FACTS Grounding: A new benchmark for evaluating the factuality of large language models - Related to now, here's, a, chatgpt, an
FACTS Grounding: A new benchmark for evaluating the factuality of large language models
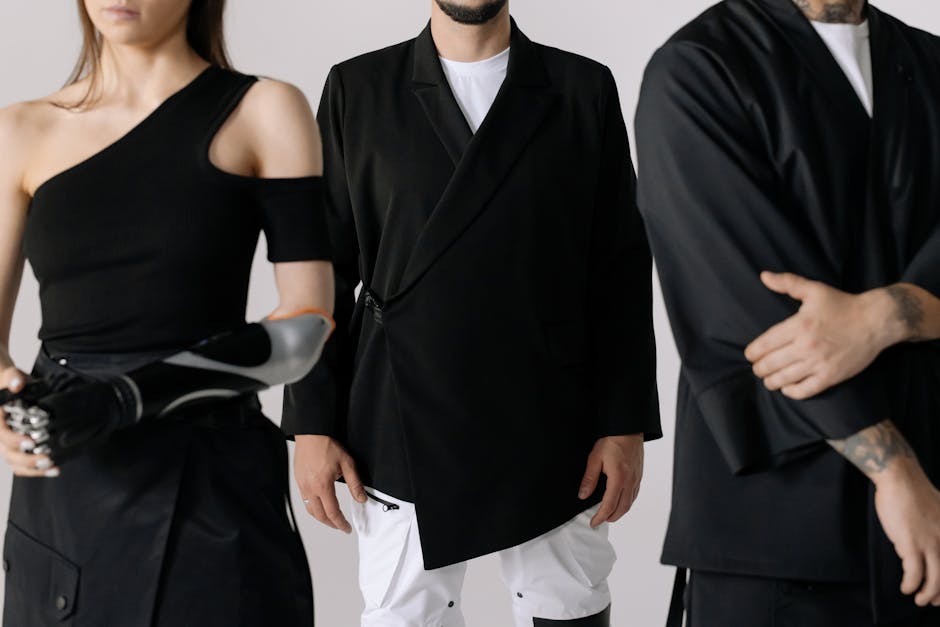
Our comprehensive benchmark and online leaderboard offer a much-needed measure of how accurately LLMs ground their responses in provided source material and avoid hallucinations.
Large language models (LLMs) are transforming how we access information, yet their grip on factual accuracy remains imperfect. They can “hallucinate” false information, particularly when given complex inputs. In turn, this can erode trust in LLMs and limit their applications in the real world.
Today, we’re introducing FACTS Grounding, a comprehensive benchmark for evaluating the ability of LLMs to generate responses that are not only factually accurate with respect to given inputs, but also sufficiently detailed to provide satisfactory answers to user queries.
We hope our benchmark will spur industry-wide progress on factuality and grounding. To track progress, we’re also launching the FACTS leaderboard on Kaggle. We’ve already tested leading LLMs using FACTS Grounding and have populated the initial leaderboard with their grounding scores. We will maintain and enhancement the leaderboard as the field advances.
Quantum computers have the potential to revolutionize drug discovery, material design and fundamental physics — that is, if we can get them to work re...
In my professional life as a data scientist, I have encountered time series multiple times. Most of my knowledge comes from my academic experience, sp...
Impact MuZero, AlphaZero, and AlphaDev: Optimizing computer systems Share.
As part of our aim to build increasingly capable and gen...
Using AI to fight climate change
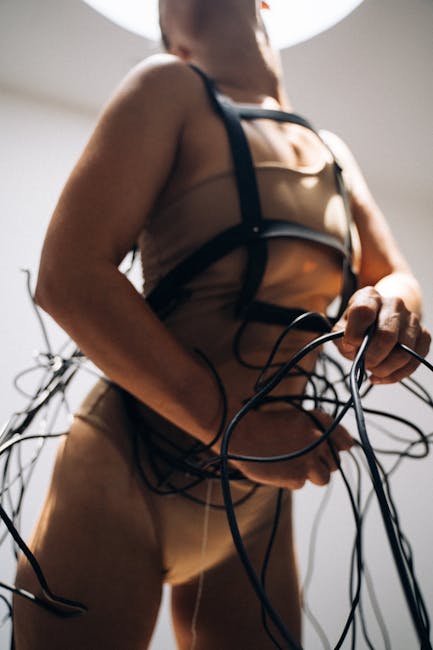
How we’re applying the latest AI developments to help fight climate change and build a more sustainable, low-carbon world.
AI is a powerful technology that will transform our future, so how can we best apply it to help combat climate change and find sustainable solutions?
Our climate & sustainability lead, Sims Witherspoon, who recently spoke about how AI can accelerate our transition to renewables at TED Countdown, explains, “Climate change is a multifaceted problem with no single solution. We need to move beyond discussing what we can do and start focusing on how we can do it.”.
The effects of climate change on Earth’s ecosystems are incredibly complex, and as part of our effort to use AI for solving some of the world’s most challenging problems, here are some of the ways we’re working to advance our understanding, optimise existing systems, and accelerate breakthrough science of climate and its effects.
Understand weather, climate, and their effects.
more effective understanding the core problems and their effects is a critical first step to tackling climate change. Working together with the UK Met Office, we developed a precipitation nowcasting model to more effective understand changing weather. This nowcasting model is more accurate than the existing state of the art, and much preferred by the Met’s expert meteorologists. Our climate and weather research spans short-range (under two hours) to medium-range (ten days) forecasting, which can hugely impact how we optimise renewable energy systems that are based on natural resources.
From modelling the behaviour of animal species across the Serengeti to supporting machine learning projects that advance conservation projects in Africa, we’ve been helping scientists track and improved understand the effects of climate change on ecosystems and biodiversity. Going forward, our team is also building upon AI systems used to identify bird song in Australia, helping advance tools that monitor changing wildlife at scale.
Moreover, we’re partnering with non-profit Climate Change AI to close critical gaps in climate-related data. Currently, this partnership focuses on building a comprehensive wishlist of datasets whose availability would advance AI solutions for climate change. We’ll make this wishlist available to the wider public when it’s complete.
While we transition to more sustainable infrastructure, we need to optimise the systems the world depends on today. For example, today’s computing infrastructure, including AI itself, is energy-intensive. To help solve some of these issues, we’ve been developing AI that can enhance existing systems, including optimising industrial cooling and more efficient computer systems.
Given our energy grids are not yet running on clean energy, it's critical we use our resources as efficiently as possible while we work on the transition to renewables. Accelerating the global transition to renewable energy reports can also greatly reduce carbon emissions.
In 2019, our climate & sustainability team collaborated with domain experts at a Google-owned wind farm to increase the value of wind energy – ultimately, aiming to support growth across the broader industry. By developing a custom AI tool to better predict wind power output and another model to recommend commitments to supply this anticipated energy to the electricity grid, this tool greatly boosted the value of the wind energy. Cloud is now developing a software product using this model, which is being piloted by French power company ENGIE.
Research Google DeepMind at ICLR 2024 Share.
Developing next-gen AI agents, exploring new modalities, and pioneering foundational l...
Applying our AI research to help enrich the lives of billions of people around the world.
Building useful products with new technologies has always be...
Technologies Pushing the frontiers of audio generation Share.
Our pioneering speech generation technologies are helping people arou...
You can access ChatGPT Search without an account now - here's how
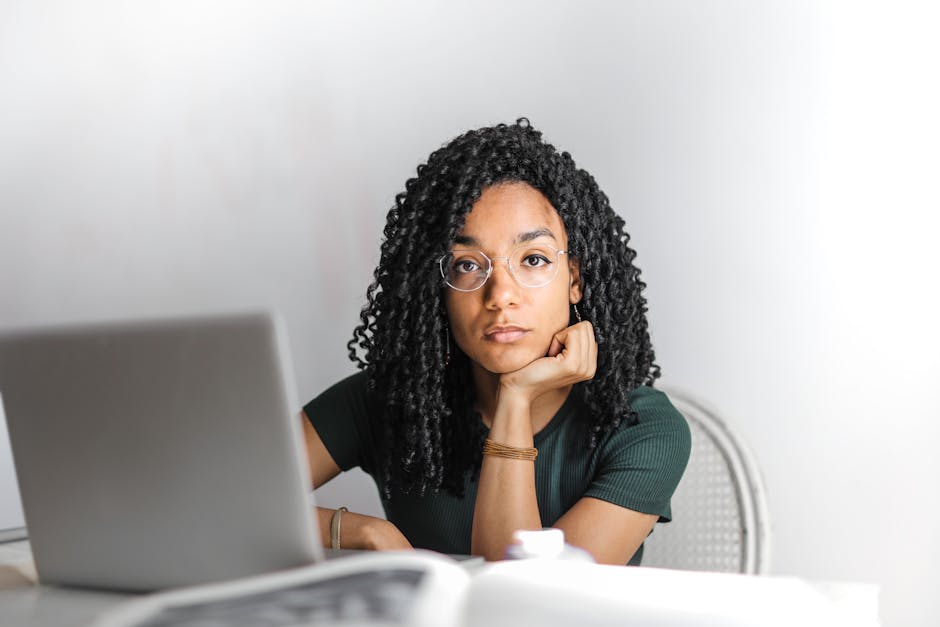
The best way to get the most out of an AI chatbot is verifying its findings and letting it direct you to more robust and useful content regarding the topic. Therefore, AI search engines are a powerful tool for everyday workflows, and, now, accessing ChatGPT Search just got a whole lot easier.
Also: How to make ChatGPT provide improved information and citations.
ChatGPT Search, originally launched in October, lets individuals search the web directly within the ChatGPT interface for timely, up-to-date information, complete with citations linked to insights. There are two big advantages that differentiate it from a traditional search engine: using conversational search queries, and getting a more personalized results page.
With ChatGPT Search, you can enter a fragmented or unorganized sentence that follows your train of thought, and the AI can still understand the meaning of your query by leveraging its natural language processing capabilities. The result is a simplified search experience in which you can spend less time crafting a tailored search query or thinking of specific keywords.
Also: The best AI for coding in 2025 (and what not to use).
For example, if you wanted to know what the technical name for a bee humming is, instead of generally searching "bee hums" on Google, you could say something like, "What is the scientific term for the sound a bee makes when it flies around you? It kinda sounds like a hum."
You can try ChatGPT Search for yourself for free now. You can also compare it to the many AI search engines available on the market for free, which we rounded up for you here.
When you're inviting friends, family, or co-workers to an event, the most convenient option i...
Firefox people can now tap into their favorite AI directly from th...
SOPA Images / Contributor / Getty Images.
Although you may be tuning into the Super Bowl for the foo...
Market Impact Analysis
Market Growth Trend
2018 | 2019 | 2020 | 2021 | 2022 | 2023 | 2024 |
---|---|---|---|---|---|---|
23.1% | 27.8% | 29.2% | 32.4% | 34.2% | 35.2% | 35.6% |
Quarterly Growth Rate
Q1 2024 | Q2 2024 | Q3 2024 | Q4 2024 |
---|---|---|---|
32.5% | 34.8% | 36.2% | 35.6% |
Market Segments and Growth Drivers
Segment | Market Share | Growth Rate |
---|---|---|
Machine Learning | 29% | 38.4% |
Computer Vision | 18% | 35.7% |
Natural Language Processing | 24% | 41.5% |
Robotics | 15% | 22.3% |
Other AI Technologies | 14% | 31.8% |
Technology Maturity Curve
Different technologies within the ecosystem are at varying stages of maturity:
Competitive Landscape Analysis
Company | Market Share |
---|---|
Google AI | 18.3% |
Microsoft AI | 15.7% |
IBM Watson | 11.2% |
Amazon AI | 9.8% |
OpenAI | 8.4% |
Future Outlook and Predictions
The Facts Grounding Benchmark landscape is evolving rapidly, driven by technological advancements, changing threat vectors, and shifting business requirements. Based on current trends and expert analyses, we can anticipate several significant developments across different time horizons:
Year-by-Year Technology Evolution
Based on current trajectory and expert analyses, we can project the following development timeline:
Technology Maturity Curve
Different technologies within the ecosystem are at varying stages of maturity, influencing adoption timelines and investment priorities:
Innovation Trigger
- Generative AI for specialized domains
- Blockchain for supply chain verification
Peak of Inflated Expectations
- Digital twins for business processes
- Quantum-resistant cryptography
Trough of Disillusionment
- Consumer AR/VR applications
- General-purpose blockchain
Slope of Enlightenment
- AI-driven analytics
- Edge computing
Plateau of Productivity
- Cloud infrastructure
- Mobile applications
Technology Evolution Timeline
- Improved generative models
- specialized AI applications
- AI-human collaboration systems
- multimodal AI platforms
- General AI capabilities
- AI-driven scientific breakthroughs
Expert Perspectives
Leading experts in the ai tech sector provide diverse perspectives on how the landscape will evolve over the coming years:
"The next frontier is AI systems that can reason across modalities and domains with minimal human guidance."
— AI Researcher
"Organizations that develop effective AI governance frameworks will gain competitive advantage."
— Industry Analyst
"The AI talent gap remains a critical barrier to implementation for most enterprises."
— Chief AI Officer
Areas of Expert Consensus
- Acceleration of Innovation: The pace of technological evolution will continue to increase
- Practical Integration: Focus will shift from proof-of-concept to operational deployment
- Human-Technology Partnership: Most effective implementations will optimize human-machine collaboration
- Regulatory Influence: Regulatory frameworks will increasingly shape technology development
Short-Term Outlook (1-2 Years)
In the immediate future, organizations will focus on implementing and optimizing currently available technologies to address pressing ai tech challenges:
- Improved generative models
- specialized AI applications
- enhanced AI ethics frameworks
These developments will be characterized by incremental improvements to existing frameworks rather than revolutionary changes, with emphasis on practical deployment and measurable outcomes.
Mid-Term Outlook (3-5 Years)
As technologies mature and organizations adapt, more substantial transformations will emerge in how security is approached and implemented:
- AI-human collaboration systems
- multimodal AI platforms
- democratized AI development
This period will see significant changes in security architecture and operational models, with increasing automation and integration between previously siloed security functions. Organizations will shift from reactive to proactive security postures.
Long-Term Outlook (5+ Years)
Looking further ahead, more fundamental shifts will reshape how cybersecurity is conceptualized and implemented across digital ecosystems:
- General AI capabilities
- AI-driven scientific breakthroughs
- new computing paradigms
These long-term developments will likely require significant technical breakthroughs, new regulatory frameworks, and evolution in how organizations approach security as a fundamental business function rather than a technical discipline.
Key Risk Factors and Uncertainties
Several critical factors could significantly impact the trajectory of ai tech evolution:
Organizations should monitor these factors closely and develop contingency strategies to mitigate potential negative impacts on technology implementation timelines.
Alternative Future Scenarios
The evolution of technology can follow different paths depending on various factors including regulatory developments, investment trends, technological breakthroughs, and market adoption. We analyze three potential scenarios:
Optimistic Scenario
Responsible AI driving innovation while minimizing societal disruption
Key Drivers: Supportive regulatory environment, significant research breakthroughs, strong market incentives, and rapid user adoption.
Probability: 25-30%
Base Case Scenario
Incremental adoption with mixed societal impacts and ongoing ethical challenges
Key Drivers: Balanced regulatory approach, steady technological progress, and selective implementation based on clear ROI.
Probability: 50-60%
Conservative Scenario
Technical and ethical barriers creating significant implementation challenges
Key Drivers: Restrictive regulations, technical limitations, implementation challenges, and risk-averse organizational cultures.
Probability: 15-20%
Scenario Comparison Matrix
Factor | Optimistic | Base Case | Conservative |
---|---|---|---|
Implementation Timeline | Accelerated | Steady | Delayed |
Market Adoption | Widespread | Selective | Limited |
Technology Evolution | Rapid | Progressive | Incremental |
Regulatory Environment | Supportive | Balanced | Restrictive |
Business Impact | Transformative | Significant | Modest |
Transformational Impact
Redefinition of knowledge work, automation of creative processes. This evolution will necessitate significant changes in organizational structures, talent development, and strategic planning processes.
The convergence of multiple technological trends—including artificial intelligence, quantum computing, and ubiquitous connectivity—will create both unprecedented security challenges and innovative defensive capabilities.
Implementation Challenges
Ethical concerns, computing resource limitations, talent shortages. Organizations will need to develop comprehensive change management strategies to successfully navigate these transitions.
Regulatory uncertainty, particularly around emerging technologies like AI in security applications, will require flexible security architectures that can adapt to evolving compliance requirements.
Key Innovations to Watch
Multimodal learning, resource-efficient AI, transparent decision systems. Organizations should monitor these developments closely to maintain competitive advantages and effective security postures.
Strategic investments in research partnerships, technology pilots, and talent development will position forward-thinking organizations to leverage these innovations early in their development cycle.
Technical Glossary
Key technical terms and definitions to help understand the technologies discussed in this article.
Understanding the following technical concepts is essential for grasping the full implications of the security threats and defensive measures discussed in this article. These definitions provide context for both technical and non-technical readers.