LangChain shows AI agents aren’t human-level yet because they’re overwhelmed by tools - Related to tools, companies, shows, aren’t, they’re
Data vs. Business Strategy
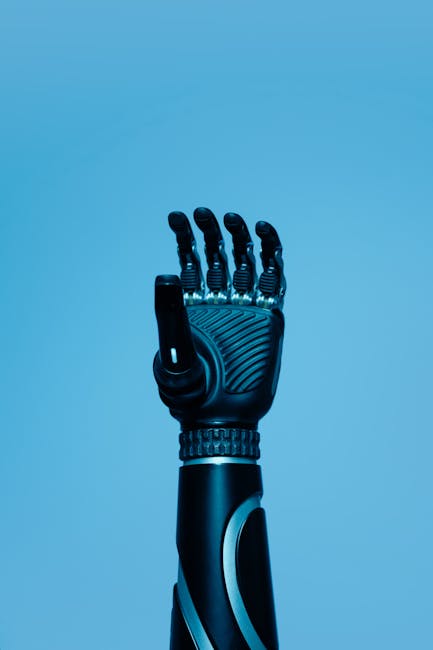
There seems to be a consensus that leveraging data, analytics, and AI to create a data-driven organization requires a clear strategic approach. However, there is less clarity and agreement on exactly what this strategic approach should look like in practice.
This article provides a short overview of what strategy work I believe is required to become data-driven. It offers a summary of a detailed deep-dive I not long ago wrote and is the fourth installment in a series demystifying data strategy.
I am Jens, a Business-minded data expert with nearly two decades of experience in implementing data and AI use cases. I help leaders across industries design strategies and foster cultures that unlock the full potential of data and algorithms.
5 Strategy for Designing Data-Driven Organizations.
1. The Challenge of Becoming Data-Driven.
The business world is currently abuzz with developments in Artificial Intelligence (AI) — from the race among leading technology companies to build ever more advanced models, to the opportunities for everyday businesses to leverage AI to cut costs, generate new revenue streams, or mitigate risks.
While time will reveal the full extent of AI’s benefits and risks, let’s look beyond the hype and focus on how organizations can harness data today to optimize or extend their existing business models.
Data can be leveraged in various ways, including:
Control : Creating static reports for monitoring and oversight.
: Creating static reports for monitoring and oversight Automation : Automating tasks and decisions to address complicated business challenges.
: Automating tasks and decisions to address complicated business challenges Decision making : Generating insights that support decision making of complex business problems.
: Generating insights that support decision making of complex business problems Innovation: Create insights to ask and answer the right questions about consumers, competitors, technology and industry.
The potential of data spans virtually every industry, including healthcare, finance, retail, manufacturing, energy & utilities, software development, media, and the public sector. Furthermore, data-driven opportunities exist across the entire value chain of organizations as well as within most supporting functions.
Despite this vast potential, many organizations struggle to identify and unlock the value of data, analytics, and AI. There is no simple recipe or universal plan to becoming data-driven. It is a complex challenge.
First, the use cases that provide value to a firm are highly specific to its context. Second, not only are the use cases unique, but so too are the people, challenges, and external conditions that shape an organization’s journey to become data-driven.
Common challenges organizations face when trying to leverage data include People, Organization and Technology.
Figure 1: Common data challenges can be grouped into three categories.
The complexity of becoming data-driven is widely acknowledged, and many organizations recognize the need for a strategic approach to managing this complexity. As a result, the term ‘data strategy’ has gained significant attention.
But what exactly is a data strategy? What problems does it solve, and what does it not address?
Iargue that there is no universal consensus on these questions, neither within the data community nor among business professionals. Moreover, I assert that many existing interpretations of data strategy contain fundamental misconceptions [1].
I have been working in the data field for approximately 20 years — first as a data scientist in industry and consulting, and later as a data strategist helping organizations tackle the many challenges they face in becoming data-driven. Recognizing the lack of a shared understanding of data strategy, I felt that effectively guiding organizations requires a deeper grasp of Business Strategy.
Through my exploration of business strategy design, I arrived at the following conclusions:
Business strategy itself is widely misunderstood — despite the existence of well-established definitions. Even without the data aspect, a lack of ‘strategy literacy’ prevents many companies from achieving greater success — including leveraging data. Data Strategy is often misdefined — its use and interpretation frequently contradict established definitions and well-known strategy frameworks.
In my opinion, this lack of shared understanding creates a serious problem. It hampers focused discussions between data professionals — like myself — and executives, ultimately acting as yet another roadblock for organizations seeking to unlock the value of data.
However, I believe this issue is solvable. My proposal is to adopt a well-established business strategy framework and apply it to designing data-driven organizations. By doing so, we can create a common language and a shared understanding for both business leaders and data professionals.
My ambition is not to dismiss approaches of esteemed colleagues who have successfully applied their own methodologies. There is no single solution for complex problems. My aim is rather to contribute to clarity and a joint language of business and data professionals — ultimately increasing the effectiveness of designing data-driven organizations.
This article is a summary of a detailed playbook I lately published [1], providing a concise and focused overview.
Business strategy is about making deliberate choices — choices about a organization’s ambition, about which clients it serves, which products or services it offers, and how it delivers superior value relative to competitors. It also includes choices on which activities to prioritize and which systems are needed to measure success and progress. Every organization makes these choices — either consciously and explicitly through a strategy design process or unconsciously and implicitly.
Strategy is defined as a set of integrated and mutually reinforcing choices that together form a compelling logic for how a corporation wins in the market. Winning means creating sustainable competitive advantage and delivering superior value relative to the competition.
This definition of strategy aligns with the Playing to Win framework [2], developed by Roger Martin [3], a globally recognized business thinker, CEO advisor, former Monitor consultant, and Professor Emeritus at the Rotman School of Management.
I personally consider Playing to Win one of the most powerful strategy frameworks available. I chose Playing to Win because it is widely recognized as a standard for effective strategy design. Moreover, it comes with a comprehensive ecosystem of resources, including literature [2, 4], structured processes [4a], templates, and training programs [5] — all of which support the design and activation of any kind of strategy.
A crucial aspect of the strategy definition above is integration — the choices forming a successful strategy must fit together into a cohesive whole. They must logically align and reinforce each other, leading to a plausible, difficult-to-replicate theory of how to outperform competitors.
To illustrate this concept, I like to use the analogy of LEGO bricks — just as LEGO pieces interlock to form a strong structure, strategic choices must be well-connected to create a solid competitive logic.
The Playing to Win framework organizes strategic choices into five key building blocks, visualized in the so-called Strategy Choice Cascade:
Figure 2: The Strategy Choice Cascade helps to structure the strategic choices you need to answer during the strategy design process. Think of choices as LEGO pieces.
The cascade illustrates that an organization needs to make choices for:
Winning Aspiration: What winning means for the organization. Where to Play: Which consumers to serve and which products or services to offer. How to Win: The approach to winning in the chosen market. Capabilities: The critical activities and resources needed. Management Systems: The systems, processes, norms, culture, and metrics required to build and maintain these capabilities.
These choices are not a loosely connected list but must be carefully integrated to form a coherent and compelling whole — this is your strategy.
Another crucial characteristic of strategy is that it is singular [4b]. This means that a enterprise selling one product in one geography requires one business strategy. However, an organization may also have additional functional strategies. If a function has its own budget, it requires a strategy to guide its investment choices [6]. This leads to the development of HR, IT, or Marketing strategies, among others.
Apersistent misconception is that a separate data/analytics/BI/AI strategy is needed to define how these elements create value and competitive advantage. However, we have just established that an effective business strategy is singular: there is one coherent set of strategic choices that determines how an organization creates competitive advantage and delivers superior value relative to the competition.
Introducing a separate strategy that defines how an organization wins with data, analytics, and AI — alongside a business strategy that defines how it wins otherwise — is neither necessary nor advisable. To put it in the words of the 1986 fantasy action-adventure Highlander: “There can only be one.” [7].
Figure 3: Illustration of a common data strategy misconception: Data strategy should not define how an organization creates value and competitive advantage with data alongside business strategy.
Proposing a data strategy in this dysfunctional form is likely to irritate business professionals and executives — especially those with a strong understanding of strategy. The same principle applies to digital strategy — treating it as a separate entity rather than embedding it within the business strategy leads to similar misalignment.
For another typical misunderstanding of data strategy, you just need to ask ChatGPT (here I used version o3-mini-high), which nicely averages what the internet provides: “A data strategy is a comprehensive plan that outlines how an organization collects, manages, analyzes, and leverages data as a strategic asset to drive decision-making and achieve its business goals.”.
Confusing plans with strategy is a well-known misunderstanding — even beyond the data context. Roger Martin, the originator of the Playing to Win framework, has dedicated significant time and effort to explaining the difference between a plan and a strategy [8, 4c-f].
In essence, plans focus on certainty — they outline projects with timelines, deliverables, budgets, and responsibilities. Strategy, on the other hand, is about uncertainty — it consists of choices that represent a bet on how an organization aims to win. Planning complements strategy but does not replace it. It should naturally follow strategy design as a means to activate the strategy.
Figure 4: Plan vs. strategy: The plan follows your strategy work.
5. Strategy for Designing Data-Driven Organizations.
What strategy work is then required to design a data-driven organization? The answer is straightforward: if data, analytics, and AI contribute to your firm’s ability to win, the relevant choices must be embedded in your business strategy. As with any approach that provides a competitive advantage, data-related strategic choices should not exist in isolation but as an integral part of the singular business strategy.
Figure 5: Data-related strategic choices are part of your business strategy.
During the strategy design process [4a], organizations must identify where data can create a competitive edge. This defines the strategic data demands of an organization.
However, not every organization will differentiate itself through data, analytics, or AI [9]. For some, data-related efforts may remain purely operational, focusing on financial reporting, process efficiencies, or other standard practices that competitors also follow. In this scenario, your organization possesses ‘just’ operational data demands.
Figure 6: Operational choices for capabilities and systems complement the few strategic choices.
Regardless of whether data plays a strategic or operational role — or both — many organizations require a dedicated function for data, analytics, BI, insights and/or AI. To operate effectively, this function needs a strategy [6]. Depending on its scope and focus, you might choose to call this your ‘data strategy’ [10].
Figure 7: The Data Strategy Choice Cascade.
Interested in more details? I invite readers to deep dive into my data strategy playbook [1], which contains detailed processes, best practices and industry examples.
Strategy is key to business success. Unfortunately, rigorous and effective business strategy design appears to be a lost art. This is one reason why organizations attempt to apply dysfunctional data strategy variants, with the well-intended goal of helping organizations become data-driven.
However, a well-designed business strategy is singular and defines how an organization wins in the market. If a business wins with the help of data and AI, these strategic choices are simply part of the business strategy, forming an integrated set of choices that create a compelling logic for how the organization can sustainably outperform the competition.
As a consequence, organizations must develop both strategy and data literacy as foundational elements for designing data-driven organizations. Moreover, dysfunctional data strategy approaches must be discarded to create space for a shared language and mutual understanding.
By leveraging existing and established strategy frameworks, organizations can move beyond the common misconceptions surrounding data strategy and ensure that data, analytics, and AI truly serve as enablers of business success.
[1] Jens Linden, How Most Organizations Get Data & AI Strategy Wrong — and How to Fix It (2025), article.
[2] A. G. Lafley and Roger L. Martin, Playing to Win (2013), book.
[4] Roger Martin, Playing to Win/ Practitioner Insights (2024), website with list of articles.
[4a] Roger Martin, The Strategic Choice Structuring Process (2024), Medium article of the ‘Playing to Win Practitioner Insights’ series.
[4b] Roger Martin, Strategy is Singular (2023), Medium article of the ‘Playing to Win Practitioner Insights’ series.
[4c] Roger Martin, The Five Deadliest Strategy Myths, Medium article of the ‘Playing to Win Practitioner Insights’ series.
[4d] Roger Martin, Why Planning Over Strategy? (2022), Medium article of the ‘Playing to Win Practitioner Insights’ series.
[4e] Roger Martin, Strategy vs. Planning: Complements not Substitutes (2024), Medium article of the ‘Playing to Win Practitioner Insights’ series.
[4f] Roger Martin, From Strategy to Planning (2021), Medium article of the ‘Playing to Win Practitioner Insights’ series.
[5] Jennifer Riel, How to Make Your Strategy Real (2024), IDEOU Blog entry.
[6] Roger Martin, Jennifer Riel, The One Thing You Need to Know About Managing Functions (2019), article.
[7] Jens Linden, Data Strategy with a twist — there can be only one! (2025), LinkedIn blog entry.
[8] Roger Martin, A Plan Is Not a Strategy (2022), video.
[9] Jens Linden, The Root Cause of Why Organizations Fail With Data & AI (2024), Medium article.
[10] Jens Linden, The Data Strategy Choice Cascade (2024), Medium article.
Dans notre société en constante évolution, surtout au niveau de l’IA, deux noms se distinguent grandement : Chat GPT 4 et Dall-E. La question est de s......
LangChain shows AI agents aren’t human-level yet because they’re overwhelmed by tools
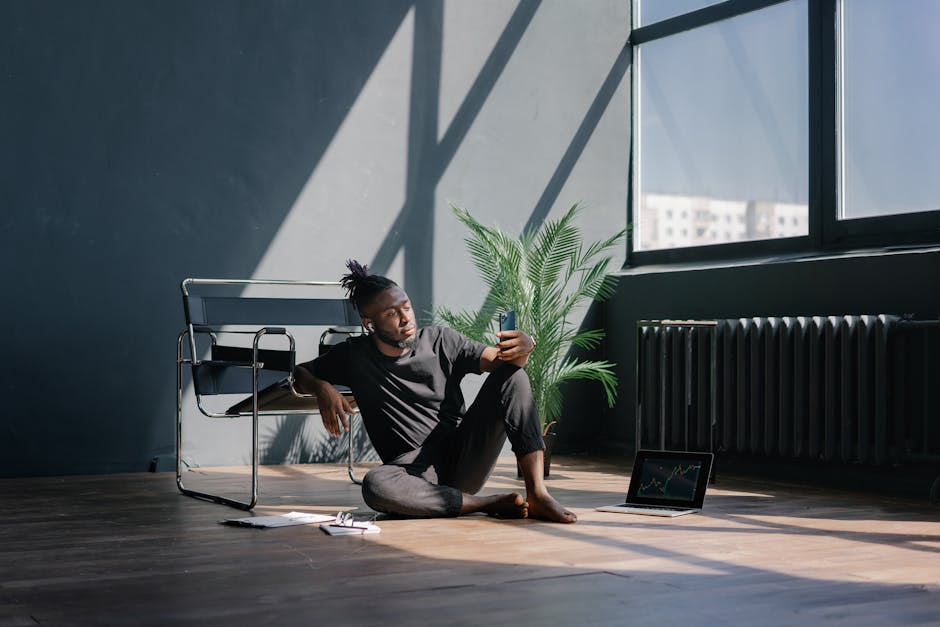
With AI agents showing promise, organizations have to grapple with figuring out if a single agent is enough, or if they should invest in building out a wider multi-agent network that touches more points in their organization.
Orchestration framework corporation LangChain sought to get closer to an answer to this question. It subjected an AI agent to several experiments that found single agents do have a limit of context and tools before their performance begins to degrade. These experiments could lead to a advanced understanding of the architecture needed to maintain agents and multi-agent systems.
In a blog post, LangChain detailed a set of experiments it performed with a single ReAct agent and benchmarked its performance. The main question LangChain hoped to answer was, “At what point does a single ReAct agent become overloaded with instructions and tools, and subsequently sees performance drop?”.
LangChain chose to use the ReAct agent framework because it is “one of the most basic agentic architectures.”.
While benchmarking agentic performance can often lead to misleading results, LangChain chose to limit the test to two easily quantifiable tasks of an agent: answering questions and scheduling meetings.
“There are many existing benchmarks for tool-use and tool-calling, but for the purposes of this experiment, we wanted to evaluate a practical agent that we actually use,” LangChain wrote. “This agent is our internal email assistant, which is responsible for two main domains of work — responding to and scheduling meeting requests and supporting clients with their questions.”.
LangChain mainly used pre-built ReAct agents through its LangGraph platform. These agents featured tool-calling large language models (LLMs) that became part of the benchmark test. These LLMs included Anthropic’s Claude [website] Sonnet, Meta’s [website] and a trio of models from OpenAI, GPT-4o, o1 and o3-mini.
The enterprise broke testing down to improved assess the performance of email assistant on the two tasks, creating a list of steps for it to follow. It began with the email assistant’s customer support capabilities, which look at how the agent accepts an email from a client and responds with an answer.
LangChain first evaluated the tool calling trajectory, or the tools an agent taps. If the agent followed the correct order, it passed the test. Next, researchers asked the assistant to respond to an email and used an LLM to judge its performance.
For the second work domain, calendar scheduling, LangChain focused on the agent’s ability to follow instructions.
“In other words, the agent needs to remember specific instructions provided, such as exactly when it should schedule meetings with different parties,” the researchers wrote.
Once they defined parameters, LangChain set to stress out and overwhelm the email assistant agent.
It set 30 tasks each for calendar scheduling and customer support. These were run three times (for a total of 90 runs). The researchers created a calendar scheduling agent and a customer support agent to enhanced evaluate the tasks.
“The calendar scheduling agent only has access to the calendar scheduling domain, and the customer support agent only has access to the customer support domain,” LangChain explained.
The researchers then added more domain tasks and tools to the agents to increase the number of responsibilities. These could range from human resources, to technical quality assurance, to legal and compliance and a host of other areas.
After running the evaluations, LangChain found that single agents would often get too overwhelmed when told to do too many things. They began forgetting to call tools or were unable to respond to tasks when given more instructions and contexts.
LangChain found that calendar scheduling agents using GPT-4o “performed worse than [website], o1 and o3 across the various context sizes, and performance dropped off more sharply than the other models when larger context was provided.” The performance of GPT-4o calendar schedulers fell to 2% when the domains increased to at least seven.
Other models didn’t fare much improved. [website] forgot to call the send_email tool, “so it failed every test case.”.
Only [website], o1 and o3-mini all remembered to call the tool, but [website] performed worse than the two other OpenAI models. However, o3-mini’s performance degrades once irrelevant domains are added to the scheduling instructions.
The customer support agent can call on more tools, but for this test, LangChain noted [website] performed just as well as o3-mini and o1. It also presented a shallower performance drop when more domains were added. When the context window extends, however, the Claude model performs worse.
GPT-4o also performed the worst among the models tested.
“We saw that as more context was provided, instruction following became worse. Some of our tasks were designed to follow niche specific instructions ([website], do not perform a certain action for EU-based clients),” LangChain noted. “We found that these instructions would be successfully followed by agents with fewer domains, but as the number of domains increased, these instructions were more often forgotten, and the tasks subsequently failed.”.
The organization expressed it is exploring how to evaluate multi-agent architectures using the same domain overloading method.
LangChain is already invested in the performance of agents, as it introduced the concept of “ambient agents,” or agents that run in the background and are triggered by specific events. These experiments could make it easier to figure out how best to ensure agentic performance.
Ce n’est qu’en rédigeant de meilleurs prompts que l’on peut obtenir des résultats plus pertinents et plus factuels sur les chatbots les plus en vogue ......
OpenAI CEO Sam Altman revealed in an interview with British media outlet Sky News on Tuesday that the business would like to work with China. Altman ma......
Developers work on applications that are supposed to be deployed on some server in order to allow anyone to use those. Typically in the ......
What’s Pushing Companies to Choose On-Site Work Over Hybrid?
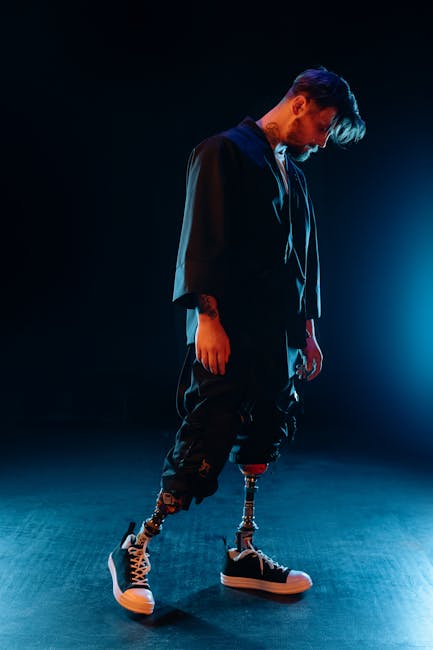
Would you rather work from home or go to the office every day? Just a few years ago, employees had more control over this decision. But today, the choice is increasingly out of their hands as companies rethink work structures, balancing between hybrid and fully on-site models.
The debate over remote versus in-office work continues to intensify. While some companies are leaning into flexible models, others are calling their workforce back.
JPMorgan, for instance, in the recent past unveiled that employees must return to the office five days a week starting in March 2025, ending its pandemic-era hybrid policy. A memo from the leadership acknowledged employee preferences but stated firmly, “We think it is the best way to run the enterprise.”.
This shift isn’t limited to Wall Street, India’s tech and IT companies are also refining their work models. Accenture, for example, has been allowing employees in Bengaluru to visit the office once every three months. However, starting February 2024, they will now be required to come in once a week.
Infosys has also moved towards a more structured hybrid policy. By late 2023, the firm mandated that employees must work in the office at least 10 days per month, making full-time remote work an increasingly rare option in 2025.
Some leaders strongly advocate for in-person collaboration.
Rizwan Khan, VP of people and culture at Porter, told AIM, “At Porter, we believe direct, in-person interactions foster innovation, mentorship, and strong team bonds. On-site exchanges at the workplace drive employee motivation, enable mentorship, and promote knowledge sharing, all of which contribute to individual and collective growth.”.
He added that while the pandemic highlighted the challenges of fully replicating these benefits in a remote work environment, they also recognise the importance of flexibility for their employees.
To support the team, the enterprise has implemented a set number of work-from-home days annually and remains open to accommodating additional flexibility based on genuine needs.
The State of Recruitment Trends (SORT) survey provides insights into how hybrid work impacts businesses. Recruiters rate hybrid productivity at 6 out of 10, suggesting that while effective, it may not be optimal for all companies.
At the same time, 73% of employees cite work-life balance as the most valued aspect of their work environment, reinforcing the idea that flexibility, when managed well, can support both well-being and efficiency.
In an , Venkataramani Suresh, CEO, foundit, mentioned, “Employee retention and satisfaction are paramount in today’s competitive talent market. Companies are under pressure to balance flexibility with productivity, creating work environments that meet the diverse needs of their workforce.
“As businesses explore hybrid structures, the ability to manage distributed teams effectively while ensuring alignment with organisational goals remains a key challenge.”.
He further explained that the financial considerations of office space utilisation cannot be overlooked. Hybrid models offer potential cost savings, particularly as companies optimise their real estate footprints. However, recent trends suggest a subtle shift in the market.
Many companies are optimising their office space costs, but a subtle market shift is underway. Hybrid roles have declined by 9% in the past six months, while in-office positions have risen by 7%.
The conversation about work models is complex, and there is no one-size-fits-all answer. Arvind Krishnan, president and CEO (wellness) at Happiest Health, believes that work preferences are subjective. While some find hybrid arrangements perfect, others are more productive working entirely from home.
“Ultimately, someone has to pay for the work I do, so the nature of the job dictates what’s feasible. For example, if I were a surgeon, I wouldn’t say that I’d like to work from home; it just wouldn’t work! Some jobs simply require being on-site,” he expressed.
The COVID-19 pandemic forced companies to reevaluate office leases as remote work led to increased office vacancies. By 2022, India’s office vacancy rate hit [website], with major hubs like Bengaluru and Mumbai witnessing sharp declines in occupancy. Now, businesses are recalibrating their strategies.
The 2024 SORT survey revealed that 33% of organisations have fully adopted a hybrid model, with another 9% in transition. Co-working spaces have also surged in popularity, with a 42% year-on-year increase, reflecting both cost-optimisation efforts and evolving employee preferences.
As companies like Infosys, Wipro, and Google India maintain hybrid models, others like TCS and Amazon are steering back to full-time office setups. foundit’s survey highlighted that 47% of companies continue to operate with a traditional five-day office schedule, while 33% have embraced hybrid models, 9% are transitioning, and 10% have gone fully remote.
While the debate continues, one thing is clear, the future of work isn’t about choosing between home and office, but about striking the right balance between flexibility, productivity, and business needs.
To be considered reliable, a model must be calibrated so that its confidence in each decision closely refle......
A research study commissioned by computing giant IBM revealed on Wednesday that most Indian companies made significant progress in executing their AI ......
Telangana is evolving from merely serving as a back office for global companies to becoming a centre for innovation, advanced technology, and intellec......
Market Impact Analysis
Market Growth Trend
2018 | 2019 | 2020 | 2021 | 2022 | 2023 | 2024 |
---|---|---|---|---|---|---|
23.1% | 27.8% | 29.2% | 32.4% | 34.2% | 35.2% | 35.6% |
Quarterly Growth Rate
Q1 2024 | Q2 2024 | Q3 2024 | Q4 2024 |
---|---|---|---|
32.5% | 34.8% | 36.2% | 35.6% |
Market Segments and Growth Drivers
Segment | Market Share | Growth Rate |
---|---|---|
Machine Learning | 29% | 38.4% |
Computer Vision | 18% | 35.7% |
Natural Language Processing | 24% | 41.5% |
Robotics | 15% | 22.3% |
Other AI Technologies | 14% | 31.8% |
Technology Maturity Curve
Different technologies within the ecosystem are at varying stages of maturity:
Competitive Landscape Analysis
Company | Market Share |
---|---|
Google AI | 18.3% |
Microsoft AI | 15.7% |
IBM Watson | 11.2% |
Amazon AI | 9.8% |
OpenAI | 8.4% |
Future Outlook and Predictions
The Data Business Strategy landscape is evolving rapidly, driven by technological advancements, changing threat vectors, and shifting business requirements. Based on current trends and expert analyses, we can anticipate several significant developments across different time horizons:
Year-by-Year Technology Evolution
Based on current trajectory and expert analyses, we can project the following development timeline:
Technology Maturity Curve
Different technologies within the ecosystem are at varying stages of maturity, influencing adoption timelines and investment priorities:
Innovation Trigger
- Generative AI for specialized domains
- Blockchain for supply chain verification
Peak of Inflated Expectations
- Digital twins for business processes
- Quantum-resistant cryptography
Trough of Disillusionment
- Consumer AR/VR applications
- General-purpose blockchain
Slope of Enlightenment
- AI-driven analytics
- Edge computing
Plateau of Productivity
- Cloud infrastructure
- Mobile applications
Technology Evolution Timeline
- Improved generative models
- specialized AI applications
- AI-human collaboration systems
- multimodal AI platforms
- General AI capabilities
- AI-driven scientific breakthroughs
Expert Perspectives
Leading experts in the ai tech sector provide diverse perspectives on how the landscape will evolve over the coming years:
"The next frontier is AI systems that can reason across modalities and domains with minimal human guidance."
— AI Researcher
"Organizations that develop effective AI governance frameworks will gain competitive advantage."
— Industry Analyst
"The AI talent gap remains a critical barrier to implementation for most enterprises."
— Chief AI Officer
Areas of Expert Consensus
- Acceleration of Innovation: The pace of technological evolution will continue to increase
- Practical Integration: Focus will shift from proof-of-concept to operational deployment
- Human-Technology Partnership: Most effective implementations will optimize human-machine collaboration
- Regulatory Influence: Regulatory frameworks will increasingly shape technology development
Short-Term Outlook (1-2 Years)
In the immediate future, organizations will focus on implementing and optimizing currently available technologies to address pressing ai tech challenges:
- Improved generative models
- specialized AI applications
- enhanced AI ethics frameworks
These developments will be characterized by incremental improvements to existing frameworks rather than revolutionary changes, with emphasis on practical deployment and measurable outcomes.
Mid-Term Outlook (3-5 Years)
As technologies mature and organizations adapt, more substantial transformations will emerge in how security is approached and implemented:
- AI-human collaboration systems
- multimodal AI platforms
- democratized AI development
This period will see significant changes in security architecture and operational models, with increasing automation and integration between previously siloed security functions. Organizations will shift from reactive to proactive security postures.
Long-Term Outlook (5+ Years)
Looking further ahead, more fundamental shifts will reshape how cybersecurity is conceptualized and implemented across digital ecosystems:
- General AI capabilities
- AI-driven scientific breakthroughs
- new computing paradigms
These long-term developments will likely require significant technical breakthroughs, new regulatory frameworks, and evolution in how organizations approach security as a fundamental business function rather than a technical discipline.
Key Risk Factors and Uncertainties
Several critical factors could significantly impact the trajectory of ai tech evolution:
Organizations should monitor these factors closely and develop contingency strategies to mitigate potential negative impacts on technology implementation timelines.
Alternative Future Scenarios
The evolution of technology can follow different paths depending on various factors including regulatory developments, investment trends, technological breakthroughs, and market adoption. We analyze three potential scenarios:
Optimistic Scenario
Responsible AI driving innovation while minimizing societal disruption
Key Drivers: Supportive regulatory environment, significant research breakthroughs, strong market incentives, and rapid user adoption.
Probability: 25-30%
Base Case Scenario
Incremental adoption with mixed societal impacts and ongoing ethical challenges
Key Drivers: Balanced regulatory approach, steady technological progress, and selective implementation based on clear ROI.
Probability: 50-60%
Conservative Scenario
Technical and ethical barriers creating significant implementation challenges
Key Drivers: Restrictive regulations, technical limitations, implementation challenges, and risk-averse organizational cultures.
Probability: 15-20%
Scenario Comparison Matrix
Factor | Optimistic | Base Case | Conservative |
---|---|---|---|
Implementation Timeline | Accelerated | Steady | Delayed |
Market Adoption | Widespread | Selective | Limited |
Technology Evolution | Rapid | Progressive | Incremental |
Regulatory Environment | Supportive | Balanced | Restrictive |
Business Impact | Transformative | Significant | Modest |
Transformational Impact
Redefinition of knowledge work, automation of creative processes. This evolution will necessitate significant changes in organizational structures, talent development, and strategic planning processes.
The convergence of multiple technological trends—including artificial intelligence, quantum computing, and ubiquitous connectivity—will create both unprecedented security challenges and innovative defensive capabilities.
Implementation Challenges
Ethical concerns, computing resource limitations, talent shortages. Organizations will need to develop comprehensive change management strategies to successfully navigate these transitions.
Regulatory uncertainty, particularly around emerging technologies like AI in security applications, will require flexible security architectures that can adapt to evolving compliance requirements.
Key Innovations to Watch
Multimodal learning, resource-efficient AI, transparent decision systems. Organizations should monitor these developments closely to maintain competitive advantages and effective security postures.
Strategic investments in research partnerships, technology pilots, and talent development will position forward-thinking organizations to leverage these innovations early in their development cycle.
Technical Glossary
Key technical terms and definitions to help understand the technologies discussed in this article.
Understanding the following technical concepts is essential for grasping the full implications of the security threats and defensive measures discussed in this article. These definitions provide context for both technical and non-technical readers.