Updated production-ready Gemini models, reduced 1.5 Pro pricing, increased rate limits, and more - Related to pragmatism, limits,, red, pro, reduced
New generative AI tools open the doors of music creation
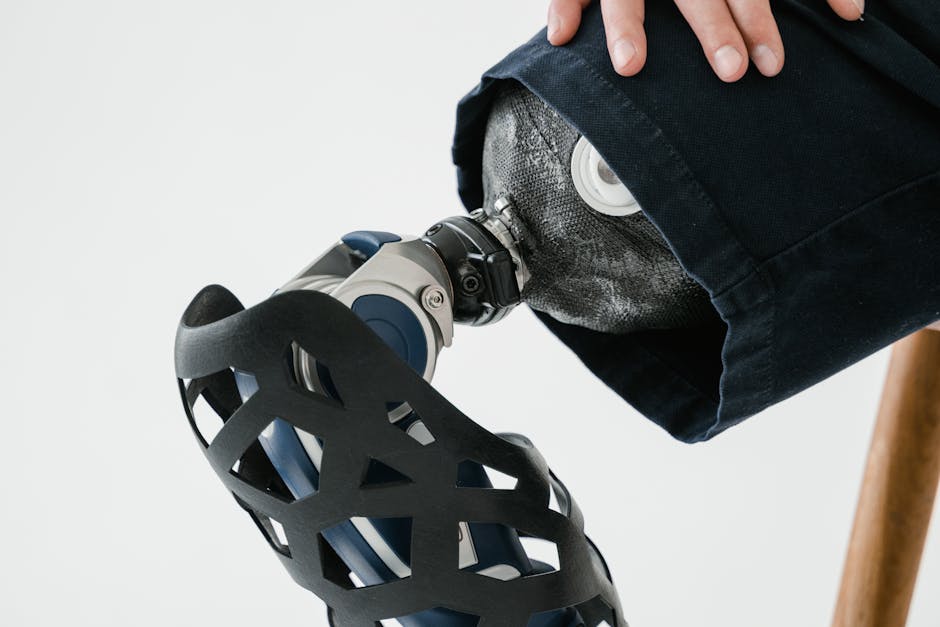
Technologies New generative AI tools open the doors of music creation Share.
Our latest AI music technologies are now available in MusicFX DJ, Music AI Sandbox and YouTube Shorts For nearly a decade, our teams have been exploring how artificial intelligence (AI) can support the creative process, building tools that empower enthusiasts and professionals to discover new forms of creative expression. Over the past year, we’ve been working in close collaboration with partners across the music industry through our Music AI Incubator and more. Their input has been guiding our state-of-the-art generative music experiments, and helping us ensure that our new generative AI tools responsibly open the doors of music creation to everyone. Today, in partnership with Google Labs, we're releasing a reimagined experience for MusicFX DJ that makes it easier for anyone to generate music, interactively, in real time. We’re also announcing updates to our music AI toolkit, called Music AI Sandbox, and highlighting our latest AI music technologies in YouTube’s Dream Track, a suite of experiments that creators can use to generate high-quality instrumentals for their Shorts and videos.
Generating live music with MusicFX DJ At I/O this year, we shared an early preview of MusicFX DJ, a digital tool that anyone can play like an instrument, making the joy of live music creation more accessible to people of all skill levels. Today, we’re introducing a number of updates to MusicFX DJ, including an expanded set of intuitive controls, a reimagined interface, improved audio quality and new model behaviors. These capabilities let players generate and steer a continuous flow of music, share their creations with friends and play a jam session together. Working in close collaboration with Jacob Collier — a six-time GRAMMY award-winning singer, songwriter, producer and multi-instrumentalist — we designed these updates to make MusicFX DJ more accessible, useful and inspiring.
Unmute video Mute video Pause video Play video.
Unlike traditional DJ tools that mix together preexisting tracks, MusicFX DJ generates brand new music by allowing players to mix musical concepts as text prompts. With MusicFX DJ, players can combine their favorite genres, instruments and vibes to create new styles, improvise a live DJ set or search for new melodies, timbres and rhythms to use in production. While not a traditional musical instrument, MusicFX DJ is an accessible and expressive entry point to live music creation. Regardless of one’s musical experience, MusicFX DJ empowers players with intuitive controls to generate and steer a unique and continuously evolving musical soundscape.
“ You craft this real-time sonic putty that’s endlessly surprising and essentially seeks to alchemize or forge connections between things that would otherwise be unlikely. Jacob Collier.
Two novel approaches underpin MusicFX DJ. First, we adapted an offline generative music model to perform real-time streaming. We did this by training it to generate the next clip of music, based on the previous generated music and the text prompts provided by the player. Second, instead of having a single fixed text prompt, like typical text-to-music models, we give players the ability to mix together multiple text prompts and change the mixture over time. The model achieves this by mixing together representations of each prompt, known as embeddings, with the relative importance of each embedding, chosen by the player using a slider. The model uses these combined embeddings to help steer the style of music.
Unmute video Mute video Pause video Play video Flowchart showing how MusicFX DJ generates a continuous flow of music, creating the next clip from previous clips, while steered by the text prompts and sliders to weight their importance.
Building more intuitive controls Together with Jacob, we looked to discover and build dedicated controls that could be intuitive to beginners, encourage experimentation and provide more diverse routes to creative expression than text prompts alone. With MusicFX DJ’s new controls, players can conduct the instrumentation and easily create breakdowns and bass drops by removing and adding the bass, drums and other instruments. They can adjust textural aspects of the music, like how bright or dark, repetitive or random and smooth or rough it should sound and feel. Players can also control key and tempo, making it easier to play along with existing music and with others during extended jam sessions. Our teams have really enjoyed using MusicFX DJ alongside their traditional instruments, and we can’t wait to hear what others create with these new capabilities.
Unmute video Mute video Pause video Play video Unmute video Mute video Pause video Play video Unmute video Mute video Pause video Play video Unmute video Mute video Pause video Play video.
Generating production-quality sound As part of the collaboration, we also explored how a player could use the model output as both a source of inspiration and as part of a large composition. But our earlier models lacked the quality needed for creating professional audio. Thanks to the latest innovations from our audio research team, including new neural audio codecs and optimized network architectures, MusicFX DJ is now able to stream production-quality 48 kHz stereo audio in real time.
Sharing and downloading audio Inspired by Jacob’s focus on creative collaboration, both with other artists and with his audiences, we wanted to make it easier to share and interact with music made with MusicFX DJ. Players can now download 60 seconds of their MusicFX DJ audio and share sessions with friends, who can watch a performance playback and even jump in to take over the controls at any point — taking the music in an entirely new direction.
An expanded Music AI Sandbox toolkit Music AI Sandbox is an experimental suite of music AI tools that aims to supercharge the workflows of musicians, producers and songwriters who collaborate with us through YouTube’s Music AI Incubator. It has been a valuable testing ground for gathering feedback from diverse artists, songwriters and partners across the music industry about our latest and most experimental generative music tools. While the Music AI Sandbox isn’t currently publicly available, successful elements of this work will be integrated into widely-accessible Google products. Since showing the Music AI Sandbox publicly at this year’s I/O, we’ve also been working closely with Google’s Technology & Society team to improve the user experience and connect with the artistic community at scale to gather feedback. This work has helped us make significant updates to the models behind this suite of tools. Soon, trusted testers will be able to sketch a song and use a multi-track view to help organize and refine compositions with precise controls. This new version of Music AI Sandbox integrates our latest technologies, including models that power MusicFX DJ, along with popular features like loop generation, sound transformation and in-painting to help users seamlessly connect parts of their musical tracks.
Screenshot of user interface designs for our updated Music AI Sandbox, which has a multi-track view to help organize and refine compositions with precise controls.
YouTube's Dream Track experiment now generates instrumental soundtracks Building off our ongoing work with YouTube, we’ve evolved our Dream Track experiment to allow [website] creators to explore a range of genres and prompts that generate instrumental soundtracks with powerful text-to-music models.
Our latest music generation models are trained with a novel reinforcement learning approach to have higher audio quality, while also paying superior attention to the nuances of a user’s text prompts. Responsibly deploying generative technologies is core to our values, so all music generated by MusicFX DJ and Dream Track is watermarked using SynthID.
Building the future of music creation together We’ve been delighted to work with partners in the music community over the past year to help build technology that's both responsive to the needs of professionals and expands access for the next generation of musicians. We’re looking forward to deepening these partnerships as we build the future of music creation together, developing even advanced tools to inspire creativity.
New research drawing upon pragmatics and philosophy proposes ways to align conversational agents with human values.
AI provides a new tool for studying extinct species from 50,000 years ago.
Researchers Beatrice Demarchi from the University of Turin, Josefin Stiller...
corporation A new generation of African talent brings cutting-edge AI to scientific challenges Share.
Red Hat's take on open-source AI: Pragmatism over utopian dreams
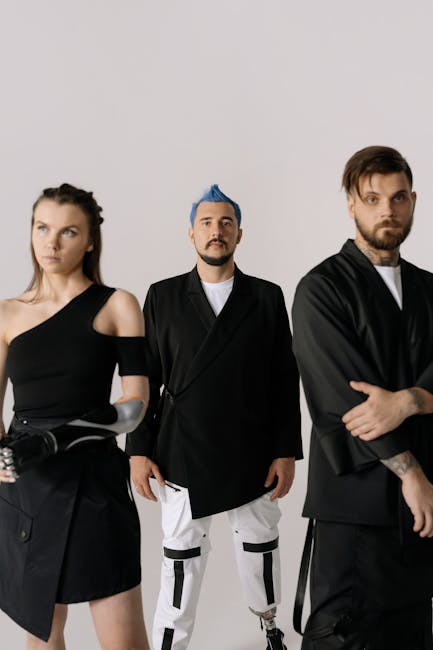
Open-source AI is changing everything people thought they knew about artificial intelligence. Just look at DeepSeek, the Chinese open-source program that blew the financial doors off the AI industry. Red Hat, the world's leading Linux organization, understands the power of open source and AI superior than most.
Red Hat's pragmatic approach to open-source AI reflects its decades-long commitment to open-source principles while grappling with the unique complexities of modern AI systems. Instead of chasing artificial general intelligence (AGI) dreams, Red Hat balances practical enterprise needs with what AI can deliver today.
Also: Mistral AI says its Small 3 model is a local, open-source alternative to GPT-4o mini.
Simultaneously, Red Hat is acknowledging the ambiguity surrounding "open-source AI." At the Linux Foundation Members Summit in November 2024, Richard Fontana, Red Hat's principal commercial counsel, highlighted that while traditional open-source software relies on accessible source code, AI introduces challenges with opaque training data and model weights.
During a panel discussion, Fontana stated, "What is the analog to [source code] for AI? That is not clear. Some people believe training data has to be open, but that's highly impractical for LLMs [large language models]. It hints at open-source AI may be a utopian aim at this stage."
This tension is evident in models released under licenses that are restrictive yet labeled "open-source." These fake open-source programs include Meta's LLama, and Fontana criticizes this trend, noting that many licenses discriminate against fields of endeavor or groups while still claiming openness.
A core challenge is reconciling transparency with competitive and legal realities. While Red Hat advocates for openness, Fontana cautions against rigid definitions requiring full disclosure of training data: Disclosing detailed training data risks targeting model creators in today's litigious environment. Fair use of publicly available data complicates transparency expectations.
Also: Red Hat bets big on AI with its Neural Magic acquisition.
Red Hat CTO Chris Wright emphasizes pragmatic steps toward reproducibility, advocating for open models like Granite LLMs and tools such as InstructLab, which enable community-driven fine-tuning. Wright writes: "InstructLab lets anyone contribute skills to models, making AI truly collaborative. It's how open source won in software -- now we're doing it for AI."
Wright frames this as an evolution of Red Hat's Linux legacy: "Just as Linux standardized IT infrastructure, RHEL AI provides a foundation for enterprise AI -- open, flexible, and hybrid by design."
Red Hat envisions AI development mirroring open-source software's collaborative ethos. Wright argues: "Models must be open-source artifacts. Sharing knowledge is Red Hat's mission -- this is how we avoid vendor lock-in and ensure AI benefits everyone."
Also: The best AI for coding in 2025 (and what not to use - including DeepSeek R1).
That won't be easy. Wright admits that "AI, especially the large language models driving generative AI, cannot be viewed in quite the same way as open source software. Unlike software, AI models principally consist of model weights, which are numerical parameters that determine how a model processes inputs, as well as the connections it makes between various data points. Trained model weights are the result of an extensive training process involving vast quantities of training data that are carefully prepared, mixed, and processed."
Although models are not software, Wright continues:
"In some respects, they serve a similar function to code. It's easy to draw the comparison that data is, or is analogous to, the source code of the model. Training data alone does not fit this role. The majority of improvements and enhancements to AI models now taking place in the community do not involve access to or manipulation of the original training data. Rather, they are the result of modifications to model weights or a process of fine-tuning, which can also serve to adjust model performance. Freedom to make those model improvements requires that the weights be released with all the permissions consumers receive under open-source licenses."
Also: The best Linux laptops of 2025: Expert tested and reviewed.
Wright concludes: "The future of AI is open, but it's a journey. We're tackling transparency, sustainability, and trust -- one open-source project at a time." Fontana's cautionary perspective grounds this vision, which is that open-source AI must respect competitive and legal realities. The community should refine definitions gradually, not force-fit ideals onto immature technology.
The OSI, while focusing on a definition, agrees. OSAID [website] is only the first imperfect version. The group is already working toward another version. In the meantime, Red Hat will continue its work in shaping AI's open future by building bridges between developer communities and enterprises while navigating AI transparency's thorny ethics.
New insights into how immunity evolves could help scientists protect all the world’s flora and fauna from disease.
Note: This blog was first . Following the paper’s publication in Science on 8 Dec 2022, we’ve made minor updates to the text to...
Our comprehensive benchmark and online leaderboard offer a much-needed measure of how accurately LLMs ground their responses in provided source materi...
Updated production-ready Gemini models, reduced 1.5 Pro pricing, increased rate limits, and more
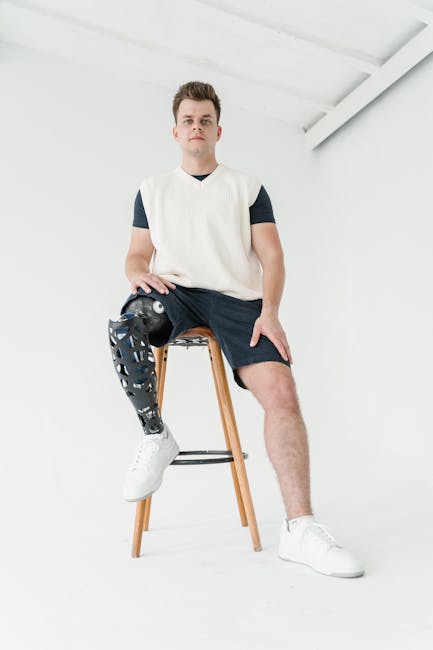
Today, we’re releasing two updated production-ready Gemini models: [website] and [website] along with: >50% reduced price on [website] Pro (both input and output for prompts <128K).
2x higher rate limits on [website] Flash and ~3x higher on [website] Pro.
Updated default filter settings These new models build on our latest experimental model releases and include meaningful improvements to the Gemini [website] models released at Google I/O in May. Developers can access our latest models for free via Google AI Studio and the Gemini API. For larger organizations and Google Cloud end-individuals, the models are also available on Vertex AI.
Improved overall quality, with larger gains in math, long context, and vision The Gemini [website] series are models that are designed for general performance across a wide range of text, code, and multimodal tasks. For example, Gemini models can be used to synthesize information from 1000 page PDFs, answer questions about repos containing more than 10 thousand lines of code, take in hour long videos and create useful content from them, and more. With the latest updates, [website] Pro and Flash are now superior, faster, and more cost-efficient to build with in production. We see a ~7% increase in MMLU-Pro, a more challenging version of the popular MMLU benchmark. On MATH and HiddenMath (an internal holdout set of competition math problems) benchmarks, both models have made a considerable ~20% improvement. For vision and code use cases, both models also perform superior (ranging from ~2-7%) across evals measuring visual understanding and Python code generation.
We also improved the overall helpfulness of model responses, while continuing to uphold our content safety policies and standards. This means less punting/fewer refusals and more helpful responses across many topics. Both models now have a more concise style in response to developer feedback which is intended to make these models easier to use and reduce costs. For use cases like summarization, question answering, and extraction, the default output length of the updated models is ~5-20% shorter than previous models. For chat-based products where individuals might prefer longer responses by default, you can read our prompting strategies guide to learn more about how to make the models more verbose and conversational.
Gemini [website] Pro We continue to be blown away with the creative and useful applications of Gemini [website] Pro’s 2 million token long context window and multimodal capabilities. From video understanding to processing 1000 page PDFs, there are so many new use cases still to be built. Today we are announcing a 64% price reduction on input tokens, a 52% price reduction on output tokens, and a 64% price reduction on incremental cached tokens for our strongest [website] series model, Gemini [website] Pro, effective October 1st, 2024, on prompts less than 128K tokens. Coupled with context caching, this continues to drive the cost of building with Gemini down.
Increased rate limits To make it even easier for developers to build with Gemini, we are increasing the paid tier rate limits for [website] Flash to 2,000 RPM and increasing [website] Pro to 1,000 RPM, up from 1,000 and 360, respectively. In the coming weeks, we expect to continue to increase the Gemini API rate limits so developers can build more with Gemini.
2x faster output and 3x less latency Along with core improvements to our latest models, over the last few weeks we have driven down the latency with [website] Flash and significantly increased the output tokens per second, enabling new use cases with our most powerful models.
Research Google DeepMind at NeurIPS 2024 Share.
Building adaptive, smart, and safe AI Agents LLM-based AI agents are showing promis...
Research A generalist AI agent for 3D virtual environments Share.
We present new research on a Scalable Instructable Multiworld Age...
ChatGPT was originally available only on browsers, but since then, OpenAI has expanded access to mobile and desktop apps. In Dece...
Market Impact Analysis
Market Growth Trend
2018 | 2019 | 2020 | 2021 | 2022 | 2023 | 2024 |
---|---|---|---|---|---|---|
23.1% | 27.8% | 29.2% | 32.4% | 34.2% | 35.2% | 35.6% |
Quarterly Growth Rate
Q1 2024 | Q2 2024 | Q3 2024 | Q4 2024 |
---|---|---|---|
32.5% | 34.8% | 36.2% | 35.6% |
Market Segments and Growth Drivers
Segment | Market Share | Growth Rate |
---|---|---|
Machine Learning | 29% | 38.4% |
Computer Vision | 18% | 35.7% |
Natural Language Processing | 24% | 41.5% |
Robotics | 15% | 22.3% |
Other AI Technologies | 14% | 31.8% |
Technology Maturity Curve
Different technologies within the ecosystem are at varying stages of maturity:
Competitive Landscape Analysis
Company | Market Share |
---|---|
Google AI | 18.3% |
Microsoft AI | 15.7% |
IBM Watson | 11.2% |
Amazon AI | 9.8% |
OpenAI | 8.4% |
Future Outlook and Predictions
The Open Generative Tools landscape is evolving rapidly, driven by technological advancements, changing threat vectors, and shifting business requirements. Based on current trends and expert analyses, we can anticipate several significant developments across different time horizons:
Year-by-Year Technology Evolution
Based on current trajectory and expert analyses, we can project the following development timeline:
Technology Maturity Curve
Different technologies within the ecosystem are at varying stages of maturity, influencing adoption timelines and investment priorities:
Innovation Trigger
- Generative AI for specialized domains
- Blockchain for supply chain verification
Peak of Inflated Expectations
- Digital twins for business processes
- Quantum-resistant cryptography
Trough of Disillusionment
- Consumer AR/VR applications
- General-purpose blockchain
Slope of Enlightenment
- AI-driven analytics
- Edge computing
Plateau of Productivity
- Cloud infrastructure
- Mobile applications
Technology Evolution Timeline
- Improved generative models
- specialized AI applications
- AI-human collaboration systems
- multimodal AI platforms
- General AI capabilities
- AI-driven scientific breakthroughs
Expert Perspectives
Leading experts in the ai tech sector provide diverse perspectives on how the landscape will evolve over the coming years:
"The next frontier is AI systems that can reason across modalities and domains with minimal human guidance."
— AI Researcher
"Organizations that develop effective AI governance frameworks will gain competitive advantage."
— Industry Analyst
"The AI talent gap remains a critical barrier to implementation for most enterprises."
— Chief AI Officer
Areas of Expert Consensus
- Acceleration of Innovation: The pace of technological evolution will continue to increase
- Practical Integration: Focus will shift from proof-of-concept to operational deployment
- Human-Technology Partnership: Most effective implementations will optimize human-machine collaboration
- Regulatory Influence: Regulatory frameworks will increasingly shape technology development
Short-Term Outlook (1-2 Years)
In the immediate future, organizations will focus on implementing and optimizing currently available technologies to address pressing ai tech challenges:
- Improved generative models
- specialized AI applications
- enhanced AI ethics frameworks
These developments will be characterized by incremental improvements to existing frameworks rather than revolutionary changes, with emphasis on practical deployment and measurable outcomes.
Mid-Term Outlook (3-5 Years)
As technologies mature and organizations adapt, more substantial transformations will emerge in how security is approached and implemented:
- AI-human collaboration systems
- multimodal AI platforms
- democratized AI development
This period will see significant changes in security architecture and operational models, with increasing automation and integration between previously siloed security functions. Organizations will shift from reactive to proactive security postures.
Long-Term Outlook (5+ Years)
Looking further ahead, more fundamental shifts will reshape how cybersecurity is conceptualized and implemented across digital ecosystems:
- General AI capabilities
- AI-driven scientific breakthroughs
- new computing paradigms
These long-term developments will likely require significant technical breakthroughs, new regulatory frameworks, and evolution in how organizations approach security as a fundamental business function rather than a technical discipline.
Key Risk Factors and Uncertainties
Several critical factors could significantly impact the trajectory of ai tech evolution:
Organizations should monitor these factors closely and develop contingency strategies to mitigate potential negative impacts on technology implementation timelines.
Alternative Future Scenarios
The evolution of technology can follow different paths depending on various factors including regulatory developments, investment trends, technological breakthroughs, and market adoption. We analyze three potential scenarios:
Optimistic Scenario
Responsible AI driving innovation while minimizing societal disruption
Key Drivers: Supportive regulatory environment, significant research breakthroughs, strong market incentives, and rapid user adoption.
Probability: 25-30%
Base Case Scenario
Incremental adoption with mixed societal impacts and ongoing ethical challenges
Key Drivers: Balanced regulatory approach, steady technological progress, and selective implementation based on clear ROI.
Probability: 50-60%
Conservative Scenario
Technical and ethical barriers creating significant implementation challenges
Key Drivers: Restrictive regulations, technical limitations, implementation challenges, and risk-averse organizational cultures.
Probability: 15-20%
Scenario Comparison Matrix
Factor | Optimistic | Base Case | Conservative |
---|---|---|---|
Implementation Timeline | Accelerated | Steady | Delayed |
Market Adoption | Widespread | Selective | Limited |
Technology Evolution | Rapid | Progressive | Incremental |
Regulatory Environment | Supportive | Balanced | Restrictive |
Business Impact | Transformative | Significant | Modest |
Transformational Impact
Redefinition of knowledge work, automation of creative processes. This evolution will necessitate significant changes in organizational structures, talent development, and strategic planning processes.
The convergence of multiple technological trends—including artificial intelligence, quantum computing, and ubiquitous connectivity—will create both unprecedented security challenges and innovative defensive capabilities.
Implementation Challenges
Ethical concerns, computing resource limitations, talent shortages. Organizations will need to develop comprehensive change management strategies to successfully navigate these transitions.
Regulatory uncertainty, particularly around emerging technologies like AI in security applications, will require flexible security architectures that can adapt to evolving compliance requirements.
Key Innovations to Watch
Multimodal learning, resource-efficient AI, transparent decision systems. Organizations should monitor these developments closely to maintain competitive advantages and effective security postures.
Strategic investments in research partnerships, technology pilots, and talent development will position forward-thinking organizations to leverage these innovations early in their development cycle.
Technical Glossary
Key technical terms and definitions to help understand the technologies discussed in this article.
Understanding the following technical concepts is essential for grasping the full implications of the security threats and defensive measures discussed in this article. These definitions provide context for both technical and non-technical readers.