Tracing the evolution of proteins back to the origin of life - Related to back, wants, tracing, redefine, supercharge
Supercharge Your RAG with Multi-Agent Self-RAG
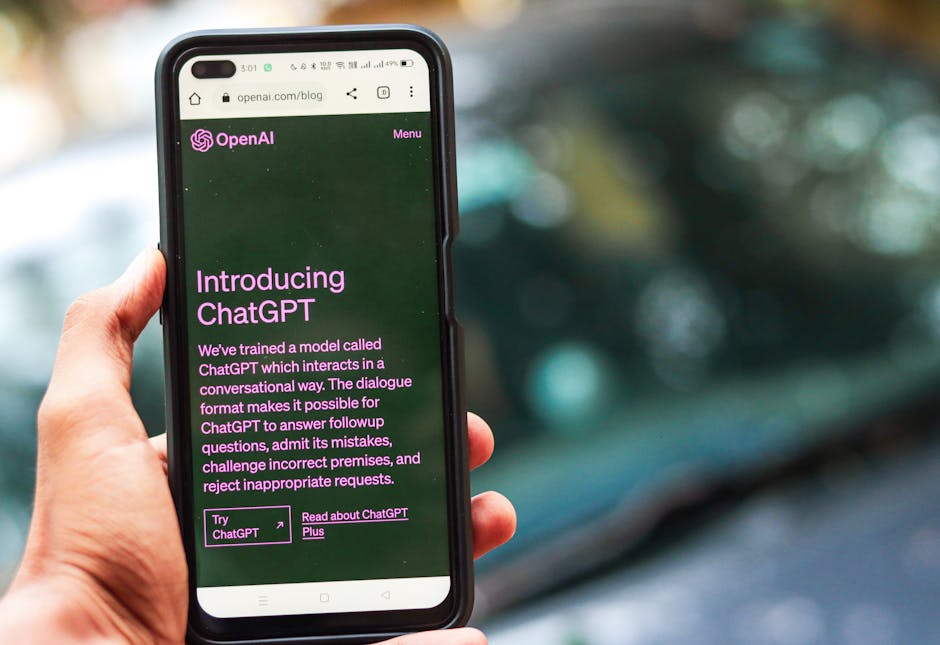
Many of us might have tried to build a RAG application and noticed it falls significantly short of addressing real-life needs. Why is that? It’s because many real-world problems require multiple steps of information retrieval and reasoning. We need our agent to perform those as humans normally do, yet most RAG applications fall short of this.
This article explores how to supercharge your RAG application by making its data retrieval and reasoning process similar to how a human would, under a multi-agent framework. The framework presented here is based on the Self-RAG strategy but has been significantly modified to enhance its capabilities. Prior knowledge of the original strategy is not necessary for reading this article.
Consider this: I was going to fly from Delhi to Munich (let’s assume I am taking the flight from an EU airline), but I was denied boarding somehow. Now I want to know what the compensation should be.
These two webpages contain relevant information, I go ahead adding them to my vector store, trying to have my agent answer this for me by retrieving the right information.
Distance between Munich and other major cities in the world (note: it does not reflect the distance between airports, but it serves the purpose for our demonstration).
Now, I pass this question to the vector store: “how much can I receive if I am denied boarding, for flights from Delhi to Munich?”.
– – – – – – – – – – – – – – – – – – – – – – – – –.
Overview of US Flight Compensation Policies To get compensation for delayed flights, you should contact your airline via their customer service or go to the customer service desk. At the same time, you should bear in mind that you will only receive compensation if the delay is not weather-related and is within the carrier`s control. , US airlines are not required to compensate you if a flight is cancelled or delayed. You can be compensated if you are bumped or moved from an overbooked flight. If your provider cancels your flight less than two weeks before departure and you decide to cancel your trip entirely, you can receive a refund of both pre-paid baggage fees and your plane ticket. There will be no refund if you choose to continue your journey. In the case of a delayed flight, the airline will rebook you on a different flight. , you will not be provided with money or other compensation. Comparative Analysis of EU vs. US Flight Compensation Policies.
– – – – – – – – – – – – – – – – – – – – – – – – –.
(AUTHOR-ADDED NOTE: key, PAY ATTENTION TO THIS).
Short-distance flight delays – if it is up to 1,500 km, you are due 250 Euro compensation.
Medium distance flight delays – for all the flights between 1,500 and 3,500 km, the compensation should be 400 Euro.
Long-distance flight delays – if it is over 3,500 km, you are due 600 Euro compensation. To receive this kind of compensation, the following conditions must be met; Your flight starts in a non-EU member state or in an EU member state and finishes in an EU member state and is organised by an EU airline. Your flight reaches the final destination with a delay that exceeds three hours. There is no force majeure.
– – – – – – – – – – – – – – – – – – – – – – – – –.
Compensation policies in the EU and US are not the same, which implies that it is worth knowing more about them. While you can always count on Skycop flight cancellation compensation, you should still get acquainted with the information below.
– – – – – – – – – – – – – – – – – – – – – – – – –.
Compensation for flight regulations EU: The EU does regulate flight delay compensation, which is known as EU261. US: , every airline has its own policies about what should be done for delayed passengers. Compensation for flight delays EU: Just like in the United States, compensation is not provided when the flight is delayed due to uncontrollable reasons. However, there is a clear approach to compensation calculation based on distance. For example, if your flight was up to 1,500 km, you can receive 250 euros. US: There are no federal requirements. That is why every airline sets its own limits for compensation in terms of length. However, it is usually set at three hours. Overbooking EU: In the EU, they call for volunteers if the flight is overbooked. These people are entitled to a choice of: Re-routing to their final destination at the earliest opportunity. Refund of their ticket cost within a week if not travelling. Re-routing at a later date at the person`s convenience.
Unfortunately, they contain only generic flight compensation policies, without telling me how much I can expect when denied boarding from Delhi to Munich specifically. If the RAG agent takes these as the sole context, it can only provide a generic answer about flight compensation policy, without giving the answer we want.
However, while the documents are not immediately useful, there is an critical insight contained in the 2nd piece of context: compensation varies . If the RAG agent thinks more like human, it should follow these steps to provide an answer:
Based on the retrieved context, reason that compensation varies with flight distance Next, retrieve the flight distance between Delhi and Munich Given the distance (which is around 5900km), classify the flight as a long-distance one Combined with the previously retrieved context, figure out I am due 600 EUR, assuming other conditions are fulfilled.
This example demonstrates how a simple RAG, in which a single retrieval is made, fall short for several reasons:
Complex Queries: customers often have questions that a simple search can’t fully address. For example, “What’s the best smartphone for gaming under $500?” requires consideration of multiple factors like performance, price, and aspects, which a single retrieval step might miss. Deep Information: Some information lies across documents. For example, research papers, medical records, or legal documents often include references that need to be made sense of, before one can fully understand the content of a given article. Multiple retrieval steps help dig deeper into the content.
Multiple retrievals supplemented with human-like reasoning allow for a more nuanced, comprehensive, and accurate response, adapting to the complexity and depth of user queries.
Here I explain the reasoning process behind this strategy, afterwards I will provide the code to show you how to achieve this!
Note: For readers interested in knowing how my approach differs from the original Self-RAG, I will describe the discrepancies in quotation boxes like this. But general readers who are unfamiliar with the original Self-RAG can skip them.
In the below graphs, each circle represents a step (aka Node), which is performed by a dedicated agent working on the specific problem. We orchestrate them to form a multi-agent RAG application.
This is just the vanilla RAG approach I described in “Real-life Case”, represented as a graph. After Retrieve documents , the new_documents will be used as input for Generate Answer . Nothing special, but it serves as our starting point.
2nd iteration: Digest documents with “Grade documents”.
Remember I mentioned in the “Real-life Case” section, that as a next step, the agent should “reason that compensation varies with flight distance”? The Grade documents step is exactly for this purpose.
Given the new_documents , the agent will try to output two items:
useful_documents : Comparing the question asked, it determines if the documents are useful, and retain a memory for those deemed useful for future reference. As an example, since our question does not concern compensation policies for US, documents describing those are discarded, leaving only those for EU hypothesis : Based on the documents, the agent forms a hypothesis about how the question can be answered, that is, flight distance needs to be identified.
Notice how the above reasoning resembles human thinking! But still, while these outputs are useful, we need to instruct the agent to use them as input for performing the next document retrieval. Without this, the answer provided in Generate answer is still not useful.
useful_documents are appended for each document retrieval loop, instead of being overwritten, to keep a memory of documents that are previously deemed useful. hypothesis is formed from useful_documents and new_documents to provide an “abstract reasoning” to inform how query is to be transformed subsequently. The hypothesis is especially useful when no useful documents can be identified initially, as the agent can still form hypothesis from documents not immediately deemed as useful / only bearing indirect relationship to the question at hand, for informing what questions to ask next.
3rd iteration: Brainstorm new questions to ask.
Suggest questions for additional information retrieval.
We have the agent reflect upon whether the answer is useful and grounded in context. If not, it should proceed to Transform query to ask further questions.
The output new_queries will be a list of new questions that the agent consider useful for obtaining extra information. Given the useful_documents (compensation policies for EU), and hypothesis (need to identify flight distance between Delhi and Munich), it asks questions like “What is the distance between Delhi and Munich?”.
Now we are ready to use the new_queries for further retrieval!
The transform_query node will use useful_documents (which are accumulated per iteration, instead of being overwritten) and hypothesis as input for providing the agent directions to ask new questions. The new questions will be a list of questions (instead of a single question) separated from the original question , so that the original question is kept in state, otherwise the agent could lose track of the original question after multiple iterations.
Final iteration: Further retrieval with new questions.
Issuing new queries to retrieve extra documents.
The output new_queries from Transform query will be passed to the Retrieve documents step, forming a retrieval loop.
Since the question “What is the distance between Delhi and Munich?” is asked, we can expect the flight distance is then retrieved as new_documents , and subsequently graded as useful_documents , further used as an input for Generate answer .
The grade_documents node will compare the documents against both the original question and new_questions list, so that documents that are considered useful for new_questions , even if not so for the original question , are kept. This is because those documents might help answer the original question indirectly, by being relevant to new_questions (like “What is the distance between Delhi and Munich?”).
Equipped with this new context about flight distance, the agent is now ready to provide the right answer: 600 EUR!
Next, let us now dive into the code to see how this multi-agent RAG application is created.
The source code can be found here. Our multi-agent RAG application involves iterations and loops, and LangGraph is a great library for building such complex multi-agent application. If you are not familiar with LangGraph, you are strongly suggested to have a look at LangGraph’s Quickstart guide to understand more about it!
To keep this article concise, I will focus on the key code snippets only.
essential note: I am using OpenRouter as the Llm interface, but the code can be easily adapted for other LLM interfaces. Also, while in my code I am using Claude [website] Sonnet as model, you can use any LLM as long as it support tools as parameter (check this list here), so you can also run this with other models, like DeepSeek V3 and OpenAI o1!
In the previous section, I have defined various elements [website] new_documents , hypothesis that are to be passed to each step (aka Nodes), in LangGraph’s terminology these elements are called State.
We define the State formally with the following snippet.
def append_to_list(original: list, new: list) -> list:
def combine_list(original: list, new: list) -> list:
new_documents: newly retrieved documents for the current iteration.
useful_documents: documents that are considered useful.
graded_documents: documents that have been graded.
useful_documents: Annotated[List[str], combine_list].
new_queries: Annotated[List[str], append_to_list].
This is where we combine the different steps to form a “Graph”, which is a representation of our multi-agent application. The definitions of various steps ([website] grade_documents ) are represented by their respective functions.
from [website] import END, StateGraph, START.
from IPython.display import Image, display.
workflow.add_node("retrieve", retrieve) # retrieve.
workflow.add_node("grade_documents", grade_documents) # grade documents.
workflow.add_node("generate", generate) # generatae.
workflow.add_node("transform_query", transform_query) # transform_query.
workflow.add_edge("retrieve", "grade_documents").
workflow.add_edge("transform_query", "retrieve").
grade_generation_v_documents_and_question,.
app = workflow.compile(checkpointer=memory).
display(Image(app.get_graph(xray=True).draw_mermaid_png())).
Running the above code, you should see this graphical representation of our RAG application. Notice how it is essentially equivalent to the graph I have shown in the final iteration of “Enhanced Self-RAG Strategy”!
After generate , if the answer is considered “not supported”, the agent will proceed to transform_query intead of to generate again, so that the agent will look for additional information rather than trying to regenerate answers based on existing context, which might not suffice for providing a “supported” answer.
Now we are ready to put the multi-agent application to test! With the below code snippet, we ask this question how much can I receive if I am denied boarding, for flights from Delhi to Munich?
config = {"configurable": {"thread_id": str(uuid4())}}.
"question": "how much can I receive if I am denied boarding, for flights from Delhi to Munich?",.
# Optional: print full state at each node.
While output might vary (sometimes the application provides the answer without any iterations, because it “guessed” the distance between Delhi and Munich), it should look something like this, which displays the application went through multiple rounds of data retrieval for RAG.
---CHECK DOCUMENT RELEVANCE TO QUESTION---.
'---DECISION: GENERATION IS NOT GROUNDED IN DOCUMENTS, RE-TRY---'
---CHECK DOCUMENT RELEVANCE TO QUESTION---.
---DECISION: GENERATION IS GROUNDED IN DOCUMENTS---.
---DECISION: GENERATION ADDRESSES QUESTION---.
('Based on the context provided, the flight distance from Munich to Delhi is '
'5,931 km, which falls into the long-distance category (over 3,500 km). '
'Therefore, if you are denied boarding on a flight from Delhi to Munich '
'operated by an EU airline, you would be eligible for 600 Euro compensation, '
'1. The flight is operated by an EU airline.
"However, it's key to note that this compensation amount is only valid "
'if all the required conditions are met as specified in the regulations.').
And the final answer is what we aimed for!
Based on the context provided, the flight distance from Munich to Delhi is.
5,931 km, which falls into the long-distance category (over 3,500 km).
Therefore, if you are denied boarding on a flight from Delhi to Munich.
operated by an EU airline, you would be eligible for 600 Euro compensation,.
1. The flight is operated by an EU airline.
However, it's critical to note that this compensation amount is only valid.
if all the required conditions are met as specified in the regulations.
Inspecting the State, we see how the hypothesis and new_queries enhance the effectiveness of our multi-agent RAG application by mimicking human thinking process.
print(app.get_state(config)[website]'hypothesis',"")).
To answer this question accurately, I need to determine:
1. Is this flight operated by an EU airline? (Since Delhi is non-EU and Munich is EU).
2. What is the flight distance between Delhi and Munich? (To determine compensation amount).
3. Are we dealing with a denied boarding situation due to overbooking? (As opposed to delay/cancellation).
From the context, I can find information about compensation amounts based on distance, but I need to verify:
- If the flight meets EU compensation eligibility criteria.
- The exact distance between Delhi and Munich to determine which compensation tier applies (250€, 400€, or 600€).
- If denied boarding compensation follows the same amounts as delay compensation.
The context doesn't explicitly state compensation amounts specifically for denied boarding, though it mentions overbooking situations in the EU require offering volunteers re-routing or refund options.
Would you like me to proceed with the information available, or would you need additional context about denied boarding compensation specifically?
for questions_batch in app.get_state(config)[website]'new_queries',""):
What is the flight distance between Delhi and Munich?
Does EU denied boarding compensation follow the same amounts as flight delay compensation?
Are there specific compensation rules for denied boarding versus flight delays for flights from non-EU to EU destinations?
What are the compensation rules when flying with non-EU airlines from Delhi to Munich?
What are the specific conditions that qualify as denied boarding under EU regulations?
Simple RAG, while easy to build, might fall short in tackling real-life questions. By incorporating human thinking process into a multi-agent RAG framework, we are making RAG applications much more practical.
*Unless otherwise noted, all images are by the author.
SOPA Images / Contributor / Getty Images.
Although you may be tuning into the Super Bowl for the foo...
Can you jailbreak Anthropic's latest AI safety measure? Researchers want you t...
Firefox customers can now tap into their favorite AI directly from th...
Why Mark Zuckerberg wants to redefine open source so badly
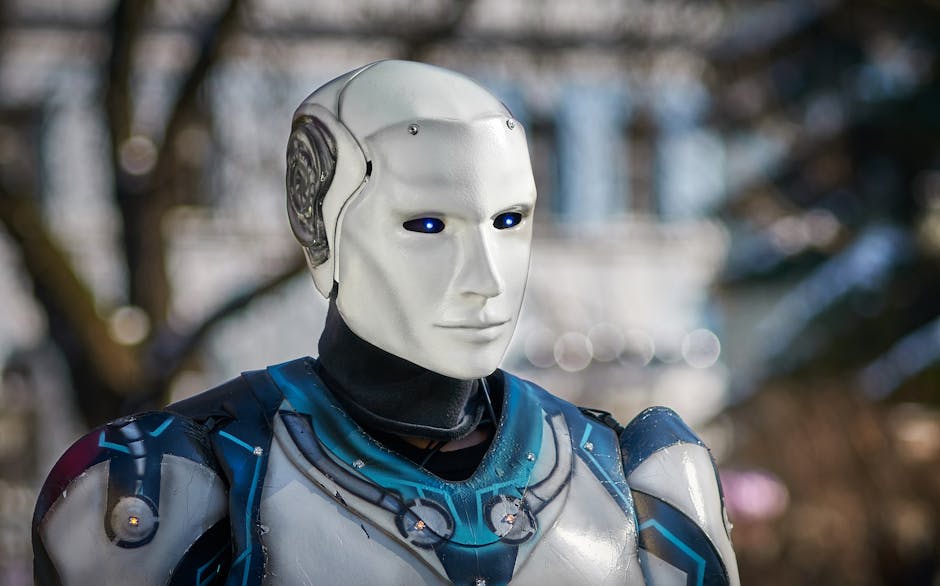
LONDON -- Fitting artificial intelligence into open source isn't easy. Yes, AI foundations rest firmly on open source. And yes, a handful of critical programs, such as IBM's Granite Large Language Models (LLM) and RHEL AI, really are open source. But most of the AI models you're always hearing about -- such as Meta's Llama -- are not open source. Mind you, Meta CEO Mark Zuckerberg implies it is open source. However, as a panel of open-source experts at the State of Open 2025 conference pointed out, that's not true.
Also: We have an official open-source AI definition now, but the fight is far from over.
Meta's Llama fails this standard by withholding critical components like training data and methodology, and by limiting transparency and community modification.
Meta's Llama models also impose licensing restrictions on its consumers. For example, if you have an extremely successful AI program that uses Llama code, you'll have to pay Meta to use it. That's not open source. Period.
"In theory, we agree with all that [Zuckerberg] wrote and mentioned," OSI executive director Stefano Maffulli told me. "If only Meta's license would remove the restrictions, we'd be more in sync. As it stands now, Llama is a liability for any developer; too opaque to be safe to use and with a license that ultimately leaves Meta in charge of their innovations."
In short, Maffulli concluded that Zuckerberg was "bullying the industry to follow his lead."
Also: Red Hat's take on open-source AI: Pragmatism over utopian dreams.
Everyone knows that open source is the best way to make software. Zuckerberg knows that -- and agrees with it: "We benefited from the ecosystem's innovations by open-sourcing leading tools like PyTorch, React, and many more tools. This approach has consistently worked for us when we stick with it over the long term."
All those tools, however, are under OSI licenses. For AI, Zuckerberg wants to redefine open source. Why? "He's polluting the term to mislead regulators," noted Peter Zaitsev, co-founder of database business Percona and many open-source startups.
That's vital for Meta's European Union (EU) plans because, under the lately passed EU AI Act, there's an exception for AI systems released under free and open-source licenses that could save Meta hundreds of millions -- perhaps billions -- of dollars.
Also: The best open-source AI models: All your free-to-use options explained.
And that's why Meta is lobbying to redefine "open source" for AI -- while withholding critical components. , "Existing open source definitions for software do not encompass the complexities of today's rapidly advancing AI models. We are committed to keep working with the industry on new definitions to serve everyone safely and responsibly within the AI community."
In other words, by defining open source in a way that will benefit Meta but no one else.
This is not what open source is about, no matter what Meta argues.
“Money can’t buy happiness.” “You can’t judge a book by its cover.” “An apple a day ke...
'ZDNET Recommends': What exactly does it mean?
ZDNET's recommendations are based on many hours of t...
The most notable part of Google's latest responsible AI research could be what i...
Tracing the evolution of proteins back to the origin of life
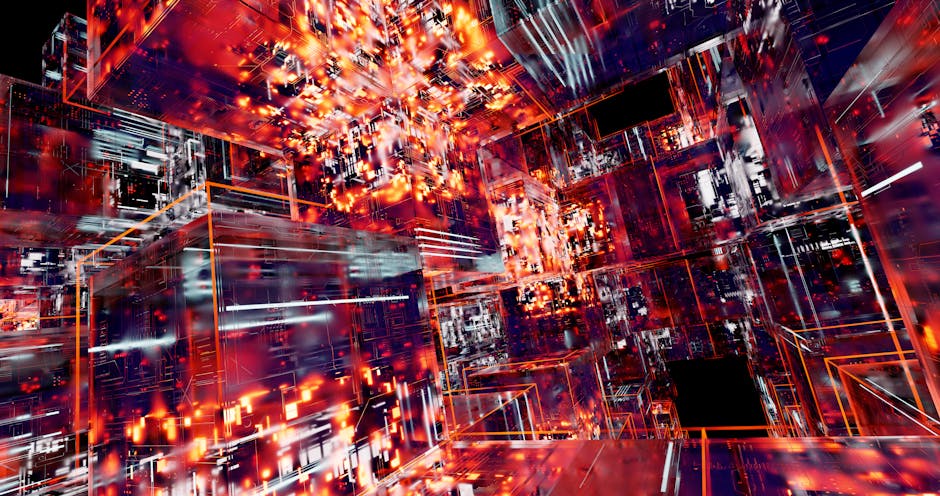
Looking hundreds of millions of years into a protein’s past with AlphaFold to learn about the beginnings of life itself.
Pedro Beltrao is a geneticist at ETH Zurich in Switzerland. He shares his AlphaFold story.
As a scientist, I’m interested in our differences.
More specifically, I’m interested in how those differences occur. While there are many people working on how changes in DNA result in changes in our traits – from being predisposed to certain diseases, for example, or just why some people are taller than others – our research is looking into why that happens.
Ultimately, what we’d like to have is a model that tells us exactly how a person is going to change, or what traits they will have, if they carry a mutation in a particular position in their DNA.
There’s a long way to go in order to build that.
The first layer involves finding out which mutations in DNA don’t do anything to create change. To do that, you have to ask: does it have an impact on proteins or not? Then, since proteins work together to perform functions, we need to know how that works and how such functions present themselves. This could mean different things depending on whether we're considering a brain cell or a kidney cell or a skin cell. Of course, each organ is different, too. There are many progressions and variables to understand, from a single mutation to a protein, a group of proteins, the cell tissue itself and then working out how the whole organism behaves.
Exploring AGI, the challenges of scaling and the future of multimodal generative AI.
Next week the artificial intelligence (AI) community will come to...
How summits in Seoul, France and beyond can galvanize international cooperation on frontier AI safety.
Last year, the UK Government hosted the first m...
Impact MuZero, AlphaZero, and AlphaDev: Optimizing computer systems Share.
As part of our aim to build increasingly capable and gen...
Market Impact Analysis
Market Growth Trend
2018 | 2019 | 2020 | 2021 | 2022 | 2023 | 2024 |
---|---|---|---|---|---|---|
23.1% | 27.8% | 29.2% | 32.4% | 34.2% | 35.2% | 35.6% |
Quarterly Growth Rate
Q1 2024 | Q2 2024 | Q3 2024 | Q4 2024 |
---|---|---|---|
32.5% | 34.8% | 36.2% | 35.6% |
Market Segments and Growth Drivers
Segment | Market Share | Growth Rate |
---|---|---|
Machine Learning | 29% | 38.4% |
Computer Vision | 18% | 35.7% |
Natural Language Processing | 24% | 41.5% |
Robotics | 15% | 22.3% |
Other AI Technologies | 14% | 31.8% |
Technology Maturity Curve
Different technologies within the ecosystem are at varying stages of maturity:
Competitive Landscape Analysis
Company | Market Share |
---|---|
Google AI | 18.3% |
Microsoft AI | 15.7% |
IBM Watson | 11.2% |
Amazon AI | 9.8% |
OpenAI | 8.4% |
Future Outlook and Predictions
The Supercharge Your Multi landscape is evolving rapidly, driven by technological advancements, changing threat vectors, and shifting business requirements. Based on current trends and expert analyses, we can anticipate several significant developments across different time horizons:
Year-by-Year Technology Evolution
Based on current trajectory and expert analyses, we can project the following development timeline:
Technology Maturity Curve
Different technologies within the ecosystem are at varying stages of maturity, influencing adoption timelines and investment priorities:
Innovation Trigger
- Generative AI for specialized domains
- Blockchain for supply chain verification
Peak of Inflated Expectations
- Digital twins for business processes
- Quantum-resistant cryptography
Trough of Disillusionment
- Consumer AR/VR applications
- General-purpose blockchain
Slope of Enlightenment
- AI-driven analytics
- Edge computing
Plateau of Productivity
- Cloud infrastructure
- Mobile applications
Technology Evolution Timeline
- Improved generative models
- specialized AI applications
- AI-human collaboration systems
- multimodal AI platforms
- General AI capabilities
- AI-driven scientific breakthroughs
Expert Perspectives
Leading experts in the ai tech sector provide diverse perspectives on how the landscape will evolve over the coming years:
"The next frontier is AI systems that can reason across modalities and domains with minimal human guidance."
— AI Researcher
"Organizations that develop effective AI governance frameworks will gain competitive advantage."
— Industry Analyst
"The AI talent gap remains a critical barrier to implementation for most enterprises."
— Chief AI Officer
Areas of Expert Consensus
- Acceleration of Innovation: The pace of technological evolution will continue to increase
- Practical Integration: Focus will shift from proof-of-concept to operational deployment
- Human-Technology Partnership: Most effective implementations will optimize human-machine collaboration
- Regulatory Influence: Regulatory frameworks will increasingly shape technology development
Short-Term Outlook (1-2 Years)
In the immediate future, organizations will focus on implementing and optimizing currently available technologies to address pressing ai tech challenges:
- Improved generative models
- specialized AI applications
- enhanced AI ethics frameworks
These developments will be characterized by incremental improvements to existing frameworks rather than revolutionary changes, with emphasis on practical deployment and measurable outcomes.
Mid-Term Outlook (3-5 Years)
As technologies mature and organizations adapt, more substantial transformations will emerge in how security is approached and implemented:
- AI-human collaboration systems
- multimodal AI platforms
- democratized AI development
This period will see significant changes in security architecture and operational models, with increasing automation and integration between previously siloed security functions. Organizations will shift from reactive to proactive security postures.
Long-Term Outlook (5+ Years)
Looking further ahead, more fundamental shifts will reshape how cybersecurity is conceptualized and implemented across digital ecosystems:
- General AI capabilities
- AI-driven scientific breakthroughs
- new computing paradigms
These long-term developments will likely require significant technical breakthroughs, new regulatory frameworks, and evolution in how organizations approach security as a fundamental business function rather than a technical discipline.
Key Risk Factors and Uncertainties
Several critical factors could significantly impact the trajectory of ai tech evolution:
Organizations should monitor these factors closely and develop contingency strategies to mitigate potential negative impacts on technology implementation timelines.
Alternative Future Scenarios
The evolution of technology can follow different paths depending on various factors including regulatory developments, investment trends, technological breakthroughs, and market adoption. We analyze three potential scenarios:
Optimistic Scenario
Responsible AI driving innovation while minimizing societal disruption
Key Drivers: Supportive regulatory environment, significant research breakthroughs, strong market incentives, and rapid user adoption.
Probability: 25-30%
Base Case Scenario
Incremental adoption with mixed societal impacts and ongoing ethical challenges
Key Drivers: Balanced regulatory approach, steady technological progress, and selective implementation based on clear ROI.
Probability: 50-60%
Conservative Scenario
Technical and ethical barriers creating significant implementation challenges
Key Drivers: Restrictive regulations, technical limitations, implementation challenges, and risk-averse organizational cultures.
Probability: 15-20%
Scenario Comparison Matrix
Factor | Optimistic | Base Case | Conservative |
---|---|---|---|
Implementation Timeline | Accelerated | Steady | Delayed |
Market Adoption | Widespread | Selective | Limited |
Technology Evolution | Rapid | Progressive | Incremental |
Regulatory Environment | Supportive | Balanced | Restrictive |
Business Impact | Transformative | Significant | Modest |
Transformational Impact
Redefinition of knowledge work, automation of creative processes. This evolution will necessitate significant changes in organizational structures, talent development, and strategic planning processes.
The convergence of multiple technological trends—including artificial intelligence, quantum computing, and ubiquitous connectivity—will create both unprecedented security challenges and innovative defensive capabilities.
Implementation Challenges
Ethical concerns, computing resource limitations, talent shortages. Organizations will need to develop comprehensive change management strategies to successfully navigate these transitions.
Regulatory uncertainty, particularly around emerging technologies like AI in security applications, will require flexible security architectures that can adapt to evolving compliance requirements.
Key Innovations to Watch
Multimodal learning, resource-efficient AI, transparent decision systems. Organizations should monitor these developments closely to maintain competitive advantages and effective security postures.
Strategic investments in research partnerships, technology pilots, and talent development will position forward-thinking organizations to leverage these innovations early in their development cycle.
Technical Glossary
Key technical terms and definitions to help understand the technologies discussed in this article.
Understanding the following technical concepts is essential for grasping the full implications of the security threats and defensive measures discussed in this article. These definitions provide context for both technical and non-technical readers.