The race to cure a billion people from a deadly parasitic disease - Related to billion, a, chatgpt, synthid, images
How to make ChatGPT provide better sources and citations
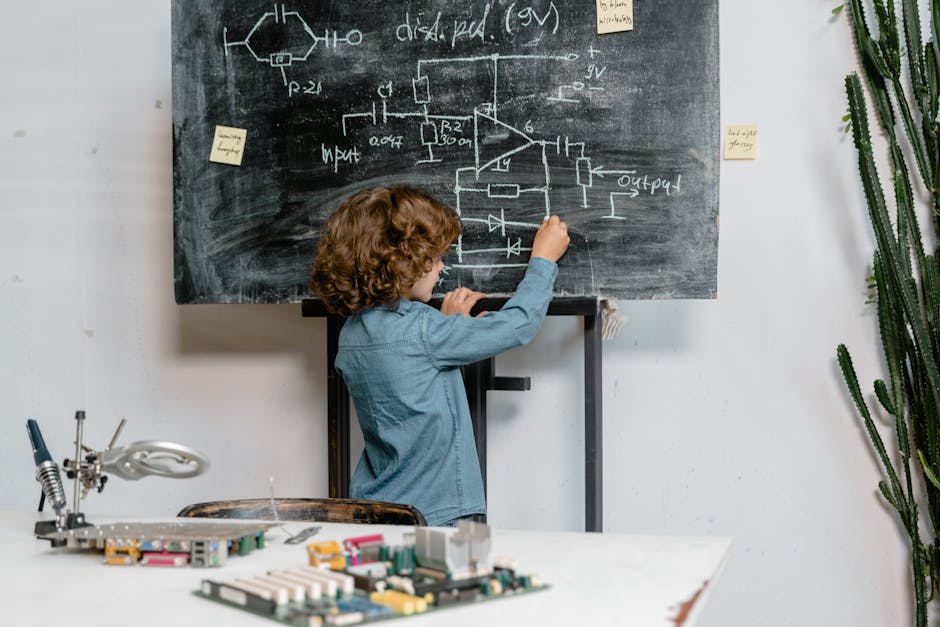
One of the biggest complaints about ChatGPT is that it provides information that is difficult to check for accuracy. Those complaints exist because ChatGPT doesn't always provide the findings, footnotes, or links from which it derived the information in its answers.
Here's how ChatGPT describes the approach: "GPT-4o in free mode provides basic and essential citations, focusing on quick and concise references to ensure information is traceable. In contrast, GPT-4o in paid mode offers enhanced, detailed, and frequent citations, including multiple findings and contextual annotations to provide comprehensive verification and understanding of the information. This ensures a robust and reliable experience, especially beneficial for clients requiring in-depth information and thorough source verification."
Also: The best AI for coding in 2025 (and what not to use).
Even with the provided citations in GPT-4o, there are ways to improve your results.
How to make ChatGPT provide findings and citations.
1. Write a query and ask ChatGPT To start, you need to ask ChatGPT something that needs reports or citations. I've found it's superior to ask a question with a longer answer, so there's more "meat" for ChatGPT to chew on. Also: The best AI chatbots Keep in mind that ChatGPT can't provide any information after January 2022 for [website], April 2023 for GPT-4, and October 2023 for GPT-4o, and requests for information pre-internet (say, for a paper on Ronald Reagan's presidency) will have far fewer available reports. Here's an example of a prompt I wrote on a topic that I worked on a lot when I was in grad school: Describe the learning theories of cognitivism, behaviorism, and constructivism Show more.
3. Push ChatGPT to give you higher-quality data Most large language models respond well to detail and specificity. So if you're asking for data, you can push for higher-quality data. You'll need to specify that you need reliable and accurate data. While this approach won't necessarily work, it may remind the AI chatbot to give you more useful responses. For example: Please provide me with reputable data to support my argument on... (whatever the topic is you're looking at) You can also tell ChatGPT the kinds of data you want. If you're looking for scholarly articles, peer-reviewed journals, books, or authoritative websites, mention these preferences explicitly. For example: Please recommend peer-reviewed journals that discuss... (and here, repeat what you discussed earlier in your conversation) When dealing with abstract concepts or theories, request that ChatGPT provide a conceptual framework and real-world examples. Here's an example: Can you describe the principles of Vygotsky's Social Development Theory and provide real-world examples where these principles were applied, including data for these examples? This approach gives you a theoretical explanation and practical instances to trace the original data or case studies. Also: Two ways you can build custom AI assistants with GPT-4o Another idea is to use data that don't have link rot (that is, they're no longer online at the URL that ChatGPT might know). Be careful with this idea, though, because ChatGPT doesn't know about things after January 2022 for [website], April 2023 for GPT-4, and October 2023 for GPT-4o. So, while you might be tempted to use a prompt like this: Please provide me with data . Instead, consider using a prompt like this: Please provide data . And, as always, don't assume that whatever output ChatGPT gives you is accurate. It's still quite possible the AI will completely fabricate answers, even to the point of making up the names of what seem like academic journals. It's a sometimes helpful tool, but it's also a fibber. Please doe a web search to find more current data. GPT-4o using the Plus plan will do web searches. Keep in mind that ChatGPT doesn't really validate web results, so while it might give you data later than October 2023, there's no guarantee that the data themselves are accurate. Show more.
4. Attempt to verify/validate the provided findings Keep this golden rule in mind about ChatGPT-provided findings: ChatGPT is more often wrong than right. Across the many times I've asked ChatGPT for URL findings, roughly half were just plain bad links. Another 25% or more of the links went to topics completely or somewhat unrelated to the one I was trying to source. GPT-4 and GPT-4o are slightly more reliable, but not by much. Also: The five biggest mistakes people make when prompting an AI For example, I asked for findings on a backgrounder for the phrase "trust but verify," generally popularized by US President Ronald Reagan. I got a lot of findings back, but most didn't exist. I got some back that correctly took me to active pages on the Reagan Presidential Library site, but the page topic had nothing to do with the phrase. I had superior luck with my learning theory question from step 1. There, I got back offline texts from people I knew from my studies who had worked on those theories. I also got back URLs. Once again, only about two in 10 worked or were accurate. Don't despair. The idea isn't to expect ChatGPT to provide findings that you can immediately use. If you instead think of ChatGPT as a research assistant, it will give you some great starting places. Use the names of the articles (which may be completely fake or just not accessible) and drop them into Google. That process will give you some interesting search queries that probably lead to interesting material that can legitimately go into your research. Also, keep in mind that you're not limited to using ChatGPT. Don't forget all the tools available to researchers and students. Do your own web searches. Check with primary findings and subject-matter experts if they're available. If you're in school, you can even ask your friendly neighborhood librarian for help. Also: How to use ChatGPT to create an app Don't forget that there are many excellent traditional findings. For example, Google Scholar and JSTOR provide access to a wide range of academically acceptable resources you can cite with reasonable confidence. One final point: if you merely cut and paste ChatGPT findings into your research, you're likely to get stung. Use the AI for clues, not as a way to avoid the real work of research. Show more.
Will different ChatGPT LLMs provide different results?
Yes. Let's discuss the main LLMs currently available:
[website] This is the OG ChatGPT and isn't really all that common anymore since GPT-4 is available even with the free version. [website] couldn't cite or verify reports, and it tended to make stuff up quite often.
This is now the main GPT version available to both free and Plus consumers. It provides higher quality citations, findings with some context, and more accurate URLs. However, the free version allocates substantially reduced computing resources, which results in less frequent and reduced detail in findings because the free version optimizes for access rather than depth. The paid version does provide web searching, which will enable you to get findings (of varying degrees of quality) from after the knowledge base cut-off date of October 2023. GPT-o1: This is OpenAI's "thinking" model, which takes a bit longer to work through answers. That presented, don't expect higher quality source citations because even though it crunches concepts more intensely, it doesn't have more or enhanced data to work with than GPT-4o.
The bottom line is that GPT-4o will provide the best findings right now, and if you want the very best ChatGPT offers when it comes to depth of findings and citations, you'll want to pay for the $20/month Plus version.
APA style is a citation style that's often required in academic programs. APA stands for American Psychological Association. I've often thought they invented these style rules to get more consumers. The definitive starting point for APA style is the Purdue OWL, which provides a wide range of style guidelines.
Be careful: online style formatters might not do a complete job, and you may get your work returned by your professor. It pays to do the work yourself -- and be careful doing it.
How can I make ChatGPT provide more reliable data for its responses?
This is a good question. I have found that sometimes -- sometimes -- if you ask ChatGPT to give you more reports or re-ask for reports, it will give you new listings. If you tell ChatGPT the reports it provided were erroneous, it will sometimes give you enhanced ones. The bot may also apologize and give excuses. Another approach is to re-ask your original question with a different focus or direction, and then ask for reports for the new answer.
Once again, my best advice is to avoid treating ChatGPT as a tool that writes for you and more as a writing assistant. Asking for findings to cut and paste a ChatGPT response is pretty much plagiarism. That mentioned, using ChatGPT's responses, and any findings you can tease out, as clues for further research and writing is a legitimate way to use this tool.
For some links, it's just link rot. Some links may have changed, since many data are more than three years old. Other data are of indeterminate age. Since we don't have a full listing of ChatGPT's data, it's impossible to tell how valid they are or were.
Since ChatGPT was trained mostly without human supervision, we know that most of its information weren't vetted and could be wrong, made up, or completely non-existent.
Deepseek has lately made quite a buzz in the AI community, thanks to its impressive performance at...
The most notable part of Google's latest responsible AI findings could be what i...
When you're inviting friends, family, or co-workers to an event, the most convenient option i...
The race to cure a billion people from a deadly parasitic disease
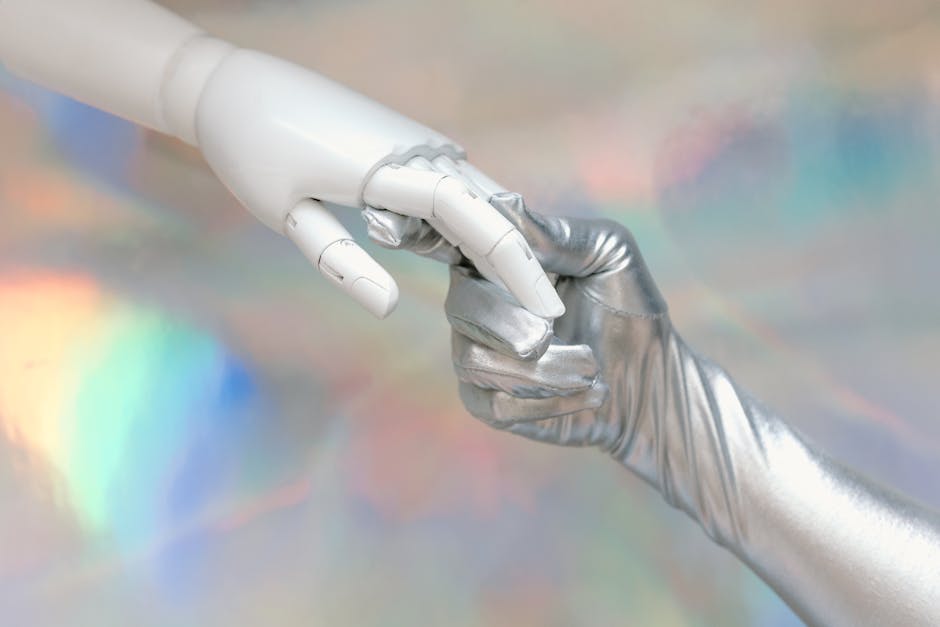
Impact The race to cure a billion people from a deadly parasitic disease Share.
Researchers accelerate their search of life-saving treatments for leishmaniasis “We were about to give up,” says Dr Benjamin Perry, a medicinal chemist at the Drugs for Neglected Diseases initiative (DNDi). When Perry joined the organization seven years ago, based in Geneva, Switzerland, his goal was to speed up the discovery of new treatments for two potentially fatal parasitic illnesses, Chagas disease and leishmaniasis. By and large, they achieved a lot of success. For one potential leishmaniasis drug in DNDi’s diverse portfolio, however, progress had slowed almost to a halt. “We couldn’t find ways of making changes that improved the drug molecule,” says Perry. “It either lost all its potency as an anti-parasitic or it kind of stayed the same.” However, things changed when Perry and his collaborators heard about DeepMind’s AI system, AlphaFold. Now, using a combination of scientific detective work and AI, the researchers have cleared a path towards turning the molecule into a real treatment for a devastating disease. New treatments for leishmaniasis can’t come soon enough. The disease is caused by parasites of the genus Leishmania and spreads through sandfly bites in countries across Asia, Africa, the Americas, and the Mediterranean. Visceral leishmaniasis, the most severe form, causes fever, weight loss, anemia, and enlargement of the spleen and liver. “If it’s not treated, it is fatal,” says Dr Gina Muthoni Ouattara, senior medical manager at DNDi in Nairobi, Kenya. Cutaneous leishmaniasis, the most common form, causes skin lesions and leaves lasting scars.
A patient with visceral leishmaniasis and an HIV co-infection. Credit: University of Gondar.
Globally, about a billion people are at risk of leishmaniasis and each year there are 50-90,000 new cases of visceral leishmaniasis, the majority in children. While medical treatments vary by region, most are lengthy and come with significant side effects. In Eastern Africa, the first-line treatment for visceral leishmaniasis involves a 17-day course of two injections each day, of two separate drugs, sodium stibogluconate and paromomycin, given in hospital. "Even for an adult, these injections are very painful, so you can imagine having to give these two injections to a child every day for 17 days,” says Ouattara. Before DNDi’s crucial work to develop a shorter and more effective combination therapy, this treatment lasted for 30 days. An alternative treatment requires an intravenous infusion that needs to be kept refrigerated and administered under sterile conditions. “The most limiting thing is that all of these treatments have to be given in hospital,” says Ouattara. That adds to the costs, and means patients and their caregivers miss out on income, school, and time with their family. “It really affects communities.”.
“ People always ask themselves, ‘Have we looked at the AlphaFold structure?’ It’s become common parlance. Michael Barrett, biochemist and parasitologist.
DNDi’s previous efforts have already cut the amount of time visceral leishmaniasis patients spend in hospital. But the organization’s ultimate goal is to come up with an oral treatment that could be administered at a local health facility, or even at home. That kind of radical improvement might require entirely new drugs. If you’re looking for completely new compounds to turn into treatments, where do you start? DNDi’s approach to drug discovery in this area of research could be called “old school”, says Perry, though he maintains there’s a reason for that – it’s often the best way to discover drugs. First, researchers screen thousands of molecules to find those that show promise in attacking the disease-causing organism as a whole. Then, they tweak those molecules to try to make them more effective. “It’s a bit more ‘brute force’,” he says. “We don’t usually know how it’s doing it.”.
Benjamin Perry and Gina Muthoni Ouattara. Credit: DNDi.
This trial-and-error approach is the best way to find new treatments for patients, says Perry. But the optimisation stage can feel a bit like stumbling around in the dark. “You're going ‘Okay, well, I've got this chemical, just make some random changes to it’ which works sometimes,” says Perry. But with their promising leishmaniasis molecule, they’d hit a brick wall. “We’d tried that and it hadn't worked.” With hope dwindling, DNDi sent the molecule to Michael Barrett, a professor at the University of Glasgow, UK, who for the last decade has been using a technique called metabolomics to unravel how drugs work. “There are all sorts of chemical processes occurring in our body where we chop molecules down into their component building blocks and then rebuild them,” says Barrett. “That's the basis of life, really.” Collectively, these chemical reactions make up our metabolism. Parasites, like the one that causes leishmaniasis, have a metabolism too. Metabolic reactions are regulated by biological catalysts known as enzymes. Many drugs work by interfering with those enzymes, so Barrett and his group look for changes in the molecules that are made during metabolic reactions to figure out what a drug is doing. He put DNDi’s molecule on to a Leishmania parasite. “Sure enough, the metabolism changed,” he says. Barrett and his colleagues saw a big increase in one molecule whose job is to turn into phospholipids, a type of fat molecule that makes up cell membranes. Yet at the same time, the number of phospholipids actually being made was decreasing. Barrett figured out that the enzyme that would have turned the first molecule into phospholipids was the one that was being affected by the drug. Interrupting this reaction was how the molecule was killing the parasite.
Stella Akiror and John Oseluo taking down details after checking on a patient. Credit: Lameck Ododo - DNDi.
But having hurdled one obstacle, Barrett’s group hit another. They wanted to know what their target enzyme looked like, but finding its structure experimentally would be near impossible because it was a type of protein that is notoriously hard to work with in the lab. “It embeds itself in the membrane, and that makes it really difficult to fiddle with,” says Barrett. That could have been the end of the story. But instead Perry put Barrett in touch with researchers at DeepMind who were working on AlphaFold, an AI system that predicts a protein’s 3D structure from its amino acid sequence. The AlphaFold team took the target protein’s amino acid sequence and came back with exactly what Barrett and his colleagues needed: a prediction for its 3D structure. Barrett’s group took that structure, and the structure of DNDi’s molecule, and were able to figure out how they fit together – pinning down, virtually at least, how the drug binds to the protein.
“ Most of the diseases we work with are endemic in countries where the [scientific] infrastructure is not necessarily that great. Benjamin Perry, medicinal chemist.
Quantum computers have the potential to revolutionize drug discovery, material design and fundamental physics — that is, if we can get them to work re...
Advancing best-in-class large models, compute-optimal RL agents, and more transparent, ethical, and fair AI systems.
Before DeepMind, I worked for a social purpose startup that increased access to mental healthcare. Then I got a job a...
Identifying AI-generated images with SynthID
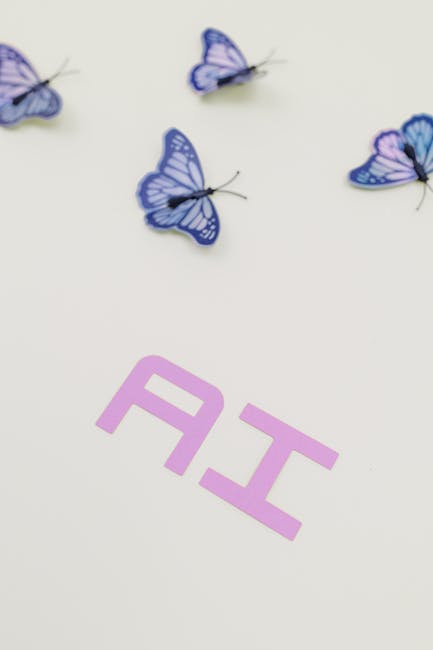
Technologies Identifying AI-generated images with SynthID Share.
New tool helps watermark and identify synthetic images created by Imagen AI-generated images are becoming more popular every day. But how can we improved identify them, especially when they look so realistic? Today, in partnership with Google Cloud, we’re launching a beta version of SynthID, a tool for watermarking and identifying AI-generated images. This technology embeds a digital watermark directly into the pixels of an image, making it imperceptible to the human eye, but detectable for identification. SynthID is being released to a limited number of Vertex AI consumers using Imagen, one of our latest text-to-image models that uses input text to create photorealistic images.
Generative AI technologies are rapidly evolving, and computer generated imagery, also known as ‘synthetic imagery’, is becoming harder to distinguish from those that have not been created by an AI system. While generative AI can unlock huge creative potential, it also presents new risks, like enabling creators to spread false information — both intentionally or unintentionally. Being able to identify AI-generated content is critical to empowering people with knowledge of when they’re interacting with generated media, and for helping prevent the spread of misinformation. We’re committed to connecting people with high-quality information, and upholding trust between creators and individuals across society. Part of this responsibility is giving individuals more advanced tools for identifying AI-generated images so their images — and even some edited versions — can be identified at a later date.
SynthID generates an imperceptible digital watermark for AI-generated images.
Google Cloud is the first cloud provider to offer a tool for creating AI-generated images responsibly and identifying them with confidence. This technology is grounded in our approach to developing and deploying responsible AI, and was developed by Google DeepMind and refined in partnership with Google Research. SynthID isn’t foolproof against extreme image manipulations, but it does provide a promising technical approach for empowering people and organisations to work with AI-generated content responsibly. This tool could also evolve alongside other AI models and modalities beyond imagery such as audio, video, and text. New type of watermark for AI images Watermarks are designs that can be layered on images to identify them. From physical imprints on paper to translucent text and symbols seen on digital photos today, they’ve evolved throughout history. Traditional watermarks aren’t sufficient for identifying AI-generated images because they’re often applied like a stamp on an image and can easily be edited out. For example, discrete watermarks found in the corner of an image can be cropped out with basic editing techniques. Finding the right balance between imperceptibility and robustness to image manipulations is difficult. Highly visible watermarks, often added as a layer with a name or logo across the top of an image, also present aesthetic challenges for creative or commercial purposes. Likewise, some previously developed imperceptible watermarks can be lost through simple editing techniques like resizing.
The watermark is detectable even after modifications like adding filters, changing colours and brightness.
We designed SynthID so it doesn't compromise image quality, and allows the watermark to remain detectable, even after modifications like adding filters, changing colours, and saving with various lossy compression schemes — most commonly used for JPEGs. SynthID uses two deep learning models — for watermarking and identifying — that have been trained together on a diverse set of images. The combined model is optimised on a range of objectives, including correctly identifying watermarked content and improving imperceptibility by visually aligning the watermark to the original content. Robust and scalable approach SynthID allows Vertex AI end-individuals to create AI-generated images responsibly and to identify them with confidence. While this technology isn’t perfect, our internal testing reveals that it’s accurate against many common image manipulations. SynthID's combined approach: Watermarking : SynthID can add an imperceptible watermark to synthetic images produced by Imagen.
: SynthID can add an imperceptible watermark to synthetic images produced by Imagen. Identification: By scanning an image for its digital watermark, SynthID can assess the likelihood of an image being created by Imagen.
SynthID can help assess how likely it is that an image was created by Imagen.
This tool provides three confidence levels for interpreting the results of watermark identification. If a digital watermark is detected, part of the image is likely generated by Imagen. SynthID contributes to the broad suite of approaches for identifying digital content. One of the most widely used methods of identifying content is through metadata, which provides information such as who created it and when. This information is stored with the image file. Digital signatures added to metadata can then show if an image has been changed. When the metadata information is intact, people can easily identify an image. However, metadata can be manually removed or even lost when files are edited. Since SynthID’s watermark is embedded in the pixels of an image, it’s compatible with other image identification approaches that are based on metadata, and remains detectable even when metadata is lost. What’s next? To build AI-generated content responsibly, we’re committed to developing safe, secure, and trustworthy approaches at every step of the way — from image generation and identification to media literacy and information security. These approaches need to be robust and adaptable as generative models advance and expand to other mediums. We hope our SynthID technology can work together with a broad range of solutions for creators and people across society, and we’re continuing to evolve SynthID by gathering feedback from people, enhancing its capabilities, and exploring new capabilities. SynthID could be expanded for use across other AI models and we’re excited about the potential of integrating it into more Google products and making it available to third parties in the near future — empowering people and organisations to responsibly work with AI-generated content. Note: The model used for producing synthetic images in this blog may be different from the model used on Imagen and Vertex AI.
Acknowledgements This project was led by Sven Gowal and Pushmeet Kohli, with key research and engineering contributions from (listed alphabetically): Rudy Bunel, Jamie Hayes, Sylvestre-Alvise Rebuffi, Florian Stimberg, David Stutz, and Meghana Thotakuri. Thanks to Nidhi Vyas and Zahra Ahmed for driving product delivery; Chris Gamble for helping initiate the project; Ian Goodfellow, Chris Bregler and Oriol Vinyals for their advice. Other contributors include Paul Bernard, Miklos Horvath, Simon Rosen, Olivia Wiles, and Jessica Yung. Thanks also to many others who contributed across Google DeepMind and Google, including our partners at Google Research and Google Cloud.
New white paper investigates models and functions of international institutions that could help manage opportunities and mitigate risks of advanced AI...
Responsibility & Safety Updating the Frontier Safety Framework Share.
Our next iteration of the FSF sets out stronger security prot...
NEVIS’22 is actually composed of 106 tasks extracted from publications randomly sampled from the online proceedings of major computer vision conferenc...
Market Impact Analysis
Market Growth Trend
2018 | 2019 | 2020 | 2021 | 2022 | 2023 | 2024 |
---|---|---|---|---|---|---|
23.1% | 27.8% | 29.2% | 32.4% | 34.2% | 35.2% | 35.6% |
Quarterly Growth Rate
Q1 2024 | Q2 2024 | Q3 2024 | Q4 2024 |
---|---|---|---|
32.5% | 34.8% | 36.2% | 35.6% |
Market Segments and Growth Drivers
Segment | Market Share | Growth Rate |
---|---|---|
Machine Learning | 29% | 38.4% |
Computer Vision | 18% | 35.7% |
Natural Language Processing | 24% | 41.5% |
Robotics | 15% | 22.3% |
Other AI Technologies | 14% | 31.8% |
Technology Maturity Curve
Different technologies within the ecosystem are at varying stages of maturity:
Competitive Landscape Analysis
Company | Market Share |
---|---|
Google AI | 18.3% |
Microsoft AI | 15.7% |
IBM Watson | 11.2% |
Amazon AI | 9.8% |
OpenAI | 8.4% |
Future Outlook and Predictions
The Make Chatgpt Provide landscape is evolving rapidly, driven by technological advancements, changing threat vectors, and shifting business requirements. Based on current trends and expert analyses, we can anticipate several significant developments across different time horizons:
Year-by-Year Technology Evolution
Based on current trajectory and expert analyses, we can project the following development timeline:
Technology Maturity Curve
Different technologies within the ecosystem are at varying stages of maturity, influencing adoption timelines and investment priorities:
Innovation Trigger
- Generative AI for specialized domains
- Blockchain for supply chain verification
Peak of Inflated Expectations
- Digital twins for business processes
- Quantum-resistant cryptography
Trough of Disillusionment
- Consumer AR/VR applications
- General-purpose blockchain
Slope of Enlightenment
- AI-driven analytics
- Edge computing
Plateau of Productivity
- Cloud infrastructure
- Mobile applications
Technology Evolution Timeline
- Improved generative models
- specialized AI applications
- AI-human collaboration systems
- multimodal AI platforms
- General AI capabilities
- AI-driven scientific breakthroughs
Expert Perspectives
Leading experts in the ai tech sector provide diverse perspectives on how the landscape will evolve over the coming years:
"The next frontier is AI systems that can reason across modalities and domains with minimal human guidance."
— AI Researcher
"Organizations that develop effective AI governance frameworks will gain competitive advantage."
— Industry Analyst
"The AI talent gap remains a critical barrier to implementation for most enterprises."
— Chief AI Officer
Areas of Expert Consensus
- Acceleration of Innovation: The pace of technological evolution will continue to increase
- Practical Integration: Focus will shift from proof-of-concept to operational deployment
- Human-Technology Partnership: Most effective implementations will optimize human-machine collaboration
- Regulatory Influence: Regulatory frameworks will increasingly shape technology development
Short-Term Outlook (1-2 Years)
In the immediate future, organizations will focus on implementing and optimizing currently available technologies to address pressing ai tech challenges:
- Improved generative models
- specialized AI applications
- enhanced AI ethics frameworks
These developments will be characterized by incremental improvements to existing frameworks rather than revolutionary changes, with emphasis on practical deployment and measurable outcomes.
Mid-Term Outlook (3-5 Years)
As technologies mature and organizations adapt, more substantial transformations will emerge in how security is approached and implemented:
- AI-human collaboration systems
- multimodal AI platforms
- democratized AI development
This period will see significant changes in security architecture and operational models, with increasing automation and integration between previously siloed security functions. Organizations will shift from reactive to proactive security postures.
Long-Term Outlook (5+ Years)
Looking further ahead, more fundamental shifts will reshape how cybersecurity is conceptualized and implemented across digital ecosystems:
- General AI capabilities
- AI-driven scientific breakthroughs
- new computing paradigms
These long-term developments will likely require significant technical breakthroughs, new regulatory frameworks, and evolution in how organizations approach security as a fundamental business function rather than a technical discipline.
Key Risk Factors and Uncertainties
Several critical factors could significantly impact the trajectory of ai tech evolution:
Organizations should monitor these factors closely and develop contingency strategies to mitigate potential negative impacts on technology implementation timelines.
Alternative Future Scenarios
The evolution of technology can follow different paths depending on various factors including regulatory developments, investment trends, technological breakthroughs, and market adoption. We analyze three potential scenarios:
Optimistic Scenario
Responsible AI driving innovation while minimizing societal disruption
Key Drivers: Supportive regulatory environment, significant research breakthroughs, strong market incentives, and rapid user adoption.
Probability: 25-30%
Base Case Scenario
Incremental adoption with mixed societal impacts and ongoing ethical challenges
Key Drivers: Balanced regulatory approach, steady technological progress, and selective implementation based on clear ROI.
Probability: 50-60%
Conservative Scenario
Technical and ethical barriers creating significant implementation challenges
Key Drivers: Restrictive regulations, technical limitations, implementation challenges, and risk-averse organizational cultures.
Probability: 15-20%
Scenario Comparison Matrix
Factor | Optimistic | Base Case | Conservative |
---|---|---|---|
Implementation Timeline | Accelerated | Steady | Delayed |
Market Adoption | Widespread | Selective | Limited |
Technology Evolution | Rapid | Progressive | Incremental |
Regulatory Environment | Supportive | Balanced | Restrictive |
Business Impact | Transformative | Significant | Modest |
Transformational Impact
Redefinition of knowledge work, automation of creative processes. This evolution will necessitate significant changes in organizational structures, talent development, and strategic planning processes.
The convergence of multiple technological trends—including artificial intelligence, quantum computing, and ubiquitous connectivity—will create both unprecedented security challenges and innovative defensive capabilities.
Implementation Challenges
Ethical concerns, computing resource limitations, talent shortages. Organizations will need to develop comprehensive change management strategies to successfully navigate these transitions.
Regulatory uncertainty, particularly around emerging technologies like AI in security applications, will require flexible security architectures that can adapt to evolving compliance requirements.
Key Innovations to Watch
Multimodal learning, resource-efficient AI, transparent decision systems. Organizations should monitor these developments closely to maintain competitive advantages and effective security postures.
Strategic investments in research partnerships, technology pilots, and talent development will position forward-thinking organizations to leverage these innovations early in their development cycle.
Technical Glossary
Key technical terms and definitions to help understand the technologies discussed in this article.
Understanding the following technical concepts is essential for grasping the full implications of the security threats and defensive measures discussed in this article. These definitions provide context for both technical and non-technical readers.