Less supervision, better results: Study shows AI models generalize more effectively on their own - Related to better, supervision,, their, exploration, a
Less supervision, better results: Study shows AI models generalize more effectively on their own
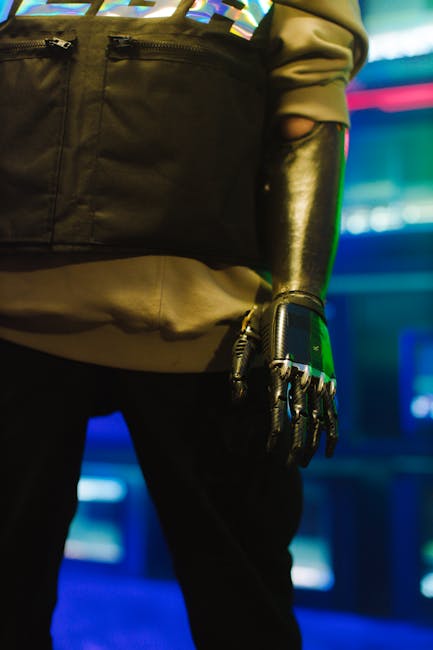
Language models can generalize superior when left to create their own solutions, a new study by Hong Kong University and University of California, Berkeley, exhibits. The findings, which apply to both large language models (LLMs) and vision language models (VLMs), challenge one of the main beliefs of the LLM community — that models require hand-labeled training examples. In fact, the researchers show that training models on too many hand-crafted examples can have adverse effects on the model’s ability to generalize to unseen data.
For a long time, supervised fine-tuning (SFT) has been the gold standard for training LLMs and VLMs. Once a model is pre-trained on raw text and image data, companies and AI labs usually post-train it on a large dataset of hand-crafted examples in question/answer or request/response format. After SFT, the model can undergo additional training stages, such as reinforcement learning from human feedback (RLHF), where the model tries to learn implicit human preferences based on signals such as answer rankings or liking/disliking the model’s responses.
SFT is useful for steering a model’s behavior toward the kind of tasks the model creators have designed it for. However, gathering the data is a slow and costly process, which is a bottleneck for many companies and labs.
Recent developments in LLMs have created interest in pure reinforcement learning (RL) approaches, where the model is given a task and left to learn it on its own without hand-crafted examples. The most critical instance is DeepSeek-R1, the OpenAI o1 competitor that mostly used reinforcement learning to learn complex reasoning tasks.
One of the key problems of machine learning (ML) systems is overfitting, where the model performs well on its training data but fails to generalize to unseen examples. During training, the model gives the false impression of having learned the task, while in practice it has just memorized its training examples. In large and complex AI models, separating generalization from memorization can be difficult.
The new study focuses on the generalization abilities of RL and SFT training in textual and visual reasoning tasks. For textual reasoning, an LLM trained on a set of rules should be able to generalize to variants of those rules. In visual reasoning, a VLM should remain consistent in task performance against changes to different aspects of visual input, such as color and spatial layout.
In their experiments, the researchers used two representative tasks. First was GeneralPoints, a benchmark that evaluates a model’s arithmetic reasoning capabilities. The model is given four cards, as textual descriptions or images, and is asked to combine them to reach a target number. For studying ruled-based generalization, the researchers trained the model using one set of rules, then evaluated it using a different rule. For visual generalization, they trained the model using cards of one color and tested its performance on cards of other colors and numbering schemes.
The second task is V-IRL, which tests the model’s spatial reasoning capabilities in an open-world navigation domain that uses realistic visual input. This task also comes in pure-language and vision-language versions. The researchers evaluated generalization by changing the kind of instructions and visual representations the model was trained and tested on.
They ran their tests on [website], warming the model up by training it on a small SFT dataset, then creating separate versions for each task and training paradigm. For each task, they separately scaled the training on RL and SFT. The SFT process trains the model on additional hand-crafted solutions, while RL lets the model generate many solutions for each problem, evaluate the results and train itself on the correct answers.
The findings show that reinforcement learning consistently improves performance on examples that are drastically different from training data. On the other hand, SFT seems to memorize the training rules and doesn’t generalize to out-of-distribution (OOD) examples. These observations apply to both text-only and multimodal settings.
SFT-trained models perform well on training examples (in-distribution) while showing poor performance on unseen examples (out-of-distribution) (source: arXiv).
Implications for real-world applications.
While their experiments show that RL is advanced at generalizing than SFT, the researchers also found that SFT is helpful for stabilizing the model’s output format, and is crucial to enabling RL to achieve its performance gains. The researchers found that, without the initial SFT stage, RL training did not achieve desirable results.
This is a bit different from the results obtained by DeepSeek-R1-Zero, which was post-trained on pure RL. The researchers suggest that this can be due to the different backbone model they used in their experiments.
It is clear that there is a lot of untapped potential in RL-heavy approaches. For use cases that have verifiable results, letting the models learn on their own can often lead to unanticipated results that humans could not have crafted themselves. This could come in very handy in settings where creating hand-crafed examples can be tedious and expensive.
Python has grown to dominate data science, and its package Pandas has become the go-to tool for data analysis. It is great for tabular data and suppor......
Prime Minister Narendra Modi met Google CEO Sundar Pichai at the AI Action Summit, which is currently underway in Paris. While highlighting India’s AI......
Meta is in talks to acquire FuriosaAI, a South Korean AI chip startup, to strengthen its custom chip efforts amid an NVIDIA GPU shortage. based on information from......
Virtualization & Containers for Data Science Newbies
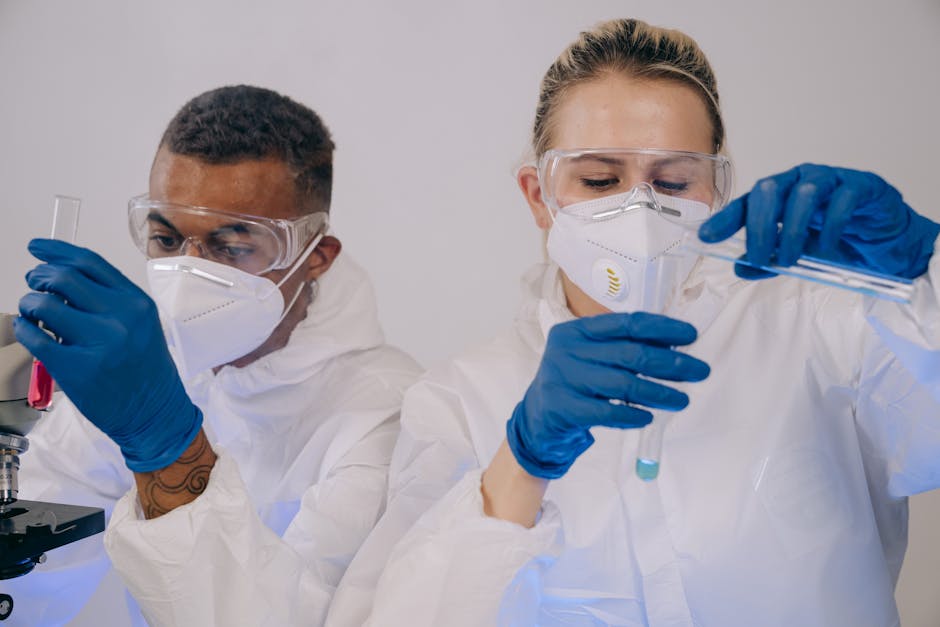
Virtualization makes it possible to run multiple virtual machines (VMs) on a single piece of physical hardware. These VMs behave like independent computers, but share the same physical computing power. A computer within a computer, so to speak.
Many cloud services rely on virtualization. But other technologies, such as containerization and serverless computing, have become increasingly significant.
Without virtualization, many of the digital services we use every day would not be possible. Of course, this is a simplification, as some cloud services also use bare-metal infrastructures.
In this article, you will learn how to set up your own virtual machine on your laptop in just a few minutes — even if you have never heard of Cloud Computing or containers before.
1 — The Origins of Cloud Computing: From Mainframes to Serverless Architecture.
2 — Understanding Virtualization: Why it’s the Basis of Cloud Computing.
3 — Create a Virtual Machine with VirtualBox.
1 — The Origins of Cloud Computing: From Mainframes to Serverless Architecture.
Cloud computing has fundamentally changed the IT landscape — but its roots go back much further than many people think. In fact, the history of the cloud began back in the 1950s with huge mainframes and so-called dumb terminals.
The era of mainframes in the 1950s: Companies used mainframes so that several individuals could access them simultaneously via dumb terminals. The central mainframes were designed for high-volume, business-critical data processing. Large companies still use them today, even if cloud services have reduced their relevance.
Companies used mainframes so that several consumers could access them simultaneously via dumb terminals. The central mainframes were designed for high-volume, business-critical data processing. Large companies still use them today, even if cloud services have reduced their relevance. Time-sharing and virtualization: In the next decade (1960s), time-sharing made it possible for multiple consumers to access the same computing power simultaneously — an early model of today’s cloud. Around the same time, IBM pioneered virtualization, allowing multiple virtual machines to run on a single piece of hardware.
In the next decade (1960s), time-sharing made it possible for multiple customers to access the same computing power simultaneously — an early model of today’s cloud. Around the same time, IBM pioneered virtualization, allowing multiple virtual machines to run on a single piece of hardware. The birth of the internet and web-based applications in the 1990s: Six years before I was born, Tim Berners-Lee developed the World Wide Web, which revolutionized online communication and our entire working and living environment. Can you imagine our lives today without internet? At the same time, PCs were becoming increasingly popular. In 1999, Salesforce revolutionized the software industry with Software as a Service (SaaS), allowing businesses to use CRM solutions over the internet without local installations.
Six years before I was born, Tim Berners-Lee developed the World Wide Web, which revolutionized online communication and our entire working and living environment. Can you imagine our lives today without internet? At the same time, PCs were becoming increasingly popular. In 1999, Salesforce revolutionized the software industry with Software as a Service (SaaS), allowing businesses to use CRM solutions over the internet without local installations. The big breakthrough of cloud computing in the 2010s:
The modern cloud era began in 2006 with Amazon Web Services (AWS): Companies were able to flexibly rent infrastructure with S3 (storage) and EC2 (virtual servers) instead of buying their own servers. Microsoft Azure and Google Cloud followed with PaaS and IaaS services.
The modern cloud era began in 2006 with Amazon Web Services (AWS): Companies were able to flexibly rent infrastructure with S3 (storage) and EC2 (virtual servers) instead of buying their own servers. Microsoft Azure and Google Cloud followed with PaaS and IaaS services. The modern cloud-native era: This was followed by the next innovation with containerization. Docker made Containers popular in 2013, followed by Kubernetes in 2014 to simplify the orchestration of containers. Next came serverless computing with AWS Lambda and Google Cloud Functions, which enabled developers to write code that automatically responds to events. The infrastructure is fully managed by the cloud provider.
Cloud computing is more the result of decades of innovation than a single new technology. From time-sharing to virtualization to serverless architectures, the IT landscape has continuously evolved. Today, cloud computing is the foundation for streaming services like Netflix, AI applications like ChatGPT and global platforms like Salesforce.
2 — Understanding Virtualization: Why Virtualization is the Basis of Cloud Computing.
Virtualization means abstracting physical hardware, such as servers, storage or networks, into multiple virtual instances.
Several independent systems can be operated on the same physical infrastructure. Instead of dedicating an entire server to a single application, virtualization enables multiple workloads to share resources efficiently. For example, Windows, Linux or another environment can be run simultaneously on a single laptop — each in an isolated virtual machine.
Even more critical, however, is the scalability: Infrastructure can be flexibly adapted to changing requirements.
Before cloud computing became widely available, companies often had to maintain dedicated servers for different applications, leading to high infrastructure costs and limited scalability. If more performance was suddenly required, for example because webshop traffic increased, new hardware was needed. The corporation had to add more servers (horizontal scaling) or upgrade existing ones (vertical scaling).
This is different with virtualization: For example, I can simply upgrade my virtual Linux machine from 8 GB to 16 GB RAM or assign 4 cores instead of 2. Of course, only if the underlying infrastructure supports this. More on this later.
And this is exactly what cloud computing makes possible: The cloud consists of huge data centers that use virtualization to provide flexible computing power — exactly when it is needed. So, virtualization is a fundamental technology behind cloud computing.
What if you didn’t even have to manage virtual machines anymore?
Serverless computing goes one step further than Virtualization and containerization. The cloud provider handles most infrastructure tasks — including scaling, maintenance and resource allocation. Developers should focus on writing and deploying code.
But does serverless really mean that there are no more servers?
Of course not. The servers are there, but they are invisible for the user. Developers no longer have to worry about them. Instead of manually provisioning a virtual machine or container, you simply deploy your code, and the cloud automatically executes it in a managed environment. Resources are only provided when the code is running. For example, you can use AWS Lambda, Google Cloud Functions or Azure Functions.
As a developer, you don’t have to worry about scaling or maintenance. This means that if there is a lot more traffic at a particular event, the resources are automatically adjusted. Serverless computing can be cost-efficient, especially in Function-as-a-Service (FaaS) models. If nothing is running, you pay nothing. However, some serverless services have baseline costs ([website] Firestore).
You have much less control over the infrastructure and no direct access to the servers. There is also a risk of vendor lock-in. The applications are strongly tied to a cloud provider.
A concrete example of serverless: API without your own server.
Imagine you have a website with an API that provides individuals with the current weather. Normally, a server runs around the clock — even at times when no one is using the API.
3 — What Data Scientists should Know about Containers and VMs — What’s the Difference?
You’ve probably heard of containers. But what is the difference to virtual machines — and what is particularly relevant as a data scientist?
Both containers and virtual machines are virtualization technologies.
Both make it possible to run applications in isolation.
Both offer advantages depending on the use case: While VMs provide strong security, containers excel in speed and efficiency.
The main difference lies in the architecture:
Virtual machines virtualize the entire hardware — including the operating system. Each VM has its own operational system (OS). This in turn requires more memory and resources.
Containers, on the other hand, share the host operating system and only virtualize the application layer. This makes them significantly lighter and faster.
Put simply, virtual machines simulate entire computers, while containers only encapsulate applications.
Why is this crucial for data scientists?
Since as a data scientist you will come into contact with machine learning, data engineering or data pipelines, it is also critical to understand something about containers and virtual machines. Sure, you don’t need to have in-depth knowledge of it like a DevOps Engineer or a Site Reliability Engineer (SRE).
Virtual machines are used in data science, for example, when a complete operating system environment is required — such as a Windows VM on a Linux host. Data science projects often need specific environments. With a VM, it is possible to provide exactly the same environment — regardless of which host system is available.
A VM is also needed when training deep learning models with GPUs in the cloud. With cloud VMs such as AWS EC2 or Azure Virtual Machines, you have the option of training the models with GPUs. VMs also completely separate different workloads from each other to ensure performance and security.
Containers are used in data science for data pipelines, for example, where tools such as Apache Airflow run individual processing steps in Docker containers. This means that each step can be executed in isolation and independently of each other — regardless of whether it involves loading, transforming or saving data. Even if you want to deploy machine learning models via Flask / FastAPI, a container ensures that everything your model needs ([website] Python libraries, framework versions) runs exactly as it should. This makes it super easy to deploy the model on a server or in the cloud.
3 — Create a Virtual Machine with VirtualBox.
Let’s make this a little more concrete and create an Ubuntu VM. 🚀.
I use the VirtualBox software with my Windows Lenovo laptop. The virtual machine runs in isolation from your main operating system so that no changes are made to your actual system. If you have Windows Pro Edition, you can also enable Hyper-V (pre-installed by default, but disabled). With an Intel Mac, you should also be able to use VirtualBox. With an Apple Silicon, Parallels Desktop or UTM is apparently the superior alternative (not tested myself).
The first step is to download the installation file for VirtualBox from the official Virtual Box website and install VirtualBox. VirtualBox is installed including all necessary drivers.
Then we start the Oracle VirtualBox Manager:
Next, we download the Ubuntu ISO file from the Ubuntu website. An ISO Ubuntu file is a compressed image file of the Ubuntu operating system. This means that it contains a complete copy of the installation data. I download the LTS version because this version receives security and maintenance updates for 5 years (Long Term Support). Note the location of the .iso file as we will use it later in VirtualBox.
3) Create a virtual machine in VirtualBox.
Next, we create a new virtual machine in the VirtualBox Manager and give it the name Ubuntu VM 2025. Here we select Linux as the type and Ubuntu (64-bit) as the version. We also select the previously downloaded ISO file from Ubuntu as the ISO image. It would also be possible to add the ISO file later in the mass storage menu.
Next, we select a user name vboxuser2025 and a password for access to the Ubuntu system. The hostname is the name of the virtual machine within the network or system. It must not contain any spaces. The domain name is optional and would be used if the network has multiple devices.
We then assign the appropriate resources to the virtual machine. I choose 8 GB (8192 MB) RAM, as my host system has 64 GB RAM. I recommend 4GB (4096) as a minimum. I assign 2 processors, as my host system has 8 cores and 16 logical processors. It would also be possible to assign 4 cores, but this way I have enough resources for my host system. You can find out how many cores your host system has by opening the Task Manager in Windows and looking at the number of cores under the Performance tab under CPU.
We can then see that the virtual machine has been created and can be used:
We can now use the newly created virtual machine like a normal separate operating system. The VM is completely isolated from the host system. This means you can experiment in it without changing or jeopardizing your main system.
If you are new to Linux, you can try out basic commands like ls, cd, mkdir or sudo to get to know the terminal. As a data scientist, you can set up your own development environments, install Python with Pandas and Scikit-learn to develop data analysis and machine learning models. Or you can install PostgreSQL and run SQL queries without having to set up a local database on your main system. You can also use Docker to create containerized applications.
Since the VM is isolated, we can install programs, experiment and even destroy the system without affecting the host system.
Let’s see if virtual machines remain relevant in the coming years. As companies increasingly use microservice architectures (instead of monoliths), containers with Docker and Kubernetes will certainly become even more significant. But knowing how to set up a virtual machine and what it is used for is certainly useful.
I simplify tech for curious minds. If you enjoy my tech insights on Python, data science, data engineering, machine learning and AI, consider subscribing to my substack.
AI search engine Perplexity says its latest release goes above and beyond for user satisfaction -- especially compared to OpenA......
iPhone individuals can now tap into Google's Deep Research agent to research a topic on their behalf. Added to the Gemini website in December and to ......
L’IA chinoise fait trembler le marché. DeepSeek affirme avoir développé un modèle IA révolutionnaire. Son modèle serait formé à un coût réduit avec du......
Understanding Model Calibration: A Gentle Introduction & Visual Exploration
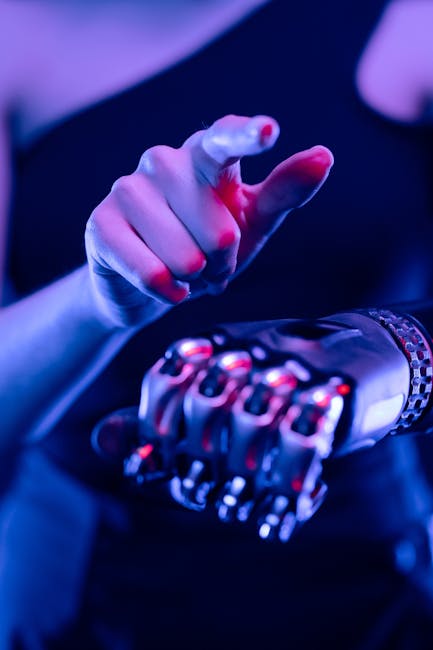
To be considered reliable, a model must be calibrated so that its confidence in each decision closely reflects its true outcome. In this blog post we’ll take a look at the most commonly used definition for calibration and then dive into a frequently used evaluation measure for Model Calibration. We’ll then cover some of the drawbacks of this measure and how these surfaced the need for additional notions of calibration, which require their own new evaluation measures. This post is not intended to be an in-depth dissection of all works on calibration, nor does it focus on how to calibrate models. Instead, it is meant to provide a gentle introduction to the different notions and their evaluation measures as well as to re-highlight some issues with a measure that is still widely used to evaluate calibration.
Calibration makes sure that a model’s estimated probabilities match real-world outcomes. For example, if a weather forecasting model predicts a 70% chance of rain on several days, then roughly 70% of those days should actually be rainy for the model to be considered well calibrated. This makes model predictions more reliable and trustworthy, which makes calibration relevant for many applications across various domains.
Now, what calibration means more precisely depends on the specific definition being considered. We will have a look at the most common notion in machine learning (ML) formalised by Guo and termed confidence calibration by Kull. But first, let’s define a bit of formal notation for this blog.
In this blog post we consider a classification task with K possible classes, with labels Y ∈ {1, …, K} and a classification model p̂ :𝕏 → Δᴷ, that takes inputs in 𝕏 ([website] an image or text) and returns a probability vector as its output. Δᴷ refers to the K-simplex, which just means that the output vector must sum to 1 and that each estimated probability in the vector is between 0 & 1. These individual probabilities (or confidences) indicate how likely an input belongs to each of the K classes.
Notation — image by author — input example sourced from Uma.
A model is considered confidence-calibrated if, for all confidences c, the model is correct c proportion of the time:
where (X,Y) is a datapoint and p̂ : 𝕏 → Δᴷ returns a probability vector as its output.
This definition of calibration, ensures that the model’s final predictions align with their observed accuracy at that confidence level. The left chart below visualises the perfectly calibrated outcome (green diagonal line) for all confidences using a binned reliability diagram. On the right hand side it demonstrates two examples for a specific confidence level across 10 samples.
Confidence Calibration — image by author.
For simplification, we assume that we only have 3 classes as in image 2 (Notation) and we zoom into confidence [website], see image above. Let’s assume we have 10 inputs here whose most confident prediction (max) equals [website] If the model correctly classifies 7 out of 10 predictions (true), it is considered calibrated at confidence level [website] For the model to be fully calibrated this has to hold across all confidence levels from 0 to 1. At the same level [website], a model would be considered miscalibrated if it makes only 4 correct predictions.
2 Evaluating Calibration — Expected Calibration Error (ECE).
One widely used evaluation measure for confidence calibration is the Expected Calibration Error (ECE). ECE measures how well a model’s estimated probabilities match the observed probabilities by taking a weighted average over the absolute difference between average accuracy (acc) and average confidence (conf). The measure involves splitting all n datapoints into M equally spaced bins:
where B is used for representing “bins” and m for the bin number, while acc and conf are:
ŷᵢ is the model’s predicted class (arg max) for sample i and yᵢ is the true label for sample i. 1 is an indicator function, meaning when the predicted label ŷᵢ equals the true label yᵢ it evaluates to 1, otherwise 0. Let’s look at an example, which will clarify acc, conf and the whole binning approach in a visual step-by-step manner.
[website] ECE — Visual Step by Step Example.
In the image below, we can see that we have 9 samples indexed by i with estimated probabilities p̂(xᵢ) (simplified as p̂ᵢ) for class cat (C), dog (D) or toad (T). The final column reveals the true class yᵢ and the penultimate column contains the predicted class ŷᵢ.
Table 1 — ECE toy example — image by author.
Only the maximum probabilities, which determine the predicted label are used in ECE. Therefore, we will only bin samples based on the maximum probability across classes (see left table in below image). To keep the example simple we split the data into 5 equally spaced bins M=5. If we now look at each sample’s maximum estimated probability, we can group it into one of the 5 bins (see right side of image below).
Table 2 & Binning Diagram — image by author.
We still need to determine if the predicted class is correct or not to be able to determine the average accuracy per bin. If the model predicts the class correctly ([website] yᵢ = ŷᵢ), the prediction is highlighted in green; incorrect predictions are marked in red:
Table 3 & Binning Diagram — image by author.
We now have visualised all the information needed for ECE and will briefly run through how to.
calculate the values for bin 5 (B₅). The other bins then simply follow the same process, see below.
Table 4 & Example for bin 5 — image by author.
We can get the empirical probability of a sample falling into B₅, by assessing how many out of all 9 samples fall into B₅, see ( 1 ). We then get the average accuracy for B₅, see ( 2 ) and lastly the average estimated probability for B₅, see ( 3 ). Repeat this for all bins and in our small example of 9 samples we end up with an ECE of [website] A perfectly calibrated model would have an ECE of 0.
For a more detailed, step-by-step explanation of the ECE, have a look at this blog post.
[website] EXPECTED CALIBRATION ERROR DRAWBACKS.
The images of binning above provide a visual guide of how ECE could result in very different values if we used more bins or perhaps binned the same number of items instead of using equal bin widths. Such and more drawbacks of ECE have been highlighted by several works early on. However, despite the known weaknesses ECE is still widely used to evaluate confidence calibration in ML.
3 Most frequently mentioned Drawbacks of ECE.
[website] Pathologies — Low ECE ≠ high accuracy.
A model which minimises ECE, does not necessarily have a high accuracy. For instance, if a model always predicts the majority class with that class’s average prevalence as the probability, it will have an ECE of 0. This is visualised in the image above, where we have a dataset with 10 samples, 7 of those are cat, 2 dog and only one is a toad. Now if the model always predicts cat with on average [website] confidence it would have an ECE of 0. There are more of such pathologies. To not only rely on ECE, some researchers use additional measures such as the Brier score or LogLoss alongside ECE.
One of the most frequently mentioned issues with ECE is its sensitivity to the change in binning. This is sometimes referred to as the Bias-Variance trade-off: Fewer bins reduce variance but increase bias, while more bins lead to sparsely populated bins increasing variance. If we look back to our ECE example with 9 samples and change the bins from 5 to 10 here too, we end up with the following:
We can see that bin 8 and 9 each contain only a single sample and also that half the bins now contain no samples. The above is only a toy example, however since modern models tend to have higher confidence values samples often end up in the last few bins, which means they get all the weight in ECE, while the average error for the empty bins contributes 0 to ECE.
To mitigate these issues of fixed bin widths some authors have proposed a more adaptive binning approach:
Binning-based evaluation with bins containing an equal number of samples are shown to have lower bias than a fixed binning approach such as ECE. This leads Roelofs to urge against using equal width binning and they suggest the use of an alternative: ECEsweep, which maximizes the number of equal-mass bins while ensuring the calibration function remains monotonic. The Adaptive Calibration Error (ACE) and Threshold Adaptive calibration Error (TACE) are two other variations of ECE that use flexible binning. However, some find it sensitive to the choice of bins and thresholds, leading to inconsistencies in ranking different models. Two other approaches aim to eliminate binning altogether: MacroCE does this by averaging over instance-level calibration errors of correct and wrong predictions and the KDE-based ECE does so by replacing the bins with non-parametric density estimators, specifically kernel density estimation (KDE).
[website] Only maximum probabilities considered.
Another frequently mentioned drawback of ECE is that it only considers the maximum estimated probabilities. The idea that more than just the maximum confidence should be calibrated, is best illustrated with a simple example:
Only Max. Probabilities — image by author — input example sourced from Schwirten.
Let’s say we trained two different models and now both need to determine if the same input image contains a person, an animal or no creature. The two models output vectors with slightly different estimated probabilities, but both have the same maximum confidence for “no creature”. Since ECE only looks at these top values it would consider these two outputs to be the same. Yet, when we think of real-world applications we might want our self-driving car to act differently in one situation over the other. This restriction to the maximum confidence prompted various authors to reconsider the definition of calibration, which gives us two additional interpretations of confidence: multi-class and class-wise calibration.
A model is considered multi-class calibrated if, for any prediction vector q=(q₁,…,qₖ) ∈ Δᴷ, the class proportions among all values of X for which a model outputs the same prediction p̂(X)=q match the values in the prediction vector q.
where (X,Y) is a datapoint and p̂ : 𝕏 → Δᴷ returns a probability vector as its output.
What does this mean in simple terms? Instead of c we now calibrate against a vector q, with k classes. Let’s look at an example below:
Multi-Class Calibration — image by author.
On the left we have the space of all possible prediction vectors. Let’s zoom into one such vector that our model predicted and say the model has 10 instances for which it predicted the vector q=[[website],[website],[website]]. Now in order for it to be multi-class calibrated, the distribution of the true (actual) class needs to match the prediction vector q. The image above presents a calibrated example with [[website],[website],[website]] and a not calibrated case with [[website],[website],[website]].
A model is considered class-wise calibrated if, for each class k, all inputs that share an estimated probability p̂ₖ(X) align with the true frequency of class k when considered on its own:
where (X,Y) is a datapoint; q ∈ Δᴷ and p̂ : 𝕏 → Δᴷ returns a probability vector as its output.
Class-wise calibration is a weaker definition than multi-class calibration as it considers each class probability in isolation rather than needing the full vector to align. The image below illustrates this by zooming into a probability estimate for class 1 specifically: q₁[website] Yet again, we assume we have 10 instances for which the model predicted a probability estimate of [website] for class 1. We then look at the true class frequency amongst all classes with q₁[website] If the empirical frequency matches q₁ it is calibrated.
Class-Wise Calibration — image by author.
To evaluate such different notions of calibration, some updates are made to ECE to calculate a class-wise error. One idea is to calculate the ECE for each class and then take the average. Others, introduce the use of the KS-test for class-wise calibration and also suggest using statistical hypothesis tests instead of ECE based approaches. And other researchers develop a hypothesis test framework (TCal) to detect whether a model is significantly mis-calibrated and build on this by developing confidence intervals for the L2 ECE.
All the approaches mentioned above share a key assumption: ground-truth labels are available. Within this gold-standard mindset a prediction is either true or false. However, annotators might unresolvably and justifiably disagree on the real label. Let’s look at a simple example below:
Gold-Standard Labelling | One-Hot-Vector — image by author.
We have the same image as in our entry example and can see that the chosen label differs between annotators. A common approach to resolving such issues in the labelling process is to use some form of aggregation. Let’s say that in our example the majority vote is selected, so we end up evaluating how well our model is calibrated against such ‘ground truth’. One might think, the image is small and pixelated; of course humans will not be certain about their choice. However, rather than being an exception such disagreements are widespread. So, when there is a lot of human disagreement in a dataset it might not be a good idea to calibrate against an aggregated ‘gold’ label. Instead of gold labels more and more researchers are using soft or smooth labels which are more representative of the human uncertainty, see example below:
Collective Opinion Labelling | Soft-label — image by author.
In the same example as above, instead of aggregating the annotator votes we could simply use their frequencies to create a distribution Pᵥₒₜₑ over the labels instead, which is then our new yᵢ. This shift towards training models on collective annotator views, rather than relying on a single source-of-truth motivates another definition of calibration: calibrating the model against human uncertainty.
A model is considered human-uncertainty calibrated if, for each specific sample x, the predicted probability for each class k matches the ‘actual’ probability Pᵥₒₜₑ of that class being correct.
where (X,Y) is a datapoint and p̂ : 𝕏 → Δᴷ returns a probability vector as its output.
This interpretation of calibration aligns the model’s prediction with human uncertainty, which means each prediction made by the model is individually reliable and matches human-level uncertainty for that instance. Let’s have a look at an example below:
Human Uncertainty Calibration — image by author.
We have our sample data (left) and zoom into a single sample x with index i=1. The model’s predicted probability vector for this sample is [[website],[website],[website]]. If the human labelled distribution yᵢ matches this predicted vector then this sample is considered calibrated.
This definition of calibration is more granular and strict than the previous ones as it applies directly at the level of individual predictions rather than being averaged or assessed over a set of samples. It also relies heavily on having an accurate estimate of the human judgement distribution, which requires a large number of annotations per item. Datasets with such properties of annotations are gradually becoming more available.
To evaluate human uncertainty calibration the researchers introduce three new measures: the Human Entropy Calibration Error (EntCE), the Human Ranking Calibration Score (RankCS) and the Human Distribution Calibration Error (DistCE).
EntCE aims to capture the agreement between the model’s uncertainty H(p̂ᵢ) and the human uncertainty H(yᵢ) for a sample i. However, entropy is invariant to the permutations of the probability values; in other words it doesn’t change when you rearrange the probability values. This is visualised in the image below:
On the left, we can see the human label distribution yᵢ, on the right are two different model predictions for that same sample. All three distributions would have the same entropy, so comparing them would result in 0 EntCE. While this is not ideal for comparing distributions, entropy is still helpful in assessing the noise level of label distributions.
where argsort simply returns the indices that would sort an array.
So, RankCS checks if the sorted order of estimated probabilities p̂ᵢ matches the sorted order of yᵢ for each sample. If they match for a particular sample i one can count it as 1; if not, it can be counted as 0, which is then used to average over all samples N.¹.
Since this approach uses ranking it doesn’t care about the actual size of the probability values. The two predictions below, while not the same in class probabilities would have the same ranking. This is helpful in assessing the overall ranking capability of models and looks beyond just the maximum confidence. At the same time though, it doesn’t fully capture human uncertainty calibration as it ignores the actual probability values.
DistCE has been proposed as an additional evaluation for this notion of calibration. It simply uses the total variation distance (TVD) between the two distributions, which aims to reflect how much they diverge from one another. DistCE and EntCE capture instance level information. So to get a feeling for the full dataset one can simply take the average expected value over the absolute value of each measure: E[∣DistCE∣] and E[∣EntCE∣]. Perhaps future efforts will introduce further measures that combine the benefits of ranking and noise estimation for this notion of calibration.
We have run through the most common definition of calibration, the shortcomings of ECE and how several new notions of calibration exist. We also touched on some of the newly proposed evaluation measures and their shortcomings. Despite several works arguing against the use of ECE for evaluating calibration, it remains widely used. The aim of this blog post is to draw attention to these works and their alternative approaches. Determining which notion of calibration best fits a specific context and how to evaluate it should avoid misleading results. Maybe, however, ECE is simply so easy, intuitive and just good enough for most applications that it is here to stay?
This was accepted at the ICLR conference Blog Post Track & is estimated to appear on the site ~ April.
In the meantime, you can cite/reference the ArXiv preprint.
¹In the paper it is stated more generally: If the argsorts match, it means the ranking is aligned, contributing to the overall RankCS score.
A research study commissioned by computing giant IBM revealed on Wednesday that most Indian companies made significant progress in executing their AI ......
AI video generators unlock new possibilities for creatives, allowing them to bring their ideas to video form with a quick prompt or reference i......
Market Impact Analysis
Market Growth Trend
2018 | 2019 | 2020 | 2021 | 2022 | 2023 | 2024 |
---|---|---|---|---|---|---|
23.1% | 27.8% | 29.2% | 32.4% | 34.2% | 35.2% | 35.6% |
Quarterly Growth Rate
Q1 2024 | Q2 2024 | Q3 2024 | Q4 2024 |
---|---|---|---|
32.5% | 34.8% | 36.2% | 35.6% |
Market Segments and Growth Drivers
Segment | Market Share | Growth Rate |
---|---|---|
Machine Learning | 29% | 38.4% |
Computer Vision | 18% | 35.7% |
Natural Language Processing | 24% | 41.5% |
Robotics | 15% | 22.3% |
Other AI Technologies | 14% | 31.8% |
Technology Maturity Curve
Different technologies within the ecosystem are at varying stages of maturity:
Competitive Landscape Analysis
Company | Market Share |
---|---|
Google AI | 18.3% |
Microsoft AI | 15.7% |
IBM Watson | 11.2% |
Amazon AI | 9.8% |
OpenAI | 8.4% |
Future Outlook and Predictions
The Less Supervision Better landscape is evolving rapidly, driven by technological advancements, changing threat vectors, and shifting business requirements. Based on current trends and expert analyses, we can anticipate several significant developments across different time horizons:
Year-by-Year Technology Evolution
Based on current trajectory and expert analyses, we can project the following development timeline:
Technology Maturity Curve
Different technologies within the ecosystem are at varying stages of maturity, influencing adoption timelines and investment priorities:
Innovation Trigger
- Generative AI for specialized domains
- Blockchain for supply chain verification
Peak of Inflated Expectations
- Digital twins for business processes
- Quantum-resistant cryptography
Trough of Disillusionment
- Consumer AR/VR applications
- General-purpose blockchain
Slope of Enlightenment
- AI-driven analytics
- Edge computing
Plateau of Productivity
- Cloud infrastructure
- Mobile applications
Technology Evolution Timeline
- Improved generative models
- specialized AI applications
- AI-human collaboration systems
- multimodal AI platforms
- General AI capabilities
- AI-driven scientific breakthroughs
Expert Perspectives
Leading experts in the ai tech sector provide diverse perspectives on how the landscape will evolve over the coming years:
"The next frontier is AI systems that can reason across modalities and domains with minimal human guidance."
— AI Researcher
"Organizations that develop effective AI governance frameworks will gain competitive advantage."
— Industry Analyst
"The AI talent gap remains a critical barrier to implementation for most enterprises."
— Chief AI Officer
Areas of Expert Consensus
- Acceleration of Innovation: The pace of technological evolution will continue to increase
- Practical Integration: Focus will shift from proof-of-concept to operational deployment
- Human-Technology Partnership: Most effective implementations will optimize human-machine collaboration
- Regulatory Influence: Regulatory frameworks will increasingly shape technology development
Short-Term Outlook (1-2 Years)
In the immediate future, organizations will focus on implementing and optimizing currently available technologies to address pressing ai tech challenges:
- Improved generative models
- specialized AI applications
- enhanced AI ethics frameworks
These developments will be characterized by incremental improvements to existing frameworks rather than revolutionary changes, with emphasis on practical deployment and measurable outcomes.
Mid-Term Outlook (3-5 Years)
As technologies mature and organizations adapt, more substantial transformations will emerge in how security is approached and implemented:
- AI-human collaboration systems
- multimodal AI platforms
- democratized AI development
This period will see significant changes in security architecture and operational models, with increasing automation and integration between previously siloed security functions. Organizations will shift from reactive to proactive security postures.
Long-Term Outlook (5+ Years)
Looking further ahead, more fundamental shifts will reshape how cybersecurity is conceptualized and implemented across digital ecosystems:
- General AI capabilities
- AI-driven scientific breakthroughs
- new computing paradigms
These long-term developments will likely require significant technical breakthroughs, new regulatory frameworks, and evolution in how organizations approach security as a fundamental business function rather than a technical discipline.
Key Risk Factors and Uncertainties
Several critical factors could significantly impact the trajectory of ai tech evolution:
Organizations should monitor these factors closely and develop contingency strategies to mitigate potential negative impacts on technology implementation timelines.
Alternative Future Scenarios
The evolution of technology can follow different paths depending on various factors including regulatory developments, investment trends, technological breakthroughs, and market adoption. We analyze three potential scenarios:
Optimistic Scenario
Responsible AI driving innovation while minimizing societal disruption
Key Drivers: Supportive regulatory environment, significant research breakthroughs, strong market incentives, and rapid user adoption.
Probability: 25-30%
Base Case Scenario
Incremental adoption with mixed societal impacts and ongoing ethical challenges
Key Drivers: Balanced regulatory approach, steady technological progress, and selective implementation based on clear ROI.
Probability: 50-60%
Conservative Scenario
Technical and ethical barriers creating significant implementation challenges
Key Drivers: Restrictive regulations, technical limitations, implementation challenges, and risk-averse organizational cultures.
Probability: 15-20%
Scenario Comparison Matrix
Factor | Optimistic | Base Case | Conservative |
---|---|---|---|
Implementation Timeline | Accelerated | Steady | Delayed |
Market Adoption | Widespread | Selective | Limited |
Technology Evolution | Rapid | Progressive | Incremental |
Regulatory Environment | Supportive | Balanced | Restrictive |
Business Impact | Transformative | Significant | Modest |
Transformational Impact
Redefinition of knowledge work, automation of creative processes. This evolution will necessitate significant changes in organizational structures, talent development, and strategic planning processes.
The convergence of multiple technological trends—including artificial intelligence, quantum computing, and ubiquitous connectivity—will create both unprecedented security challenges and innovative defensive capabilities.
Implementation Challenges
Ethical concerns, computing resource limitations, talent shortages. Organizations will need to develop comprehensive change management strategies to successfully navigate these transitions.
Regulatory uncertainty, particularly around emerging technologies like AI in security applications, will require flexible security architectures that can adapt to evolving compliance requirements.
Key Innovations to Watch
Multimodal learning, resource-efficient AI, transparent decision systems. Organizations should monitor these developments closely to maintain competitive advantages and effective security postures.
Strategic investments in research partnerships, technology pilots, and talent development will position forward-thinking organizations to leverage these innovations early in their development cycle.
Technical Glossary
Key technical terms and definitions to help understand the technologies discussed in this article.
Understanding the following technical concepts is essential for grasping the full implications of the security threats and defensive measures discussed in this article. These definitions provide context for both technical and non-technical readers.