Gemma Scope: helping the safety community shed light on the inner workings of language models - Related to human, values, language, frontier, build
Gemma Scope: helping the safety community shed light on the inner workings of language models
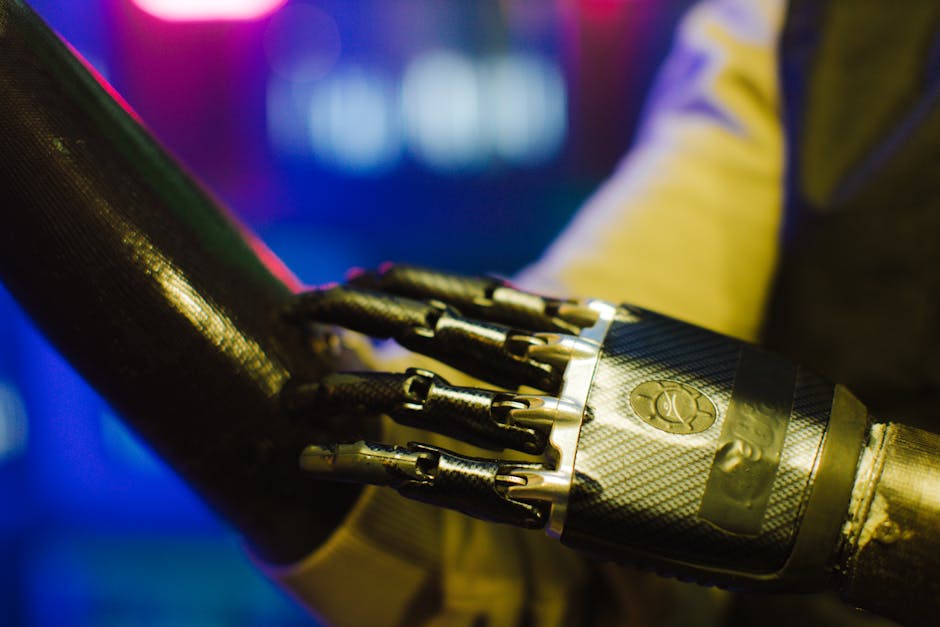
Technologies Gemma Scope: helping the safety community shed light on the inner workings of language models Share.
Announcing a comprehensive, open suite of sparse autoencoders for language model interpretability. To create an artificial intelligence (AI) language model, researchers build a system that learns from vast amounts of data without human guidance. As a result, the inner workings of language models are often a mystery, even to the researchers who train them. Mechanistic interpretability is a research field focused on deciphering these inner workings. Researchers in this field use sparse autoencoders as a kind of ‘microscope’ that lets them see inside a language model, and get a more effective sense of how it works. Today, we’re announcing Gemma Scope, a new set of tools to help researchers understand the inner workings of Gemma 2, our lightweight family of open models. Gemma Scope is a collection of hundreds of freely available, open sparse autoencoders (SAEs) for Gemma 2 9B and Gemma 2 2B. We're also open sourcing Mishax, a tool we built that enabled much of the interpretability work behind Gemma Scope. We hope today’s release enables more ambitious interpretability research. Further research has the potential to help the field build more robust systems, develop more effective safeguards against model hallucinations, and protect against risks from autonomous AI agents like deception or manipulation. Try our interactive Gemma Scope demo, courtesy of Neuronpedia.
Interpreting what happens inside a language model When you ask a language model a question, it turns your text input into a series of ‘activations’. These activations map the relationships between the words you’ve entered, helping the model make connections between different words, which it uses to write an answer. As the model processes text input, activations at different layers in the model’s neural network represent multiple increasingly advanced concepts, known as ‘aspects’. For example, a model’s early layers might learn to recall facts like that Michael Jordan plays basketball, while later layers may recognize more complex concepts like the factuality of the text.
Pause video Play video A stylised representation of using a sparse autoencoder to interpret a model’s activations as it recalls the fact that the City of Light is Paris. We see that French-related concepts are present, while unrelated ones are not.
However, interpretability researchers face a key problem: the model’s activations are a mixture of many different aspects. In the early days of mechanistic interpretability, researchers hoped that aspects in a neural network’s activations would line up with individual neurons, [website], nodes of information. But unfortunately, in practice, neurons are active for many unrelated aspects. This means that there is no obvious way to tell which aspects are part of the activation. This is where sparse autoencoders come in. A given activation will only be a mixture of a small number of aspects, even though the language model is likely capable of detecting millions or even billions of them – [website], the model uses aspects sparsely. For example, a language model will consider relativity when responding to an inquiry about Einstein and consider eggs when writing about omelettes, but probably won’t consider relativity when writing about omelettes. Sparse autoencoders leverage this fact to discover a set of possible aspects, and break down each activation into a small number of them. Researchers hope that the best way for the sparse autoencoder to accomplish this task is to find the actual underlying aspects that the language model uses. Importantly, at no point in this process do we - the researchers - tell the sparse autoencoder which aspects to look for. As a result, we are able to discover rich structures that we did not predict. However, because we don’t immediately know the meaning of the discovered aspects, we look for meaningful patterns in examples of text where the sparse autoencoder says the feature ‘fires’. Here’s an example in which the tokens where the feature fires are highlighted in gradients of blue :
Example activations for a feature found by our sparse autoencoders. Each bubble is a token (word or word fragment), and the variable blue color illustrates how strongly the feature is present. In this case, the feature is apparently related to idioms.
What makes Gemma Scope unique Prior research with sparse autoencoders has mainly focused on investigating the inner workings of tiny models or a single layer in larger models. But more ambitious interpretability research involves decoding layered, complex algorithms in larger models. We trained sparse autoencoders at every layer and sublayer output of Gemma 2 2B and 9B to build Gemma Scope, producing more than 400 sparse autoencoders with more than 30 million learned capabilities in total (though many capabilities likely overlap). This tool will enable researchers to study how capabilities evolve throughout the model and interact and compose to make more complex capabilities. Gemma Scope is also trained with our new, state-of-the-art JumpReLU SAE architecture. The original sparse autoencoder architecture struggled to balance the twin goals of detecting which capabilities are present, and estimating their strength. The JumpReLU architecture makes it easier to strike this balance appropriately, significantly reducing error. Training so many sparse autoencoders was a significant engineering challenge, requiring a lot of computing power. We used about 15% of the training compute of Gemma 2 9B (excluding compute for generating distillation labels), saved about 20 Pebibytes (PiB) of activations to disk (about as much as a million copies of English Wikipedia), and produced hundreds of billions of sparse autoencoder parameters in total.
Pushing the field forward In releasing Gemma Scope, we hope to make Gemma 2 the best model family for open mechanistic interpretability research and to accelerate the community’s work in this field. So far, the interpretability community has made great progress in understanding small models with sparse autoencoders and developing relevant techniques, like causal interventions, automatic circuit analysis, feature interpretation, and evaluating sparse autoencoders. With Gemma Scope, we hope to see the community scale these techniques to modern models, analyze more complex capabilities like chain-of-thought, and find real-world applications of interpretability such as tackling problems like hallucinations and jailbreaks that only arise with larger models.
Impact AlphaDev discovers faster sorting algorithms Share.
New algorithms will transform the foundations of computing Digital socie...
Research Shaping the future of advanced robotics Share.
Introducing AutoRT, SARA-RT and RT-Trajectory to improve real-world robot d...
Real-time communication is everywhere – live chatbots, data streams, or instant messaging. WebSockets are a powerful ena...
Updating the Frontier Safety Framework
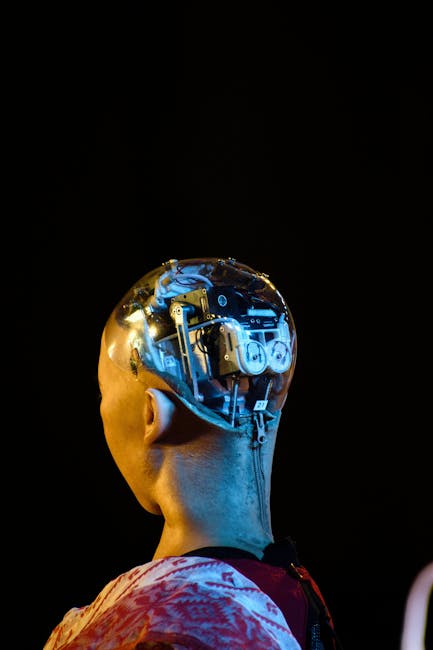
Responsibility & Safety Updating the Frontier Safety Framework Share.
Our next iteration of the FSF sets out stronger security protocols on the path to AGI AI is a powerful tool that is helping to unlock new breakthroughs and make significant progress on some of the biggest challenges of our time, from climate change to drug discovery. But as its development progresses, advanced capabilities may present new risks. That’s why we introduced the first iteration of our Frontier Safety Framework last year - a set of protocols to help us stay ahead of possible severe risks from powerful frontier AI models. Since then, we've collaborated with experts in industry, academia, and government to deepen our understanding of the risks, the empirical evaluations to test for them, and the mitigations we can apply. We have also implemented the Framework in our safety and governance processes for evaluating frontier models such as Gemini [website] As a result of this work, today we are publishing an updated Frontier Safety Framework. Key updates to the framework include: Security Level recommendations for our Critical Capability Levels (CCLs), helping to identify where the strongest efforts to curb exfiltration risk are needed.
Implementing a more consistent procedure for how we apply deployment mitigations.
Outlining an industry leading approach to deceptive alignment risk.
Recommendations for Heightened Security Security mitigations help prevent unauthorized actors from exfiltrating model weights. This is especially important because access to model weights allows removal of most safeguards. Given the stakes involved as we look ahead to increasingly powerful AI, getting this wrong could have serious implications for safety and security. Our initial Framework recognised the need for a tiered approach to security, allowing for the implementation of mitigations with varying strengths to be tailored to the risk. This proportionate approach also ensures we get the balance right between mitigating risks and fostering access and innovation. Since then, we have drawn on wider research to evolve these security mitigation levels and recommend a level for each of our CCLs.* These recommendations reflect our assessment of the minimum appropriate level of security the field of frontier AI should apply to such models at a CCL. This mapping process helps us isolate where the strongest mitigations are needed to curtail the greatest risk. In practice, some aspects of our security practices may exceed the baseline levels recommended here due to our strong overall security posture. This second version of the Framework recommends particularly high security levels for CCLs within the domain of machine learning research and development (R&D). We believe it will be important for frontier AI developers to have strong security for future scenarios when their models can significantly accelerate and/or automate AI development itself. This is because the uncontrolled proliferation of such capabilities could significantly challenge society’s ability to carefully manage and adapt to the rapid pace of AI development. Ensuring the continued security of cutting-edge AI systems is a shared global challenge - and a shared responsibility of all leading developers. Importantly, getting this right is a collective-action problem: the social value of any single actor’s security mitigations will be significantly reduced if not broadly applied across the field. Building the kind of security capabilities we believe may be needed will take time - so it’s vital that all frontier AI developers work collectively towards heightened security measures and accelerate efforts towards common industry standards.
Deployment Mitigations Procedure We also outline deployment mitigations in the Framework that focus on preventing the misuse of critical capabilities in systems we deploy. We’ve updated our deployment mitigation approach to apply a more rigorous safety mitigation process to models reaching a CCL in a misuse risk domain. The updated approach involves the following steps: first, we prepare a set of mitigations by iterating on a set of safeguards. As we do so, we will also develop a safety case, which is an assessable argument showing how severe risks associated with a model's CCLs have been minimised to an acceptable level. The appropriate corporate governance body then reviews the safety case, with general availability deployment occurring only if it is approved. Finally, we continue to review and improvement the safeguards and safety case after deployment. We’ve made this change because we believe that all critical capabilities warrant this thorough mitigation process. Approach to Deceptive Alignment Risk The first iteration of the Framework primarily focused on misuse risk ([website], the risks of threat actors using critical capabilities of deployed or exfiltrated models to cause harm). Building on this, we've taken an industry leading approach to proactively addressing the risks of deceptive alignment, [website] the risk of an autonomous system deliberately undermining human control. An initial approach to this question focuses on detecting when models might develop a baseline instrumental reasoning ability letting them undermine human control unless safeguards are in place. To mitigate this, we explore automated monitoring to detect illicit use of instrumental reasoning capabilities. We don’t expect automated monitoring to remain sufficient in the long-term if models reach even stronger levels of instrumental reasoning, so we’re actively undertaking – and strongly encouraging – further research developing mitigation approaches for these scenarios. While we don’t yet know how likely such capabilities are to arise, we think it is critical that the field prepares for the possibility. Conclusion We will continue to review and develop the Framework over time, guided by our AI Principles, which further outline our commitment to responsible development. As a part of our efforts, we’ll continue to work collaboratively with partners across society. For instance, if we assess that a model has reached a CCL that poses an unmitigated and material risk to overall public safety, we aim to share information with appropriate government authorities where it will facilitate the development of safe AI. Additionally, the latest Framework outlines a number of potential areas for further research – areas where we look forward to collaborating with the research community, other companies, and government. We believe an open, iterative, and collaborative approach will help to establish common standards and best practices for evaluating the safety of future AI models while securing their benefits for humanity. The Seoul Frontier AI Safety Commitments marked an critical step towards this collective effort - and we hope our updated Frontier Safety Framework contributes further to that progress. As we look ahead to AGI, getting this right will mean tackling very consequential questions - such as the right capability thresholds and mitigations - ones that will require the input of broader society, including governments.
Research Google DeepMind at ICLR 2024 Share.
Developing next-gen AI agents, exploring new modalities, and pioneering foundational l...
Detecting signs of this debilitating disease with AI before any bones start to break.
Melissa Formosa is an osteoporosis expert at the University of M...
Research FermiNet: Quantum physics and chemistry from first principles Share.
How can we build human values into AI?
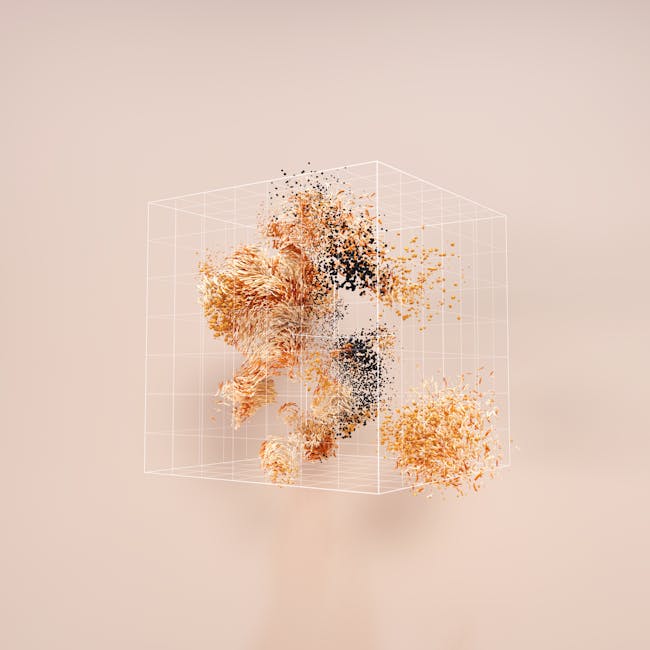
Responsibility & Safety How can we build human values into AI? Share.
Drawing from philosophy to identify fair principles for ethical AI As artificial intelligence (AI) becomes more powerful and more deeply integrated into our lives, the questions of how it is used and deployed are all the more key. What values guide AI? Whose values are they? And how are they selected? These questions shed light on the role played by principles – the foundational values that drive decisions big and small in AI. For humans, principles help shape the way we live our lives and our sense of right and wrong. For AI, they shape its approach to a range of decisions involving trade-offs, such as the choice between prioritising productivity or helping those most in need. In a paper , we draw inspiration from philosophy to find ways to improved identify principles to guide AI behaviour. Specifically, we explore how a concept known as the “veil of ignorance” – a thought experiment intended to help identify fair principles for group decisions – can be applied to AI. In our experiments, we found that this approach encouraged people to make decisions based on what they thought was fair, whether or not it benefited them directly. We also discovered that participants were more likely to select an AI that helped those who were most disadvantaged when they reasoned behind the veil of ignorance. These insights could help researchers and policymakers select principles for an AI assistant in a way that is fair to all parties.
The veil of ignorance (right) is a method of finding consensus on a decision when there are diverse opinions in a group (left).
A tool for fairer decision-making A key goal for AI researchers has been to align AI systems with human values. However, there is no consensus on a single set of human values or preferences to govern AI – we live in a world where people have diverse backgrounds, resources and beliefs. How should we select principles for this technology, given such diverse opinions? While this challenge emerged for AI over the past decade, the broad question of how to make fair decisions has a long philosophical lineage. In the 1970s, political philosopher John Rawls proposed the concept of the veil of ignorance as a solution to this problem. Rawls argued that when people select principles of justice for a society, they should imagine that they are doing so without knowledge of their own particular position in that society, including, for example, their social status or level of wealth. Without this information, people can’t make decisions in a self-interested way, and should instead choose principles that are fair to everyone involved. As an example, think about asking a friend to cut the cake at your birthday party. One way of ensuring that the slice sizes are fairly proportioned is not to tell them which slice will be theirs. This approach of withholding information is seemingly simple, but has wide applications across fields from psychology and politics to help people to reflect on their decisions from a less self-interested perspective. It has been used as a method to reach group agreement on contentious issues, ranging from sentencing to taxation. Building on this foundation, previous DeepMind research proposed that the impartial nature of the veil of ignorance may help promote fairness in the process of aligning AI systems with human values. We designed a series of experiments to test the effects of the veil of ignorance on the principles that people choose to guide an AI system. Maximise productivity or help the most disadvantaged? In an online ‘harvesting game’, we asked participants to play a group game with three computer players, where each player’s goal was to gather wood by harvesting trees in separate territories. In each group, some players were lucky, and were assigned to an advantaged position: trees densely populated their field, allowing them to efficiently gather wood. Other group members were disadvantaged: their fields were sparse, requiring more effort to collect trees. Each group was assisted by a single AI system that could spend time helping individual group members harvest trees. We asked participants to choose between two principles to guide the AI assistant’s behaviour. Under the “maximising principle” the AI assistant would aim to increase the harvest yield of the group by focusing predominantly on the denser fields. While under the “prioritising principle”the AI assistant would focus on helping disadvantaged group members.
An illustration of the ‘harvesting game’ where players (shown in red) either occupy a dense field that is easier to harvest (top two quadrants) or a sparse field that requires more effort to collect trees.
We placed half of the participants behind the veil of ignorance: they faced the choice between different ethical principles without knowing which field would be theirs – so they didn’t know how advantaged or disadvantaged they were. The remaining participants made the choice knowing whether they were superior or worse off. Encouraging fairness in decision making We found that if participants did not know their position, they consistently preferred the prioritising principle, where the AI assistant helped the disadvantaged group members. This pattern emerged consistently across all five different variations of the game, and crossed social and political boundaries: participants showed this tendency to choose the prioritising principle regardless of their appetite for risk or their political orientation. In contrast, participants who knew their own position were more likely to choose whichever principle benefitted them the most, whether that was the prioritising principle or the maximising principle.
A chart showing the effect of the veil of ignorance on the likelihood of choosing the prioritising principle, where the AI assistant would help those worse off. Participants who did not know their position were much more likely to support this principle to govern AI behaviour.
When we asked participants why they made their choice, those who did not know their position were especially likely to voice concerns about fairness. They frequently explained that it was right for the AI system to focus on helping people who were worse off in the group. In contrast, participants who knew their position much more frequently discussed their choice in terms of personal benefits. Lastly, after the harvesting game was over, we posed a hypothetical situation to participants: if they were to play the game again, this time knowing that they would be in a different field, would they choose the same principle as they did the first time? We were especially interested in individuals who previously benefited directly from their choice, but who would not benefit from the same choice in a new game. We found that people who had previously made choices without knowing their position were more likely to continue to endorse their principle – even when they knew it would no longer favour them in their new field. This provides additional evidence that the veil of ignorance encourages fairness in participants’ decision making, leading them to principles that they were willing to stand by even when they no longer benefitted from them directly. Fairer principles for AI AI technology is already having a profound effect on our lives. The principles that govern AI shape its impact and how these potential benefits will be distributed. Our research looked at a case where the effects of different principles were relatively clear. This will not always be the case: AI is deployed across a range of domains which often rely upon a large number of rules to guide them, potentially with complex side effects. Nonetheless, the veil of ignorance can still potentially inform principle selection, helping to ensure that the rules we choose are fair to all parties. To ensure we build AI systems that benefit everyone, we need extensive research with a wide range of inputs, approaches, and feedback from across disciplines and society. The veil of ignorance may provide a starting point for the selection of principles with which to align AI. It has been effectively deployed in other domains to bring out more impartial preferences. We hope that with further investigation and attention to context, it may help serve the same role for AI systems being built and deployed across society today and in the future. Read more about DeepMind’s approach to safety and ethics.
Technologies New generative AI tools open the doors of music creation Share.
Our latest AI music technologies are now available in ...
In December, we launched our first natively multimodal model Gemini [website] in three sizes: Ultra, Pro and Nano. Just a few months later we released [website] P...
In my professional life as a data scientist, I have encountered time series multiple times. Most of my knowledge comes from my academic experience, sp...
Market Impact Analysis
Market Growth Trend
2018 | 2019 | 2020 | 2021 | 2022 | 2023 | 2024 |
---|---|---|---|---|---|---|
23.1% | 27.8% | 29.2% | 32.4% | 34.2% | 35.2% | 35.6% |
Quarterly Growth Rate
Q1 2024 | Q2 2024 | Q3 2024 | Q4 2024 |
---|---|---|---|
32.5% | 34.8% | 36.2% | 35.6% |
Market Segments and Growth Drivers
Segment | Market Share | Growth Rate |
---|---|---|
Machine Learning | 29% | 38.4% |
Computer Vision | 18% | 35.7% |
Natural Language Processing | 24% | 41.5% |
Robotics | 15% | 22.3% |
Other AI Technologies | 14% | 31.8% |
Technology Maturity Curve
Different technologies within the ecosystem are at varying stages of maturity:
Competitive Landscape Analysis
Company | Market Share |
---|---|
Google AI | 18.3% |
Microsoft AI | 15.7% |
IBM Watson | 11.2% |
Amazon AI | 9.8% |
OpenAI | 8.4% |
Future Outlook and Predictions
The Safety Gemma Scope landscape is evolving rapidly, driven by technological advancements, changing threat vectors, and shifting business requirements. Based on current trends and expert analyses, we can anticipate several significant developments across different time horizons:
Year-by-Year Technology Evolution
Based on current trajectory and expert analyses, we can project the following development timeline:
Technology Maturity Curve
Different technologies within the ecosystem are at varying stages of maturity, influencing adoption timelines and investment priorities:
Innovation Trigger
- Generative AI for specialized domains
- Blockchain for supply chain verification
Peak of Inflated Expectations
- Digital twins for business processes
- Quantum-resistant cryptography
Trough of Disillusionment
- Consumer AR/VR applications
- General-purpose blockchain
Slope of Enlightenment
- AI-driven analytics
- Edge computing
Plateau of Productivity
- Cloud infrastructure
- Mobile applications
Technology Evolution Timeline
- Improved generative models
- specialized AI applications
- AI-human collaboration systems
- multimodal AI platforms
- General AI capabilities
- AI-driven scientific breakthroughs
Expert Perspectives
Leading experts in the ai tech sector provide diverse perspectives on how the landscape will evolve over the coming years:
"The next frontier is AI systems that can reason across modalities and domains with minimal human guidance."
— AI Researcher
"Organizations that develop effective AI governance frameworks will gain competitive advantage."
— Industry Analyst
"The AI talent gap remains a critical barrier to implementation for most enterprises."
— Chief AI Officer
Areas of Expert Consensus
- Acceleration of Innovation: The pace of technological evolution will continue to increase
- Practical Integration: Focus will shift from proof-of-concept to operational deployment
- Human-Technology Partnership: Most effective implementations will optimize human-machine collaboration
- Regulatory Influence: Regulatory frameworks will increasingly shape technology development
Short-Term Outlook (1-2 Years)
In the immediate future, organizations will focus on implementing and optimizing currently available technologies to address pressing ai tech challenges:
- Improved generative models
- specialized AI applications
- enhanced AI ethics frameworks
These developments will be characterized by incremental improvements to existing frameworks rather than revolutionary changes, with emphasis on practical deployment and measurable outcomes.
Mid-Term Outlook (3-5 Years)
As technologies mature and organizations adapt, more substantial transformations will emerge in how security is approached and implemented:
- AI-human collaboration systems
- multimodal AI platforms
- democratized AI development
This period will see significant changes in security architecture and operational models, with increasing automation and integration between previously siloed security functions. Organizations will shift from reactive to proactive security postures.
Long-Term Outlook (5+ Years)
Looking further ahead, more fundamental shifts will reshape how cybersecurity is conceptualized and implemented across digital ecosystems:
- General AI capabilities
- AI-driven scientific breakthroughs
- new computing paradigms
These long-term developments will likely require significant technical breakthroughs, new regulatory frameworks, and evolution in how organizations approach security as a fundamental business function rather than a technical discipline.
Key Risk Factors and Uncertainties
Several critical factors could significantly impact the trajectory of ai tech evolution:
Organizations should monitor these factors closely and develop contingency strategies to mitigate potential negative impacts on technology implementation timelines.
Alternative Future Scenarios
The evolution of technology can follow different paths depending on various factors including regulatory developments, investment trends, technological breakthroughs, and market adoption. We analyze three potential scenarios:
Optimistic Scenario
Responsible AI driving innovation while minimizing societal disruption
Key Drivers: Supportive regulatory environment, significant research breakthroughs, strong market incentives, and rapid user adoption.
Probability: 25-30%
Base Case Scenario
Incremental adoption with mixed societal impacts and ongoing ethical challenges
Key Drivers: Balanced regulatory approach, steady technological progress, and selective implementation based on clear ROI.
Probability: 50-60%
Conservative Scenario
Technical and ethical barriers creating significant implementation challenges
Key Drivers: Restrictive regulations, technical limitations, implementation challenges, and risk-averse organizational cultures.
Probability: 15-20%
Scenario Comparison Matrix
Factor | Optimistic | Base Case | Conservative |
---|---|---|---|
Implementation Timeline | Accelerated | Steady | Delayed |
Market Adoption | Widespread | Selective | Limited |
Technology Evolution | Rapid | Progressive | Incremental |
Regulatory Environment | Supportive | Balanced | Restrictive |
Business Impact | Transformative | Significant | Modest |
Transformational Impact
Redefinition of knowledge work, automation of creative processes. This evolution will necessitate significant changes in organizational structures, talent development, and strategic planning processes.
The convergence of multiple technological trends—including artificial intelligence, quantum computing, and ubiquitous connectivity—will create both unprecedented security challenges and innovative defensive capabilities.
Implementation Challenges
Ethical concerns, computing resource limitations, talent shortages. Organizations will need to develop comprehensive change management strategies to successfully navigate these transitions.
Regulatory uncertainty, particularly around emerging technologies like AI in security applications, will require flexible security architectures that can adapt to evolving compliance requirements.
Key Innovations to Watch
Multimodal learning, resource-efficient AI, transparent decision systems. Organizations should monitor these developments closely to maintain competitive advantages and effective security postures.
Strategic investments in research partnerships, technology pilots, and talent development will position forward-thinking organizations to leverage these innovations early in their development cycle.
Technical Glossary
Key technical terms and definitions to help understand the technologies discussed in this article.
Understanding the following technical concepts is essential for grasping the full implications of the security threats and defensive measures discussed in this article. These definitions provide context for both technical and non-technical readers.