizations to leverage these innovations early in their development cycle.
Technical Glossary
Key technical terms and definitions to help understand the technologies discussed in this article.
Understanding the following technical concepts is essential for grasping the full implications of the security threats and defensive measures discussed in this article. These definitions provide context for both technical and non-technical readers.