75% Indian Enterprises Face Losses Due to SaaS Implementation Delays: IDC-Zoho Study - Related to data, millions, study, gartner, implementation
75% Indian Enterprises Face Losses Due to SaaS Implementation Delays: IDC-Zoho Study
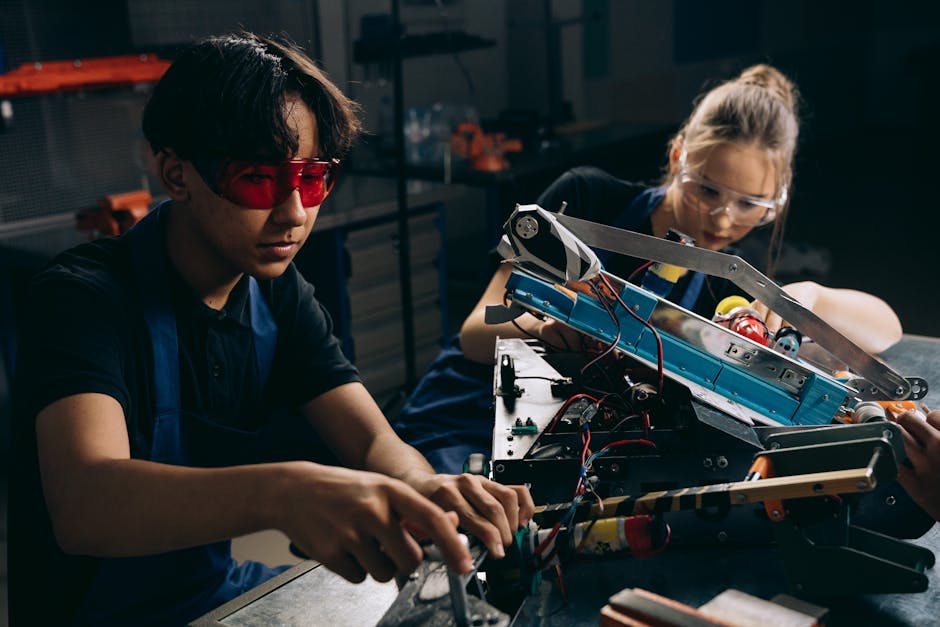
Indian enterprises adopting software-as-a-service (SaaS) solutions are encountering significant implementation delays, resulting in rising costs and missed business opportunities, .
The analysis, titled ‘IDC State of SaaS Adoption in India Survey 2024’, revealed that 75% of enterprises implementing SaaS since 2020 have faced timeline overruns averaging 57%, with cost escalations reaching 43%.
These setbacks have led to an estimated average loss of ₹[website] crore per business due to missed opportunities, while also impacting productivity, customer satisfaction, and competitive positioning.
Highlighting the critical need for streamlined SaaS implementation, Sharath Srinivasamurthy, associate vice president of IDC India, stated, “The ability to deploy SaaS solutions efficiently is no longer just an IT priority, it is a business necessity. Long deployment cycles escalate costs, slow down innovation, and reduce market responsiveness. Enterprises need a strategic approach, one that integrates automation, contextual intelligence, and development tools, to accelerate implementation and unlock SaaS value faster.”.
Zoho CEO Mani Vembu echoed this sentiment, emphasising the importance of reducing deployment timelines.
“Our platform-first approach eliminates common implementation bottlenecks by offering deeply integrated applications, low-code extensibility, and AI-powered automation. This enables businesses to deploy solutions and go live faster, reduce implementation risks, and accelerate their digital transformation efforts,” he revealed.
Financial Impact and Business Performance.
The study highlighted the far-reaching consequences of SaaS implementation delays. While [website] of enterprises acknowledge the importance of timely deployment, 67% reported increased costs due to extended timelines.
Moreover, 53% saw digital transformation efforts hindered, 48% noted customer dissatisfaction, and 46% suffered missed revenue opportunities, directly affecting overall business performance.
The financial burden was particularly pronounced in financial and accounting (F&A) solutions, which recorded the highest cost overruns at 60%. With 66% of enterprises deploying these solutions, delays disrupted crucial processes such as invoicing and payment processing, leading to penalties and compliance risks.
Customer experience solutions were the most widely adopted post-pandemic (87%), experiencing an average timeline overrun of 51%. However, email and collaboration solutions recorded the highest delays at 68%, followed by legal solutions at 61%.
Key reasons for implementation delays included project management inefficiencies (47%), integration and security challenges (38%), talent shortages (38%), and technical complexities (38%).
The study mentioned how different industries have been affected by SaaS implementation delays. In the healthcare sector, 63% of enterprises reported cost increases, while 58% faced reduced productivity and customer dissatisfaction. In financial services, 75% of enterprises encountered rising costs, with 60% reporting disruptions in digital transformation and 55% experiencing customer dissatisfaction.
In the manufacturing sector, 72% of enterprises faced cost overruns, while 41% reported revenue losses. Retail businesses also struggled, with 61% reporting revenue losses, 58% facing higher costs, and 51% seeing declines in productivity.
Transitioning to a Platform-First Approach.
To overcome these challenges, enterprises are increasingly adopting a platform-driven approach to SaaS implementation. This strategy adopts automation, reduces customisation efforts, and ensures seamless integration.
The study found that 59% of enterprises consider automation and DevOps essential for accelerating deployments. Automated workflows help minimise manual dependencies, decrease errors, and improve implementation speed.
Furthermore, 53% of enterprises highlighted the role of integrated developer platforms with low-code, no-code, and pro-code capabilities in overcoming customisation bottlenecks. These platforms allow business consumers and IT teams to build and modify applications quickly, reducing deployment delays. Another 48% stressed the importance of plug-and-play solutions that simplify integrations and system connectivity.
“Businesses cannot afford to be slowed down by complex and fragmented implementations. Zoho’s platform architecture ensures enterprises have the flexibility to implement solutions at scale with speed and minimal disruption,” Vembu added.
He noted that by offering pre-configured industry workflows, AI-driven analytics, and seamless interoperability, organisations can accelerate their SaaS deployments while minimising costs and maximising business impact.
Accenture has strengthened its AI capabilities with the acquisition of Danish AI firm Halfspace and an investment in AI-driven analytics firm Aaru.......
Une nouvelle fuite de données frappe La Poste. Cela met d’ailleurs en vente près de 50 000 informations sensibles. Cette cyberattaque soulève des ques......
If you're an Android user, you are familiar with Gemini, as it has replaced Google Assistant as the default.
How Confluent is Helping Swiggy Deliver Millions of Orders Daily
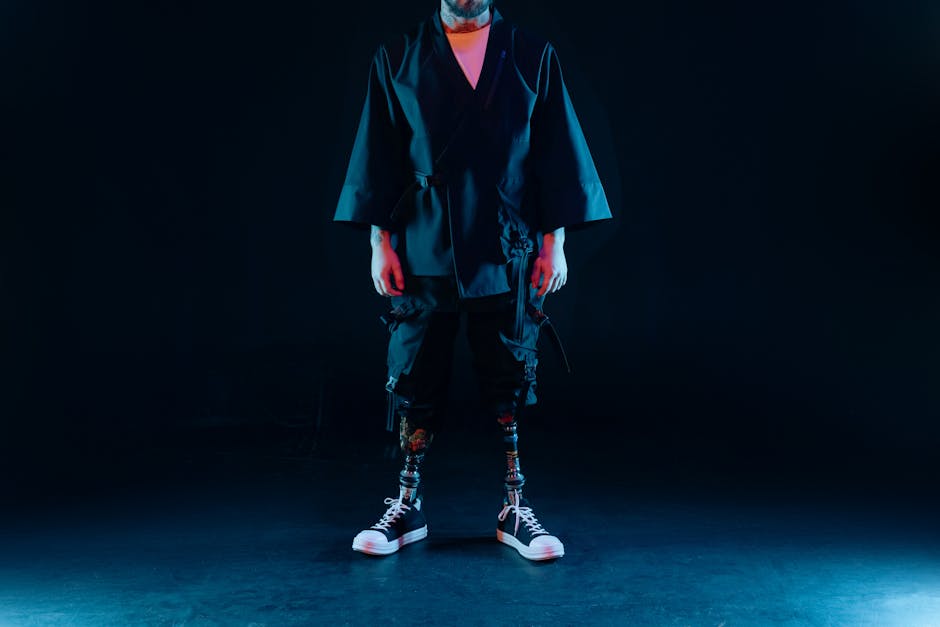
Food and grocery delivery platform Swiggy handles billions of orders annually across approximately 700 cities. Managing such a scale requires real-time coordination between restaurants, delivery partners, and end-clients. This is where data streaming platform Confluent steps in, supporting Swiggy’s massive data flow for seamless order processing.
As the , Confluent has been supporting Swiggy for over three years now.
At its core, Confluent’s platform is built on Apache Kafka, facilitating real-time data streaming. Notably, Confluent was founded in 2014 by Jay Kreps, Neha Narkhede, and Jun Rao, the original creators of Apache Kafka.
“If Confluent is down, Swiggy is down,” Rohit Vyas, country presales leader of India at Confluent, told AIM in an . He emphasised the platform’s role in Swiggy’s infrastructure.
Data streaming platform Confluent has been in the space for over a decade, catering to more than 5,000 enterprises.
“We are the second fastest software firm to reach a billion dollars in revenue,” noted Rubal Sahni, area vice president and country manager at Confluent, to AIM.
Catering to different domains, Confluent in the recent past partnered with Jio Platforms to integrate its data streaming platform with JioCloud Services. Another major customer is Swiggy, where real-time data processing is mission-critical.
Swiggy has processed over 3 billion orders, requiring a system that can scale dynamically. Initially, Swiggy relied on open-source Apache Kafka for its data streaming needs. However, as order volumes surged, maintaining an in-house Kafka setup became resource-intensive for Swiggy’s engineering teams.
This led to the transition to Confluent’s fully managed service, which provides real-time data streaming while reducing operational overhead. This move not only enabled Swiggy to streamline operations, and handle order surges efficiently, but also optimise resource allocation during high-demand periods such as festivals.
“So when they scaled up in a hyper scaling manner and their customer base began to bulge on, they realised that babysitting open source Kafka was draining a lot of their engineering talent. Then they approached Confluent,” Vyas explained. “What we enable for them is a multitude and a multi-dimensional aspect.”.
Confluent plays a critical role in enabling Swiggy to track order progress in real time, ensuring transparency at every stage. By ingesting vast amounts of data and processing it within milliseconds, the platform updates order statuses, estimated delivery times, and driver locations without delays. “If Confluent is down, apparently, Swiggy is down,” Vyas expressed.
From the moment a customer places an order, Confluent’s data streaming platform ensures that every step, from restaurant confirmation to assigning a delivery partner, happens seamlessly.
This entire complex system of fulfillment is happening over Confluent, making real-time updates possible, Vyas explained. The platform dynamically maps delivery partners, estimates preparation and packing times, and continuously syncs this information with Swiggy’s app.
Beyond scaling, ensuring security and governance is another challenge. Swiggy processes massive volumes of customer data, making compliance and security a top priority. Confluent provides built-in governance and monitoring tools, helping Swiggy maintain regulatory compliance while securing sensitive information.
Beyond logistics, Swiggy utilises AI-driven analytics powered by real-time data streaming. Predictive algorithms help optimise delivery routes and inventory management. “Swiggy Instamart tracks high-demand items in specific locations, ensuring superior stock availability in dark stores,” Vyas further stated.
As Confluent continues to expand its presence in India, the enterprise is focusing on scaling its industry reach and strengthening partnerships. “We have scaled our teams in India. We have opened newer industry verticals. We have invested in the public sector,” Sahni presented. The recent partnership with Jio Platforms aims to support public sector organisations with infrastructure for GenAI applications.
Beyond public sector expansion, Confluent is also increasing its footprint across industries such as healthcare, manufacturing, and tier-two markets. “We are adding more resources to cater to newer industry verticals, not just metro cities but also down south and up north,” Sahni added. To further strengthen its presence, Confluent is working closely with hyperscalers like AWS, Azure, and Google Cloud while expanding partnerships with enterprises looking for scalable data solutions.
The organization will continue to cater to markets of different sizes, from startups to large-scale enterprises. “We have various offerings depending on the size of the customer and nature of use cases,” Sahni mentioned.
The ability to analyze and interpret data is no longer limited to data scientists or highly skilled analysts. With the launch of Datalyze, MachineHack......
Une nouvelle fuite de données frappe La Poste. Cela met d’ailleurs en vente près de 50 000 informations sensibles. Cette cyberattaque soulève des ques......
Hugging Face, one of the most sought-out platforms to host AI models, revealed a partnership with software supply chain platform JFrog to improve sec......
Gartner identifies top trends in data and analytics for 2025 - and AI takes the lead
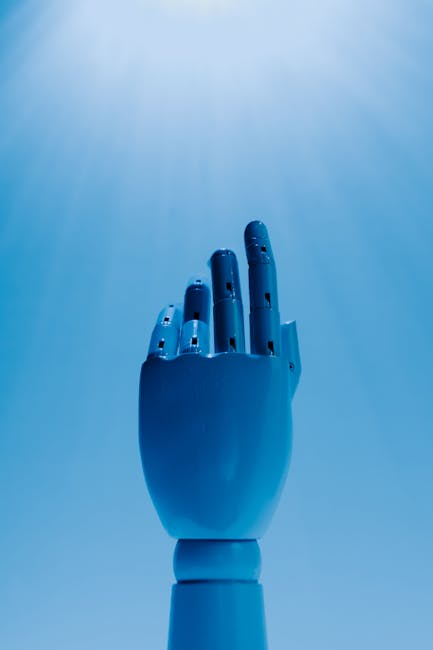
Data is at the heart of most organizations, fueling everyday business functions. To help digital leaders better prepare their data and analytics (D&A) strategies, Gartner has identified the top D&A trends for 2025.
"D&A is going from the domain of the few, to ubiquity. At the same time D&A leaders are under pressure not to do more with less, but to do a lot more with a lot more, and that can be even more challenging because the stakes are being raised," said Gareth Herschel, VP analyst at Gartner. "There are certain trends that will help D&A leaders meet the pressures, expectations and demands they are facing."
Also: 5 easy Gemini settings tweaks to protect your privacy from AI.
Out of the nine trends Gartner identified, unsurprisingly, AI-related technologies made up more than half of the list, including the biggest AI trend of the time -- agents. Agentic AI has begun permeating every business sector, with organizations finding ways to implement the autonomous assistance that AI agents offer.
Gartner advised D&A leaders to use agents to access and share their organization's data across applications. The analyst also recommended that D&A leaders use AI agents to automate closed-loop business outcomes, where data-driven insights continuously inform and optimize decisions.
Also: 4 ways to get your business ready for the agentic AI revolution.
When generative AI first became mainstream, the focus for many D&A leaders and their organizations was developing and implementing large language models (LLMs).
However, greater emphasis has since been placed on the value of small language models (SLMs). These small models are lightweight, tailored, cheaper, and faster to train, which is better for specific use cases. As a result, Gartner advised D&A leaders to consider SLMs for more accurate and contextually appropriate AI outputs.
Also: Opera unveils impressive preview of AI agentic browsing - see it in action.
As there are so many different tools that D&A leaders can use, Gartner also recommended composite AI, which is the process of leveraging multiple AI techniques to increase technological effectiveness. This approach means exploring technology beyond generative AI and LLMs to take a deeper look at related disciplines, such as machine learning and data science.
Some of the trends that Gartner identified are indirectly related to AI. For example, the analyst encouraged using synthetic data to supplement areas where insight is missing or incomplete. This approach is especially valuable when using data for AI initiatives, as these projects require organized, complete data foundations for training and deployment. Another advantage of synthetic data is that it can replace sensitive data, prioritizing privacy, which is especially essential for AI.
Building on this conception, Gartner identified metadata management solutions as an imperative trend, advising organizations to implement tools that automate finding and analyzing metadata. The analyst expressed various metadata types, including technical and business metadata, can then be used for data catalogs, data lineage, and AI-driven use cases. In its multimodal data fabric trend, Gartner advised collecting and analyzing information at the metadata stage of the data pipeline.
Also: 25% of enterprises using AI will deploy AI agents by 2025.
Other key trends highlighted by Gartner include decision intelligence platforms, which help organizations shift from simply using data to making smarter, decision-focused strategies. The analyst expressed this shift is critical to success.
Gartner also pointed to highly consumable data products as a trend, emphasizing the need for organizations to create useful, reusable data products that different teams can access to optimize and improve business-critical use cases over time.
AI coding tools have been both a boon and a bane for software engineers and developers. While many have managed to upskill themselves with AI and use ......
AI agents are revolutionizing the workplace by performing actions autonomously for workers and, in turn, str......
Market Impact Analysis
Market Growth Trend
2018 | 2019 | 2020 | 2021 | 2022 | 2023 | 2024 |
---|---|---|---|---|---|---|
23.1% | 27.8% | 29.2% | 32.4% | 34.2% | 35.2% | 35.6% |
Quarterly Growth Rate
Q1 2024 | Q2 2024 | Q3 2024 | Q4 2024 |
---|---|---|---|
32.5% | 34.8% | 36.2% | 35.6% |
Market Segments and Growth Drivers
Segment | Market Share | Growth Rate |
---|---|---|
Machine Learning | 29% | 38.4% |
Computer Vision | 18% | 35.7% |
Natural Language Processing | 24% | 41.5% |
Robotics | 15% | 22.3% |
Other AI Technologies | 14% | 31.8% |
Technology Maturity Curve
Different technologies within the ecosystem are at varying stages of maturity:
Competitive Landscape Analysis
Company | Market Share |
---|---|
Google AI | 18.3% |
Microsoft AI | 15.7% |
IBM Watson | 11.2% |
Amazon AI | 9.8% |
OpenAI | 8.4% |
Future Outlook and Predictions
The Indian Enterprises Face landscape is evolving rapidly, driven by technological advancements, changing threat vectors, and shifting business requirements. Based on current trends and expert analyses, we can anticipate several significant developments across different time horizons:
Year-by-Year Technology Evolution
Based on current trajectory and expert analyses, we can project the following development timeline:
Technology Maturity Curve
Different technologies within the ecosystem are at varying stages of maturity, influencing adoption timelines and investment priorities:
Innovation Trigger
- Generative AI for specialized domains
- Blockchain for supply chain verification
Peak of Inflated Expectations
- Digital twins for business processes
- Quantum-resistant cryptography
Trough of Disillusionment
- Consumer AR/VR applications
- General-purpose blockchain
Slope of Enlightenment
- AI-driven analytics
- Edge computing
Plateau of Productivity
- Cloud infrastructure
- Mobile applications
Technology Evolution Timeline
- Improved generative models
- specialized AI applications
- AI-human collaboration systems
- multimodal AI platforms
- General AI capabilities
- AI-driven scientific breakthroughs
Expert Perspectives
Leading experts in the ai tech sector provide diverse perspectives on how the landscape will evolve over the coming years:
"The next frontier is AI systems that can reason across modalities and domains with minimal human guidance."
— AI Researcher
"Organizations that develop effective AI governance frameworks will gain competitive advantage."
— Industry Analyst
"The AI talent gap remains a critical barrier to implementation for most enterprises."
— Chief AI Officer
Areas of Expert Consensus
- Acceleration of Innovation: The pace of technological evolution will continue to increase
- Practical Integration: Focus will shift from proof-of-concept to operational deployment
- Human-Technology Partnership: Most effective implementations will optimize human-machine collaboration
- Regulatory Influence: Regulatory frameworks will increasingly shape technology development
Short-Term Outlook (1-2 Years)
In the immediate future, organizations will focus on implementing and optimizing currently available technologies to address pressing ai tech challenges:
- Improved generative models
- specialized AI applications
- enhanced AI ethics frameworks
These developments will be characterized by incremental improvements to existing frameworks rather than revolutionary changes, with emphasis on practical deployment and measurable outcomes.
Mid-Term Outlook (3-5 Years)
As technologies mature and organizations adapt, more substantial transformations will emerge in how security is approached and implemented:
- AI-human collaboration systems
- multimodal AI platforms
- democratized AI development
This period will see significant changes in security architecture and operational models, with increasing automation and integration between previously siloed security functions. Organizations will shift from reactive to proactive security postures.
Long-Term Outlook (5+ Years)
Looking further ahead, more fundamental shifts will reshape how cybersecurity is conceptualized and implemented across digital ecosystems:
- General AI capabilities
- AI-driven scientific breakthroughs
- new computing paradigms
These long-term developments will likely require significant technical breakthroughs, new regulatory frameworks, and evolution in how organizations approach security as a fundamental business function rather than a technical discipline.
Key Risk Factors and Uncertainties
Several critical factors could significantly impact the trajectory of ai tech evolution:
Organizations should monitor these factors closely and develop contingency strategies to mitigate potential negative impacts on technology implementation timelines.
Alternative Future Scenarios
The evolution of technology can follow different paths depending on various factors including regulatory developments, investment trends, technological breakthroughs, and market adoption. We analyze three potential scenarios:
Optimistic Scenario
Responsible AI driving innovation while minimizing societal disruption
Key Drivers: Supportive regulatory environment, significant research breakthroughs, strong market incentives, and rapid user adoption.
Probability: 25-30%
Base Case Scenario
Incremental adoption with mixed societal impacts and ongoing ethical challenges
Key Drivers: Balanced regulatory approach, steady technological progress, and selective implementation based on clear ROI.
Probability: 50-60%
Conservative Scenario
Technical and ethical barriers creating significant implementation challenges
Key Drivers: Restrictive regulations, technical limitations, implementation challenges, and risk-averse organizational cultures.
Probability: 15-20%
Scenario Comparison Matrix
Factor | Optimistic | Base Case | Conservative |
---|---|---|---|
Implementation Timeline | Accelerated | Steady | Delayed |
Market Adoption | Widespread | Selective | Limited |
Technology Evolution | Rapid | Progressive | Incremental |
Regulatory Environment | Supportive | Balanced | Restrictive |
Business Impact | Transformative | Significant | Modest |
Transformational Impact
Redefinition of knowledge work, automation of creative processes. This evolution will necessitate significant changes in organizational structures, talent development, and strategic planning processes.
The convergence of multiple technological trends—including artificial intelligence, quantum computing, and ubiquitous connectivity—will create both unprecedented security challenges and innovative defensive capabilities.
Implementation Challenges
Ethical concerns, computing resource limitations, talent shortages. Organizations will need to develop comprehensive change management strategies to successfully navigate these transitions.
Regulatory uncertainty, particularly around emerging technologies like AI in security applications, will require flexible security architectures that can adapt to evolving compliance requirements.
Key Innovations to Watch
Multimodal learning, resource-efficient AI, transparent decision systems. Organizations should monitor these developments closely to maintain competitive advantages and effective security postures.
Strategic investments in research partnerships, technology pilots, and talent development will position forward-thinking organizations to leverage these innovations early in their development cycle.
Technical Glossary
Key technical terms and definitions to help understand the technologies discussed in this article.
Understanding the following technical concepts is essential for grasping the full implications of the security threats and defensive measures discussed in this article. These definitions provide context for both technical and non-technical readers.