From Resume to Cover Letter Using AI and LLM, with Python and Streamlit - Related to cover, against, deepmind, mentor, using
From Resume to Cover Letter Using AI and LLM, with Python and Streamlit
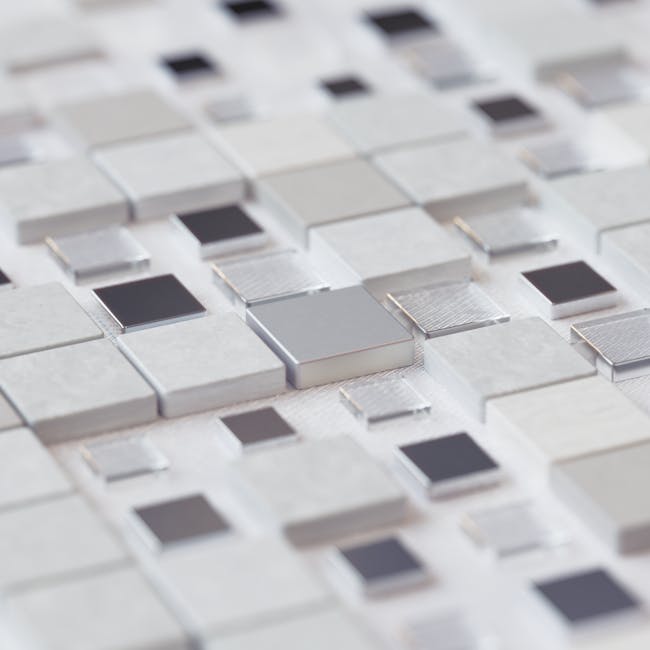
DISCLAIMER: The idea of doing Cover Letter or even Resume with AI does not obviously start with me. A lot of people have done this before (very successfully) and have built websites and even companies from the idea. This is just a tutorial on how to build your own Cover Letter AI Generator App using Python and a few lines of code. All the code you will say in this blog post can be found in my public Github folder. Enjoy. 🙂.
Pep Guardiola is a (very successful) Manchester City football coach. During Barcelona’s Leo Messi years, he invented a way of playing football known as “Tiki-Taka”. This means that as soon as you receive the ball, you pass the ball, immediately, without even controlling it. You can pass the ball 30–40 times before scoring a goal.
More than a decade later, we can see how the way of playing football made Guardiola and his Barcelona famous is gone. If you look at a Manchester City match, they take the ball and immediately look for the striker or the winger. You only need a few, vertical passes, immediately looking for the opportunity. It is more predictable, but you do it so many times that you will eventually find the space to hit the target.
I think that the job market has somehow gone in the same direction.
Before you had the opportunity to go to the business, hand in your resume, talk to them, be around them, schedule an interview, and actively talk to people. You would spend weeks preparing for that trip, polishing your resume, and reviewing questions and answers.
For many, this old-fashioned strategy still works, and I believe it. If you have a good networking opportunity, or the right time and place, the handing the resume thing works very well. We love the human connection, and it is very effective to actually know someone.
It is essential to consider that there is a whole other approach as well. Companies like LinkedIn, Indeed, and even in general the internet completely changed the game. You can send so many resumes to so many companies and find a job out of statistics. AI is changing this game a little bit further. There are a lot of AI tools to tailor your resume for the specific corporation, make your resume more impressive, or build the job specific cover letter. There are indeed many companies that sell this kind of services to people that are looking for jobs.
Now, believe me, I have got nothing against these companies, at all, but the AI that they are using it’s not really “their AI”. What I mean by that is that if you use ChatGPT, Gemini, or the super new DeepSeek to do the exact task you will very likely not get a worse response than the (paid) tool that you are using on their website. You are really paying for the “commodity” of having a backend API that does what we would have to do through ChatGPT. And that’s fair.
Nonetheless, I want to show you that it is indeed very simple and cheap to make your own “resume assistant” using Large Language Models. In particular, I want to focus on cover letters. You give me your resume and the job description, and I give you your cover letter you can copy and paste to LinkedIn, Indeed, or your email.
Image made by the author, credits on the image.
Now, Large Language Models (LLMs) are specific AI models that produce text. More specifically, they are HUGE Machine Learning models (even the small ones are very big).
This means that building your own LLM or training one from scratch is very, very expensive. We won’t do anything like that. We will use a perfectly working LLM and we will smartly instruct it to perform our task. More specifically, we will do that in Python and using some APIs. Formally, it is a paid API. Nonetheless, since I started the whole project (with all the trial and error process) I spent less than 30 cents. You will likely spend 4 or 5 cents on it.
To motivate you, here are screenshots of the final app:
Pretty cool right? It took me less than 5 hours to build the whole thing from scratch. Believe me: it’s that simple. In this blog post, we will describe, in order:
The LLM API Strategy. This part will help the reader understand what LLM Agents we are using and how we are connecting them. The LLM Object. This is the implementation of the LLM API strategy above using Python. The Web App and results. The LLM Object is then transferred into a web app using Streamlit. I’ll show you how to access it and some results.
I’ll try to be as specific as possible so that you have everything you need to make it yourself, but if this stuff gets too technical, feel free to skip to part 3 and just enjoy the sunset 🙃.
This is the Machine Learning System Design part of this project, which I kept extremely light, because I wanted to maximize the readability of the whole approach (and because it honestly didn’t need to be more complicated than that).
A Document Parser LLM API will read the Resume and extract all the meaningful information. This information will be put in a .json file so that, in production, we will have the resume already processed and stored somewhere in our memory. A cover letter LLM API. This API will read the parsed resume (the output of the previous API) and the job description and it will output the Cover Letter.
Image made by the author, credits on the image.
What is the best LLM for this task? For text extraction and summarization, LLama or Gemma are known to be a reasonably cheap and efficient LLM. As we are going to use LLama for the summarization task, in order to keep consistency, we can adopt it for the other API as well. If you want to use another model, feel free to do so. How do we connect the APIs? There are a variety of ways you can do that. I decided to give it a try to Llama API. The documentation is not exactly extensive, but it works well and it allows you to play with many models. You will need to log in, buy some credit ($1 is more than sufficient for this task), and save your API key. Feel free to switch to another solution (like Hugging Face or Langchain) if you feel like it.
Ok, now that we know what to do, we just need to actually implement it in Python.
The first thing that we need is the actual LLM prompts. In the API, the prompts are usually passed using a dictionary. As they can be pretty long, and their structure is always similar, it makes sense to store them in .json files. We will read the JSON files and use them as inputs for the API call.
In this .json file, you will have the model (you can call whatever model you like) and the content which is the instruction for the LLM. Of course, the content key has a static part, which is the “instruction” and a “dynamic” part, which is the specific input of the API call. For example: this is the .json file for the first API, I called it [website].
As you can see from the “content” there is the static call:
“You are a resume parser. You will extract information from this resume and put them in a .json file. The keys of your dictionary will be first_name, last_name, location, work_experience, school_experience, skills. In selecting the information, keep track of the most insightful.”.
The keys I want to extract out of my “.json” files are:
[first_name, last_name, location, work_experience, school_experience, skills].
Feel free to add anything more information that you want to be “extracted” out of your resume, but remember that this is stuff that should matter only for your cover letter. The specific resume will be added after this text to form the full call/instruction. More on that later.
“You are an expert in job hunting and a cover letter writer. Given a resume json file, the job description, and the date, write a cover letter for this candidate. Be persuasive and professional. Resume JSON: {resume_json} ; Job Description: {job_description}, Date: {date}”.
As you can see, there are three placeholders: “Resume_json”, “job_description” and “date”. As before, these placeholders will then be replaced with the correct information to form the full prompt.
I made a very small [website] file with the path of the two .json prompt files and the API that you have to generate from LLamaApi (or really whatever API you are using). Modify this if you want to run the file locally.
This file is a collection of “loaders” for your resume. Boring stuff but key.
The whole implementation of the LLM Strategy can be found in this object that I called CoverLetterAI. There it is:
I spent quite some time trying to make everything modular and easy to read. I also made a lot of comments to all the functions so you can see exactly what does what. How do we use this beast?
So the whole code runs in 5 simple lines. Like this:
cover_letter_AI.read_candidate_data('path_to_your_resume_file').
cover_letter_AI.add_job_description('Insert job description').
You call the CoverLetterAI object. It will be the star of the show You give me the path to your resume. It can be PDF or Word and I read your information and store them in a variable. You call profile_candidate(), and I run my first LLM. This process the candidate word info and creates the .json file we will use for the second LLM You give me the job_description and you add it to the system. Stored. You call write_cover_letter() and I run my second LLM that generates, given the job description and the resume .json file, the cover letter.
So that is really it. You saw all the technical details of this blog post in the previous paragraphs.
Now, the cover letters that are generated are scary good.
[firm I am intentionally blurring] I am thrilled to apply for the Distinguished AI Engineer position at [firm I am intentionally blurring], where I can leverage my passion for building responsible and scalable AI systems to revolutionize the banking industry. As a seasoned machine learning engineer and researcher with a strong background in physics and engineering, I am confident that my skills and experience align with the requirements of this role. With a [website] in Aerospace Engineering and Engineering Mechanics from the University of Cincinnati and a Master’s degree in Physics of Complex Systems and Big Data from the University of Rome Tor Vergata, I possess a unique blend of theoretical and practical knowledge. My experience in developing and deploying AI models, designing and implementing machine learning algorithms, and working with large datasets has equipped me with the skills to drive innovation in AI engineering. As a Research and Teaching Assistant at the University of Cincinnati, I applied surrogate models to detect and classify cracks in pipes, achieving a 14% improvement in damage detection experiments. I also developed surrogate models using deep learning algorithms to accelerate Finite Element Methods (FEM) simulations, resulting in a 1M-fold reduction in computational time. My experience in teaching and creating courses in signal processing and image processing for teens interested in AI has honed my ability to communicate complex concepts effectively. In my previous roles as a Machine Learning Engineer at Gen Nine, Inc., Apex Microdevices, and Accenture, I have successfully designed, developed, and deployed AI-powered solutions, including configuring mmWave radar and Jetson devices for data collection, implementing state-of-the-art point cloud algorithms, and leading the FastMRI project to accelerate MRI scan times. My expertise in programming languages such as Python, TensorFlow, PyTorch, and MATLAB, as well as my experience with cloud platforms like AWS, Docker, and Kubernetes, has enabled me to develop and deploy scalable AI solutions. I am particularly drawn to [firm I am intentionally blurring] commitment to creating responsible and reliable AI systems that prioritize customer experience and simplicity. My passion for staying abreast of the latest AI research and my ability to judiciously apply novel techniques in production align with the firm’s vision. I am excited about the opportunity to work with a cross-functional team of engineers, research scientists, and product managers to deliver AI-powered products that transform how [firm I am intentionally blurring] serves its individuals. In addition to my technical skills and experience, I possess excellent communication and presentation skills, which have been demonstrated through my technical writing experience at Towards Data Science, where I have written comprehensive articles on machine learning and data science, reaching a broad audience of 50k+ monthly viewers. Thank you for considering my application. I am eager to discuss how my skills and experience can contribute to the success of the [firm I am intentionally blurring] and [firm I am intentionally blurring]’s mission to bring humanity and simplicity to banking through AI. I am confident that my passion for AI, my technical expertise, and my ability to work collaboratively will make me a valuable asset to your team. Sincerely, Piero Paialunga.
They look just like I would write them for a specific job description. That being expressed, in 2025, you need to be careful because hiring managers do know that you are using AI to write them and the “computer tone” is pretty easy to spot ([website] words like “eager” are very ChatGPT-ish lol). For this reason, I’d like to say to use these tools wisely. Sure, you can build your “template” with them, but be sure to add your personal touch, otherwise your cover letter will be exactly like the other thousands of cover letters that the other applicants are sending in.
In this blog article, we discovered how to use LLM to convert your resume and job description into a specific cover letter. These are the points we touched:
The use of AI in job hunting. In the first chapter we discussed how job hunting is now completely revolutionized by AI. Large Language Models idea. It is crucial to design the LLM APIs wisely. We did that in the second paragraph LLM API implementation. We used Python to implement the LLM APIs organically and efficiently The Web App. We used streamlit to build a Web App API to display the power of this approach. Limits of this approach. I think that AI generated cover letters are indeed very good. They are on point, professional and well crafted. Nonetheless, if everyone starts using AI to build cover letters, they all really look the same, or at least they all have the same tone, which is not great. Something to think about.
5. References and other brilliant implementations.
I feel that is just fair to mention a lot of brilliant people that have had this idea before me and have made this public and available for anyone. This is only a few of them I found online.
Cover Letter Craft by Balaji Kesavan is a Streamlit app that implements a very similar idea of crafting the cover letter using AI. What we do different from that app is that we extract the resume directly from the word or PDF, while his app requires copy-pasteing. That being expressed, I think the guy is incredibly talented and very creative and I recommend giving a look to his portoflio.
Randy Pettus has a similar idea as well. The difference between his approach and the one proposed in this tutorial is that he is very specific in the information, asking questions like “current hiring manager” and the temperature of the model. It’s very interesting (and smart) that you can clearly see the way he is thinking of Cover Letters to guide the AI to build it the way he likes them. Highly recommended.
Juan Esteban Cepeda does a very good job in his app as well. You can also tell that he was working on making it bigger than a simple streamlit add because he added the link to his enterprise and a bunch of reviews by people. Great job and great hustle. 🙂.
Thank you again for your time. It means a lot ❤.
My name is Piero Paialunga and I’m this guy here:
I am a [website] candidate at the University of Cincinnati Aerospace Engineering Department and a Machine Learning Engineer for Gen Nine. I talk about AI, and Machine Learning in my blog posts and on Linkedin. If you liked the article and want to know more about machine learning and follow my studies you can:
A. Follow me on Linkedin, where I publish all my stories.
C. Become a referred member, so you won’t have any “maximum number of stories for the month” and you can read whatever I (and thousands of other Machine Learning and Data Science top writers) write about the newest technology available.
D. Want to work with me? Check my rates and projects on Upwork!
If you want to ask me questions or start a collaboration, leave a message here or on Linkedin:
Can you jailbreak Anthropic's latest AI safety measure? Researchers want you t...
Deepseek has in the recent past made quite a buzz in the AI community, thanks to its impressive performance at...
We all have to deal with contracts and agreements as consumers or business professionals. How...
The Cultural Backlash Against Generative AI
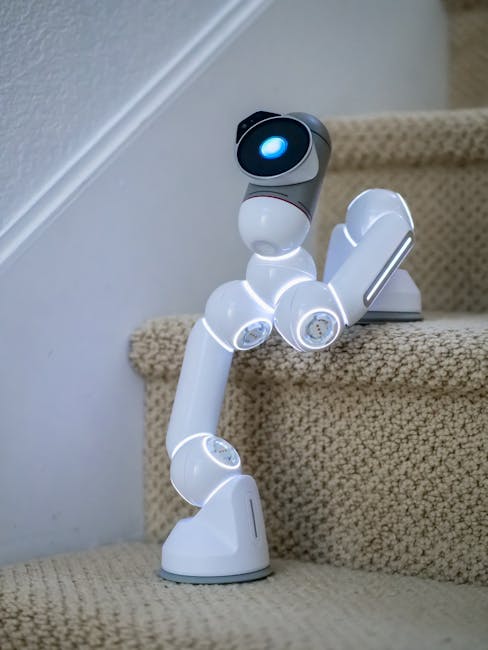
The Cultural Backlash Against Generative AI.
What’s making many people resent generative AI, and what impact does that have on the companies responsible? Stephanie Kirmer · Follow · 9 min read · 18 hours ago 18 hours ago -- Listen Share.
The recent reveal of DeepSeek-R1, the large scale LLM developed by a Chinese enterprise (also named DeepSeek), has been a very interesting event for those of us who spend time observing and analyzing the cultural and social phenomena around AI. Evidence points to that R1 was trained for a fraction of the price that it cost to train ChatGPT (any of their recent models, really), and there are a few reasons that might be true. But that’s not really what I want to talk about here — tons of thoughtful writers have commented on what DeepSeek-R1 is, and what really happened in the training process.
What I’m more interested in at the moment is how this news shifted some of the momentum in the AI space. Nvidia and other related stocks dropped precipitously when the news of DeepSeek-R1 came out, largely (it seems) because it didn’t require the newest GPUs to train, and by training more efficiently, it required less power than an OpenAI model. I had already been thinking about the cultural backlash that Big Generative AI was facing, and something like this opens up even more space for people to be critical of the practices and promises of generative AI companies.
Where are we in terms of the critical voices against generative AI as a business or as a technology? Where is that coming from, and why might it be occurring?
The two often overlapping angles of criticism that I think are most interesting are first, the social or community good perspective, and second, the practical perspective. From a social good perspective, critiques of generative AI as a business and an industry are myriad, and I’ve talked a lot about them in my writing here. Making generative AI into something ubiquitous comes at extraordinary costs, from the environmental to the economic and beyond.
As a practical matter, it might be simplest to boil it down to “this technology doesn’t work the way we were promised”. Generative AI lies to us, or “hallucinates”, and it performs poorly on many of the kinds of tasks that we have most need for technological help on. We are led to believe we can trust this technology, but it fails to meet expectations, while simultaneously being used for such misery-inducing and criminal things as synthetic CSAM and deepfakes to undermine democracy.
So when we look at these together, you can develop a pretty strong argument: this technology is not living up to the overhyped expectations, and in exchange for this underwhelming performance, we’re giving up electricity, water, climate, money, culture, and jobs. Not a worthwhile trade, in many people’s eyes, to put it mildly!
I do like to bring a little nuance to the space, because I think when we accept the limitations on what generative AI can do, and the harm it can cause, and don’t play the overhype game, we can find a passable middle ground. I don’t think we should be paying the steep price for training and for inference of these models unless the results are really, REALLY worth it. Developing new molecules for medical research? Maybe, yes. Helping kids cheat (poorly) on homework? No thanks. I’m not even sure it’s worth the externality cost to help me write code a little bit more efficiently at work, unless I’m doing something really valuable. We need to be honest and realistic about the true price of both creating and using this technology.
So, with that noted, I’d like to dive in and look at how this situation came to be. I wrote way back in September 2023 that machine learning had a public perception problem, and in the case of generative AI, I think that has been proven out by events. Specifically, if people don’t have realistic expectations and understanding of what LLMs are good for and what they’re not good for, they’re going to bounce off, and backlash will ensue.
“My argument goes something like this: 1. People are not naturally prepared to understand and interact with machine learning. 2. Without understanding these tools, some people may avoid or distrust them. 3. Worse, some individuals may misuse these tools due to misinformation, resulting in detrimental outcomes. 4. After experiencing the negative consequences of misuse, people might become reluctant to adopt future machine learning tools that could enhance their lives and communities.” me, in Machine Learning’s Public Perception Problem, Sept 2023.
So what happened? Well, the generative AI industry dove head first into the problem and we’re seeing the repercussions.
Generative AI applications don’t meet people’s needs.
Part of the problem is that generative AI really can’t effectively do everything the hype asserts. An LLM can’t be reliably used to answer questions, because it’s not a “facts machine”. It’s a “probable next word in a sentence machine”. But we’re seeing promises of all kinds that ignore these limitations, and tech companies are forcing generative AI elements into every kind of software you can think of. People hated Microsoft’s Clippy because it wasn’t any good and they didn’t want to have it shoved down their throats — and one might say they’re doing the same basic thing with an improved version, and we can see that some people still understandably resent it.
When someone goes to an LLM today and asks for the price of ingredients in a recipe at their local grocery store right now, there’s absolutely no chance that model can answer that correctly, reliably. That is not within its capabilities, because the true data about those prices is not available to the model. The model might accidentally guess that a bag of carrots is $[website] at Publix, but it’s just that, an accident. In the future, with chaining models together in agentic forms, there’s a chance we could develop a narrow model to do this kind of thing correctly, but right now it’s absolutely bogus.
But people are asking LLMs these questions today! And when they get to the store, they’re very disappointed about being lied to by a technology that they thought was a magic answer box. If you’re OpenAI or Anthropic, you might shrug, because if that person was paying you a monthly fee, well, you already got the cash. And if they weren’t, well, you got the user number to tick up one more, and that’s growth.
However, this is actually a major business problem. When your product fails like this, in an obvious, predictable (inevitable!) way, you’re beginning to singe the bridge between that user and your product. It may not burn it all at once, but it’s gradually tearing down the relationship the user has with your product, and you only get so many chances before someone gives up and goes from a user to a critic. In the case of generative AI, it seems to me like you don’t get many chances at all. Plus, failure in one mode can make people mistrust the entire technology in all its forms. Is that user going to trust or believe you in a few years when you’ve hooked up the LLM backend to realtime price APIs and can in fact correctly return grocery store prices? I doubt it. That user might not even let your model help revise emails to coworkers after it failed them on some other task.
From what I can see, tech companies think they can just wear people down, forcing them to accept that generative AI is an inescapable part of all their software now, whether it works or not. Maybe they can, but I think this is a self defeating strategy. people may trudge along and accept the state of affairs, but they won’t feel positive towards the tech or towards your brand as a result. Begrudging acceptance is not the kind of energy you want your brand to inspire among people!
You might think, well, that’s clear enough —let’s back off on the generative AI aspects in software, and just apply it to tasks where it can wow the user and works well. They’ll have a good experience, and then as the technology gets advanced, we’ll add more where it makes sense. And this would be somewhat reasonable thinking (although, as I mentioned before, the externality costs will be extremely high to our world and our communities).
However, I don’t think the big generative AI players can really do that, and here’s why. Tech leaders have spent a truly exorbitant amount of money on creating and trying to improve this technology — from investing in companies that develop it, to building power plants and data centers, to lobbying to avoid copyright laws, there are hundreds of billions of dollars sunk into this space already with more soon to come.
In the tech industry, profit expectations are quite different from what you might encounter in other sectors — a VC funded software startup has to make back 10–100x what’s invested (depending on stage) to look like a really standout success. So investors in tech push companies, explicitly or implicitly, to take bigger swings and bigger risks in order to make higher returns plausible. This starts to develop into what we call a “bubble” — valuations become out of alignment with the real economic possibilities, escalating higher and higher with no hope of ever becoming reality. As Gerrit De Vynck in the Washington Post noted, “… Wall Street analysts are expecting Big Tech companies to spend around $60 billion a year on developing AI models by 2026, but reap only around $20 billion a year in revenue from AI by that point… Venture capitalists have also poured billions more into thousands of AI start-ups. The AI boom has helped contribute to the $[website] billion that venture investors put into [website] start-ups in the second quarter of 2024, the highest amount in a single quarter in two years, .”.
So, given the billions invested, there are serious arguments to be made that the amount invested in developing generative AI to date is impossible to match with returns. There just isn’t that much money to be made here, by this technology, certainly not in comparison to the amount that’s been invested. But, companies are certainly going to try. I believe that’s part of the reason why we’re seeing generative AI inserted into all manner of use cases where it might not actually be particularly helpful, effective, or welcomed. In a way, “we’ve spent all this money on this technology, so we have to find a way sell it” is kind of the framework. Keep in mind, too, that the investments are continuing to be sunk in to try and make the tech work more effective, but any LLM advancement these days is proving very slow and incremental.
Generative AI tools are not proving essential to people’s lives, so the economic calculus is not working to make a product available and convince folks to buy it. So, we’re seeing companies move to the “feature” model of generative AI, which I theorized could happen in my article from August 2024. However, the approach is taking a very heavy hand, as with Microsoft adding generative AI to Office365 and making the aspects and the accompanying price increase both mandatory. I admit I hadn’t made the connection between the public image problem and the feature vs product model problem until not long ago — but now we can see that they are intertwined. Giving people a feature that has the functionality problems we’re seeing, and then upcharging them for it, is still a real problem for companies. Maybe when something just doesn’t work for a task, it’s neither a product nor a feature? If that turns out to be the case, then investors in generative AI will have a real problem on their hands, so companies are committing to generative AI aspects, whether they work well or not.
I’m going to be watching with great interest to see how things progress in this space. I do not expect any great leaps in generative AI functionality, although depending on how things turn out with DeepSeek, we may see some leaps in efficiency, at least in training. If companies listen to their people’ complaints and pivot, to target generative AI at the applications it’s actually useful for, they may have a enhanced chance of weathering the backlash, for enhanced or for worse. However, that to me seems highly, highly unlikely to be compatible with the desperate profit incentive they’re facing. Along the way, we’ll end up wasting tremendous resources on foolish uses of generative AI, instead of focusing our efforts on advancing the applications of the technology that are really worth the trade.
Gaining a competitive advantage from generative AI (Gen AI) is about implementing technology at the right time. Go too early a......
5 Essential Tips Learned from My Data Science Journey.
Ten years ago, I embarked on my journey in the field of data scien......
On Thursday, French lab Mistral AI launched Small 3, which the firm calls "the most efficient model of its category" and say......
My journey from DeepMind intern to mentor
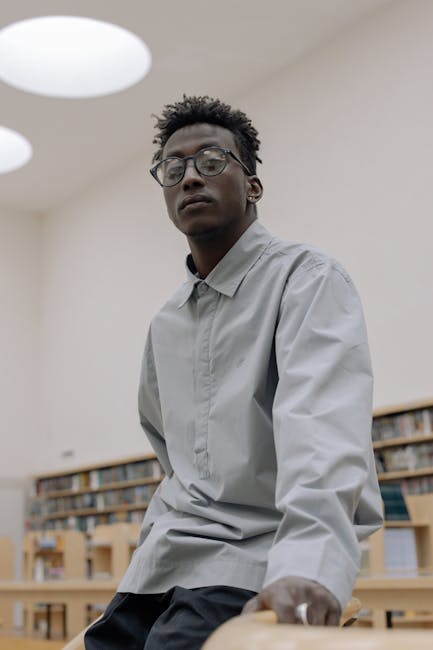
Former intern turned intern manager, Richard Everett, describes his journey to DeepMind, sharing tips and advice for aspiring DeepMinders. The 2023 internship applications will open on the 16th September, please visit [website] for more information.
Like many people, I loved playing multiplayer video games growing up. The interactions between human players and seemingly intelligent computer-controlled players fascinated me, and I dreamed about a career in AI. This dream led me to pursue an undergraduate degree in computer science; a common (but not exclusive!) pathway into the industry. However, after working on several research projects with my professors, I developed a taste for research and decided to continue on towards a PhD.
Around the time I started my PhD, a small startup called DeepMind was acquired by Google. As I looked closer at their research, I quickly found it inspiring my own research, and so in 2016 I decided to apply for an internship. After a handful of interviews with engineers, researchers, and program managers, I didn’t get an offer. However, having met a bunch of great researchers I decided to reapply the following year and got the internship. That experience led to a full-time offer and I’ve been here since, working on AI and helping interns who are going through the same experience.
Can you describe the internship interview process?
The interview process was thorough, but it’s evolved since I applied. Today's interns can expect the entire process to last just a few months, which includes a technical and a team interview. In my application, I listed the researchers that I was particularly interested in working with, and was lucky enough to speak with them after my technical interview. I was so excited. This was a unique opportunity to talk about my past work and brainstorm potential internship projects with world-class researchers I had followed for years, and ask them questions about DeepMind.
My recruiters were incredibly helpful in guiding me through the process and providing resources to help prepare for the interviews. For the technical interview, I prepared by revisiting my first-year undergraduate courses on mathematics, statistics, and computer science. For example, reviewing linear algebra, calculus, probability, algorithms, and data structures. I also practised some coding exercises where I tried to talk through what I was doing.
For the team interviews, I reviewed the team’s recent work ([website] papers, blog posts, articles, talks), and thought about how my work could relate to it. I also came up with a short list of questions I wanted to know more about, like the collaboration style of the team and how past internships had worked out.
Exploring examples of goal misgeneralisation – where an AI system's capabilities generalise but its goal doesn't.
Technologies Gemma Scope: helping the safety community shed light on the inner workings of language models Share.
corporation How our principles helped define AlphaFold’s release Share.
Reflections and lessons on sharing one of our biggest breakthro...
Market Impact Analysis
Market Growth Trend
2018 | 2019 | 2020 | 2021 | 2022 | 2023 | 2024 |
---|---|---|---|---|---|---|
23.1% | 27.8% | 29.2% | 32.4% | 34.2% | 35.2% | 35.6% |
Quarterly Growth Rate
Q1 2024 | Q2 2024 | Q3 2024 | Q4 2024 |
---|---|---|---|
32.5% | 34.8% | 36.2% | 35.6% |
Market Segments and Growth Drivers
Segment | Market Share | Growth Rate |
---|---|---|
Machine Learning | 29% | 38.4% |
Computer Vision | 18% | 35.7% |
Natural Language Processing | 24% | 41.5% |
Robotics | 15% | 22.3% |
Other AI Technologies | 14% | 31.8% |
Technology Maturity Curve
Different technologies within the ecosystem are at varying stages of maturity:
Competitive Landscape Analysis
Company | Market Share |
---|---|
Google AI | 18.3% |
Microsoft AI | 15.7% |
IBM Watson | 11.2% |
Amazon AI | 9.8% |
OpenAI | 8.4% |
Future Outlook and Predictions
The From Resume Cover landscape is evolving rapidly, driven by technological advancements, changing threat vectors, and shifting business requirements. Based on current trends and expert analyses, we can anticipate several significant developments across different time horizons:
Year-by-Year Technology Evolution
Based on current trajectory and expert analyses, we can project the following development timeline:
Technology Maturity Curve
Different technologies within the ecosystem are at varying stages of maturity, influencing adoption timelines and investment priorities:
Innovation Trigger
- Generative AI for specialized domains
- Blockchain for supply chain verification
Peak of Inflated Expectations
- Digital twins for business processes
- Quantum-resistant cryptography
Trough of Disillusionment
- Consumer AR/VR applications
- General-purpose blockchain
Slope of Enlightenment
- AI-driven analytics
- Edge computing
Plateau of Productivity
- Cloud infrastructure
- Mobile applications
Technology Evolution Timeline
- Improved generative models
- specialized AI applications
- AI-human collaboration systems
- multimodal AI platforms
- General AI capabilities
- AI-driven scientific breakthroughs
Expert Perspectives
Leading experts in the ai tech sector provide diverse perspectives on how the landscape will evolve over the coming years:
"The next frontier is AI systems that can reason across modalities and domains with minimal human guidance."
— AI Researcher
"Organizations that develop effective AI governance frameworks will gain competitive advantage."
— Industry Analyst
"The AI talent gap remains a critical barrier to implementation for most enterprises."
— Chief AI Officer
Areas of Expert Consensus
- Acceleration of Innovation: The pace of technological evolution will continue to increase
- Practical Integration: Focus will shift from proof-of-concept to operational deployment
- Human-Technology Partnership: Most effective implementations will optimize human-machine collaboration
- Regulatory Influence: Regulatory frameworks will increasingly shape technology development
Short-Term Outlook (1-2 Years)
In the immediate future, organizations will focus on implementing and optimizing currently available technologies to address pressing ai tech challenges:
- Improved generative models
- specialized AI applications
- enhanced AI ethics frameworks
These developments will be characterized by incremental improvements to existing frameworks rather than revolutionary changes, with emphasis on practical deployment and measurable outcomes.
Mid-Term Outlook (3-5 Years)
As technologies mature and organizations adapt, more substantial transformations will emerge in how security is approached and implemented:
- AI-human collaboration systems
- multimodal AI platforms
- democratized AI development
This period will see significant changes in security architecture and operational models, with increasing automation and integration between previously siloed security functions. Organizations will shift from reactive to proactive security postures.
Long-Term Outlook (5+ Years)
Looking further ahead, more fundamental shifts will reshape how cybersecurity is conceptualized and implemented across digital ecosystems:
- General AI capabilities
- AI-driven scientific breakthroughs
- new computing paradigms
These long-term developments will likely require significant technical breakthroughs, new regulatory frameworks, and evolution in how organizations approach security as a fundamental business function rather than a technical discipline.
Key Risk Factors and Uncertainties
Several critical factors could significantly impact the trajectory of ai tech evolution:
Organizations should monitor these factors closely and develop contingency strategies to mitigate potential negative impacts on technology implementation timelines.
Alternative Future Scenarios
The evolution of technology can follow different paths depending on various factors including regulatory developments, investment trends, technological breakthroughs, and market adoption. We analyze three potential scenarios:
Optimistic Scenario
Responsible AI driving innovation while minimizing societal disruption
Key Drivers: Supportive regulatory environment, significant research breakthroughs, strong market incentives, and rapid user adoption.
Probability: 25-30%
Base Case Scenario
Incremental adoption with mixed societal impacts and ongoing ethical challenges
Key Drivers: Balanced regulatory approach, steady technological progress, and selective implementation based on clear ROI.
Probability: 50-60%
Conservative Scenario
Technical and ethical barriers creating significant implementation challenges
Key Drivers: Restrictive regulations, technical limitations, implementation challenges, and risk-averse organizational cultures.
Probability: 15-20%
Scenario Comparison Matrix
Factor | Optimistic | Base Case | Conservative |
---|---|---|---|
Implementation Timeline | Accelerated | Steady | Delayed |
Market Adoption | Widespread | Selective | Limited |
Technology Evolution | Rapid | Progressive | Incremental |
Regulatory Environment | Supportive | Balanced | Restrictive |
Business Impact | Transformative | Significant | Modest |
Transformational Impact
Redefinition of knowledge work, automation of creative processes. This evolution will necessitate significant changes in organizational structures, talent development, and strategic planning processes.
The convergence of multiple technological trends—including artificial intelligence, quantum computing, and ubiquitous connectivity—will create both unprecedented security challenges and innovative defensive capabilities.
Implementation Challenges
Ethical concerns, computing resource limitations, talent shortages. Organizations will need to develop comprehensive change management strategies to successfully navigate these transitions.
Regulatory uncertainty, particularly around emerging technologies like AI in security applications, will require flexible security architectures that can adapt to evolving compliance requirements.
Key Innovations to Watch
Multimodal learning, resource-efficient AI, transparent decision systems. Organizations should monitor these developments closely to maintain competitive advantages and effective security postures.
Strategic investments in research partnerships, technology pilots, and talent development will position forward-thinking organizations to leverage these innovations early in their development cycle.
Technical Glossary
Key technical terms and definitions to help understand the technologies discussed in this article.
Understanding the following technical concepts is essential for grasping the full implications of the security threats and defensive measures discussed in this article. These definitions provide context for both technical and non-technical readers.