Our latest advances in robot dexterity - Related to 2024, robot, latest, generating, our
Generating audio for video
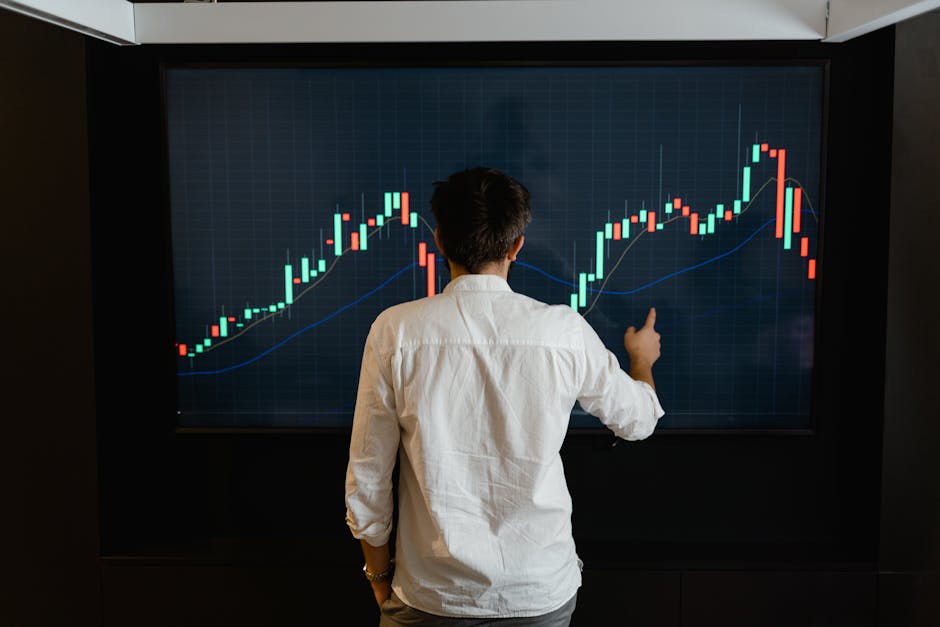
Technologies Generating audio for video Share.
Video-to-audio research uses video pixels and text prompts to generate rich soundtracks Video generation models are advancing at an incredible pace, but many current systems can only generate silent output. One of the next major steps toward bringing generated movies to life is creating soundtracks for these silent videos. Today, we're sharing progress on our video-to-audio (V2A) technology, which makes synchronized audiovisual generation possible. V2A combines video pixels with natural language text prompts to generate rich soundscapes for the on-screen action. Our V2A technology is pairable with video generation models like Veo to create shots with a dramatic score, realistic sound effects or dialogue that matches the characters and tone of a video. It can also generate soundtracks for a range of traditional footage, including archival material, silent films and more — opening a wider range of creative opportunities.
Watch Prompt for audio: Cinematic, thriller, horror film, music, tension, ambience, footsteps on concrete Watch Prompt for audio: Cute baby dinosaur chirps, jungle ambience, egg cracking Watch Prompt for audio: Jellyfish pulsating under water, marine life, ocean Watch Prompt for audio: A drummer on a stage at a concert surrounded by flashing lights and a cheering crowd Watch Prompt for audio: Cars skidding, car engine throttling, angelic electronic music Watch Prompt for audio: A slow mellow harmonica plays as the sun goes down on the prairie Watch Prompt for audio: Wolf howling at the moon.
Enhanced creative control Importantly, V2A can generate an unlimited number of soundtracks for any video input. Optionally, a ‘positive prompt’ can be defined to guide the generated output toward desired sounds, or a ‘negative prompt’ to guide it away from undesired sounds. This flexibility gives people more control over V2A’s audio output, making it possible to rapidly experiment with different audio outputs and choose the best match.
Watch Prompt for audio: A spaceship hurtles through the vastness of space, stars streaking past it, high speed, Sci-fi Watch Prompt for audio: Ethereal cello atmosphere Watch Prompt for audio: A spaceship hurtles through the vastness of space, stars streaking past it, high speed, Sci-fi.
How it works We experimented with autoregressive and diffusion approaches to discover the most scalable AI architecture, and the diffusion-based approach for audio generation gave the most realistic and compelling results for synchronizing video and audio information. Our V2A system starts by encoding video input into a compressed representation. Then, the diffusion model iteratively refines the audio from random noise. This process is guided by the visual input and natural language prompts given to generate synchronized, realistic audio that closely aligns with the prompt. Finally, the audio output is decoded, turned into an audio waveform and combined with the video data.
Diagram of our V2A system, taking video pixel and audio prompt input to generate an audio waveform synchronized to the underlying video. First, V2A encodes the video and audio prompt input and iteratively runs it through the diffusion model. Then it generates compressed audio, which is decoded into an audio waveform.
To generate higher quality audio and add the ability to guide the model towards generating specific sounds, we added more information to the training process, including AI-generated annotations with detailed descriptions of sound and transcripts of spoken dialogue. By training on video, audio and the additional annotations, our technology learns to associate specific audio events with various visual scenes, while responding to the information provided in the annotations or transcripts. Further research underway Our research stands out from existing video-to-audio solutions because it can understand raw pixels and adding a text prompt is optional. Also, the system doesn't need manual alignment of the generated sound with the video, which involves tediously adjusting different elements of sounds, visuals and timings.
Still, there are a number of other limitations we’re trying to address and further research is underway. Since the quality of the audio output is dependent on the quality of the video input, artifacts or distortions in the video, which are outside the model’s training distribution, can lead to a noticeable drop in audio quality. We’re also improving lip synchronization for videos that involve speech. V2A attempts to generate speech from the input transcripts and synchronize it with characters' lip movements. But the paired video generation model may not be conditioned on transcripts. This creates a mismatch, often resulting in uncanny lip-syncing, as the video model doesn’t generate mouth movements that match the transcript.
Watch Prompt for audio: Music, Transcript: “this turkey looks amazing, I’m so hungry”.
Our commitment to safety and transparency We’re committed to developing and deploying AI technologies responsibly. To make sure our V2A technology can have a positive impact on the creative community, we’re gathering diverse perspectives and insights from leading creators and filmmakers, and using this valuable feedback to inform our ongoing research and development. We’ve also incorporated our SynthID toolkit into our V2A research to watermark all AI-generated content to help safeguard against the potential for misuse of this technology. Before we consider opening access to it to the wider public, our V2A technology will undergo rigorous safety assessments and testing. Initial results are showing this technology will become a promising approach for bringing generated movies to life. Note: All examples are generated by our V2A technology, which is paired with Veo, our most capable generative video model.
Research A glimpse of the next generation of AlphaFold Share.
Progress upgrade: Our latest AlphaFold model displays significantly impro...
Impact Google Cloud: Driving digital transformation Share.
Google Cloud empowers organizations to digitally transform themselves in...
firm Realising scientists are the real superheroes Share.
Meet Edgar Duéñez-Guzmán, a research engineer on our Multi-Agent Resea...
Our latest advances in robot dexterity
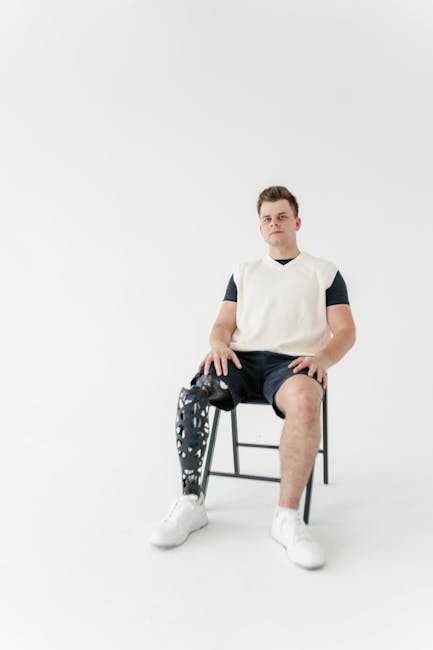
Research Our latest advances in robot dexterity Share.
Two new AI systems, ALOHA Unleashed and DemoStart, help robots learn to perform complex tasks that require dexterous movement People perform many tasks on a daily basis, like tying shoelaces or tightening a screw. But for robots, learning these highly-dexterous tasks is incredibly difficult to get right. To make robots more useful in people’s lives, they need to get more effective at making contact with physical objects in dynamic environments. Today, we introduce two new papers featuring our latest artificial intelligence (AI) advances in robot dexterity research: ALOHA Unleashed which helps robots learn to perform complex and novel two-armed manipulation tasks; and DemoStart which uses simulations to improve real-world performance on a multi-fingered robotic hand. By helping robots learn from human demonstrations and translate images to action, these systems are paving the way for robots that can perform a wide variety of helpful tasks.
Improving imitation learning with two robotic arms Until now, most advanced AI robots have only been able to pick up and place objects using a single arm. In our new paper, we present ALOHA Unleashed, which achieves a high level of dexterity in bi-arm manipulation. With this new method, our robot learned to tie a shoelace, hang a shirt, repair another robot, insert a gear and even clean a kitchen.
Pause video Play video Example of a bi-arm robot straightening shoe laces and tying them into a bow.
Pause video Play video Example of a bi-arm robot laying out a polo shirt on a table, putting it on a clothes hanger and then hanging it on a rack.
Pause video Play video Example of a bi-arm robot repairing another robot.
The ALOHA Unleashed method builds on our ALOHA 2 platform that was based on the original ALOHA (a low-cost open-source hardware system for bimanual teleoperation) from Stanford University. ALOHA 2 is significantly more dexterous than prior systems because it has two hands that can be easily teleoperated for training and data collection purposes, and it allows robots to learn how to perform new tasks with fewer demonstrations. We’ve also improved upon the robotic hardware’s ergonomics and enhanced the learning process in our latest system. First, we collected demonstration data by remotely operating the robot’s behavior, performing difficult tasks like tying shoelaces and hanging t-shirts. Next, we applied a diffusion method, predicting robot actions from random noise, similar to how our Imagen model generates images. This helps the robot learn from the data, so it can perform the same tasks on its own.
Learning robotic behaviors from few simulated demonstrations Controlling a dexterous, robotic hand is a complex task, which becomes even more complex with every additional finger, joint and sensor. In another new paper, we present DemoStart, which uses a reinforcement learning algorithm to help robots acquire dexterous behaviors in simulation. These learned behaviors are especially useful for complex embodiments, like multi-fingered hands. DemoStart first learns from easy states, and over time, starts learning from more difficult states until it masters a task to the best of its ability. It requires 100x fewer simulated demonstrations to learn how to solve a task in simulation than what’s usually needed when learning from real world examples for the same purpose. The robot achieved a success rate of over 98% on a number of different tasks in simulation, including reorienting cubes with a certain color showing, tightening a nut and bolt, and tidying up tools. In the real-world setup, it achieved a 97% success rate on cube reorientation and lifting, and 64% at a plug-socket insertion task that required high-finger coordination and precision.
Pause video Play video Example of a robotic arm learning to successfully insert a yellow connector in simulation (left) and in a real-world setup (right).
Pause video Play video Example of a robotic arm learning to tighten a bolt on a screw in simulation.
We developed DemoStart with MuJoCo, our open-source physics simulator. After mastering a range of tasks in simulation and using standard techniques to reduce the sim-to-real gap, like domain randomization, our approach was able to transfer nearly zero-shot to the physical world. Robotic learning in simulation can reduce the cost and time needed to run actual, physical experiments. But it’s difficult to design these simulations, and moreover, they don’t always translate successfully back into real-world performance. By combining reinforcement learning with learning from a few demonstrations, DemoStart’s progressive learning automatically generates a curriculum that bridges the sim-to-real gap, making it easier to transfer knowledge from a simulation into a physical robot, and reducing the cost and time needed for running physical experiments. To enable more advanced robot learning through intensive experimentation, we tested this new approach on a three-fingered robotic hand, called DEX-EE, which was developed in collaboration with Shadow Robot.
Image of the DEX-EE dexterous robotic hand, developed by Shadow Robot, in collaboration with the Google DeepMind robotics team (Credit: Shadow Robot).
The future of robot dexterity Robotics is a unique area of AI research that exhibits how well our approaches work in the real world. For example, a large language model could tell you how to tighten a bolt or tie your shoes, but even if it was embodied in a robot, it wouldn’t be able to perform those tasks itself. One day, AI robots will help people with all kinds of tasks at home, in the workplace and more. Dexterity research, including the efficient and general learning approaches we’ve described today, will help make that future possible. We still have a long way to go before robots can grasp and handle objects with the ease and precision of people, but we’re making significant progress, and each groundbreaking innovation is another step in the right direction.
Former intern turned intern manager, Richard Everett, describes his journey to DeepMind, sharing tips and advice for aspiring DeepMinders. The 2023 in...
Research Google DeepMind at NeurIPS 2024 Share.
Building adaptive, smart, and safe AI Agents LLM-based AI agents are showing promis...
How summits in Seoul, France and beyond can galvanize international cooperation on frontier AI safety.
Last year, the UK Government hosted the first m...
Google DeepMind at NeurIPS 2024
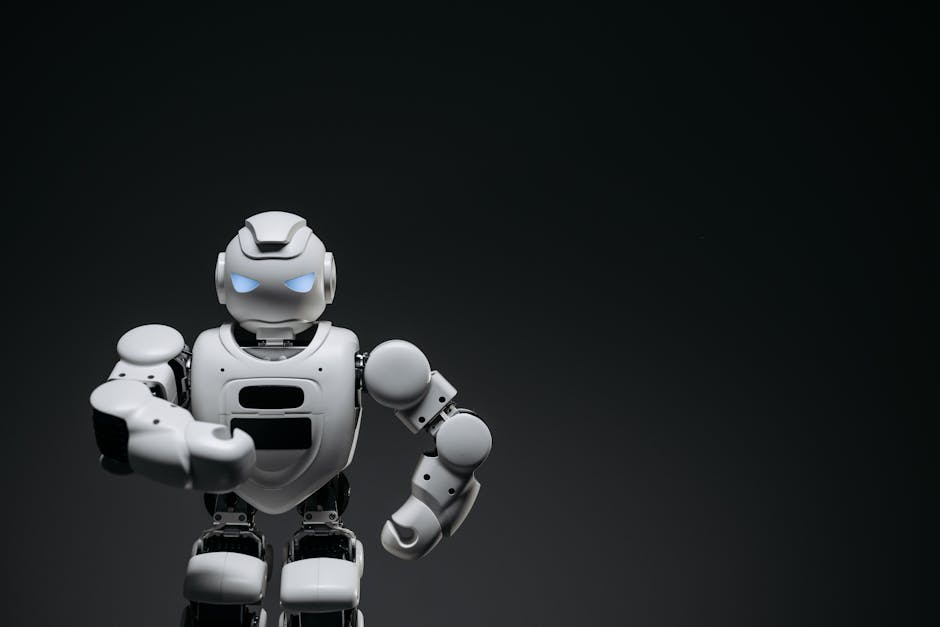
Research Google DeepMind at NeurIPS 2024 Share.
Building adaptive, smart, and safe AI Agents LLM-based AI agents are showing promise in carrying out digital tasks via natural language commands. Yet their success depends on precise interaction with complex user interfaces, which requires extensive training data. With AndroidControl, we share the most diverse control dataset to date, with over 15,000 human-collected demos across more than 800 apps. AI agents trained using this dataset showed significant performance gains which we hope helps advance research into more general AI agents. For AI agents to generalize across tasks, they need to learn from each experience they encounter. We present a method for in-context abstraction learning that helps agents grasp key task patterns and relationships from imperfect demos and natural language feedback, enhancing their performance and adaptability.
A frame from a video demonstration of someone making a sauce, with individual elements identified and numbered. ICAL is able to extract the critical aspects of the process.
Developing agentic AI that works to fulfill individuals’ goals can help make the technology more useful, but alignment is critical when developing AI that acts on our behalf. To that end, we propose a theoretical method to measure an AI system’s goal-directedness, and also show how a model’s perception of its user can influence its safety filters. Together, these insights underscore the importance of robust safeguards to prevent unintended or unsafe behaviors, ensuring that AI agents’ actions remain aligned with safe, intended uses.
Advancing 3D scene creation and simulation As demand for high-quality 3D content grows across industries like gaming and visual effects, creating lifelike 3D scenes remains costly and time-intensive. Our recent work introduces novel 3D generation, simulation, and control approaches, streamlining content creation for faster, more flexible workflows. Producing high-quality, realistic 3D assets and scenes often requires capturing and modeling thousands of 2D photos. We showcase CAT3D, a system that can create 3D content in as little as a minute, from any number of images — even just one image, or a text prompt. CAT3D accomplishes this with a multi-view diffusion model that generates additional consistent 2D images from many different viewpoints, and uses those generated images as input for traditional 3D modelling techniques. Results surpass previous methods in both speed and quality.
CAT3D enables 3D scene creation from any number of generated or real images. Left to right: Text-to-image-to-3D, a real photo to 3D, several photos to 3D.
Simulating scenes with many rigid objects, like a cluttered tabletop or tumbling Lego bricks, also remains computationally intensive. To overcome this roadblock, we present a new technique called SDF-Sim that represents object shapes in a scalable way, speeding up collision detection and enabling efficient simulation of large, complex scenes.
A complex simulation of shoes falling and colliding, accurately modelled using SDF-Sim.
AI image generators based on diffusion models struggle to control the 3D position and orientation of multiple objects. Our solution, Neural Assets, introduces object-specific representations that capture both appearance and 3D pose, learned through training on dynamic video data. Neural Assets enables individuals to move, rotate, or swap objects across scenes—a useful tool for animation, gaming, and virtual reality.
Given a source image and object 3D bounding boxes, we can translate, rotate, and rescale the object, or transfer objects or backgrounds between images.
Responsibility & Safety The ethics of advanced AI assistants Share.
Exploring the promise and risks of a future with more capable A...
This morning, Co-founder and CEO of Google DeepMind and Isomorphic Labs Sir Demis Hassabis, and Google DeepMind Director Dr. John Jumper were co-award...
Impact AlphaFold unlocks one of the greatest puzzles in biology Share.
AI system helps researchers piece together one of the larges...
Market Impact Analysis
Market Growth Trend
2018 | 2019 | 2020 | 2021 | 2022 | 2023 | 2024 |
---|---|---|---|---|---|---|
23.1% | 27.8% | 29.2% | 32.4% | 34.2% | 35.2% | 35.6% |
Quarterly Growth Rate
Q1 2024 | Q2 2024 | Q3 2024 | Q4 2024 |
---|---|---|---|
32.5% | 34.8% | 36.2% | 35.6% |
Market Segments and Growth Drivers
Segment | Market Share | Growth Rate |
---|---|---|
Machine Learning | 29% | 38.4% |
Computer Vision | 18% | 35.7% |
Natural Language Processing | 24% | 41.5% |
Robotics | 15% | 22.3% |
Other AI Technologies | 14% | 31.8% |
Technology Maturity Curve
Different technologies within the ecosystem are at varying stages of maturity:
Competitive Landscape Analysis
Company | Market Share |
---|---|
Google AI | 18.3% |
Microsoft AI | 15.7% |
IBM Watson | 11.2% |
Amazon AI | 9.8% |
OpenAI | 8.4% |
Future Outlook and Predictions
The Generating Audio Video landscape is evolving rapidly, driven by technological advancements, changing threat vectors, and shifting business requirements. Based on current trends and expert analyses, we can anticipate several significant developments across different time horizons:
Year-by-Year Technology Evolution
Based on current trajectory and expert analyses, we can project the following development timeline:
Technology Maturity Curve
Different technologies within the ecosystem are at varying stages of maturity, influencing adoption timelines and investment priorities:
Innovation Trigger
- Generative AI for specialized domains
- Blockchain for supply chain verification
Peak of Inflated Expectations
- Digital twins for business processes
- Quantum-resistant cryptography
Trough of Disillusionment
- Consumer AR/VR applications
- General-purpose blockchain
Slope of Enlightenment
- AI-driven analytics
- Edge computing
Plateau of Productivity
- Cloud infrastructure
- Mobile applications
Technology Evolution Timeline
- Improved generative models
- specialized AI applications
- AI-human collaboration systems
- multimodal AI platforms
- General AI capabilities
- AI-driven scientific breakthroughs
Expert Perspectives
Leading experts in the ai tech sector provide diverse perspectives on how the landscape will evolve over the coming years:
"The next frontier is AI systems that can reason across modalities and domains with minimal human guidance."
— AI Researcher
"Organizations that develop effective AI governance frameworks will gain competitive advantage."
— Industry Analyst
"The AI talent gap remains a critical barrier to implementation for most enterprises."
— Chief AI Officer
Areas of Expert Consensus
- Acceleration of Innovation: The pace of technological evolution will continue to increase
- Practical Integration: Focus will shift from proof-of-concept to operational deployment
- Human-Technology Partnership: Most effective implementations will optimize human-machine collaboration
- Regulatory Influence: Regulatory frameworks will increasingly shape technology development
Short-Term Outlook (1-2 Years)
In the immediate future, organizations will focus on implementing and optimizing currently available technologies to address pressing ai tech challenges:
- Improved generative models
- specialized AI applications
- enhanced AI ethics frameworks
These developments will be characterized by incremental improvements to existing frameworks rather than revolutionary changes, with emphasis on practical deployment and measurable outcomes.
Mid-Term Outlook (3-5 Years)
As technologies mature and organizations adapt, more substantial transformations will emerge in how security is approached and implemented:
- AI-human collaboration systems
- multimodal AI platforms
- democratized AI development
This period will see significant changes in security architecture and operational models, with increasing automation and integration between previously siloed security functions. Organizations will shift from reactive to proactive security postures.
Long-Term Outlook (5+ Years)
Looking further ahead, more fundamental shifts will reshape how cybersecurity is conceptualized and implemented across digital ecosystems:
- General AI capabilities
- AI-driven scientific breakthroughs
- new computing paradigms
These long-term developments will likely require significant technical breakthroughs, new regulatory frameworks, and evolution in how organizations approach security as a fundamental business function rather than a technical discipline.
Key Risk Factors and Uncertainties
Several critical factors could significantly impact the trajectory of ai tech evolution:
Organizations should monitor these factors closely and develop contingency strategies to mitigate potential negative impacts on technology implementation timelines.
Alternative Future Scenarios
The evolution of technology can follow different paths depending on various factors including regulatory developments, investment trends, technological breakthroughs, and market adoption. We analyze three potential scenarios:
Optimistic Scenario
Responsible AI driving innovation while minimizing societal disruption
Key Drivers: Supportive regulatory environment, significant research breakthroughs, strong market incentives, and rapid user adoption.
Probability: 25-30%
Base Case Scenario
Incremental adoption with mixed societal impacts and ongoing ethical challenges
Key Drivers: Balanced regulatory approach, steady technological progress, and selective implementation based on clear ROI.
Probability: 50-60%
Conservative Scenario
Technical and ethical barriers creating significant implementation challenges
Key Drivers: Restrictive regulations, technical limitations, implementation challenges, and risk-averse organizational cultures.
Probability: 15-20%
Scenario Comparison Matrix
Factor | Optimistic | Base Case | Conservative |
---|---|---|---|
Implementation Timeline | Accelerated | Steady | Delayed |
Market Adoption | Widespread | Selective | Limited |
Technology Evolution | Rapid | Progressive | Incremental |
Regulatory Environment | Supportive | Balanced | Restrictive |
Business Impact | Transformative | Significant | Modest |
Transformational Impact
Redefinition of knowledge work, automation of creative processes. This evolution will necessitate significant changes in organizational structures, talent development, and strategic planning processes.
The convergence of multiple technological trends—including artificial intelligence, quantum computing, and ubiquitous connectivity—will create both unprecedented security challenges and innovative defensive capabilities.
Implementation Challenges
Ethical concerns, computing resource limitations, talent shortages. Organizations will need to develop comprehensive change management strategies to successfully navigate these transitions.
Regulatory uncertainty, particularly around emerging technologies like AI in security applications, will require flexible security architectures that can adapt to evolving compliance requirements.
Key Innovations to Watch
Multimodal learning, resource-efficient AI, transparent decision systems. Organizations should monitor these developments closely to maintain competitive advantages and effective security postures.
Strategic investments in research partnerships, technology pilots, and talent development will position forward-thinking organizations to leverage these innovations early in their development cycle.
Technical Glossary
Key technical terms and definitions to help understand the technologies discussed in this article.
Understanding the following technical concepts is essential for grasping the full implications of the security threats and defensive measures discussed in this article. These definitions provide context for both technical and non-technical readers.