Ansys says simulations will close the gap with reality and make the world more sustainable - Related to says, do, tasks, it, ansys
5 ways AI can help you do your taxes - and 10 tax tasks you shouldn't trust it with
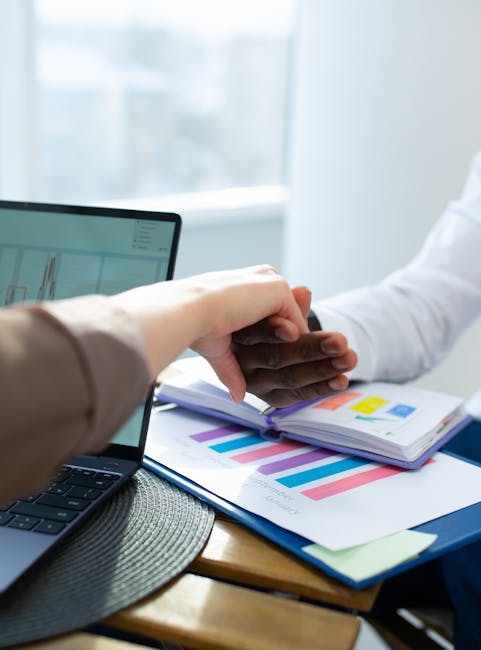
In a recent test of ChatGPT's Deep Research feature, the AI was asked to identify 20 jobs that OpenAI's new o3 model was likely to replace. As ZDNET's Sabrina Ortiz reported, "Right in time with tax return season, leading the table was the role of 'tax preparer' with a probability of 98% replacement, which ChatGPT deemed as 'near-certain automation.'"
There is no doubt that retail tax preparation services are using some level of AI to reduce their workload, but while tax preparers may well be replaced by a machine, I'm not convinced that will lead to accurate or reliable tax returns -- certainly not yet.
Also: From zero to millions? How regular people are cashing in on AI.
For example, there may come a time when you'll be able to tell an AI to find all your receipts and transactions, divide them into categories, identify which categories your deductions are in, and then enter them into the appropriate tax forms and bookkeeping systems. But that series of linked activities does not appear to be available now.
For those who already keep their records in a clear and organized manner, AI might help. But in my first year as a business owner, I kept all -- all -- my paperwork in a big duffle bag. When tax time came, I just dumped the duffle bag on the desk of my local tax preparer. Turning that disaster into a good tax return was a massive by-hand effort.
My business made very little that first year, but the fee I had to pay that preparer to excavate my documents and prepare my taxes was breathtaking. After learning my lesson, I got more rigorous about organizing my records. My point is that young entrepreneur me is not the only person with sloppy documentation. Some jobs will always require a human for at least some of the work.
Also: The best AI for coding in 2025 (and what not to use).
Even with good organization and rigorous bookkeeping (which I've done religiously for a few decades now), the various tools that we need to work together are usually from different vendors. The AIs are just not up to that level of organization across a wide range of documents and activities.
That presented, there are areas where an AI can help. Here are five of them.
1. Use AI attributes available in tax prep software.
Tax programs like TurboTax and H&R Block tax software now offer varying degrees of AI assistance for your tax preparation. Don't expect your tax preparation software to do all the work for you, but it can help save time and provide some quick assistance.
Intuit has several AI-related offerings that are part of its TurboTax product. TurboTax can import data from 350 financial institutions and can auto-fill tax form fields using a tool called IntuitAssist. The AI is also used to check forms for accuracy and find errors, recommend deductions, and answer tax questions.
Also: This is the best money management app I've tested.
Intuit is also pitching a TurboTax Live Assisted program where an AI will match you with a tax expert who will work with you in a Zoom-like call to fill out your taxes. This is sort of a mix of artificial and real intelligence.
H&R Block Tax Assist is a new generative AI tool that can provide tax information, help with tax preparation, and answer free-form tax-related questions to help you understand the tax issues you're dealing with as you complete your returns.
H&R Block also says its Tax Assistant can answer questions about recent changes to tax code, but be careful because AI knowledge tends to lag a bit behind real-time regulation changes.
Now, all of this might sound good, but keep in mind that generative AI has the tendency to make mistakes, make stuff up, and mislead. That's not exactly what you want when preparing taxes. Geoffrey A. Fowler of the Washington Post provides a cautionary tale. He tried both TurboTax and H&R Block's AI features and found them to be "awful."
Also: Stop paying full price for PCs and Macs: 7 ways to save money.
To be fair, I've paid real-world human accountants for tax prep help, and have found some of them to be awful, too. Taxes aren't fun, and you have to double-check everything, whether it's your own work, the work of an AI, or the work of someone who indicates to be an expert.
2. Use AI capabilities in expense tracking software.
Not all expense tracking services offer AI elements, but Fyle, SparkReceipt, and QuickBooks do.
I am a somewhat involuntary QuickBooks user. The price has gone up considerably over the years, but the switching costs are even higher. So I stick with QuickBooks. For imported expenses that don't have custom rules, QuickBooks attempts to assign categories using some AI capabilities. Don't count on this feature. Those assignments are almost always incorrect.
QuickBooks also constantly pushes its related services, some of which have AI capabilities. But I haven't found anything on offer that seems worth the upsell, so I haven't tapped into those additional AI capabilities.
Also: The US Copyright Office's new ruling on AI art is here - and it could change everything.
Fyle's big claim to fame is what it calls Conversational AI for Expense Tracking. Basically, all you do is snap a picture of your receipts with your phone and text it to Fyle. Fyle processes it in and categorizes it all automatically, saving a lot of time.
SparkReceipt also automates receipt scanning and categorization, along with invoices and bank statements. It will then enter your information, without the need for manual entry. The key feature here is the categorization of expenses, which can often take both time and effort to do by hand.
Microsoft's Copilot has powerful integration with Excel. No matter what data you're organizing for your tax filing or accounting process, some of it is likely to be run through Excel. Copilot will automate many of the Excel setup tasks that used to take a lot of time and sometimes hard-to-find Excel knowledge.
Also: The Microsoft 365 Copilot launch was a total disaster.
Rather than go into more details here, I recommend you watch this video from Singapore, where the instructor provides a detailed look at how Excel works with taxes. While tax policy in each country is different, the tasks the instructor performs are very similar throughout the world.
4. Chat with a chatbot for tax advice and guidance.
You can also use a chatbot like ChatGPT or Perplexity to get tax guidance and advice. Just keep in mind you want to ask questions about topics that have been written about in prior years, are stable with unchanging tax rules, and for which there is .
Also: How to make ChatGPT provide improved insights and citations.
Here are some examples I tried. They all resulted in good and accurate answers (at least as best as this non-accountant could tell).
Who needs to file a US federal tax return?
What are the IRS standard deduction amounts?
What are the tax brackets for past years?
What is the difference between a tax deduction and a tax credit?
What tax credits are available for education expenses?
How can I check the status of my federal tax refund?
Make sure you preface any questions you ask with your filing jurisdiction. If you're in the US, say so. If you're in Singapore, tell the AI that. Otherwise, the AI will probably not know which jurisdiction's tax rules are appropriate for you.
5. Upload and scan documents for analysis, summarization, and explanation.
You can feed your favorite chatbot PDFs for it to analyze and explain. For example, I uploaded a copy of the instructions for IRS Form 2553, which is the form used to elect S corporation status.
Also: US sets AI safety aside in favor of 'AI dominance'
I asked ChatGPT, "Explain this." I then asked it, "What are the most essential things I should know?" It scanned the document and provided me with a list of essential informational nuggets.
I asked ChatGPT to provide me with a list of 10 areas where you should not use AI to help with taxes. It's a good list, and I fully agree with all of its points.
Providing legally binding tax advice: AI does not replace professional tax advisors, CPAs, or attorneys. Ensuring complete tax compliance: AI may not account for the latest IRS rule changes, state-specific laws, or unique tax situations. Filing your tax return on your behalf: AI cannot submit tax forms directly to the IRS or state tax agencies. Determining eligibility for complex tax deductions and credits: Some deductions and credits (like the Qualified Business Income Deduction) require professional assessment. Guaranteeing IRS audit protection: AI cannot ensure you won't be audited or provide direct representation if you are audited. Handling late tax election relief requests: The IRS may require a written explanation of "reasonable cause," which is best handled by a tax professional. Interpreting ambiguous tax laws and regulations: AI cannot provide definitive answers on gray areas of tax law or IRS rulings. Preparing multi-state or international tax returns: AI may not accurately handle tax liabilities across multiple jurisdictions. Detecting tax fraud or avoiding penalties: AI cannot verify whether deductions, credits, or income reporting comply fully with IRS standards. Giving investment or retirement tax strategy recommendations: AI cannot advise on tax-efficient investment decisions, Roth IRA conversions, or estate planning strategies.
What do you think? Have you tried AI-powered tax tools like TurboTax Assist, H&R Block Tax Assist, or QuickBooks? Did they help or make things more complicated? Do you trust AI to handle tax prep, or do you still prefer human expertise? Where do you think AI tax tools need the most improvement? Let us know in the comments below.
India’s global capability centre (GCC) ecosystem is expanding rapidly, set to grow from 1,700 centres in 2024 to 2,100 by 2030. While US-based GCCs co......
A research study commissioned by computing giant IBM revealed on Wednesday that most Indian companies made significant progress in executing their AI ......
Would you rather work from home or go to the office every day? Just a few years ago, employees had more control over this decision. But today, the cho......
Branching Out: 4 Git Workflows for Collaborating on ML
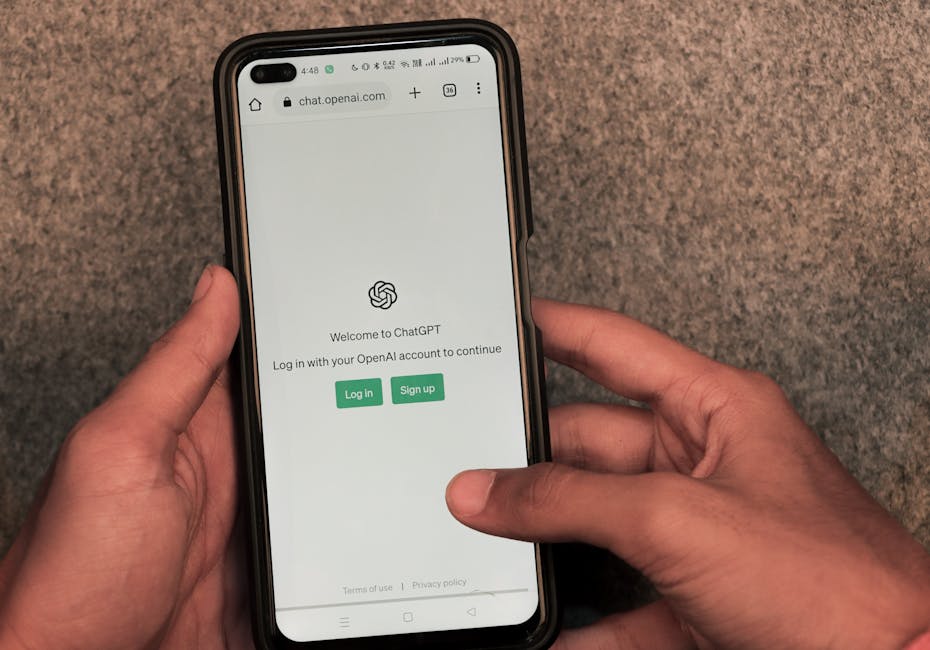
It’s been more than 15 years since I finished my master’s degree, but I’m still haunted by the hair-pulling frustration of managing my of R scripts. As a (recovering) perfectionist, I named each script very systematically by date (think: [website] ). A system I just *knew* was more effective than _v1 , _v2 , _final and its frenemies. Right?
Trouble was, every time I wanted to tweak my model inputs or review a previous model version, I had to swim through a sea of scripts.
Fast forward a few years, a few programming languages, and a career slalom later, I can clearly see how my solo struggles with code versioning were a lucky wake-up call.
While I managed to navigate those early challenges (with a few cringey moments!), I now recognise that most development, especially with Agile ways of working, thrives on robust version control systems. The ability to track changes, revert to previous versions, and ensure reproducibility within a collaborative codebase can’t be an afterthought. It’s actually a necessity.
When we use version control workflows, often in Git, we lay the groundwork for developing and deploying more reliable and higher quality data and AI solutions.
If you already use version control and you’re thinking about different workflows for your team, welcome! You’ve come to the right place.
If you’re new to Git or have only used it on solo projects, I recommend reviewing some introductory Git principles. You’ll want more background before jumping into team workflows. GitHub provides links to several Git and GitHub tutorials here. And this getting started post introduces basics like how to create a repo and add a file.
Development teams work in different ways.
But a ubiquitous feature is reliance on version control.
Git is incredibly flexible as a version control system, and it allows developers a lot of freedom in how they manage their code. If you’re not careful, though, flexibility leaves room for chaos if not managed effectively. Establishing Git workflows can guide your team’s development so you’re using Git more consistently and efficiently. Think of it as the team’s shared roadmap for navigating Git’s highways and byways.
By defining when we create branches, how we merge changes, and why we review code, we create a common understanding and foster more reliable ways of developing as a team. Which means that every team has the opportunity to create their own Git workflows that work for their specific organisational structure, use-cases, tech stack, and requirements. It’s possible to have as many ways of using Git as a team as there are development teams. Ultimate flexibility.
You may find that idea liberating. You and your team have the freedom to design a Git workflow that works for you!
But if that sounds intimidating, not to worry. There are several established protocols to use as a starting point for agreeing on team workflows.
Version control is useful in so many ways, but the benefits I see over and over on my teams cluster into a few essential categories. We’re here to focus on workflows so I won’t go into great depth, but the central premise and advantages of Git and GitHub are worth highlighting.
(Almost) anything is reversible. Which means that version control systems free you up to get creative and make mistakes. Rolling back any regrettable code changes is as simple as git revert . Like a good neighbour, Git commands are there.
Simplifies code Collaboration. Once you get into the flow of using it, Git really facilitates seamless collaboration across the team. Work can happen concurrently without interfering with anyone else’s code, and code changes are all documented in commit snapshots. This means anyone on the team can take a peek at what the others have been working on and how they went about it, because the changes are captured in the Git history. Collaboration made easy.
Isolating exploratory work in feature branches. How will you know which model gives the best performance for your specific business problem? In a recent revenues use case, it could’ve been time series models, maybe tree-based methods, or convolutional neural networks. Possibly even Bayesian approaches. Without the parallel branching ability Git provided my team, trialling the different methods would’ve resulted in a codebase of pure chaos.
In-built review process (massively improves code quality). By putting code through peer review using GitHub’s pull request system, I’ve seen team after team grow in their abilities to leverage their collective knowledge to write cleaner, faster, more modular code. As code review helps team members identify and address bugs, design flaws, and maintainability, it ultimately leads to higher quality code.
Reproducibility. As in, every change made to the codebase is recorded in the Git history. Which makes it incredibly easy to track changes, revert to previous versions, and reproduce past experiments. I can’t understate its importance for debugging, code maintenance, and ensuring the reliability of any experimental findings.
Different flavours of workflows for different types of work.
Feature-branching workflow: The Standard Bearer.
This is the most common Git workflow used in dev teams. It’d be difficult to unseat it in terms of its popularity, and for good reason. In a feature branching workflow, each new functionality or improvement to the code is developed in its own dedicated branch, separate from the main codebase.
A branching workflow provides each developer with an isolated workspace (a branch) — their own complete copy of the project. This lets every person on the team do focused work, independent of what’s happening elsewhere in the project. They can make code changes and forget about upstream development, working independently until they’re ready to share their code.
At that point, they can take advantage of GitHub’s pull request (PR) functionality to facilitate code review and collaborate with the team to ensure the changes are evaluated and approved before being merged into the codebase.
This approach is especially beneficial to Agile development teams and teams working on complex projects that call for frequent code changes.
A feature branching workflow might look like this:
# In your terminal: $ git switch # Creates and switches onto a new branch $ git push -u origin # For first push only. Creates new working branch on the remote repository # Create and activate your virtual environment. Pip install any required packages. $ python3 -m venv $ source new_venv_name/bin/activate $ pip install [website] (or ) # Make changes to your code in feature branch # Regularly stage and commit your code changes, and push to remote. For example: $ git add # Stages the file to prepare repo snapshot for commit $ git commit -m "" # Records file snapshots into your version history $ git push # Sends local commits to the remote repository; to your working branch # Raise Pull Request (PR) on repo's webpage. Request reviewer(s) in PR. # After PR is approved and merged to `main`, delete working branch.
This approach is what I think of as an introductory workflow. What I mean is that the main trunk is the only point where changes enter the repository. A single main branch is used for all development and all changes are committed to this branch, ignoring the existence of branching (we ignore software capabilities all the time, right?).
This isn’t an approach you’ll find being used by high-velocity dev teams or continuous delivery teams. So you might be wondering — is there ever good reason for a centralised Git workflow?
First, centralised Git workflows can streamline the initial explorations of a very small team. When the focus is on rapid prototyping and the risk of conflicts is minimal — as in a project’s early days — a centralised workflow can be convenient.
And second, using a centralised Git workflow can be a good way to migrate a team onto version control because it doesn’t require any branches other than main . Just use with caution as things can quickly go pear shaped. As the codebase grows or as more people contribute there’s an greater risk of code conflicts and accidental overwrites.
Otherwise, centralised Git workflows are generally not recommended for sustained development, especially in a team setting.
A centralised workflow might look like this:
# In your terminal: $ git checkout # Switches onto `main` branch # Create and activate your virtual environment. Pip install any required packages. $ python3 -m venv $ source new_venv_name/bin/activate $ pip install [website] (or ) # Make changes to code # Regularly stage and commit your code changes, and push to remote. For example: $ git add # Stages the file to prepare repo snapshot for commit $ git commit -m "" # Records file snapshots into your version history $ git push # Sends local commits to the remote repository; to whichever branch you're working on. In this case, the `main` branch.
Data scientists and Mlops teams have a somewhat unique use-case compared to traditional software development teams. The development of machine learning and AI projects is inherently experimental. So from a Git workflow perspective, protocols need to flex to accommodate frequent iteration and complex branching strategies. You may also need the ability to track more than code, like experiment results, data, or model artifacts.
Feature branching augmented with experiment branches is probably the most popular approach.
This approach starts with the familiar feature branching workflow. Then within a feature branch, you create sub-branches for specific experiments. Think: “experiment_hyperparam_tuning”, or “experiment_xgboost”. This workflow affords enough granularity and flexibility to track individual experiments. And as with standard feature branches, this isolates development allowing experimental approaches to be explored without affecting the main codebase or other developers’ work.
But caveat emptor: I noted it was popular, but that doesn’t mean the branching experiments workflow is simple to manage. It can all turn to a tangled mess of spaghetti-branches if things are allowed to grow overly complex. This workflow involves frequent branching and merging, which can feel like unnecessary overhead in the face of rapid experimentation.
A branching experiments workflow might look like this:
# In your terminal: $ git checkout # Move onto a feature branch ready for ML experiments $ git switch # Creates and switches onto a new branch for experiments # Create and activate your virtual environment. Pip install any required packages. # Make changes to your code in feature branch. # Continue as in Feature Branching workflow.
Integrating tools like MLflow into a feature branching workflow or branching experiments workflow offers additional possibilities. Reproducibility is a key concern for ML projects, which is why tools like MLflow exist. To help manage the full machine learning lifecycle.
For our workflows, MLflow enhances our capabilities by enabling experiment tracking, logging model runs in the registry, and comparing the performance of various model specifications.
For a branching experiments workflow, the MLflow integration might look like this:
# In your terminal: $ git checkout # Move onto a feature branch ready for ML experiments $ git switch # Creates and switches onto a new branch for experiments # Create and activate your virtual environment. Pip install any required packages. # Initialise MLflow within your Python script. # Make changes to branch. As you experiment with different hyperparameters or model architectures, create new experiment branches and log the results with MLflow. # Regularly stage and commit your code changes and MLflow experiment logs. For example: $ git add # Stages the file to prepare repo snapshot for commit $ git commit -m "" # Records file snapshots into your version history $ git push # Sends local commits to the remote repository; to your working branch # Use the MLflow UI or API to compare the performance of different experiments within your feature branch. You may want to select the best-performing model based on the logged metrics. # Merge experimental branch(es) into the parent feature branch. For example: $ git checkout # Switch back onto the parent feature branch $ git merge # Merge experiment branch into the parent feature branch # Raise Pull Request (PR) to merge it into `main` once the feature branch work is completed. Request reviewers. Delete merged branches. # Deploy if applicable. If the model is ready for deployment, use the logged model artifact from MLflow to deploy it to a production environment.
The Git workflows I’ve shared above should provide a good starting point for your team to streamline collaborative development and help them to build high-quality data and AI solutions. They’re not rigid templates, but rather adaptable frameworks. Try experimenting with different workflows. Then adjust them to craft the an approach that’s most effective for your needs.
Git Workflows Simplify: The alternative is too frightening, too messy, too slow to be sustainable. It’s holding you back.
The alternative is too frightening, too messy, too slow to be sustainable. It’s holding you back. Your Team Matters: The ideal workflow will vary depending on your team’s size, structure, and project complexity.
The ideal workflow will vary depending on your team’s size, structure, and project complexity. Project Requirements: The specific needs of the project, such as the frequency of releases and the level of ML experimentation, will also influence your choice of workflow.
Ultimately, the best Git workflow for any data or MLOps dev team is the one that suits the specific requirements and development process of that team.
There seems to be a consensus that leveraging data, analytics, and AI to create a data-driven organization requires a clear strategic approach. Howeve......
Accenture and Google Cloud are collaborating to accelerate the adoption of cloud solutions and generative AI in Saudi Arabia. The goal is to help busi......
Ansys says simulations will close the gap with reality and make the world more sustainable
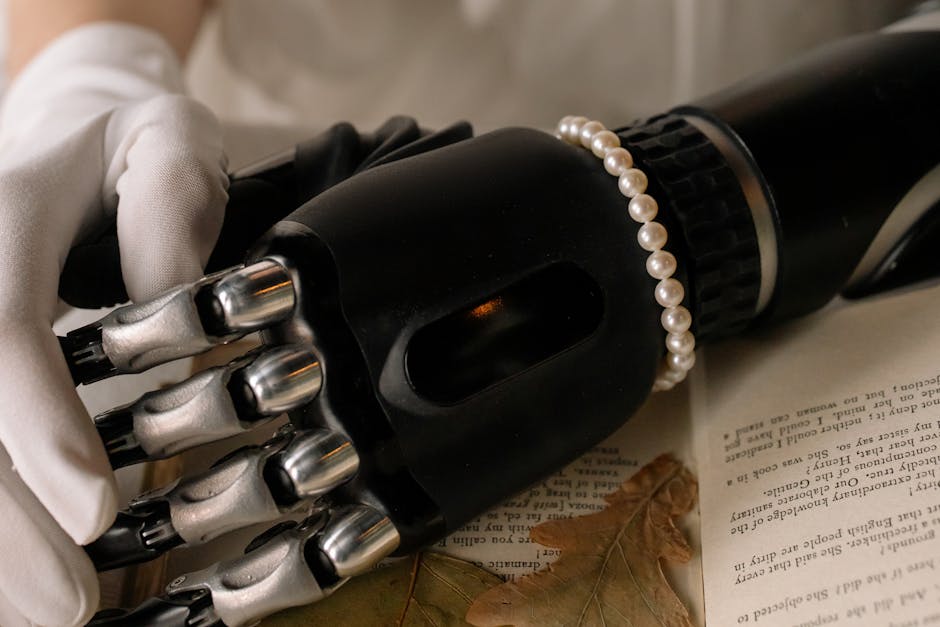
You may not have heard of Ansys, but it’s in the process of being acquired by chip design tool firm Synopsys for $35 billion.
That’s happening because Ansys, an engineering software firm, specializes in the simulation of the world’s complex electronic systems, and the world of chip design is increasingly moving into the more complex world of system design, expressed Prith Banerjee, CTO of Ansys, in an interview with GamesBeat.
Ansys spans a lot of businesses. It works in the automotive space with carmakers (original equipment manufacturers, or OEMs). It works with the tier one suppliers in the car industry, and it works with chip companies that are making chips for the cars and more. Ansys makes tools for engineering simulations and more, expressed Banerjee. And he noted that companies all over the world are embracing AI and machine learning. He can see it in his clients’ simulations.
“With AI and ML, we are able to use simulation much easier as well as much faster. Something that takes a hundred hours to run can run in a matter of minutes, so we have got some techniques to aid us in that area,” Banerjee stated. “AI was big in general at CES. I mean Jensen (Huang, CEO of Nvidia) talked about AI and all the GPUs and so on, but we are embracing AI like never before.”.
Ansys simulation tools help companies design race cars.
Ansys is working with a lot of companies. At CES 2025, it unveiled a collaboration with Sony Semiconductor Solutions to improve perception system validation in smart cars. Ansys’ solutions are used by more than 200 automotive and tech companies that show off stuff in Las Vegas each January. Every year, Ansys tries to close the gap between engineering design and reality using the power of simulation.
It creates virtual wind tunnel technology to optimize F1 racing car designs with Oracle Red Bull Racing, Porsche and Ferrari.
Increasingly, this simulation superpower also speeds time-to-market, lowers manufacturing costs, improves quality, and decreases risk.
LightSolver, another Ansys partner being showcased today, says that the fourth industrial revolution, also known as Industry [website], is fully underway. Almost every industry — from automotive and aerospace to consumer goods and healthcare — is demonstrating a shift toward digitalization.
The industrial equipment and manufacturing industries are no exception. A global industrial robotics survey revealed that industrial companies are expected to invest 25% of their capital spending on automation from 2022 to 2027. The survey also found that automation is already being implemented or piloted for many popular industrial tasks, including palletization and packaging, material handling, goods receiving, unloading, and storage.
BMW is building a digital twin of a factory that will open for real in 2025.
Banerjee is excited about the tools like Nvidia’s Omniverse, which is enabling the creation of virtual designs known as digital twins. With such twins, companies like BMW are designing car factories in a virtual space of the Omniverse first. When the design is perfect, they build the factory in the real world. They outfit the factory with sensors that collect data and feed it back to the digital design. That makes the virtual design improved and creates a feedback cycle of continuous improvement. That means that the simulations of everything from Microsoft Flight Simulator to the car factories are getting closer to real life.
“Digital twins as a topic is very big for us. Of all the conversations that I had with all end-clients, we talked most about our concept of hybrid digital twins the most,” Banerjee mentioned. “The rest of the industry is doing digital twins by putting sensors on the actual assets, right? You’re making a digital model of the asset by just putting in sensors. And we’re using data analytics. What we do in terms of digital twins is physics-based, simulation-based digital twins.”.
Banerjee added, “We combine it with data analytics to do what is called hybrid digital twins. Sustainability is big for us. So we are driving a lot of things around how to make the world more sustainable, lower carbon emissions using simulations.”.
Asked about whether Ansys would like to see more of Nvidia’s digital twin technology as open source, Banerjee noted he would like to see open standards in the ecosystem.
“The faster this whole thing comes, the bigger the opportunities for everyone,” he revealed. “It doesn’t help anyone to have four different standards.” No one wants to be tied to a single GPU or a single software stack.
Nvidia is bringing OpenUSD to metaverse-like industrial applications.
Banerjee mentioned the metaverse is real, as many companies are taking it seriously beyond Meta. He noted those include Amazon Web Services, Microsoft, Google and Nvidia.
“They all have some form of the metaverse. So we believe that the metaverse is real, that that is going to happen. And we, as the leading simulation corporation, need to integrate with the metaverse,” Banerjee introduced. “And what is it? The metaverse allows you to combine the physical world with the virtual world, which is the concept of digital. For example, what we bring to the Omniverse from Nvidia is that they have got a solution, a stack.
He added, “The are using their simulators like Isaac, simulating robots and so on, right? But their simulations are kind of at a high level, an approximate simulation. They say it’s a physics-based simulation, but it’s not the level of accuracy that we bring to the table.”.
He expressed that Ansys is focused on physics-based simulations, and the enterprise’s work revolves around core physics solvers. These cover mechanical structures, fluids and electromagnetic areas.
“These are the four core solvers. We are in discussions with Nvidia and we have an active partnership going on to take each of the solvers to visualize the output so the engineer will see the output on the desktop as it is happening,” Banerjee stated.
He mentioned the world is moving to the cloud the world and AI. In that new world of AI plus cloud plus GPUs, the metaverse is the right way to do the user interface and interact with the results of simulation.
“We are working hand-in-hand with Nvidia to make sure our four core solvers are integrated with the Omniverse. So that’s one very core area of collaboration,” he introduced.
Asked what he means by hybrid digital twins, Banerjee stated he used to be the CTO at a couple of other large industrial companies. He was CTO at ABB, a power and automation business in Switzerland. And he was also CTO at Schneider Electric, a power and automation business based in France.
In those roles, he saw that large industrial companies have lots of large assets. The assets can be transformers, robots or switch gears. And these assets are there for a long time.
“What you try to do is to see to see when that asset fails. Say a million dollar transformer fails, and when it does, you lose power and that’s bad for the environment and clients. So what you try to do is put sensors on these assets to see if my transformer working or not,” he showcased. “And so before the transformer fails, it starts giving signals. So just like the human body, we have the normal things like our temperature. But before we fall sick, the temperature goes to 99. 100, 101 and then you get the fever and then it’s really pulsing. So before you really fall sick, you start giving signals. The same analogy works for digital twins.”.
A virtual wind tunnel used to help design a race car.
He added, “So you put sensors, collect data and before that asset fails, it starts giving different signals. So if you monitor the changes, you can predict that it’s going to fail. This is how when I was at ABB and Schneider Electric and again all the other companies like Caterpillar or GE and everybody, all these companies, they use digital twins using data analytics. So if they pull the data and they look at here’s the normal behavior and notice the abnormal behavior. And then based on the abnormal behavior, you see it’s going to fail.”.
He continued, “Now, what I found out when I was at ABB and Schneider is the accuracy of that prediction is based on pure data analytics at about 70%. And you say, oh, 70% is pretty good. Well, if you have a million-dollar part and you are 70% accurate, that means you made a 30% error. So you made an error to replace a part with a 30% probability. You just made a $300,000 mistake. You made a decision which was wrong because your accuracy was only 70%. So this was the problem I was facing when I was at Ansys.”.
Banerjee stated he always knew that if you could tie that to physics-based simulation, the accuracy would go up.
“I joined Ansys about six, seven years ago and I told my CEO, I showcased, this is the problem that we need to solve. If you could solve it through physics-based simulation, that would be absolutely amazing. So physics-based simulation says, “Here is a transformer, here is a robot, here is whatever, right? And you go back to the basic physics. This is how the transformer works, right? It is electrical, it signals going through the coils and is generating this and if there is a cut in the coil, right, those electrical signals, the mechanical signals will not come. That’s why the failure happens. Let’s go back to the first principles of the physics. So at Ansys, we did physics-based digital twins and simulation. The accuracy went from 70% to 90%.”.
He stated, “You say, ‘Wow, 90% is great.’ But with that million-dollar part and 90% accuracy, you’re still making a $100,000 mistake. So then we stated, what if you could combine the two? Combine the data analytics-based digital twins with the physics, and that is what we did called fusion technology, or a hybrid digital twin, which is now called a product called Twin AI.”.
“The accuracy of that combination is 99%. So on that million-dollar part, I will only make a $1,000 mistake. So our clients are super excited,” he mentioned.
“At CES, I talked to many clients about our Twin AI technology, digital twin technology that works at the system level. We could build a digital twin of an entire car or a subsystem. You can take an EV car, break it down into different components of power electronics — the battery the drive train or inside the battery. We can keep going down and down now and build digital twins of the system, the subsystem, the components. But at every level, if there are sensors, we can actually build this fusion-based digital twin, this sort of hybrid digital twin. That is an absolutely amazing technology, and this is something I’ve been proud of.”.
The intersection of simulation, game worlds and the real world.
Microsoft Flight Simulator 2024 simulates the African savannah because it can.
I noted how there’s an intersection of simulated worlds and game worlds and the real world with products like the game, Microsoft Flight Simulator 2024. The 2024 game had 4,000 times more detail on the ground than the 2020 version. It enabled them to do amazing simulations like using a helicopter to herd a flock of sheep on the ground.
They added gliders to the game and that meant you could land anywhere, so they needed well-simulated places where you could land just about anywhere on the planet. They enlisted aircraft manufacturers to give them CAD models of the designs for aircraft in the game, and they pulled camera video footage from the planes after they flew over parts of the planet. My question was whether we would ever get to one-to-one accuracy between simulation and reality.
“So that’s a great question. So let me take a step back and give you the approach to simulation that we use. In our world of computer-aided engineering simulation, CAE simulation, we take the world around us which is governed by the laws of physics. Physics doesn’t lie, right? When in the world of fluids, there’s an equation called Navier Stokes equation. These are second order partial differential equations. That is the equation that is the way nature works. So we take those equations, and we solve them numerically.”.
He added, “Now when you solve it numerically you can take a particular type. You can break it up into four quadrants or more. Four or 16 or 32. The more elements I have, the more accuracy I get.”.
And he noted, “The trouble is, as you add more elements, more accuracy, your runtime goes out the window. Because runtime is sort of N cubed, right? So the number of elements, it’s N cubed. So this has been the challenge in our industry. With CAE simulation, you can absolutely get more accuracy, but your runtime increases. So how do you get more accuracy faster?”.
As CTO at Ansys, Banerjee has multiple technology pillars. There are advanced numerical methods where you’re looking at just the algorithm itself on a single processor, making it go faster, more accurate, and so on. The second thing is using HPC, high-performance computing.
“You have a thousand hours of work to do. I have a hundred processors, and I’ll give them to you to run much faster just by adding parallelism to it,” he expressed. “That’s why GPUs come in the partnership with Nvidia, helping us to take a fixed amount of work using GPUs to make it run much faster right with the same accuracy.”.
The third focus is AI, where the organization is training those its four core simulators. Once trained, the AI model runs 100 times faster.
“In the world of digital twins, we have actually taken all those technologies, GPU, HPC technology and AI technology, and we call that reduced order models, ROMs, and it’s because of Ansys’ leading position in the area of reduced order models and AI cost simulation.
Simulations can reduce the risks of maintenance failures.
The simulation market is around $10 billion today and it’s growing around 12% a year.
If you look at the entire R&D budget across all industries in the world, it’s about $[website] trillion dollars, Banerjee said. In the automotive industry, the R&D is about $250 billion. About 75% of that cost is banging up cars in “physical validation” of the vehicles. It’s physical prototyping, he said.
“What we believe is that the simulation becomes so accurate and so fast that companies will stop doing physical programming,” Banerjee stated. “In fact, the CEO of GM has stated that by 2035, GM will stop doing physical programming if everything will be virtual. So simulation is growing at 12% today. But once those use cases come in, there will be a hockey stick event.”.
The complexity of chip design and the coming of systems design.
Banerjee noted that one reason that Synopsys is acquiring Ansys for $35 billion in cash and stock is that the world is moving from chips to systems when it comes to design.
“You have electronic chips that were designed with tools from Cadence and Synopsys and Mentor Graphics, but they’re only building the chip inside a system. So it’s going inside a car, right? But now you are going from chips to systems and the opportunity for simulation to design these really complicated chips to systems is enormous. It’s powered by GPUs, powered by the Omniverse, powered by AI. I am very excited about the future of simulation and synthesis for the vision of chips to systems across industries like automotive, aerospace, energy, high tech and healthcare. These are the five verticals that I we look at in terms of the opportunity to move from chips to systems.”.
Banerjee has worked in the electronic design automation (EDA, or using software to automate chip design) for more than 20 years. He spent his first 20 years in academia, building EDA tools. Forty years ago, he had to teach VLSI design by drawing rectangles on the screen, which is now called Custom IC. Then the whole design industry moved. Chips had perhaps 10,000 transistors, which was pretty hard for engineers to process. Then each progressive improvement, from standard cells to Synopsys’ synthesis, the level of complexity of the designs improved. Now chip designers can create 200-billion transistor chips.
“My projection is that we could do a similar thing with synthesis tools for systems. Can you have a synthesis tool for a system as complicated as an automobile or an airplane? Today, it is done. You look at a specification and a human designer goes and does the CAD of the airplane engine,” he expressed.
He added, “I am saying at some point in the future you will not have to do the CAD. It will be synthesized, right? Just like we use synthesis tools for chip design, there will be system level synthesis tools. Now this is like I’m talking five to ten years out. We have got things going on at Ansys, but that’s the opportunity. Once that happens, the design of the systems will be accelerated by many factors.” So you could do a thing like a 200 billion transistor chip, right? It’s something much more complicated than what you can do. And as the automotive companies are struggling to reduce that design time from four years to two years to whatever, could you imagine a new car design coming out in a matter of a month? Yeah. that can be enabled by synthesis.”.
Credit: VentureBeat made with Midjourney V6.
I asked Banerjee what was the most complicated design possible. Is it the human brain? The human heart?
“I’m glad you mentioned the human heart. I will tell you, at Ansys, I am really passionate about the healthcare area and we in the CTO office are working on simulating the human body, the heart, the brain, the lungs and so on,” her revealed. “That is just such a complicated thing that we live and breathe every day. Simulation of a human body accurately will enable us to come up with solutions to heart disease. When you have arrhythmia, you have irregular heartbeat. That can be treated through either you take a drug, AstraZeneca, and it will treat your [condition]. Or you take a pacemaker from Medtronic and that will take it. Or you do more jogging, right? Changing your behavior.”.
He expressed, “Each of these things. or you can do actually an operation, right? You go in and you insert a stent. We are imagining a future where each of these things can be simulated. Here is this cardiovascular drug. If I take that medication, will it interact with the molecules in the human body? If I put a stent in, what is going to happen? So imagine in the future you will not require what is called clinical trials. Everything will be done through simulation of a human body, right? Virtual humans. And that will accelerate the use of the discovery of drugs and discovery of medical devices.”.
L’intelligence artificielle a facilité la création de visuels plus réalistes que jamais. Cependant, ce progrès s’accompagne d’un défi majeur : comment......
ByteDance, the parent business of TikTok, has dropped a family of joint image-video generation models called Goku. The models seem to be named after th......
Market Impact Analysis
Market Growth Trend
2018 | 2019 | 2020 | 2021 | 2022 | 2023 | 2024 |
---|---|---|---|---|---|---|
23.1% | 27.8% | 29.2% | 32.4% | 34.2% | 35.2% | 35.6% |
Quarterly Growth Rate
Q1 2024 | Q2 2024 | Q3 2024 | Q4 2024 |
---|---|---|---|
32.5% | 34.8% | 36.2% | 35.6% |
Market Segments and Growth Drivers
Segment | Market Share | Growth Rate |
---|---|---|
Machine Learning | 29% | 38.4% |
Computer Vision | 18% | 35.7% |
Natural Language Processing | 24% | 41.5% |
Robotics | 15% | 22.3% |
Other AI Technologies | 14% | 31.8% |
Technology Maturity Curve
Different technologies within the ecosystem are at varying stages of maturity:
Competitive Landscape Analysis
Company | Market Share |
---|---|
Google AI | 18.3% |
Microsoft AI | 15.7% |
IBM Watson | 11.2% |
Amazon AI | 9.8% |
OpenAI | 8.4% |
Future Outlook and Predictions
The Ways Help Your landscape is evolving rapidly, driven by technological advancements, changing threat vectors, and shifting business requirements. Based on current trends and expert analyses, we can anticipate several significant developments across different time horizons:
Year-by-Year Technology Evolution
Based on current trajectory and expert analyses, we can project the following development timeline:
Technology Maturity Curve
Different technologies within the ecosystem are at varying stages of maturity, influencing adoption timelines and investment priorities:
Innovation Trigger
- Generative AI for specialized domains
- Blockchain for supply chain verification
Peak of Inflated Expectations
- Digital twins for business processes
- Quantum-resistant cryptography
Trough of Disillusionment
- Consumer AR/VR applications
- General-purpose blockchain
Slope of Enlightenment
- AI-driven analytics
- Edge computing
Plateau of Productivity
- Cloud infrastructure
- Mobile applications
Technology Evolution Timeline
- Improved generative models
- specialized AI applications
- AI-human collaboration systems
- multimodal AI platforms
- General AI capabilities
- AI-driven scientific breakthroughs
Expert Perspectives
Leading experts in the ai tech sector provide diverse perspectives on how the landscape will evolve over the coming years:
"The next frontier is AI systems that can reason across modalities and domains with minimal human guidance."
— AI Researcher
"Organizations that develop effective AI governance frameworks will gain competitive advantage."
— Industry Analyst
"The AI talent gap remains a critical barrier to implementation for most enterprises."
— Chief AI Officer
Areas of Expert Consensus
- Acceleration of Innovation: The pace of technological evolution will continue to increase
- Practical Integration: Focus will shift from proof-of-concept to operational deployment
- Human-Technology Partnership: Most effective implementations will optimize human-machine collaboration
- Regulatory Influence: Regulatory frameworks will increasingly shape technology development
Short-Term Outlook (1-2 Years)
In the immediate future, organizations will focus on implementing and optimizing currently available technologies to address pressing ai tech challenges:
- Improved generative models
- specialized AI applications
- enhanced AI ethics frameworks
These developments will be characterized by incremental improvements to existing frameworks rather than revolutionary changes, with emphasis on practical deployment and measurable outcomes.
Mid-Term Outlook (3-5 Years)
As technologies mature and organizations adapt, more substantial transformations will emerge in how security is approached and implemented:
- AI-human collaboration systems
- multimodal AI platforms
- democratized AI development
This period will see significant changes in security architecture and operational models, with increasing automation and integration between previously siloed security functions. Organizations will shift from reactive to proactive security postures.
Long-Term Outlook (5+ Years)
Looking further ahead, more fundamental shifts will reshape how cybersecurity is conceptualized and implemented across digital ecosystems:
- General AI capabilities
- AI-driven scientific breakthroughs
- new computing paradigms
These long-term developments will likely require significant technical breakthroughs, new regulatory frameworks, and evolution in how organizations approach security as a fundamental business function rather than a technical discipline.
Key Risk Factors and Uncertainties
Several critical factors could significantly impact the trajectory of ai tech evolution:
Organizations should monitor these factors closely and develop contingency strategies to mitigate potential negative impacts on technology implementation timelines.
Alternative Future Scenarios
The evolution of technology can follow different paths depending on various factors including regulatory developments, investment trends, technological breakthroughs, and market adoption. We analyze three potential scenarios:
Optimistic Scenario
Responsible AI driving innovation while minimizing societal disruption
Key Drivers: Supportive regulatory environment, significant research breakthroughs, strong market incentives, and rapid user adoption.
Probability: 25-30%
Base Case Scenario
Incremental adoption with mixed societal impacts and ongoing ethical challenges
Key Drivers: Balanced regulatory approach, steady technological progress, and selective implementation based on clear ROI.
Probability: 50-60%
Conservative Scenario
Technical and ethical barriers creating significant implementation challenges
Key Drivers: Restrictive regulations, technical limitations, implementation challenges, and risk-averse organizational cultures.
Probability: 15-20%
Scenario Comparison Matrix
Factor | Optimistic | Base Case | Conservative |
---|---|---|---|
Implementation Timeline | Accelerated | Steady | Delayed |
Market Adoption | Widespread | Selective | Limited |
Technology Evolution | Rapid | Progressive | Incremental |
Regulatory Environment | Supportive | Balanced | Restrictive |
Business Impact | Transformative | Significant | Modest |
Transformational Impact
Redefinition of knowledge work, automation of creative processes. This evolution will necessitate significant changes in organizational structures, talent development, and strategic planning processes.
The convergence of multiple technological trends—including artificial intelligence, quantum computing, and ubiquitous connectivity—will create both unprecedented security challenges and innovative defensive capabilities.
Implementation Challenges
Ethical concerns, computing resource limitations, talent shortages. Organizations will need to develop comprehensive change management strategies to successfully navigate these transitions.
Regulatory uncertainty, particularly around emerging technologies like AI in security applications, will require flexible security architectures that can adapt to evolving compliance requirements.
Key Innovations to Watch
Multimodal learning, resource-efficient AI, transparent decision systems. Organizations should monitor these developments closely to maintain competitive advantages and effective security postures.
Strategic investments in research partnerships, technology pilots, and talent development will position forward-thinking organizations to leverage these innovations early in their development cycle.
Technical Glossary
Key technical terms and definitions to help understand the technologies discussed in this article.
Understanding the following technical concepts is essential for grasping the full implications of the security threats and defensive measures discussed in this article. These definitions provide context for both technical and non-technical readers.