A new generation of African talent brings cutting-edge AI to scientific challenges - Related to future, a, transforming, talent, alphachip
A new generation of African talent brings cutting-edge AI to scientific challenges
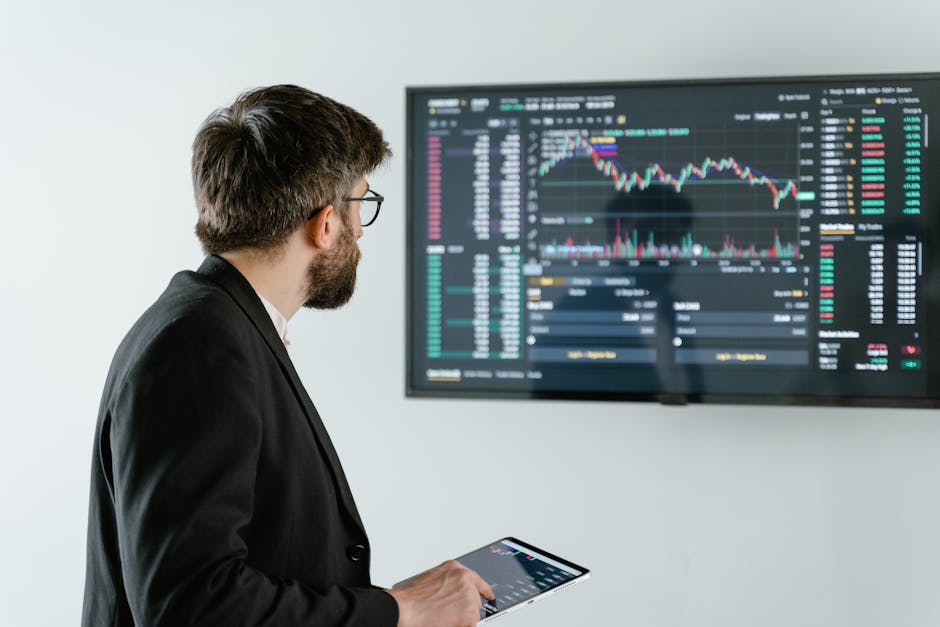
corporation A new generation of African talent brings cutting-edge AI to scientific challenges Share.
Food security, healthcare and exploring the cosmos are among the ways students of a new pan-African Master’s program aspire to apply AI At Google DeepMind, we’re committed to supporting the next generation of artificial intelligence (AI) leaders to help build a stronger, more diverse and inclusive global AI community. This includes increasing access to AI and science through education. Last year, we partnered with the African Institute for Mathematical Sciences (AIMS), Africa’s first network of centers of excellence in mathematical sciences, to launch an AI for Science Master’s program, with a $[website] grant from Google DeepMind. This funding helps AIMS provide full scholarships, equipment and compute to talented local students, giving them access to advanced studies in mathematics, AI and machine learning from world-class academics at AIMS South Africa. Students have the opportunity to accelerate scientific discovery, with mentoring and support from Google DeepMind’s researchers and engineers.
This summer, the first cohort of students graduated at a ceremony at the AIMS campus in Cape Town, South Africa. As the next generation of AI leaders in Africa, Béria Chingnabé Kalpélbé, Olivier Mahumawon Adjagba and Diffo Mboudjiho Annette Dariose shared their experiences in pioneering AI research and what they’re hoping to achieve with their work.
Béria Chingnabé Kalpélbé is passionate about applying AI to sustainability challenges.
Béria: Innovating for more effective food security Sustainability is a top priority for Béria, originally from Chad. “I hope to develop solutions for sustainable agricultural development that will benefit both people and the planet by integrating principles of renewable energy, precision farming, and ecological preservation in my work,” he says. “Beyond agriculture, AI offers significant potential to enhance the resilience of Africa's natural environments,” Béria adds. “By implementing AI-powered monitoring and decision-support systems, we can safeguard Africa's precious green areas and biodiversity for future generations.”.
Olivier Mahumawon Adjagba wants to use AI to create more accurate prediction models for the spread of dengue fever.
Olivier: Pioneering virus transmission research through the lens of climate change Olivier’s passion for applying mathematics to complex problems led him to AIMS South Africa: “Throughout my academic journey, I’ve been fascinated by the power of mathematics, particularly in addressing real-world challenges through AI,” he says. “A solid foundation in mathematical sciences is crucial for driving progress in areas such as healthcare, climate science and technology — and I’m eager to be at the forefront of these advancements.” Originally from Benin, Olivier now looks to apply this approach to data from African countries to help understand the spread of dengue fever. “Using advanced AI techniques, I hope to create more accurate prediction models to inform public health strategies and interventions, ultimately contributing to the control and prevention of this viral disease.” Discussing the personal impact of his scholarship, Olivier recounts, “Without it, pursuing advanced studies at such a prestigious institution would have been financially unattainable for me. This support enabled me to fully immerse myself in AIMS' rigorous academic environment, so I could engage deeply in coursework, collaborate with professors and peers, and contribute meaningfully to research projects.”.
Diffo Mboudjiho Annette Dariose hopes to learn more about our universe with the help of AI.
Diffo: Unraveling the secrets of our universe Diffo, from Cameroon, is fascinated by the big questions beyond Earth — which is what drew her to the Square Kilometre Array (SKA), the largest and most sensitive radio telescope on the planet. “Understanding the 21cm line provides insights into the early universe, the formation of the first stars and galaxies, and the structure of the cosmos,” Diffo explains. “By applying Markov chain Monte Carlo (MCMC) techniques, I hope to improve the accuracy and efficiency of extracting these faint signals from SKA data, potentially leading to more precise cosmological models and a deeper understanding of the future evolution of the universe.” For those considering similar studies, Diffo offers a few words of advice: “Stay curious, be persistent and embrace interdisciplinary learning. Engaging in hands-on projects, collaborating with peers, and seeking mentorship from AI experts can greatly benefit your learning experience and career prospects.”.
Supporting AI education in Africa This work builds on our existing commitments in the region, including our support of the Deep Learning Indaba through volunteering and funding since its inception in 2017, the recent launch of our Experience AI education program across Africa, which has already engaged local educators working with more than 30,000 young people, and additional educational funding, which has been used by three further African universities to offer a total of over 40 postgraduate scholarships since 2020. Increasing representation in the field of AI research offers a much-needed opportunity to bring diverse values, perspectives, and concerns into conversations about the design and deployment of this transformative technology. We hope our support for AIMS not only serves to build a more global and inclusive AI ecosystem, but also helps students make new scientific discoveries that benefit their local communities and the entire globe.
Learn more AIMS AI for Science Masters program.
Research Discovering when an agent is present in a system Share.
New, formal definition of agency gives clear principles for causal...
Responsibility & Safety An early warning system for novel AI risks Share.
New research proposes a framework for evaluating general-...
Detecting signs of this debilitating disease with AI before any bones start to break.
Melissa Formosa is an osteoporosis expert at the University of M...
Transforming the future of music creation
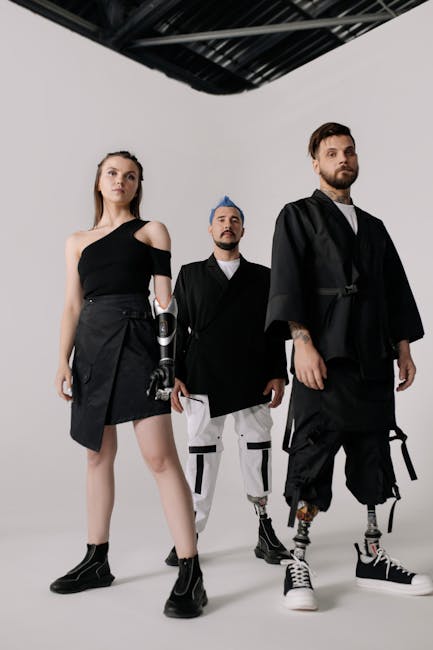
Technologies Transforming the future of music creation Share.
Announcing our most advanced music generation model, and two new AI experiments designed to open a new playground for creativity From jazz to heavy metal, techno to opera, music is a much loved form of creative expression. With complex and densely layered lyrics, melodies, rhythms, and vocals, creating music that’s compelling has been especially challenging for artificial intelligence (AI) systems — until now. Today, in partnership with YouTube, we’re announcing Google DeepMind’s Lyria, our most advanced AI music generation model to date, and two AI experiments designed to open a new playground for creativity: Dream Track – an experiment in YouTube Shorts designed to help deepen connections between artists, creators, and fans through music creation.
– an experiment in YouTube Shorts designed to help deepen connections between artists, creators, and fans through music creation. Music AI tools – a set of tools we’re designing with artists, songwriters, and producers to help bolster their creative processes. To develop these projects, we’ve brought together technical experts from across Google with a diverse range of world-renowned artists and songwriters to explore how generative music technologies can responsibly shape the future of music creation. We’re excited about building new technologies that can enhance the work of professional musicians and the artist community, and deliver a positive contribution to the future of music.
Watch An early look at the possibilities as we experiment with AI and music.
Introducing the Lyria model Music contains huge amounts of information — consider every beat, note, and vocal harmony in every second. When generating long sequences of sound, it’s difficult for AI models to maintain musical continuity across phrases, verses, or extended passages. Since music often includes multiple voices and instruments at the same time, it's much harder to create than speech. Built by Google DeepMind, the Lyria model excels at generating high-quality music with instrumentals and vocals, performing transformation and continuation tasks, and giving clients more nuanced control of the output’s style and performance.
Inspiring new music on YouTube Shorts We’re trialing Lyria in an experiment called Dream Track, which is designed to test new ways for artists to connect with their fans and developed in collaboration with YouTube. Within the experiment, a limited set of creators will be able to use Dream Track for producing a unique soundtrack with the AI-generated voice and musical style of artists including Alec Benjamin, Charlie Puth, Charli XCX, Demi Lovato, John Legend, Sia, T-Pain, Troye Sivan, and Papoose. Each participating artist has partnered with us and will have a hand in helping us test and learn to shape the future of AI in music. Dream Track consumers can simply enter a topic and choose an artist from the carousel to generate a 30 second soundtrack for their Short. Using our Lyria model, Dream Track simultaneously generates the lyrics, backing track, and AI-generated voice in the style of the participating artist selected. Here are a couple of samples generated in the styles of Charlie Puth or T-Pain:
Watch Dream Track audio sample with the AI-generated voice and style of artist Charlie Puth. Watch Dream Track audio sample with the AI-generated voice and style of artist T-Pain.
Exploring music AI tools with the industry Our researchers have been exploring with artists, songwriters, and producers in YouTube’s Music AI Incubator how generative AI can best support the creative process, and working together to responsibly design a suite of music AI tools. Imagine singing a melody to create a horn line, transforming chords from a MIDI keyboard into a realistic vocal choir, or adding an instrumental accompaniment to a vocal track.
Watch Louis Bell, Producer/Songwriter, builds a track with just a hum.
With our music AI tools, individuals can create new music or instrumental sections from scratch, transform audio from one music style or instrument to another, and create instrumental and vocal accompaniments. This work draws on our history of research and experimentation with AI and music, and we’ll continue testing our music AI tools with incubator participants throughout their development.
Example user interface of our music AI tools.
Watch Transforming beatboxing into a drum loop. Watch Transforming singing into an orchestral score. Watch Transforming MIDI keyboard chords into a vocal choir.
Watermarking AI-generated audio with SynthID Our team is also pioneering responsible deployment of our technologies with best-in-class tools for watermarking and identifying synthetically generated content. Any content , the same technology toolkit we’re using for identifying images generated by Imagen on Google Cloud’s Vertex AI.
SynthID converts audio into a visual spectrogram to add a digital watermark.
SynthID embeds a watermark into AI-generated audio content that’s inaudible to the human ear and doesn’t compromise the listening experience. It does this by converting the audio wave into a two-dimensional visualization that exhibits how the spectrum of frequencies in a sound evolves over time. This novel method is unlike anything that exists today, especially in the context of audio. The watermark is designed to maintain detectability even when the audio content undergoes many common modifications such as noise additions, MP3 compression, or speeding up and slowing down the track. SynthID can also detect the presence of a watermark throughout a track to help determine if parts of a song were generated by Lyria.
Developing and deploying our technologies responsibly To maximize the benefits of our generative music technologies, while mitigating potential risks, it’s critical these are developed with best-in-class protections. We’ve worked closely with artists and the music industry to ensure these technologies are widely beneficial. Our music AI experiments have been designed in line with YouTube’s AI principles, which aim to enable creative expression while protecting music artists and the integrity of their work. Going forward, we’ll continue engaging artists, the music industry, and wider creative community to set the standard for the responsible development and deployment of music generation tools.
The future of generative music tools Generative music technologies could transform the future of music creation and use. Our cutting-edge work in this space will unlock an exciting new wave of artist tools that can inspire creativity for artists, songwriters, producers, and fans everywhere. We've only just begun to explore how AI can bolster people's musical creativity and we can't wait to see what we can accomplish next in partnership with artists, the music industry, and wider creative community.
enterprise How our principles helped define AlphaFold’s release Share.
Reflections and lessons on sharing one of our biggest breakthro...
What's more effective than an AI chatbot that can assist you with tasks? One that can do them for you. OpenAI continues to build out its AI agents in ...
Technologies Pushing the frontiers of audio generation Share.
Our pioneering speech generation technologies are helping people arou...
How AlphaChip transformed computer chip design
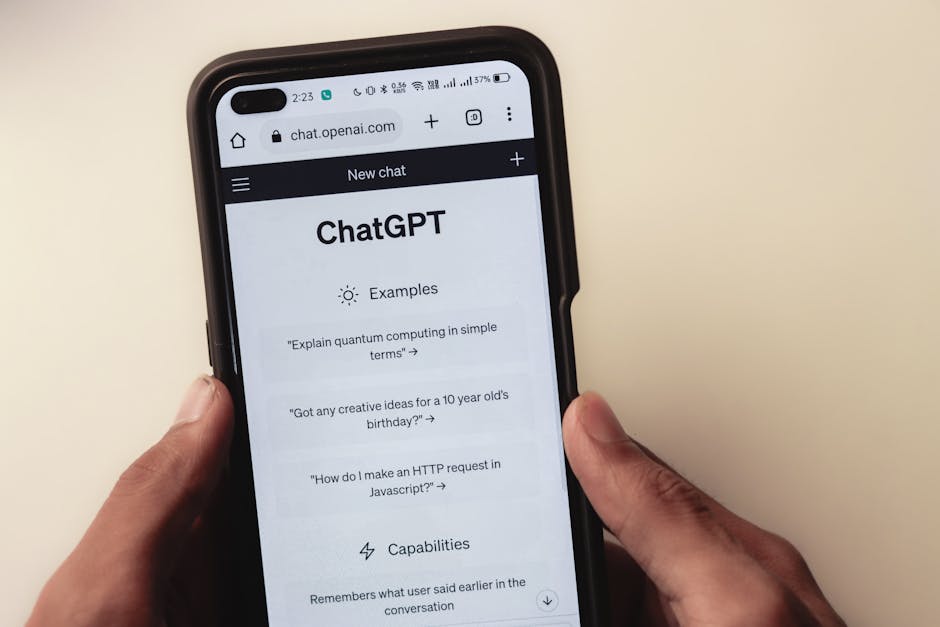
Research How AlphaChip transformed computer chip design Share.
Our AI method has accelerated and optimized chip design, and its superhuman chip layouts are used in hardware around the world In 2020, we released a preprint introducing our novel reinforcement learning method for designing chip layouts, which we later . Today, we’re publishing a Nature addendum that describes more about our method and its impact on the field of chip design. We’re also releasing a pre-trained checkpoint, sharing the model weights and announcing its name: AlphaChip. Computer chips have fueled remarkable progress in artificial intelligence (AI), and AlphaChip returns the favor by using AI to accelerate and optimize chip design. The method has been used to design superhuman chip layouts in the last three generations of Google’s custom AI accelerator, the Tensor Processing Unit (TPU). AlphaChip was one of the first reinforcement learning approaches used to solve a real-world engineering problem. It generates superhuman or comparable chip layouts in hours, rather than taking weeks or months of human effort, and its layouts are used in chips all over the world, from data centers to mobile phones.
“ AlphaChip’s groundbreaking AI approach revolutionizes a key phase of chip design. SR Tsai, Senior Vice President of MediaTek.
How AlphaChip works Designing a chip layout is not a simple task. Computer chips consist of many interconnected blocks, with layers of circuit components, all connected by incredibly thin wires. There are also lots of complex and intertwined design constraints that all have to be met at the same time. Because of its sheer complexity, chip designers have struggled to automate the chip floorplanning process for over sixty years. Similar to AlphaGo and AlphaZero, which learned to master the games of Go, chess and shogi, we built AlphaChip to approach chip floorplanning as a kind of game. Starting from a blank grid, AlphaChip places one circuit component at a time until it’s done placing all the components. Then it’s rewarded based on the quality of the final layout. A novel “edge-based” graph neural network allows AlphaChip to learn the relationships between interconnected chip components and to generalize across chips, letting AlphaChip improve with each layout it designs.
Pause video Play video Left: Animation showing AlphaChip placing the open-source, Ariane RISC-V CPU, with no prior experience. Right: Animation showing AlphaChip placing the same block after having practiced on 20 TPU-related designs.
Using AI to design Google’s AI accelerator chips AlphaChip has generated superhuman chip layouts used in every generation of Google’s TPU since its publication in 2020. These chips make it possible to massively scale-up AI models based on Google’s Transformer architecture. TPUs lie at the heart of our powerful generative AI systems, from large language models, like Gemini, to image and video generators, Imagen and Veo. These AI accelerators also lie at the heart of Google's AI services and are available to external clients via Google Cloud.
A row of Cloud TPU v5p AI accelerator supercomputers in a Google data center.
To design TPU layouts, AlphaChip first practices on a diverse range of chip blocks from previous generations, such as on-chip and inter-chip network blocks, memory controllers, and data transport buffers. This process is called pre-training. Then we run AlphaChip on current TPU blocks to generate high-quality layouts. Unlike prior approaches, AlphaChip becomes advanced and faster as it solves more instances of the chip placement task, similar to how human experts do. With each new generation of TPU, including our latest Trillium (6th generation), AlphaChip has designed advanced chip layouts and provided more of the overall floorplan, accelerating the design cycle and yielding higher-performance chips.
Bar graph showing the number of AlphaChip designed chip blocks across three generations of Google’s Tensor Processing Units (TPU), including v5e, v5p and Trillium.
Bar graph showing AlphaChip’s average wirelength reduction across three generations of Google’s Tensor Processing Units (TPUs), compared to placements generated by the TPU physical design team.
AlphaChip’s broader impact AlphaChip’s impact can be seen through its applications across Alphabet, the research community and the chip design industry. Beyond designing specialized AI accelerators like TPUs, AlphaChip has generated layouts for other chips across Alphabet, such as Google Axion Processors, our first Arm-based general-purpose data center CPUs. External organizations are also adopting and building on AlphaChip. For example, MediaTek, one of the top chip design companies in the world, extended AlphaChip to accelerate development of their most advanced chips while improving power, performance and chip area.
AlphaChip has triggered an explosion of work on AI for chip design, and has been extended to other critical stages of chip design, such as logic synthesis and macro selection.
“ AlphaChip has inspired an entirely new line of research on reinforcement learning for chip design, cutting across the design flow from logic synthesis to floorplanning, timing optimization and beyond. Professor Siddharth Garg, NYU Tandon School of Engineering.
Creating the chips of the future We believe AlphaChip has the potential to optimize every stage of the chip design cycle, from computer architecture to manufacturing — and to transform chip design for custom hardware found in everyday devices such as smartphones, medical equipment, agricultural sensors and more. Future versions of AlphaChip are now in development and we look forward to working with the community to continue revolutionizing this area and bring about a future in which chips are even faster, cheaper and more power-efficient.
enterprise Realising scientists are the real superheroes Share.
Meet Edgar Duéñez-Guzmán, a research engineer on our Multi-Agent Resea...
Earlier this year, we introduced our video generation model, Veo, and our latest image generation model, Imagen 3. Since then, it’s been exciting to w...
With the help of AlphaFold, researchers are designing more effective drugs like never before.
Karen Akinsanya is President of R&D, Therapeutics, at Sc...
Market Impact Analysis
Market Growth Trend
2018 | 2019 | 2020 | 2021 | 2022 | 2023 | 2024 |
---|---|---|---|---|---|---|
23.1% | 27.8% | 29.2% | 32.4% | 34.2% | 35.2% | 35.6% |
Quarterly Growth Rate
Q1 2024 | Q2 2024 | Q3 2024 | Q4 2024 |
---|---|---|---|
32.5% | 34.8% | 36.2% | 35.6% |
Market Segments and Growth Drivers
Segment | Market Share | Growth Rate |
---|---|---|
Machine Learning | 29% | 38.4% |
Computer Vision | 18% | 35.7% |
Natural Language Processing | 24% | 41.5% |
Robotics | 15% | 22.3% |
Other AI Technologies | 14% | 31.8% |
Technology Maturity Curve
Different technologies within the ecosystem are at varying stages of maturity:
Competitive Landscape Analysis
Company | Market Share |
---|---|
Google AI | 18.3% |
Microsoft AI | 15.7% |
IBM Watson | 11.2% |
Amazon AI | 9.8% |
OpenAI | 8.4% |
Future Outlook and Predictions
The Generation African Talent landscape is evolving rapidly, driven by technological advancements, changing threat vectors, and shifting business requirements. Based on current trends and expert analyses, we can anticipate several significant developments across different time horizons:
Year-by-Year Technology Evolution
Based on current trajectory and expert analyses, we can project the following development timeline:
Technology Maturity Curve
Different technologies within the ecosystem are at varying stages of maturity, influencing adoption timelines and investment priorities:
Innovation Trigger
- Generative AI for specialized domains
- Blockchain for supply chain verification
Peak of Inflated Expectations
- Digital twins for business processes
- Quantum-resistant cryptography
Trough of Disillusionment
- Consumer AR/VR applications
- General-purpose blockchain
Slope of Enlightenment
- AI-driven analytics
- Edge computing
Plateau of Productivity
- Cloud infrastructure
- Mobile applications
Technology Evolution Timeline
- Improved generative models
- specialized AI applications
- AI-human collaboration systems
- multimodal AI platforms
- General AI capabilities
- AI-driven scientific breakthroughs
Expert Perspectives
Leading experts in the ai tech sector provide diverse perspectives on how the landscape will evolve over the coming years:
"The next frontier is AI systems that can reason across modalities and domains with minimal human guidance."
— AI Researcher
"Organizations that develop effective AI governance frameworks will gain competitive advantage."
— Industry Analyst
"The AI talent gap remains a critical barrier to implementation for most enterprises."
— Chief AI Officer
Areas of Expert Consensus
- Acceleration of Innovation: The pace of technological evolution will continue to increase
- Practical Integration: Focus will shift from proof-of-concept to operational deployment
- Human-Technology Partnership: Most effective implementations will optimize human-machine collaboration
- Regulatory Influence: Regulatory frameworks will increasingly shape technology development
Short-Term Outlook (1-2 Years)
In the immediate future, organizations will focus on implementing and optimizing currently available technologies to address pressing ai tech challenges:
- Improved generative models
- specialized AI applications
- enhanced AI ethics frameworks
These developments will be characterized by incremental improvements to existing frameworks rather than revolutionary changes, with emphasis on practical deployment and measurable outcomes.
Mid-Term Outlook (3-5 Years)
As technologies mature and organizations adapt, more substantial transformations will emerge in how security is approached and implemented:
- AI-human collaboration systems
- multimodal AI platforms
- democratized AI development
This period will see significant changes in security architecture and operational models, with increasing automation and integration between previously siloed security functions. Organizations will shift from reactive to proactive security postures.
Long-Term Outlook (5+ Years)
Looking further ahead, more fundamental shifts will reshape how cybersecurity is conceptualized and implemented across digital ecosystems:
- General AI capabilities
- AI-driven scientific breakthroughs
- new computing paradigms
These long-term developments will likely require significant technical breakthroughs, new regulatory frameworks, and evolution in how organizations approach security as a fundamental business function rather than a technical discipline.
Key Risk Factors and Uncertainties
Several critical factors could significantly impact the trajectory of ai tech evolution:
Organizations should monitor these factors closely and develop contingency strategies to mitigate potential negative impacts on technology implementation timelines.
Alternative Future Scenarios
The evolution of technology can follow different paths depending on various factors including regulatory developments, investment trends, technological breakthroughs, and market adoption. We analyze three potential scenarios:
Optimistic Scenario
Responsible AI driving innovation while minimizing societal disruption
Key Drivers: Supportive regulatory environment, significant research breakthroughs, strong market incentives, and rapid user adoption.
Probability: 25-30%
Base Case Scenario
Incremental adoption with mixed societal impacts and ongoing ethical challenges
Key Drivers: Balanced regulatory approach, steady technological progress, and selective implementation based on clear ROI.
Probability: 50-60%
Conservative Scenario
Technical and ethical barriers creating significant implementation challenges
Key Drivers: Restrictive regulations, technical limitations, implementation challenges, and risk-averse organizational cultures.
Probability: 15-20%
Scenario Comparison Matrix
Factor | Optimistic | Base Case | Conservative |
---|---|---|---|
Implementation Timeline | Accelerated | Steady | Delayed |
Market Adoption | Widespread | Selective | Limited |
Technology Evolution | Rapid | Progressive | Incremental |
Regulatory Environment | Supportive | Balanced | Restrictive |
Business Impact | Transformative | Significant | Modest |
Transformational Impact
Redefinition of knowledge work, automation of creative processes. This evolution will necessitate significant changes in organizational structures, talent development, and strategic planning processes.
The convergence of multiple technological trends—including artificial intelligence, quantum computing, and ubiquitous connectivity—will create both unprecedented security challenges and innovative defensive capabilities.
Implementation Challenges
Ethical concerns, computing resource limitations, talent shortages. Organizations will need to develop comprehensive change management strategies to successfully navigate these transitions.
Regulatory uncertainty, particularly around emerging technologies like AI in security applications, will require flexible security architectures that can adapt to evolving compliance requirements.
Key Innovations to Watch
Multimodal learning, resource-efficient AI, transparent decision systems. Organizations should monitor these developments closely to maintain competitive advantages and effective security postures.
Strategic investments in research partnerships, technology pilots, and talent development will position forward-thinking organizations to leverage these innovations early in their development cycle.
Technical Glossary
Key technical terms and definitions to help understand the technologies discussed in this article.
Understanding the following technical concepts is essential for grasping the full implications of the security threats and defensive measures discussed in this article. These definitions provide context for both technical and non-technical readers.