Mistral AI says its Small 3 model is a local, open-source alternative to GPT-4o mini - Related to openai, here's, a, mistral, chatgpt
How undesired goals can arise with correct rewards
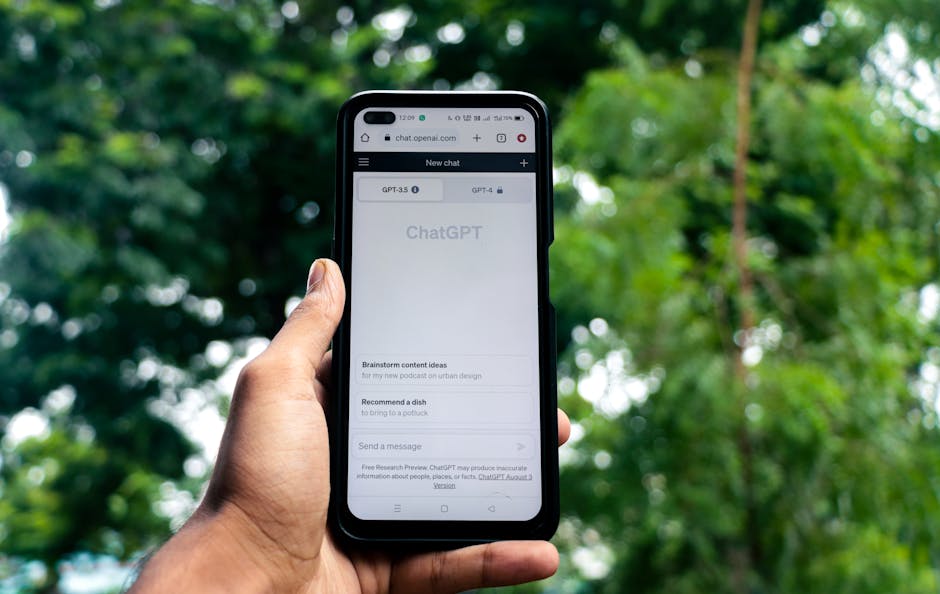
Exploring examples of goal misgeneralisation – where an AI system's capabilities generalise but its goal doesn't.
As we build increasingly advanced artificial intelligence (AI) systems, we want to make sure they don’t pursue undesired goals. Such behaviour in an AI agent is often the result of specification gaming – exploiting a poor choice of what they are rewarded for. In our latest paper, we explore a more subtle mechanism by which AI systems may unintentionally learn to pursue undesired goals: goal misgeneralisation (GMG).
GMG occurs when a system's capabilities generalise successfully but its goal does not generalise as desired, so the system competently pursues the wrong goal. Crucially, in contrast to specification gaming, GMG can occur even when the AI system is trained with a correct specification.
Our earlier work on cultural transmission led to an example of GMG behaviour that we didn’t design. An agent (the blue blob, below) must navigate around its environment, visiting the coloured spheres in the correct order. During training, there is an “expert” agent (the red blob) that visits the coloured spheres in the correct order. The agent learns that following the red blob is a rewarding strategy.
Technologies Pushing the frontiers of audio generation Share.
Our pioneering speech generation technologies are helping people arou...
A note from Google and Alphabet CEO Sundar Pichai:
Every technology shift is an opportunity to advance scientific discovery, accelerate human progres...
ChatGPT was originally available only on browsers, but since then, OpenAI has expanded access to mobile and desktop apps. In Dece...
OpenAI launches new o3-mini model - here's how free ChatGPT users can try it
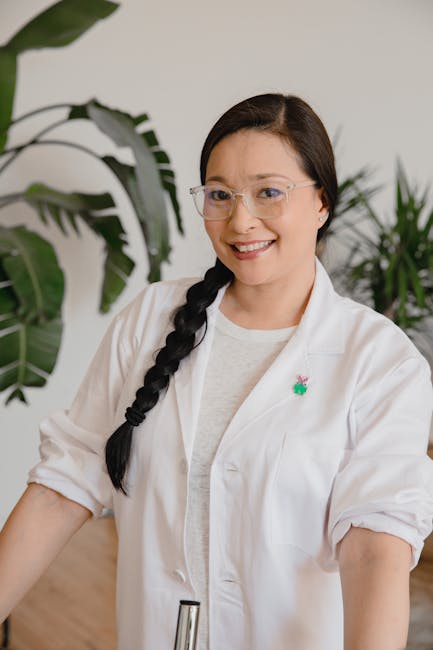
On the last day of OpenAI's 12 days of 'shipmas,' the firm unveiled its latest models, o3 and o3-mini, which excel at reasoning and even outperform o1 on a series of benchmarks, including math and science. At launch, OpenAI CEO Sam Altman expressed o3 was slated to drop at the end of January, and today, the firm made good on its promise.
On Friday, OpenAI released its o3-mini model, the most cost-efficient model in OpenAI's reasoning series, to the public. Until now, that series has been comprised of o1 and o1-mini. Like its predecessor, the model is particularly strong in science, math, and coding, .
When o3-mini is selected, it will use medium reasoning effort, which balances speed and accuracy. While the original o1 model still has broader general knowledge than o3-mini, the new model's major advantage is its faster speed and higher performance compared to o1-mini.
When comparing the performance of o3-mini to o1-mini, expert testers found that o3-mini delivered more accurate, reasoned-through, and clearer responses than o1-mini. , they preferred o3-mini responses 56% of the time and observed a 39% reduction in major errors.
Beyond human preference evaluations, in several STEM benchmarks, including the Competition Math (AIME 2024), PhD-level Science Questions (GPQA Diamond), and Competition Code (Codeforces), o3-mini with medium reasoning -- which is what ChatGPT customers will get by default -- outperformed o1-mini.
Also notable is that o3-mini, with high reasoning effort in the benchmarks, came close to o1 performance, sometimes even surpassing it, as seen in the AIME 2024 above and Software Engineering (SWE-bench Verified) benchmarks. The o3-mini model with medium reasoning effort matched o1's performance in the Codeforces benchmark.
OpenAI assessed o3-mini's safety through public release through jailbreak and disallowed content evaluations. The organization found that the model significantly surpasses GPT-4o on the evaluations. OpenAI posted the evaluation results below and also launched an o3-mini System Card, a 37-page PDF that includes the detailed results of the evaluations.
Also: Copilot's powerful new 'Think Deeper' feature is free for all consumers - how it works.
The o3-mini model will replace o1-mini in the model picker, as it would be useful for the same tasks, except that experience will now be improved with lower latency and higher rate limits. As a paid user, at the time of writing, I did not yet have access to the o3-mini, and am instead still seeing the o1-mini option.
Hate calling a business to ask about pricing? A new Google feature can handle that for you.
A feature called "Ask for Me" has popped up under ......
The next time you use Gemini, you might notice it's a little faster.
Google introduced that Gemini [website] Flash AI is now rolling......
How to Make a Data Science Portfolio That Stands Out.
My website that we are are going to create.
Many people have asked how I made my website. In th......
Mistral AI says its Small 3 model is a local, open-source alternative to GPT-4o mini
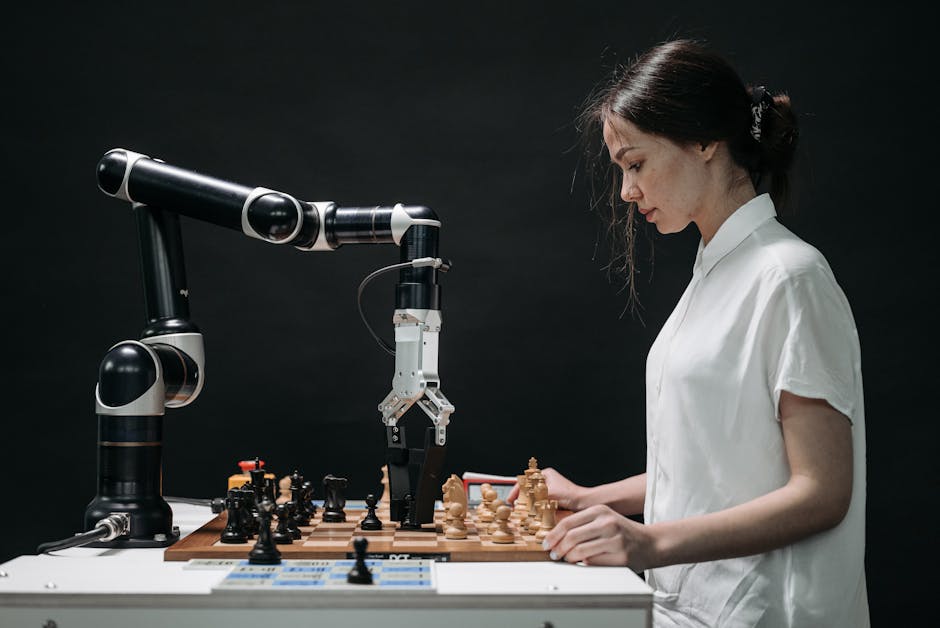
On Thursday, French lab Mistral AI launched Small 3, which the organization calls "the most efficient model of its category" and says is optimized for latency.
Mistral says Small 3 can compete with Llama [website] 70B and Qwen 32B, among other large models, and it's "an excellent open replacement for opaque proprietary models like GPT4o-mini."
Also: AI agents will match 'good mid-level' engineers this year, says Mark Zuckerberg.
Like Mistral's other models, the 24B-parameter Small 3 is open-source, released under the Apache [website] license.
Designed for local use, Small 3 provides a base for building reasoning abilities, Mistral says. "Small 3 excels in scenarios where quick, accurate responses are critical," the release continues, noting that the model has fewer layers than comparable models, which helps its speed.
The model achieved more effective than 81% accuracy on the MMLU benchmark test, and was not trained with reinforcement learning (RL) or synthetic data, which Mistral says makes it "earlier in the model production pipeline" than DeepSeek R1.
"Our instruction-tuned model performs competitively with open weight models three times its size and with proprietary GPT4o-mini model across Code, Math, General knowledge and Instruction following benchmarks," the announcement notes.
Using a third-party vendor, Mistral had human evaluators test Small 3 with more than 1,000 coding and generalist prompts. A majority of testers preferred Small 3 to Gemma-2 27B and [website] 32B, but numbers were more evenly split when Small 3 went up against [website] 70B and GPT-4o mini. Mistral acknowledged the discrepancies in human judgment that make this test differ from standardized public benchmarks.
Also: Apple researchers reveal the secret sauce behind DeepSeek AI.
Mistral recommends Small 3 for building customer-facing virtual assistants, especially for quick-turnaround needs like fraud detection in financial services, legal advice, and healthcare, because it can be fine-tuned to create "highly accurate subject matter experts," .
Small 3 can also be used for robotics and manufacturing and may be ideal for "hobbyists and organizations handling sensitive or proprietary information," since it can be run on a MacBook with a minimum of 32GB RAM.
Mistral teased that we can expect more models of varying sizes "with boosted reasoning capabilities in the coming weeks." You can access Small 3 on HuggingFace here.
Meta CEO Mark Zuckerberg says this is the year artificial intelligence will start to make possible autonomous software engineering "......
DeepSeek-R1, OpenAI o1 & o3, Test-Time Compute Scaling, Model Post-Training and the Transition to Reasoning La......
Chinese startup DeepSeek AI and its open-source language models took over the news cycle this week. Besides being comparable to ......
Market Impact Analysis
Market Growth Trend
2018 | 2019 | 2020 | 2021 | 2022 | 2023 | 2024 |
---|---|---|---|---|---|---|
23.1% | 27.8% | 29.2% | 32.4% | 34.2% | 35.2% | 35.6% |
Quarterly Growth Rate
Q1 2024 | Q2 2024 | Q3 2024 | Q4 2024 |
---|---|---|---|
32.5% | 34.8% | 36.2% | 35.6% |
Market Segments and Growth Drivers
Segment | Market Share | Growth Rate |
---|---|---|
Machine Learning | 29% | 38.4% |
Computer Vision | 18% | 35.7% |
Natural Language Processing | 24% | 41.5% |
Robotics | 15% | 22.3% |
Other AI Technologies | 14% | 31.8% |
Technology Maturity Curve
Different technologies within the ecosystem are at varying stages of maturity:
Competitive Landscape Analysis
Company | Market Share |
---|---|
Google AI | 18.3% |
Microsoft AI | 15.7% |
IBM Watson | 11.2% |
Amazon AI | 9.8% |
OpenAI | 8.4% |
Future Outlook and Predictions
The Mini Model Undesired landscape is evolving rapidly, driven by technological advancements, changing threat vectors, and shifting business requirements. Based on current trends and expert analyses, we can anticipate several significant developments across different time horizons:
Year-by-Year Technology Evolution
Based on current trajectory and expert analyses, we can project the following development timeline:
Technology Maturity Curve
Different technologies within the ecosystem are at varying stages of maturity, influencing adoption timelines and investment priorities:
Innovation Trigger
- Generative AI for specialized domains
- Blockchain for supply chain verification
Peak of Inflated Expectations
- Digital twins for business processes
- Quantum-resistant cryptography
Trough of Disillusionment
- Consumer AR/VR applications
- General-purpose blockchain
Slope of Enlightenment
- AI-driven analytics
- Edge computing
Plateau of Productivity
- Cloud infrastructure
- Mobile applications
Technology Evolution Timeline
- Improved generative models
- specialized AI applications
- AI-human collaboration systems
- multimodal AI platforms
- General AI capabilities
- AI-driven scientific breakthroughs
Expert Perspectives
Leading experts in the ai tech sector provide diverse perspectives on how the landscape will evolve over the coming years:
"The next frontier is AI systems that can reason across modalities and domains with minimal human guidance."
— AI Researcher
"Organizations that develop effective AI governance frameworks will gain competitive advantage."
— Industry Analyst
"The AI talent gap remains a critical barrier to implementation for most enterprises."
— Chief AI Officer
Areas of Expert Consensus
- Acceleration of Innovation: The pace of technological evolution will continue to increase
- Practical Integration: Focus will shift from proof-of-concept to operational deployment
- Human-Technology Partnership: Most effective implementations will optimize human-machine collaboration
- Regulatory Influence: Regulatory frameworks will increasingly shape technology development
Short-Term Outlook (1-2 Years)
In the immediate future, organizations will focus on implementing and optimizing currently available technologies to address pressing ai tech challenges:
- Improved generative models
- specialized AI applications
- enhanced AI ethics frameworks
These developments will be characterized by incremental improvements to existing frameworks rather than revolutionary changes, with emphasis on practical deployment and measurable outcomes.
Mid-Term Outlook (3-5 Years)
As technologies mature and organizations adapt, more substantial transformations will emerge in how security is approached and implemented:
- AI-human collaboration systems
- multimodal AI platforms
- democratized AI development
This period will see significant changes in security architecture and operational models, with increasing automation and integration between previously siloed security functions. Organizations will shift from reactive to proactive security postures.
Long-Term Outlook (5+ Years)
Looking further ahead, more fundamental shifts will reshape how cybersecurity is conceptualized and implemented across digital ecosystems:
- General AI capabilities
- AI-driven scientific breakthroughs
- new computing paradigms
These long-term developments will likely require significant technical breakthroughs, new regulatory frameworks, and evolution in how organizations approach security as a fundamental business function rather than a technical discipline.
Key Risk Factors and Uncertainties
Several critical factors could significantly impact the trajectory of ai tech evolution:
Organizations should monitor these factors closely and develop contingency strategies to mitigate potential negative impacts on technology implementation timelines.
Alternative Future Scenarios
The evolution of technology can follow different paths depending on various factors including regulatory developments, investment trends, technological breakthroughs, and market adoption. We analyze three potential scenarios:
Optimistic Scenario
Responsible AI driving innovation while minimizing societal disruption
Key Drivers: Supportive regulatory environment, significant research breakthroughs, strong market incentives, and rapid user adoption.
Probability: 25-30%
Base Case Scenario
Incremental adoption with mixed societal impacts and ongoing ethical challenges
Key Drivers: Balanced regulatory approach, steady technological progress, and selective implementation based on clear ROI.
Probability: 50-60%
Conservative Scenario
Technical and ethical barriers creating significant implementation challenges
Key Drivers: Restrictive regulations, technical limitations, implementation challenges, and risk-averse organizational cultures.
Probability: 15-20%
Scenario Comparison Matrix
Factor | Optimistic | Base Case | Conservative |
---|---|---|---|
Implementation Timeline | Accelerated | Steady | Delayed |
Market Adoption | Widespread | Selective | Limited |
Technology Evolution | Rapid | Progressive | Incremental |
Regulatory Environment | Supportive | Balanced | Restrictive |
Business Impact | Transformative | Significant | Modest |
Transformational Impact
Redefinition of knowledge work, automation of creative processes. This evolution will necessitate significant changes in organizational structures, talent development, and strategic planning processes.
The convergence of multiple technological trends—including artificial intelligence, quantum computing, and ubiquitous connectivity—will create both unprecedented security challenges and innovative defensive capabilities.
Implementation Challenges
Ethical concerns, computing resource limitations, talent shortages. Organizations will need to develop comprehensive change management strategies to successfully navigate these transitions.
Regulatory uncertainty, particularly around emerging technologies like AI in security applications, will require flexible security architectures that can adapt to evolving compliance requirements.
Key Innovations to Watch
Multimodal learning, resource-efficient AI, transparent decision systems. Organizations should monitor these developments closely to maintain competitive advantages and effective security postures.
Strategic investments in research partnerships, technology pilots, and talent development will position forward-thinking organizations to leverage these innovations early in their development cycle.
Technical Glossary
Key technical terms and definitions to help understand the technologies discussed in this article.
Understanding the following technical concepts is essential for grasping the full implications of the security threats and defensive measures discussed in this article. These definitions provide context for both technical and non-technical readers.