Empowering YouTube creators with generative AI - Related to healthcare, youtube, tools, reliable, our
Developing reliable AI tools for healthcare
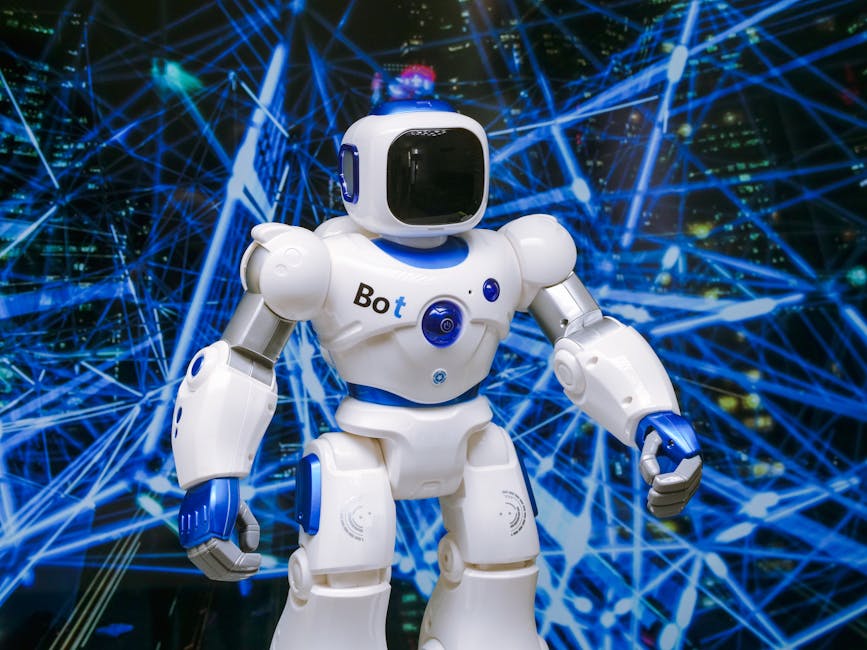
New research proposes a system to determine the relative accuracy of predictive AI in a hypothetical medical setting, and when the system should defer to a human clinician.
Artificial intelligence (AI) has great potential to enhance how people work across a range of industries. But to integrate AI tools into the workplace in a safe and responsible way, we need to develop more robust methods for understanding when they can be most useful.
So when is AI more accurate, and when is a human? This question is particularly essential in healthcare, where predictive AI is increasingly used in high-stakes tasks to assist clinicians.
Today in Nature Medicine, we’ve , which proposes CoDoC (Complementarity-driven Deferral-to-Clinical Workflow), an AI system that learns when to rely on predictive AI tools or defer to a clinician for the most accurate interpretation of medical images.
CoDoC explores how we could harness human-AI collaboration in hypothetical medical settings to deliver the best results. In one example scenario, CoDoC reduced the number of false positives by 25% for a large, de-identified UK mammography dataset, compared with commonly used clinical workflows – without missing any true positives.
This work is a collaboration with several healthcare organisations, including the United Nations Office for Project Services’ Stop TB Partnership. To help researchers build on our work to improve the transparency and safety of AI models for the real world, we’ve also open-sourced CoDoC’s code on GitHub.
CoDoC: Add-on tool for human-AI collaboration.
Building more reliable AI models often requires re-engineering the complex inner workings of predictive AI models. However, for many healthcare providers, it’s simply not possible to redesign a predictive AI model. CoDoC can potentially help improve predictive AI tools for its consumers without requiring them to modify the underlying AI tool itself.
When developing CoDoC, we had three criteria:
Non-machine learning experts, like healthcare providers, should be able to deploy the system and run it on a single computer.
Training would require a relatively small amount of data – typically, just a few hundred examples.
The system could be compatible with any proprietary AI models and would not need access to the model’s inner workings or data it was trained on.
Determining when predictive AI or a clinician is more accurate.
With CoDoC, we propose a simple and usable AI system to improve reliability by helping predictive AI systems to ‘know when they don’t know’. We looked at scenarios, where a clinician might have access to an AI tool designed to help interpret an image, for example, examining a chest x-ray for whether a tuberculosis test is needed.
For any theoretical clinical setting, CoDoC’s system requires only three inputs for each case in the training dataset.
The predictive AI outputs a confidence score between 0 (certain no disease is present) and 1 (certain that disease is present). The clinician’s interpretation of the medical image. The ground truth of whether disease was present, as, for example, established via biopsy or other clinical follow-up.
Note: CoDoC requires no access to any medical images.
Research RT-2: New model translates vision and language into action Share.
Robotic Transformer 2 (RT-2) is a novel vision-language-...
Real-time communication is everywhere – live chatbots, data streams, or instant messaging. WebSockets are a powerful ena...
Research Mastering Stratego, the classic game of imperfect information Share.
DeepNash learns to play Stratego from scratch by comb...
Empowering YouTube creators with generative AI
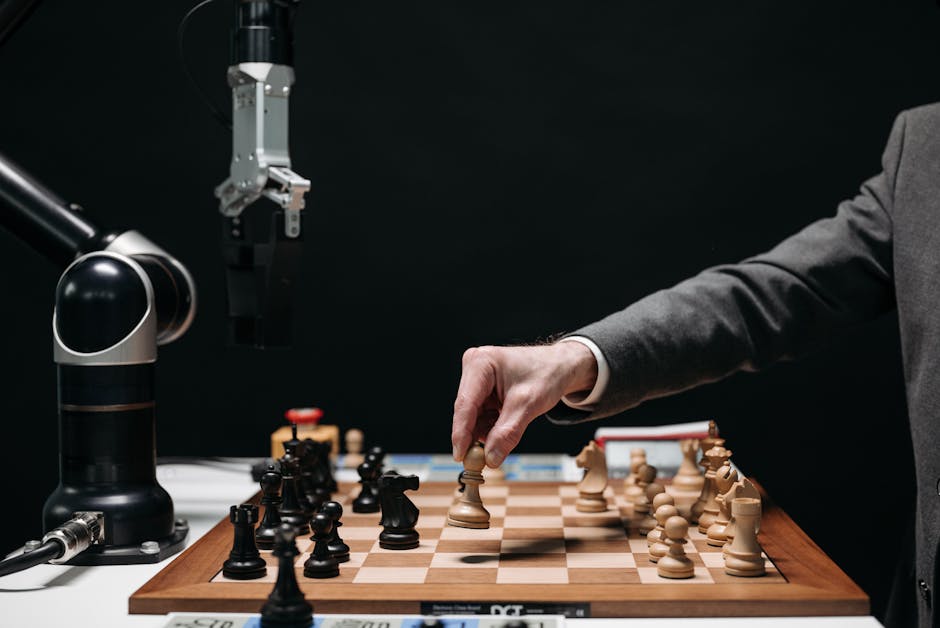
Throughout this journey, we’ve worked closely with artists and creators and have been guided by their curiosity and feedback to ensure our technologies help more people realize their creative vision.
From our groundbreaking Transformer architecture to years of research in diffusion models, these models are built on nearly a decade of innovation at Google and are now optimized for wide-scale use.
We’re changing that, and making these incredible technologies more easily accessible to millions of creators and billions of customers around the world. Over the next few months, we’re bringing our advanced generative AI models, Veo and Imagen 3 , to YouTube creators through Dream Screen .
Artificial intelligence (AI) technologies for generating creative content are improving rapidly, but seamless ways of using them still aren’t widely available.
New video generation technology in YouTube Shorts will help millions of people realize their creative vision.
In 2025, creators will also be able to use Dream Screen for generating 6 second standalone video clips for their Shorts, like a cinematic underwater reveal of the Golden Gate Bridge.
Starting with an initial text prompt, Dream Screen uses Imagen 3 to generate four different images. Creators can pick an image in their preferred style, composition or aesthetic from these options. With the selected image, Veo generates a high-quality 6 second background video, tailored to the user’s creative vision.
By integrating Veo into Dream Screen, YouTube creators will soon be able to generate exciting backgrounds for their Shorts. Early next year, we’re also making it possible to generate 6 second standalone video clips.
We hope these new video and music generation technologies will inspire more people to bring their ideas to life in vivid, transformative ways.
To help identify AI-generated content, these creations will be watermarked using SynthID, and YouTube will apply a label that clearly communicates to viewers that it was generated with AI.
We’re continuing to improve our generative AI models and look forward to helping build technologies that unlock creativity for everyone, everywhere.
Earlier this year, we introduced our video generation model, Veo, and our latest image generation model, Imagen 3. Since then, it’s been exciting to w...
A note from Google and Alphabet CEO Sundar Pichai:
Last week, we rolled out our most capable model, Gemini [website] Ultra, and took a significant step for...
Research Building interactive agents in video game worlds Share.
Introducing a framework to create AI agents that can understand hu...
The next chapter of our Gemini era
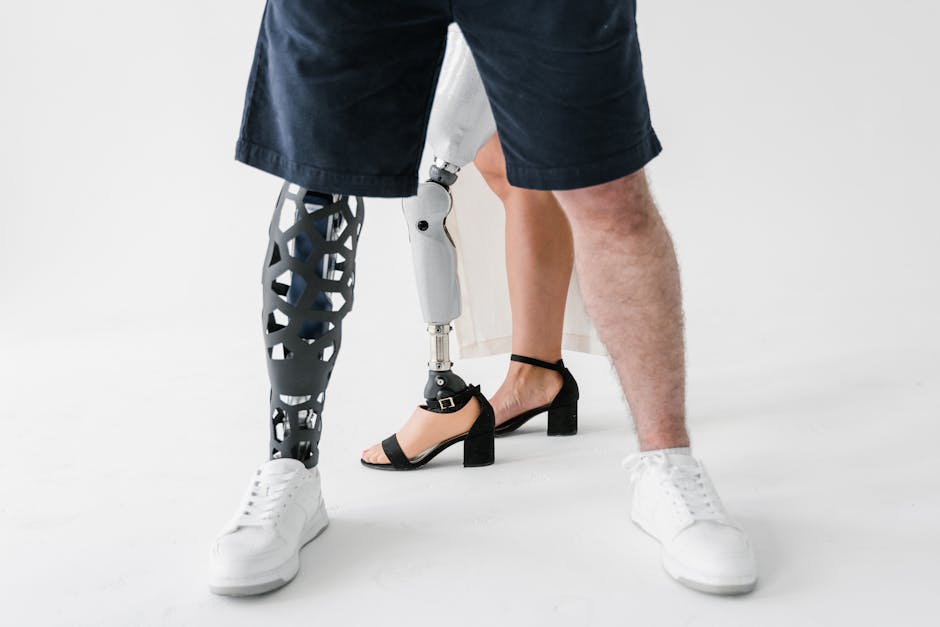
In December, we took a significant step on our journey to make AI more helpful for everyone with the start of the Gemini era, setting a new state of the art across a wide range of text, image, audio, and video benchmarks. However, Gemini is evolving to be more than just the models. It supports an entire ecosystem — from the products that billions of people use every day, to the APIs and platforms helping developers and businesses innovate.
The largest model Ultra [website] is the first to outperform human experts on MMLU (massive multitask language understanding), which uses a combination of 57 subjects — including math, physics, history, law, medicine and ethics — to test knowledge and problem-solving abilities.
Today we’re taking our next step and bringing Ultra to our products and the world.
Bard has been the best way for people to directly experience our most capable models. To reflect the advanced tech at its core, Bard will now simply be called Gemini. It’s available in 40 languages on the web, and is coming to a new Gemini app on Android and on the Google app on iOS.
The version with Ultra will be called Gemini Advanced, a new experience far more capable at reasoning, following instructions, coding, and creative collaboration. For example, it can be a personal tutor, tailored to your learning style. Or it can be a creative partner, helping you plan a content strategy or build a business plan. You can read more in this post.
You can start using Gemini Advanced by subscribing to the new Google One AI Premium plan, which offers the best of Google’s AI elements in a single place. This premium plan builds off the popular Google One service offering expanded storage and .
Impact Stopping malaria in its tracks Share.
Developing a improved malaria vaccine with the help of AI that could save hundreds of th...
corporation 2023: A Year of Groundbreaking Advances in AI and Computing Share.
This has been a year of incredible progress in the field...
Technologies Watermarking AI-generated text and video with SynthID Share.
Announcing our novel watermarking method for AI-generated...
Market Impact Analysis
Market Growth Trend
2018 | 2019 | 2020 | 2021 | 2022 | 2023 | 2024 |
---|---|---|---|---|---|---|
23.1% | 27.8% | 29.2% | 32.4% | 34.2% | 35.2% | 35.6% |
Quarterly Growth Rate
Q1 2024 | Q2 2024 | Q3 2024 | Q4 2024 |
---|---|---|---|
32.5% | 34.8% | 36.2% | 35.6% |
Market Segments and Growth Drivers
Segment | Market Share | Growth Rate |
---|---|---|
Machine Learning | 29% | 38.4% |
Computer Vision | 18% | 35.7% |
Natural Language Processing | 24% | 41.5% |
Robotics | 15% | 22.3% |
Other AI Technologies | 14% | 31.8% |
Technology Maturity Curve
Different technologies within the ecosystem are at varying stages of maturity:
Competitive Landscape Analysis
Company | Market Share |
---|---|
Google AI | 18.3% |
Microsoft AI | 15.7% |
IBM Watson | 11.2% |
Amazon AI | 9.8% |
OpenAI | 8.4% |
Future Outlook and Predictions
The Developing Reliable Tools landscape is evolving rapidly, driven by technological advancements, changing threat vectors, and shifting business requirements. Based on current trends and expert analyses, we can anticipate several significant developments across different time horizons:
Year-by-Year Technology Evolution
Based on current trajectory and expert analyses, we can project the following development timeline:
Technology Maturity Curve
Different technologies within the ecosystem are at varying stages of maturity, influencing adoption timelines and investment priorities:
Innovation Trigger
- Generative AI for specialized domains
- Blockchain for supply chain verification
Peak of Inflated Expectations
- Digital twins for business processes
- Quantum-resistant cryptography
Trough of Disillusionment
- Consumer AR/VR applications
- General-purpose blockchain
Slope of Enlightenment
- AI-driven analytics
- Edge computing
Plateau of Productivity
- Cloud infrastructure
- Mobile applications
Technology Evolution Timeline
- Improved generative models
- specialized AI applications
- AI-human collaboration systems
- multimodal AI platforms
- General AI capabilities
- AI-driven scientific breakthroughs
Expert Perspectives
Leading experts in the ai tech sector provide diverse perspectives on how the landscape will evolve over the coming years:
"The next frontier is AI systems that can reason across modalities and domains with minimal human guidance."
— AI Researcher
"Organizations that develop effective AI governance frameworks will gain competitive advantage."
— Industry Analyst
"The AI talent gap remains a critical barrier to implementation for most enterprises."
— Chief AI Officer
Areas of Expert Consensus
- Acceleration of Innovation: The pace of technological evolution will continue to increase
- Practical Integration: Focus will shift from proof-of-concept to operational deployment
- Human-Technology Partnership: Most effective implementations will optimize human-machine collaboration
- Regulatory Influence: Regulatory frameworks will increasingly shape technology development
Short-Term Outlook (1-2 Years)
In the immediate future, organizations will focus on implementing and optimizing currently available technologies to address pressing ai tech challenges:
- Improved generative models
- specialized AI applications
- enhanced AI ethics frameworks
These developments will be characterized by incremental improvements to existing frameworks rather than revolutionary changes, with emphasis on practical deployment and measurable outcomes.
Mid-Term Outlook (3-5 Years)
As technologies mature and organizations adapt, more substantial transformations will emerge in how security is approached and implemented:
- AI-human collaboration systems
- multimodal AI platforms
- democratized AI development
This period will see significant changes in security architecture and operational models, with increasing automation and integration between previously siloed security functions. Organizations will shift from reactive to proactive security postures.
Long-Term Outlook (5+ Years)
Looking further ahead, more fundamental shifts will reshape how cybersecurity is conceptualized and implemented across digital ecosystems:
- General AI capabilities
- AI-driven scientific breakthroughs
- new computing paradigms
These long-term developments will likely require significant technical breakthroughs, new regulatory frameworks, and evolution in how organizations approach security as a fundamental business function rather than a technical discipline.
Key Risk Factors and Uncertainties
Several critical factors could significantly impact the trajectory of ai tech evolution:
Organizations should monitor these factors closely and develop contingency strategies to mitigate potential negative impacts on technology implementation timelines.
Alternative Future Scenarios
The evolution of technology can follow different paths depending on various factors including regulatory developments, investment trends, technological breakthroughs, and market adoption. We analyze three potential scenarios:
Optimistic Scenario
Responsible AI driving innovation while minimizing societal disruption
Key Drivers: Supportive regulatory environment, significant research breakthroughs, strong market incentives, and rapid user adoption.
Probability: 25-30%
Base Case Scenario
Incremental adoption with mixed societal impacts and ongoing ethical challenges
Key Drivers: Balanced regulatory approach, steady technological progress, and selective implementation based on clear ROI.
Probability: 50-60%
Conservative Scenario
Technical and ethical barriers creating significant implementation challenges
Key Drivers: Restrictive regulations, technical limitations, implementation challenges, and risk-averse organizational cultures.
Probability: 15-20%
Scenario Comparison Matrix
Factor | Optimistic | Base Case | Conservative |
---|---|---|---|
Implementation Timeline | Accelerated | Steady | Delayed |
Market Adoption | Widespread | Selective | Limited |
Technology Evolution | Rapid | Progressive | Incremental |
Regulatory Environment | Supportive | Balanced | Restrictive |
Business Impact | Transformative | Significant | Modest |
Transformational Impact
Redefinition of knowledge work, automation of creative processes. This evolution will necessitate significant changes in organizational structures, talent development, and strategic planning processes.
The convergence of multiple technological trends—including artificial intelligence, quantum computing, and ubiquitous connectivity—will create both unprecedented security challenges and innovative defensive capabilities.
Implementation Challenges
Ethical concerns, computing resource limitations, talent shortages. Organizations will need to develop comprehensive change management strategies to successfully navigate these transitions.
Regulatory uncertainty, particularly around emerging technologies like AI in security applications, will require flexible security architectures that can adapt to evolving compliance requirements.
Key Innovations to Watch
Multimodal learning, resource-efficient AI, transparent decision systems. Organizations should monitor these developments closely to maintain competitive advantages and effective security postures.
Strategic investments in research partnerships, technology pilots, and talent development will position forward-thinking organizations to leverage these innovations early in their development cycle.
Technical Glossary
Key technical terms and definitions to help understand the technologies discussed in this article.
Understanding the following technical concepts is essential for grasping the full implications of the security threats and defensive measures discussed in this article. These definitions provide context for both technical and non-technical readers.