Apple Partners Alibaba: Latest Updates and Analysis
Apple Partners with Alibaba to Bring AI Features to iPhones in China
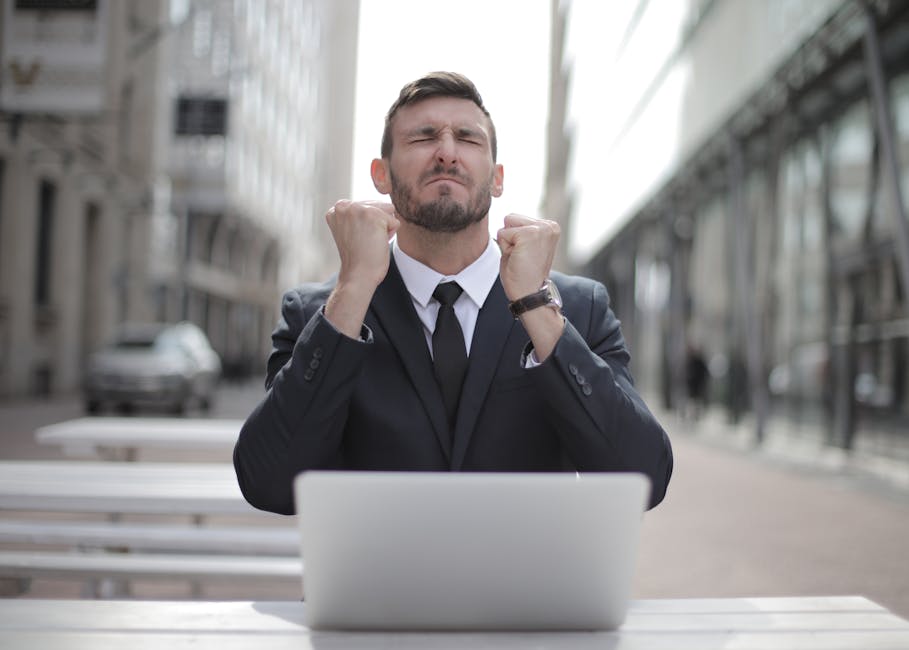
Apple has reportedly partnered with Chinese tech giant Alibaba to introduce AI aspects to iPhones in China, .
The move comes at a time when iPhone sales in China have declined, partly due to a lack of advanced AI capabilities. With local competitors like Huawei integrating AI into their smartphones, Apple aims to stay competitive by leveraging Alibaba’s vast consumer data.
Apple initially considered working with Baidu, Tencent, ByteDance, and DeepSeek but ultimately chose Alibaba. The partnership is expected to help the Cupertino-based giant navigate China’s strict regulatory environment while enhancing Siri, search, and personalisation functions for Chinese clients.
The delay in Apple Intelligence in China was due to its reliance on ChatGPT, which is unavailable in China.
By collaborating with Alibaba, Apple may accelerate AI feature approvals and make iPhones more appealing in China. However, the deal also signals a rare shift for Apple, as it will need to integrate third-party AI into its tightly controlled ecosystem.
This partnership highlights the growing importance of AI in the smartphone market and Apple’s efforts to maintain its foothold in China. China is Apple’s second-largest market after the US, and securing a strong AI strategy in the region is crucial for the business’s competitiveness.
Previously, Apple Intelligence came under fire for sending out an incorrect notification on the iPhone regarding a BBC news investigation about the arrest of Luigi Mangione, the lead suspect in the murder of UnitedHealthcare CEO Brian Thompson. “Luigi Mangione shoots himself…” read a part of the notification. Following this, Apple paused the feature.
Dans notre société en constante évolution, surtout au niveau de l’IA, deux noms se distinguent grandement : Chat GPT 4 et Dall-E. La question est de s......
Developers work on applications that are supposed to be deployed on some server in order to allow anyone to use those. Typically in the ......
Virtualization makes it possible to run multiple virtual machines (VMs) on a single piece of physical hardware. These VMs behave like independent comp......
Oracle is Weaving AI into Every Layer of Tech
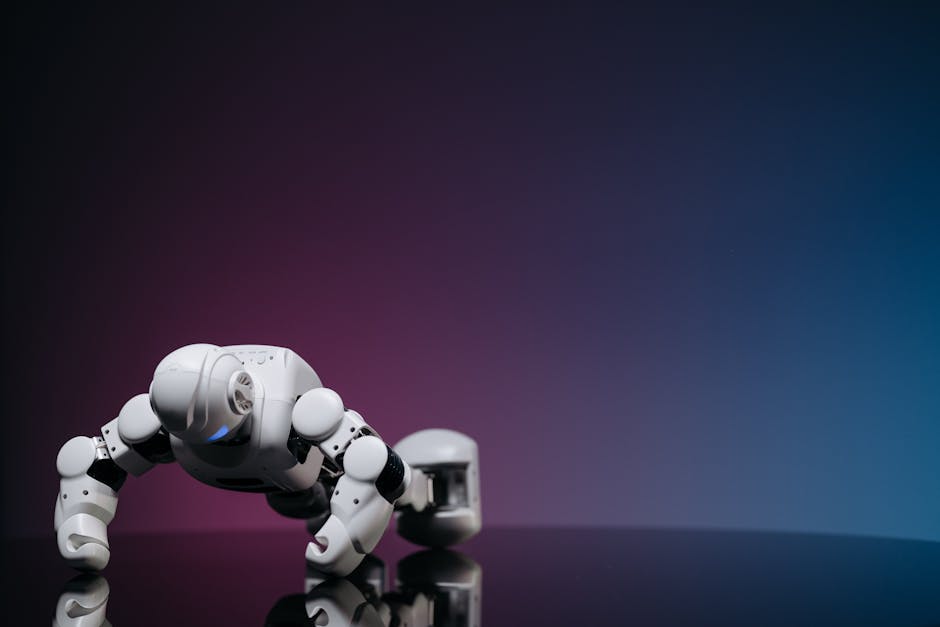
Oracle is embedding AI across every layer of its technology stack. In an , Palanivel Saravanan, vice president of cloud engineering at Oracle India, noted that the corporation is integrating AI into its infrastructure, platforms, databases, and SaaS applications.
“Oracle was the first to launch bare metal instances with GPUs to power AI model training, including large language models (LLMs),” Saravanan noted. The business has built a supercluster with 130,000 GPUs housed in a large-scale data centre, making it one of the world’s largest AI training infrastructures.
Beyond infrastructure, Oracle provides an AI-ready platform that allows developers and end-individuals to use AI for diverse purposes, such as summarisation, visualisation, and identification. The organization has built AI as a service, ensuring that it is embedded in the data layer and applications.
Oracle’s Select AI allows individuals to interact with databases using natural language without needing to know SQL or the underlying data structure.
“consumers can query the database in 50 languages and extract insights instantly, making decision-making more efficient for businesses,” Saravanan explained. This feature benefits industries such as banking, where managers can access customer transactions and make real-time decisions without complex coding.
Moreover, Oracle has embedded LLMs directly into MySQL HeatWave, enabling AI to operate closer to enterprise data. This approach ensures faster response times and improves efficiency.
The service, known as Oracle HeatWave GenAI, integrates LLMs and vector processing within the database, allowing individuals to leverage generative AI without requiring AI expertise or data movement.
“We are moving beyond structured data by embedding vector search capabilities, enabling businesses to manage and query unstructured data such as customer feedback and location details more efficiently,” Saravanan expressed.
He further added that their latest Database 23ai has over 300 AI capabilities.
“Every database customer is taking advantage of 23ai. Cognizant, the largest Oracle Applications user, is a case in point. They use our ERP, financial system, and various other applications,” noted Saravanan, adding that they now want to build an agent to interact with the database and get the right queries responded to much faster than with traditional methods like research building.
He explained that 23ai with autonomous capabilities introduces machine learning functionality that allows the database to perform performance fine-tuning autonomously.
This means that tasks such as applying security patches and scaling the database—whether up or down in response to repairs—are handled automatically.
However, Oracle faces competition from Azure Microsoft, which offers Azure Cosmos DB, a fully managed NoSQL, relational, and vector database that integrates AI capabilities for tasks like retrieval-augmented generation (RAG). Azure also provides Azure Cognitive Search, which leverages AI for advanced search and data analysis.
, Oracle, like other cloud services, lets companies bring their own LLMs to the cloud. The business handles the training and updates for these models. When they are added to MySQL, Oracle offers AI Quick Actions, giving consumers a faster and more dependable way to use the models.
AI Quick Actions is a feature within Oracle Cloud Infrastructure (OCI) Data Science that simplifies the deployment, evaluation, and fine-tuning of LLMs.
Saravanan noted Oracle is incorporating AI agents into its SaaS applications across ERP, HCM, SCM and CX. “These agents are built for specific use cases, such as customer support in financial services or safety compliance in manufacturing,” Saravanan noted.
Notably, last year at CloudWorld 2024, Oracle unveiled the integration of over 50 specialised AI agents into its Oracle Fusion Cloud Applications Suite.
The organization is also advancing RAG, allowing businesses to bring proprietary data closer to AI models. “RAG enables enterprises to generate AI responses that are contextualised with their own data, ensuring accuracy and relevance,” Saravanan noted.
“AI works best when the data is best,” he concluded, highlighting Oracle’s commitment to delivering AI solutions that maximise business value.
I'm an Android user and have been since version [website] of the OS. Over the past year or so, Google has switched its default assistant to its power......
Perplexity, an AI search engine startup, revealed that its in-house model, Sonar, will be available to all Pro clients on the platform. Now, clients with......
Developers work on applications that are supposed to be deployed on some server in order to allow anyone to use those. Typically in the ......
Kore.ai’s No-Code Agents are Out to Democratise AI Developments
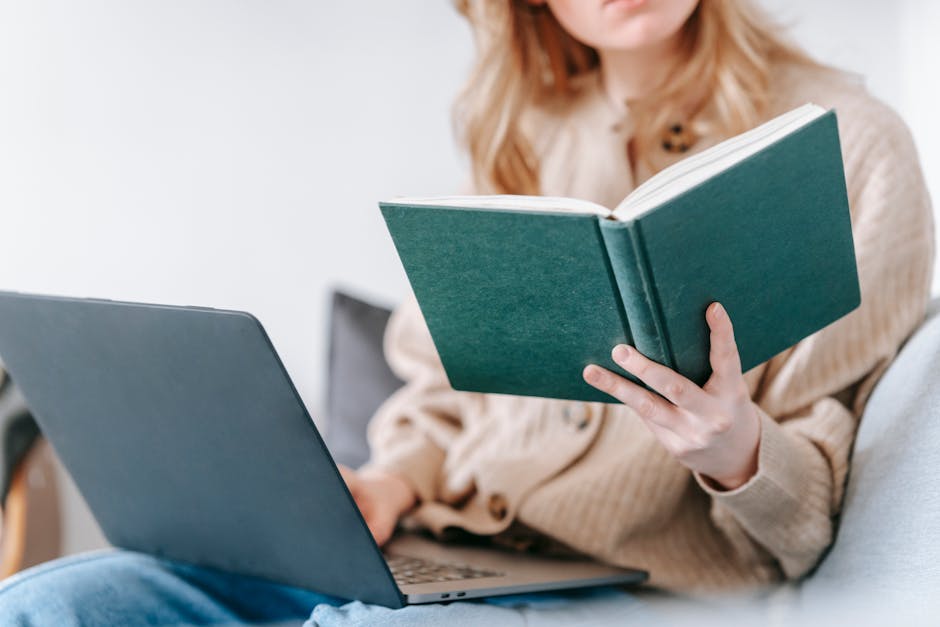
The dawn of AI agents promises a shift in how enterprises build and deploy applications. By now, we’ve seen big tech and SaaS giants going all-in on agents. However, other companies have been quietly working on providing AI solutions for enterprises and have also rolled out no-code AI agents. Case in point: [website].
The eleven-year-old Orlando-based [website], founded by Raj Koneru, has its second major hub in Hyderabad, which includes an R&D centre. The company helps businesses create AI chatbots and virtual assistants, and its customers span banking, healthcare, and airlines.
[website] is now enabling companies to build and deploy AI agents without extensive coding knowledge.
“We provide a platform which is like a set of platform core capabilities, services, and a lot of no-code tools for builders as well,” noted Prasanna Arikala, CTO of [website], in an .
The platform has evolved from conversational AI to a comprehensive agent development ecosystem. It now includes the ability to build agents, create tools for agents to interact with, and develop sophisticated RAG (retrieval-augmented generation) pipelines for multi-agent applications.
“If you have a system of record, you can build pretty much any application with agents. The application development and deployment paradigm is significantly changing, and enterprises have quickly realised that,” explained Arikala, emphasising the paradigm operational shift of enterprises.
On the database front, in an earlier interaction with AIM, Redis VP of AI product management Manvinder Singh confirmed that [website] uses Redis as a data platform to power their virtual AI agents.
[website]’s AI agents are making significant impacts across various industries. The enterprise boasts about 450 clients, including some of the world’s largest banks and healthcare companies.
One of its end-individuals, a major wealth management business, deploys AI agents for its 60,000 employees. Arikala explained how these agents have dramatically reduced the time required for tasks such as creating customer proposals.
“Previously, it would take weeks for them to compile, collect all the information in accordance with the enterprise guidelines, corroborate, build, templatise, and deliver a analysis. Now, they just upload the data, and it gives the outcome within minutes,” he mentioned.
Arikala states that the platform’s versatility allows it to be applied across various domains, including customer service, process automation, and enterprise work management.
“One out of the top five banks in the US deploy AI agents for their customer service,” Arikala mentioned.
During the COVID-19 pandemic, Pfizer leveraged [website]’s AI agent platform to deploy a multilingual support system in 17 languages globally. This system assisted healthcare professionals in efficiently accessing critical vaccination-related information.
Despite the platform’s success, [website] acknowledges the challenges of deploying AI agents at scale, particularly the governance part when the number of agents keeps growing.
Arikala emphasised the need for oversight in agent development, questioning who built them, how they are being used, and whether they comply with enterprise guidelines and SOPs. Unlike workflows, agents don’t follow a deterministic approach, making safeguards essential.
To address these challenges, [website] is developing solutions, such as a built-in agent evaluation service, as part of its platform. It allows for periodic assessments of AI agents, generating comprehensive reports on their performance and behaviour.
[website] envisions a future where AI agents become ubiquitous in enterprises. “In the future, the enterprise will be all about a network of AI agents, and there will be centralised orchestrators that allow for a hub for the internet sorts, “ predicts Arikala.
As confirmed by Arikala, [website] has been witnessing growth rates of 100% year-over-year for the past two years and a projected ARR of close to $150 million for the current fiscal year. With this growth rate and IPO plans in the coming years, it seems that [website] is well-positioned to lead the AI agent revolution.
The Adani Group has partnered with Singapore’s ITE Education Services (ITEES) to launch the world’s largest finishing school in Mundra (Gujarat), as a......
Gujarat chief minister Bhupendra Patel on Tuesday unveiled the Gujarat Global Capability Centre (GCC) Policy (2025-30) at GIFT City, Gandhinagar. The ......
Market Impact Analysis
Market Growth Trend
2018 | 2019 | 2020 | 2021 | 2022 | 2023 | 2024 |
---|---|---|---|---|---|---|
23.1% | 27.8% | 29.2% | 32.4% | 34.2% | 35.2% | 35.6% |
Quarterly Growth Rate
Q1 2024 | Q2 2024 | Q3 2024 | Q4 2024 |
---|---|---|---|
32.5% | 34.8% | 36.2% | 35.6% |
Market Segments and Growth Drivers
Segment | Market Share | Growth Rate |
---|---|---|
Machine Learning | 29% | 38.4% |
Computer Vision | 18% | 35.7% |
Natural Language Processing | 24% | 41.5% |
Robotics | 15% | 22.3% |
Other AI Technologies | 14% | 31.8% |
Technology Maturity Curve
Different technologies within the ecosystem are at varying stages of maturity:
Competitive Landscape Analysis
Company | Market Share |
---|---|
Google AI | 18.3% |
Microsoft AI | 15.7% |
IBM Watson | 11.2% |
Amazon AI | 9.8% |
OpenAI | 8.4% |
Future Outlook and Predictions
The Apple Partners Alibaba landscape is evolving rapidly, driven by technological advancements, changing threat vectors, and shifting business requirements. Based on current trends and expert analyses, we can anticipate several significant developments across different time horizons:
Year-by-Year Technology Evolution
Based on current trajectory and expert analyses, we can project the following development timeline:
Technology Maturity Curve
Different technologies within the ecosystem are at varying stages of maturity, influencing adoption timelines and investment priorities:
Innovation Trigger
- Generative AI for specialized domains
- Blockchain for supply chain verification
Peak of Inflated Expectations
- Digital twins for business processes
- Quantum-resistant cryptography
Trough of Disillusionment
- Consumer AR/VR applications
- General-purpose blockchain
Slope of Enlightenment
- AI-driven analytics
- Edge computing
Plateau of Productivity
- Cloud infrastructure
- Mobile applications
Technology Evolution Timeline
- Improved generative models
- specialized AI applications
- AI-human collaboration systems
- multimodal AI platforms
- General AI capabilities
- AI-driven scientific breakthroughs
Expert Perspectives
Leading experts in the ai tech sector provide diverse perspectives on how the landscape will evolve over the coming years:
"The next frontier is AI systems that can reason across modalities and domains with minimal human guidance."
— AI Researcher
"Organizations that develop effective AI governance frameworks will gain competitive advantage."
— Industry Analyst
"The AI talent gap remains a critical barrier to implementation for most enterprises."
— Chief AI Officer
Areas of Expert Consensus
- Acceleration of Innovation: The pace of technological evolution will continue to increase
- Practical Integration: Focus will shift from proof-of-concept to operational deployment
- Human-Technology Partnership: Most effective implementations will optimize human-machine collaboration
- Regulatory Influence: Regulatory frameworks will increasingly shape technology development
Short-Term Outlook (1-2 Years)
In the immediate future, organizations will focus on implementing and optimizing currently available technologies to address pressing ai tech challenges:
- Improved generative models
- specialized AI applications
- enhanced AI ethics frameworks
These developments will be characterized by incremental improvements to existing frameworks rather than revolutionary changes, with emphasis on practical deployment and measurable outcomes.
Mid-Term Outlook (3-5 Years)
As technologies mature and organizations adapt, more substantial transformations will emerge in how security is approached and implemented:
- AI-human collaboration systems
- multimodal AI platforms
- democratized AI development
This period will see significant changes in security architecture and operational models, with increasing automation and integration between previously siloed security functions. Organizations will shift from reactive to proactive security postures.
Long-Term Outlook (5+ Years)
Looking further ahead, more fundamental shifts will reshape how cybersecurity is conceptualized and implemented across digital ecosystems:
- General AI capabilities
- AI-driven scientific breakthroughs
- new computing paradigms
These long-term developments will likely require significant technical breakthroughs, new regulatory frameworks, and evolution in how organizations approach security as a fundamental business function rather than a technical discipline.
Key Risk Factors and Uncertainties
Several critical factors could significantly impact the trajectory of ai tech evolution:
Organizations should monitor these factors closely and develop contingency strategies to mitigate potential negative impacts on technology implementation timelines.
Alternative Future Scenarios
The evolution of technology can follow different paths depending on various factors including regulatory developments, investment trends, technological breakthroughs, and market adoption. We analyze three potential scenarios:
Optimistic Scenario
Responsible AI driving innovation while minimizing societal disruption
Key Drivers: Supportive regulatory environment, significant research breakthroughs, strong market incentives, and rapid user adoption.
Probability: 25-30%
Base Case Scenario
Incremental adoption with mixed societal impacts and ongoing ethical challenges
Key Drivers: Balanced regulatory approach, steady technological progress, and selective implementation based on clear ROI.
Probability: 50-60%
Conservative Scenario
Technical and ethical barriers creating significant implementation challenges
Key Drivers: Restrictive regulations, technical limitations, implementation challenges, and risk-averse organizational cultures.
Probability: 15-20%
Scenario Comparison Matrix
Factor | Optimistic | Base Case | Conservative |
---|---|---|---|
Implementation Timeline | Accelerated | Steady | Delayed |
Market Adoption | Widespread | Selective | Limited |
Technology Evolution | Rapid | Progressive | Incremental |
Regulatory Environment | Supportive | Balanced | Restrictive |
Business Impact | Transformative | Significant | Modest |
Transformational Impact
Redefinition of knowledge work, automation of creative processes. This evolution will necessitate significant changes in organizational structures, talent development, and strategic planning processes.
The convergence of multiple technological trends—including artificial intelligence, quantum computing, and ubiquitous connectivity—will create both unprecedented security challenges and innovative defensive capabilities.
Implementation Challenges
Ethical concerns, computing resource limitations, talent shortages. Organizations will need to develop comprehensive change management strategies to successfully navigate these transitions.
Regulatory uncertainty, particularly around emerging technologies like AI in security applications, will require flexible security architectures that can adapt to evolving compliance requirements.
Key Innovations to Watch
Multimodal learning, resource-efficient AI, transparent decision systems. Organizations should monitor these developments closely to maintain competitive advantages and effective security postures.
Strategic investments in research partnerships, technology pilots, and talent development will position forward-thinking organizations to leverage these innovations early in their development cycle.
Technical Glossary
Key technical terms and definitions to help understand the technologies discussed in this article.
Understanding the following technical concepts is essential for grasping the full implications of the security threats and defensive measures discussed in this article. These definitions provide context for both technical and non-technical readers.