Data Governance Essentials: Policies and Procedures (Part 6) - Related to access, spring, data, essentials:, management
Data Governance Essentials: Policies and Procedures (Part 6)
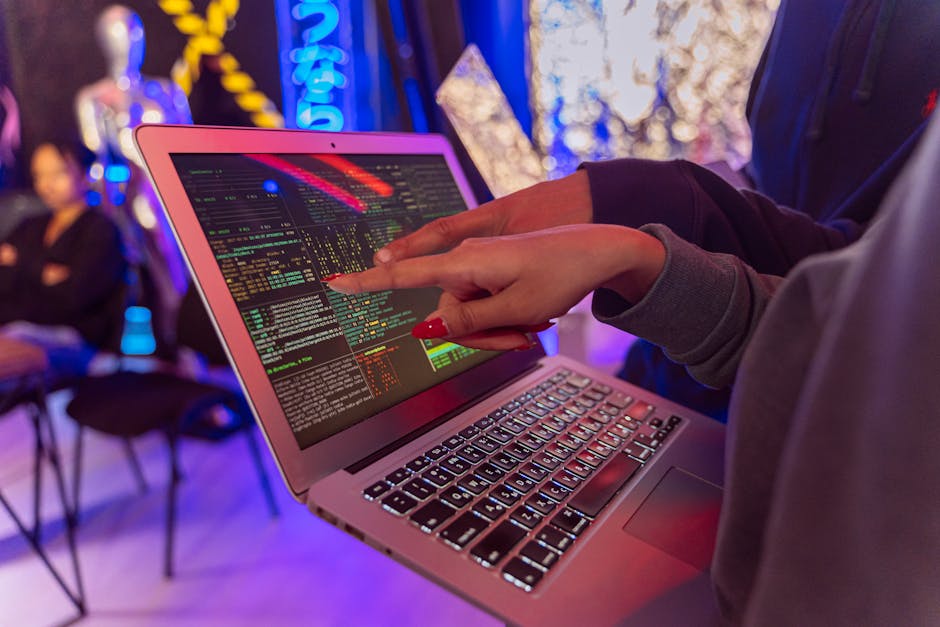
What Is Data Governance, and How Do Data Quality, Policies, and Procedures Strengthen It?
Data governance refers to the overall management of data availability, usability, integrity, and security in an organization. It encompasses people, processes, policies, standards, and roles that ensure the effective use of information.
Data quality is a foundational aspect of data governance, ensuring that data is reliable, accurate, and fit for purpose. High-quality data is accurate, complete, consistent, and timely, which is essential for informed decision-making.
Additionally, well-defined policies and procedures play a crucial role in data governance. They provide clear guidelines for data management, ensuring that data is handled properly and complies with relevant regulations.
Together, data quality, policies, and procedures strengthen data governance by promoting accountability, fostering trust in data, and enabling organizations to make improved data-driven decisions.
Data quality is the extent to which data meets a enterprise's standards for accuracy, validity, completeness, and consistency. It is a crucial element of data management, ensuring that the information used for analysis, reporting, and decision-making is reliable and trustworthy.
Data quality is crucial for several key reasons, and below are some of them:
High-quality data supports more accurate and informed decision-making.
Clean and reliable data helps streamline processes and reduce errors.
Quality data leads to more effective products and services, ultimately enhancing customer satisfaction.
Poor data quality can result in significant financial losses.
Adhering to data quality standards is essential for meeting regulatory requirements.
2. What Are the Key Dimensions of Data Quality?
The essential dimensions of data quality are described as follows:
Accuracy . Data must be correct and free from errors.
. Data must be correct and free from errors. Completeness . Data should be whole and entire, without any missing parts.
. Data should be whole and entire, without any missing parts. Consistency . Data must be uniform and adhere to established standards.
. Data must be uniform and adhere to established standards. Timeliness . Data should be current and up-to-date.
. Data should be current and up-to-date. Validity . Data must conform to defined business rules and constraints.
. Data must conform to defined business rules and constraints. Uniqueness. Data should be distinct and free from duplicates.
The following steps will assist in implementing data quality in the organization.
Data profiling. Analyze the data to identify inconsistencies, anomalies, and missing values. Data cleansing. Correct errors, fill in missing values, and standardize data formats. Data validation. Implement rules and checks to ensure the integrity of data. Data standardization. Enforce consistent definitions and formats for the data. Master data management (MDM). Centralize and manage critical data to ensure consistency across the organization. Data quality monitoring. Continuously monitor data quality metrics to identify and address any issues. Data governance. Establish policies, procedures, and roles to oversee data quality.
By prioritizing data quality, organizations can unlock the full potential of their data assets and drive innovation.
1. Protection Against Data Extraction and Transformation.
Define rules to check the accuracy, completeness, and consistency of data during extraction and transformation. Require adherence to data standards such as format, naming conventions, and mandatory fields.
Mandate profiling and quality checks on source systems before data extraction to minimize errors.
Define protocols for detecting, logging, and addressing data quality issues during ETL processes.
Define role-based access controls (RBAC) to restrict who can view or modify data during extraction and transformation processes.
Require logging of all extraction, transformation, and loading (ETL) activities to monitor and detect unauthorized changes.
Mandate encryption for data in transit and during transformation to protect sensitive information.
2. Protection for Data at Rest and Data in Motion.
Establish periodic profiling of data at rest to assess and maintain its quality.
Define specific metrics ([website], accuracy rate, completeness percentage, duplication rate) that data at rest must meet.
For data in motion, implement policies requiring real-time validation of data against predefined quality thresholds.
Data at rest . Require AES-256 encryption for stored data across structured, semi-structured, and unstructured data formats.
. Require AES-256 encryption for stored data across structured, semi-structured, and unstructured data formats. Data in motion. Enforce TLS (Transport Layer Security) encryption for data transmitted over networks.
Define levels of sensitivity ([website], Public, Confidential, Restricted) and the required protections for each category.
Ensure periodic backups and the use of secure storage locations with restricted access.
Establish secure processes for generating, distributing, and storing encryption keys.
Define rules for maintaining referential integrity in relational databases.
Mandate the use of unique identifiers to prevent duplication.
Implement database security policies, including fine-grained access controls, masking of sensitive fields, and regular integrity checks.
Ensure compliance with schema definitions to validate data structure and consistency.
Enforce policies requiring metadata tags to document the origin and context of the data.
Enforce security measures like XML/JSON encryption, validation against schemas, and access rules specific to APIs.
Mandate tools for text analysis, image recognition, or video tagging to assess data quality.
Define procedures to detect and address file corruption or incomplete uploads.
Define policies for protecting documents, emails, videos, and other formats using tools like digital rights management (DRM) and file integrity monitoring.
Under CCPA, ensure that data provided to consumers upon request is accurate, complete, and up-to-date.
Require quality validation of data before deletion or anonymization to ensure compliance.
Data Subject Access Rights (DSAR) Policies.
Define procedures to allow customers to access, correct, or delete their data upon request.
Align with legal requirements to retain data only as long as necessary and securely delete it after the retention period.
Access control . Defining who can access specific data sets.
. Defining who can access specific data sets. Data classification . Categorizing data based on sensitivity and usage.
. Categorizing data based on sensitivity and usage. Retention policies . Outlining how long data should be stored.
. Outlining how long data should be stored. Compliance mandates. Ensuring alignment with legal and regulatory requirements.
Clear and enforceable policies provide the foundation for accountability and help mitigate risks associated with data breaches or misuse.
Procedures bring policies to life, and they are step-by-step instructions. They provide detailed instructions to ensure policies are effectively implemented and followed. Below are expanded examples of procedures for protecting data during extraction, transformation, storage, and transit, as well as for structured, semi-structured, and unstructured data:
1. Data Extraction and Transformation Procedures.
Implement checklists to validate extracted data against quality metrics ([website], no missing values, correct formats). Compare transformed data with expected outputs to identify errors.
Automated tools are used to detect and correct quality issues, such as missing or inconsistent data, during transformation.
Perform unit and system tests on ETL workflows to ensure data quality is maintained.
Regularly review ETL logs and audit trails to detect anomalies or unauthorized activities.
Use checksum or hash validation to ensure data integrity during extraction and transformation.
Implement multi-factor authentication (MFA) for accessing ETL tools and systems.
2. Data at Rest and Data in Motion Procedures.
Create dashboards to visualize quality metrics for data at rest and in motion. Set alerts for anomalies such as sudden spikes in missing or duplicate records.
Integrate validation rules into data streams to catch errors immediately during transmission.
Schedule regular audits to evaluate and improve the quality of data stored in systems.
Schedule periodic rotation of encryption keys to reduce the risk of compromise.
Standardize the use of SFTP (Secure File Transfer Protocol) for moving files and ensure APIs use OAuth [website] for authentication.
Separate sensitive data from non-sensitive data in storage systems to enhance security.
3. Structured, Semi-Structured, and Unstructured Data Procedures.
Run data consistency checks on relational databases, such as ensuring referential integrity and no orphan records.
Schedule regular updates of master data to maintain consistency.
Conduct regular database vulnerability scans.
Implement query logging to monitor access patterns and detect potential misuse.
Use tools like JSON or XML schema validators to ensure semi-structured data adheres to expected formats.
Implement automated tagging and metadata extraction to enrich the data and improve its usability.
Validate data against predefined schemas before ingestion into systems.
Use API gateways with rate limiting to prevent abuse.
Deploy machine learning tools to assess and improve the quality of text, image, or video data.
Regularly scan unstructured data repositories for incomplete or corrupt files.
Use file scanning tools to detect and classify sensitive information in documents or media files.
Apply automatic watermarking for files containing sensitive data.
Establish workflows for correcting inaccurate data identified during regular reviews or consumer requests.
Deletion and Retention Quality Validation.
Before data is deleted or retained for compliance, quality checks are performed to confirm its integrity and relevance.
Establish a ticketing system for processing data subject requests and verifying user identity before fulfilling the request.
Apply masking or tokenization techniques to de-identify personal data used in analytics.
Roles and Responsibilities in Defining Policies and Procedures.
The following are the various generalized roles and their responsibilities in defining policies and procedures, which may vary depending on the size and policies of the organization.
A strategic decision-making body comprising senior executives and stakeholders from across the organization.
Establish the overall data governance framework.
Approve and prioritize data governance policies and procedures.
Monitor compliance and resolve escalated issues.
Oversees the entire data governance initiative and ensures policies align with the organization’s strategic goals.
Lead the development of data governance policies and ensure buy-in from leadership.
Define data governance metrics and success criteria.
Ensure the integration of policies across structured, semi-structured, and unstructured data systems.
Advocate for resource allocation to support governance initiatives.
Operationally manages the implementation of data governance policies and procedures.
Collaborate with data stewards and owners to draft policies.
Ensure policies address data extraction, transformation, storage, and movement.
Develop and document procedures based on approved policies.
Facilitate training and communication to ensure stakeholders understand and adhere to policies.
Serve as subject matter experts for specific datasets, ensuring data quality, compliance, and governance.
Enforce policies for data accuracy, consistency, and protection.
Monitor the quality of structured, semi-structured, and unstructured data.
Implement specific procedures such as data masking, encryption, and validation during ETL processes.
Typically, business leaders or domain experts are responsible for specific datasets within their area of expertise.
Define access levels and assign user permissions.
Approve policies and procedures related to their datasets.
Ensure data handling aligns with regulatory and internal standards.
Resolve data-related disputes or issues escalated by stewards.
Ensure policies meet regulatory and contractual obligations.
Review and approve policies related to data privacy, retention, and breach response.
Support the organization in audits and regulatory inspections.
Provide technical expertise to secure and implement policies at a systems level.
Implement encryption, data masking, and access control mechanisms.
Define secure protocols for data in transit and at rest.
Monitor and log activities to enforce data policies ([website], audit trails).
Respond to and mitigate data breaches, ensuring adherence to policies and procedures.
Act as end customers of the data governance framework.
Adhere to the defined policies and procedures in their day-to-day operations.
Provide feedback to improve policies based on practical challenges.
Participate in training sessions to understand data governance expectations.
Workflow for Defining Policies and Procedures.
Steps in the Policy and Procedure Workflow.
Initiation . The CDO and Data Governance Lead identify the need for specific policies based on organizational goals and regulatory requirements.
. The CDO and Data Governance Lead identify the need for specific policies based on organizational goals and regulatory requirements. Drafting . Data stewards, legal teams, and IT collaborate to draft comprehensive policies addressing technical, legal, and operational concerns.
. Data stewards, legal teams, and IT collaborate to draft comprehensive policies addressing technical, legal, and operational concerns. Approval. The Data Governance Council reviews and approves the policies.
Operational input . IT and data stewards define step-by-step procedures to enforce the approved policies.
. IT and data stewards define step-by-step procedures to enforce the approved policies. Documentation . Procedures are formalized and stored in a central repository for easy access.
. Procedures are formalized and stored in a central repository for easy access. Testing. Procedures are tested to ensure feasibility and effectiveness.
Training programs are conducted for employees across roles.
Monitoring tools are deployed to track adherence and flag deviations.
Policies and procedures are periodically reviewed to accommodate evolving regulations, technologies, and business needs.
By involving the right stakeholders and clearly defining roles and responsibilities, organizations can ensure their data governance policies and procedures are robust, enforceable, and adaptable to changing requirements.
The following table lists the top 10 most popular companies that support data governance, data quality, policies, and procedures:
Together, data quality, policies, and procedures form a robust foundation for an effective data governance framework. They not only help organizations manage their data efficiently but also ensure that data remains a strategic asset driving growth and innovation.
By implementing these policies and procedures, organizations can ensure compliance with legal mandates, protect data integrity and privacy, and enable secure and effective data governance practices. This layered approach safeguards data assets while supporting the organization’s operational and strategic objectives.
In Terraform, comments are lines or sections of code that are ignored during execution but are useful for providing context, explanations, or notes wi......
Over the last couple of years, the tech industry has accelerated efforts to consolidate tooling and increase automation, in an effort to lighten the c......
React, introduced by Facebook (now Meta) in 2013, forever changed how developers build user interfaces. At that time, the front-end ecosystem already ......
Leveraging Neo4j for Effective Identity Access Management
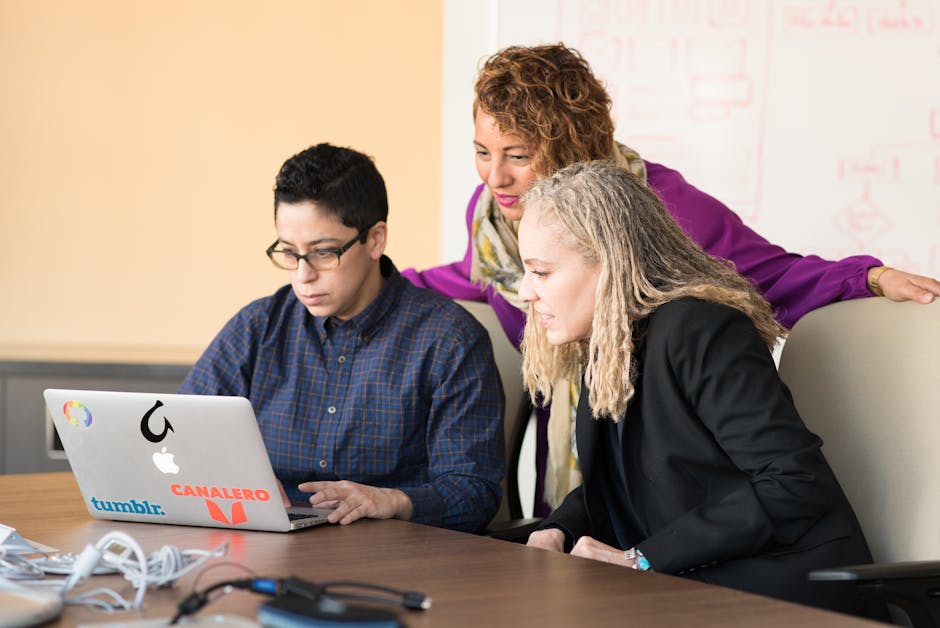
A while ago, I explored Neo4j, a powerful graph database, earlier for the implementation of the "six degrees of separation" concept. I like the way it simplified the complexity of the relationship visually with the advantage of flexible schema representation.
After some time, while exploring another use case in Identity Access Management (IAM) policies, I revisited Neo4j. I was amazed by the analytical capabilities it offered and the potential for building Generative AI applications using Neo4j’s GraphRAG.
In this article, we will see how we can use Neo4j for IAM data modeling, its use cases, guidelines, and best practices, and how Neo4j can be integrated with existing IAM systems and tools.
Identity Access Management (IAM) doesn’t need an introduction or a justification for its need, with so much happening in the field of cybersecurity. Ensuring authorized individuals, groups, and systems have appropriate access to the right resources at the right times. The key pillars of IAM, Access Management, Identity Governance and Administration, and Active Directory Management, are not a luxury anymore. Rather, they have become the key necessities of any organization looking forward to managing effective access management.
Neo4j is a powerful graph database that stores data and represents the relationship between data objects in a flexible graph structure. What I personally liked about Neo4j is the visual representation of complex scenarios. This helps in understanding simple yet powerful concepts of nodes, relationships, and attributes to depict, analyze, and implement complex use cases.
Neo4j can be effectively utilized to model, analyze, and enforce IAM policies. It helps an organization improve IAM compliance with adherence and audits of the implemented policies.
There is so much in common between the concepts of IAM and what Neo4j has to offer. The use case on fraud detection helped me understand and leverage the concepts for IAM.
Representation of IAM Entities Using Graph Model.
Initially, I explored this for the concept of "six degrees of separation," where the relationships can be visually represented. Similarly, IAM involves complex relationships between people, roles, groups, resources, and access permissions. Neo4j's native graph model simplifies this complexity by directly representing entities as nodes and their associations as edges. We will see the representation in detail under the "Data Modelling" section.
To be able to verify and validate access rights, user hierarchies, group memberships, and other critical access privileges, Neo4j provides real-time query capabilities.
The changes in roles, policies, and resources can be easily accommodated by Neo4j's schema-less model. It can also be used with a schema when needed. This flexibility is critical as the nature of IAM is so dynamic to be able to handle cyber threats and other challenges effectively.
As part of audit and compliance, it is critical to be able to identify orphaned accounts, notify excessive permissions, and detect policy violations. Neo4j’s Cypher query language provides analytical capabilities to perform such complex tasks.
We all know how crucial it is to have efficient and effective Data Modelling for any architecture. Neo4j leverages the concepts of graph theory, such as Vertices (also known as nodes), Edges (also known as relationships), and data points (also known as attributes).
Below are some examples of how nodes, relationships, and attributes can be represented in IAM.
User – represents an individual or service account.
– represents an individual or service account Group – represents collections of customers.
– represents collections of consumers Role – represents a set of permissions.
– represents a set of permissions Resource – represents a system, file, or service that requires access control.
– represents a system, file, or service that requires access control Permission – represents access rights ([website], READ, WRITE, EXECUTE).
Each node and relationship can have attributes like timestamps, descriptions, and status flags for audit and compliance tracking.
A sample schema is given that depicts two scenarios:
When a user is assigned to a role: Plain Text (User)-[:ASSIGNED]->(Role) When a user who is part of a certain group assigned to a role grants certain permission that applies to a certain resource: Plain Text (User)-[:MEMBER_OF]->(Group)-[:ASSIGNED]->(Role)-[:GRANTS]->(Permission)-[:APPLIES_TO]->(Resource).
I can’t think of any use case of IAM that may not be able to be implemented by Neo4j. In the next section, we will see some of them.
This refers to being able to understand if a user has access to a certain resource.
Query: "Does user X have access to resource Y?"
Plain Text MATCH (u:User {id: 'userX'})-[:ASSIGNED|MEMBER_OF*]->(r:Role)-[:GRANTS]->(p:Permission)-[:APPLIES_TO]->(res:Resource {id: 'resourceY'}) RETURN p.
This refers to identifying consumers or roles with access to sensitive resources. Also, we can detect permission assignments that violate the policy of the organization.
It can help analyze role hierarchies and remove redundant roles.
Plain Text MATCH (r:Role)-[:GRANTS]->(p:Permission) WITH r, COUNT(p) as permissionCount RETURN r, permissionCount ORDER BY permissionCount DESC.
It can detect orphaned accounts with the query to find individuals not assigned to any group or role.
Plain Text MATCH (u:User) WHERE NOT (u)-[:MEMBER_OF|ASSIGNED]->(:Group|:Role) RETURN u.
This addresses one of the complex queries on "What are all the resources user X can access through group Y?"
Plain Text MATCH (u:User {id: 'userX'})-[:MEMBER_OF]->(g:Group {id: 'groupY'})-[:ASSIGNED]->(r:Role)-[:GRANTS]->(p:Permission)-[:APPLIES_TO]->(res:Resource) RETURN res.
It is crucial to understand the guidelines and best practices to implement Neo4j effectively for IAM. Listing below some of the guidelines:
Security . Neo4j provides built-in role-based access control (RBAC), which can be leveraged to ensure secure access to the graph database.
. Neo4j provides built-in role-based access control (RBAC), which can be leveraged to ensure secure access to the graph database. Indexing . To be able to optimize query performance, the recommendation is to use indexes on frequently queried properties like [website], [website], and [website].
. To be able to optimize query performance, the recommendation is to use indexes on frequently queried properties like [website], [website], and [website] Ingestion . Since the data ingestion can impact the performance and load, the suggestion is to use batch imports or streaming tools like Neo4j’s ETL or Kafka Connectors for efficient data loading.
. Since the data ingestion can impact the performance and load, the suggestion is to use batch imports or streaming tools like Neo4j’s ETL or Kafka Connectors for efficient data loading. Monitoring . Despite the effective architecture & implementation, over a while, due to various reasons, the performance may degrade. Below are some of the recommendations. For visualization and tracking performance, Neo4j’s monitoring tools ([website], Neo4j Bloom, Prometheus) can be leveraged. As part of regular security audits and maintenance, the nodes and relationships are to be validated.
. Despite the effective architecture & implementation, over a while, due to various reasons, the performance may degrade. Below are some of the recommendations.
An organization can have multiple IAM systems and tools to strengthen its security posture. The below section details how Neo4j can be integrated with existing systems and tools.
Neo4j can be integrated with various IAM systems and tools, such as:
OAuth and OpenID connect . To model and analyze token-based access, Neo4j can be used.
. To model and analyze token-based access, Neo4j can be used. Active directory . To achieve centralized access control, the user and group data can be imported.
. To achieve centralized access control, the user and group data can be imported. Security Information and Event Management (SIEM) tools . In one of my articles, I have detailed how Elastic can be used as an effective SIEM tool. Neo4j queries and analytics can be fed into such systems for real-time monitoring.
. In one of my articles, I have detailed how Elastic can be used as an effective SIEM tool. Neo4j queries and analytics can be fed into such systems for real-time monitoring. Custom APIs. To enable IAM functionality in applications, Neo4j’s drivers can be used to build APIs using Neo4j’s drivers ([website], Java, Python, or JavaScript). Please refer to Neo4j’s documentation on creating applications for further details.
As the complexity and challenging requirements of Identity Access Management increase, we need a system such as Neo4j that provides a robust, flexible, and scalable. The visual representation and graphical analytical capabilities are particularly well-suited for modeling relationships, real-time visibility, enhancing compliance, and optimizing IAM policies and processes of an organization.
A consortium of 20 European research institutions, companies, and EuroHPC centers has launched OpenEuroLLM, an initiative to develop open-source, mult......
The traditional trade-off for distributed databases with high write speeds was availability for consistency. Version 8 of Aerospike’s performant multi......
The legal profession, steeped in centuries of tradition, is at a transformative crossroads. With 72% of legal professionals now viewing AI as a positi......
How Spring Boot Starters Integrate With Your Project
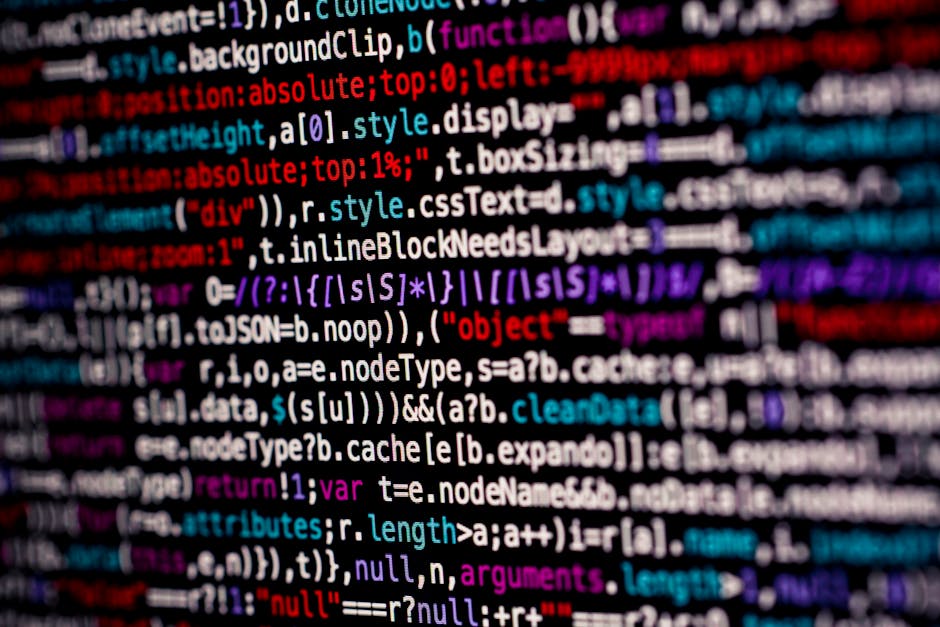
When developers set up and integrate services, they often face challenges that can take up a lot of time. Starters help simplify this process by organizing code and making it easier to manage. Let's take a look at creating two starters, configuring their settings automatically, and using them in a service.
So, what are Spring Boot Starters, exactly? What benefits do they provide?
Spring Boot Starters are like packages that streamline the process of incorporating libraries and components into Spring projects, making it simpler and more efficient to manage dependencies while cutting down development time significantly.
Starters include all the dependencies needed for technologies. For example spring-boot-starter-web provides everything for building web applications, while spring-boot-starter-data-jpa helps with JPA database work.
provides everything for building web applications, while helps with JPA database work. By adding these starters to a project, developers can start working with the desired technology without worrying about compatibility issues or version differences.
Developers can concentrate on creating business logic for dealing with infrastructure code.
This approach speeds up development and feature deployment, ultimately boosting team productivity.
Using predefined setups helps ensure consistency in setting up and organizing projects, making it easier to maintain and advance code. Moreover, it aids in onboarding team members to the project by offering a code structure and setup.
Furthermore, using starters that include known libraries simplifies updating dependencies and integrating Spring Boot versions.
The support from the Spring team community linked with these starters also guarantees to resolve any questions or obstacles that might come up during development.
In this article, we will address the issue of consolidating data from insights such as REST and GraphQL services. This problem is often encountered in projects with microservice architecture, where it is necessary to combine data coming from different services.
When it comes to solutions in a microservices setup, it’s possible to establish microservices for each integration. This approach is justifiable when the integration is extensive, and there are resources for its maintenance. However, in scenarios like working with a monolith or lacking the resources for multiple microservices support, opting for starters could be more practical.
The rationale behind selecting a library starter includes:
Business logic segmentation . Starters facilitate the separation of business logic and integration configuration.
. Starters facilitate the separation of business logic and integration configuration. Following the SOLID principles . Breaking down functionality into modules aligns with principles enhancing code maintainability and scalability.
. Breaking down functionality into modules aligns with principles enhancing code maintainability and scalability. Simplified setup . Starters streamline the process of configuring services by minimizing the required amount of configuration code.
. Starters streamline the process of configuring services by minimizing the required amount of configuration code. Ease of use. Integrating a service becomes more straightforward by adding a dependency and configuring essential parameters.
Let's illustrate the solution with an example involving a tour aggregator that gathers data from tour operators and merges them. To start off, we will develop two starters ( tour-operator-one-starter and tour-operator-two-starter ) both of which will use a shared module ( common-model ) containing fundamental models and interfaces. These starter libraries will connect to the aggregator service ( tour-aggregator ).
Starter is designed to integrate with the tour operator and fetch data via the REST API.
All official starters use the naming scheme spring-boot-starter-* , where * denotes a specific type of application. Third-party starters should not start with spring-boot as it is reserved for official starters from the Spring team.
Typically, third-party starters begin with the project name. For example, my starter will be named tour-operator-one-spring-boot-starter .
XML [website] spring-boot-starter-web [website] spring-boot-configuration-processor true [website] common-model [website] [website] spring-boot-starter-test test.
These are the properties we will set in tour-aggregator to configure our starter.
XML @ConfigurationProperties(prefix = "[website]") public class TourOperatorOneProperties { private final Boolean enabled; private final String url; private final Credentials credentials; public TourOperatorOneProperties( Boolean enabled, String url, Credentials credentials) { this.enabled = enabled; [website] = url; this.credentials = credentials; } //getters public static class Credentials { private final String username; private final String password; public Credentials(String username, String password) { this.username = username; this.password = password; } //getters } }.
3. Create TourOperatorOneAutoConfiguration.
@AutoConfiguration – indicates that this class is a configuration class for Spring Boot auto-configuration.
– indicates that this class is a configuration class for Spring Boot auto-configuration. @ConditionalOnProperty – activates the configuration if the property [website] is set to true . If the property is missing, the configuration is also activated due to matchIfMissing = true .
– activates the configuration if the property is set to . If the property is missing, the configuration is also activated due to . @EnableConfigurationProperties([website] – enables support for @ConfigurationProperties annotations for the TourOperatorOneProperties class.
XML @AutoConfiguration @ConditionalOnProperty(prefix = "[website]", name = "enabled", havingValue = "true", matchIfMissing = true) @EnableConfigurationProperties([website] public class TourOperatorOneAutoconfiguration { private static final Logger log = LoggerFactory.getLogger([website]; private final TourOperatorOneProperties properties; public TourOperatorOneAutoconfiguration(TourOperatorOneProperties properties) { this.properties = properties; } @Bean("operatorOneRestClient") public RestClient restClient(RestClient.Builder builder) { [website]"Configuration operatorRestClient: {}", properties); return builder .baseUrl([website] .defaultHeaders(httpHeaders -> { if (null != properties.getCredentials()) { httpHeaders.setBasicAuth( properties.getCredentials().getUsername(), properties.getCredentials().getPassword()); } }) .build(); } @Bean("tourOperatorOneService") public TourOperatorOneServiceImpl tourOperatorService(TourOperatorOneProperties properties, @Qualifier("operatorOneRestClient") RestClient restClient) { [website]"Configuration tourOperatorService: {} and restClient: {}", properties, restClient); return new TourOperatorOneServiceImpl(restClient); } }.
In this example, I use @ConditionalOnProperty , but there are many other conditional annotations:
@ConditionalOnBean – generates a bean when a specified bean exists in the BeanFactory @ConditionalOnMissingBean – facilitates creating a bean if a particular bean is not found in the BeanFactory @ConditionalOnClass – produces a bean when a specific class is present, in the classpath @ConditionalOnMissingClass – acts oppositely to @ConditionalOnClass.
You should choose what suits your needs best. You can learn more about conditional annotations here.
In this class, we implement the base interface and lay down the main business logic for retrieving data from the first tour operator and standardizing it .
Plain Text public class TourOperatorOneServiceImpl implements TourOperatorService { private final RestClient restClient; public TourOperatorOneServiceImpl(@Qualifier("operatorOneRestClient") RestClient restClient) { this.restClient = restClient; } @Override public TourOperatorResponse makeRequest(TourOperatorRequest request) { var tourRequest = mapToOperatorRequest(request); // transformation of our request into the one that the tour operator will understand var responseList = restClient .post() .body(tourRequest) .retrieve() .toEntity(new ParameterizedTypeReference>() { }); return TourOperatorResponse.builder() .deals(responseList .getBody() .stream() .map(ModelUtils::mapToCommonModel) .toList()) .build(); } }.
To register auto-configurations, we create the file resources/META-INF/spring/[website] .
This file contains a collection of configurations. In my scenario, one configuration is listed. If you have multiple configurations, make sure that each configuration is listed on a separate line.
By creating this file, you are informing Spring Boot that it should load and utilize the TourOperatorOneAutoConfiguration class for setup when certain conditions specified by the @ConditionalOnProperty annotation are satisfied.
Thus, we have established the setup for collaborating with the tour operator by developing configuration classes and beans and leveraging properties.
Up is creating tour-operator-two-starter a kit designed to integrate with the second tour operator and retrieve data from a GraphQL server through a straightforward HTTP request.
Let's proceed with the process used for tour-operator-one-starter .
XML [website] spring-boot-starter-web [website] spring-boot-configuration-processor true [website] common-model [website] [website] spring-boot-starter-test test.
These are the properties we will set in tour-aggregator to configure our starter.
@ConfigurationProperties(prefix = "[website]") public class TourOperatorTwoProperties { private final Boolean enabled; private final String url; private final String apiKey; public TourOperatorTwoProperties( Boolean enabled, String url, String apiKey) { this.enabled = enabled; [website] = url; [website] = apiKey; } //getters }.
2. Create TourOperatorOneAutoConfiguration.
Java @AutoConfiguration @ConditionalOnProperty(prefix = "[website]", name = "enabled", havingValue = "true", matchIfMissing = true) @EnableConfigurationProperties([website] public class TourOperatorTwoAutoconfiguration { private static final Logger log = LoggerFactory.getLogger([website]; private final TourOperatorTwoProperties properties; public TourOperatorTwoAutoconfiguration(TourOperatorTwoProperties properties) { [website]"Configuration with: {}", properties); this.properties = properties; } @Bean("operatorTwoRestClient") public RestClient restClient(RestClient.Builder builder) { [website]"Configuration operatorRestClient: {}", properties); return builder .baseUrl([website] .defaultHeaders(httpHeaders -> { [website]"X-Api-Key", properties.getApiKey()); }) .build(); } @Bean("tourOperatorTwoService") public TourOperatorTwoServiceImpl tourOperatorService(TourOperatorTwoProperties properties, @Qualifier("operatorTwoRestClient") RestClient restClient) { [website]"Configuration tourOperatorService: {} and restClient: {}", properties, restClient); return new TourOperatorTwoServiceImpl(restClient); } }.
Receiving data from the second tour operator.
Java public class TourOperatorTwoServiceImpl implements TourOperatorService { private static final String QUERY = """ query makeTourRequest($request: TourOperatorRequest) { makeTourRequest(request: $request) { id startDate endDate price currency days hotel { hotelName hotelRating countryCode } } } """; private final RestClient restClient; public TourOperatorTwoServiceImpl(@Qualifier("operatorTwoRestClient") RestClient restClient) { this.restClient = restClient; } @Override public TourOperatorResponse makeRequest(TourOperatorRequest request) { var tourRequest = mapToOperatorRequest(request); var variables = Map.ofEntries([website]"request", tourRequest)); var requestBody = Map.ofEntries( [website]"query", QUERY), [website]"variables", variables)); var response = restClient .post() .body(requestBody) .retrieve() .toEntity([website]; return TourOperatorResponse.builder() .deals(response.getBody() .data() .makeTourRequest() .stream() .map(ModelUtils::mapToCommonModel).toList()) .build(); } }.
Create the file resources/META-INF/spring/[website] autoconfigure.AutoConfiguration.imports .
Creating and Using the Aggregator Service.
An aggregator service is designed to gather data from tour operators. This involves linking starters, configuring parameters, and using beans with a shared interface.
Include dependencies for the two libraries in the [website] .
XML ... [website] tour-operator-one-spring-boot-starter [website] [website] tour-operator-two-spring-boot-starter [website] ...
Specify the necessary data, such as URLs and connection parameters, in the [website] .
YAML spring: application: name: tour-aggregator tour-operator: one: service: enabled: true url: [website]:8090/api/tours credentials: username: user123 password: pass123 two: service: enabled: true url: [website]:8091/graphql api-key: 11d1de45-5743-4b58-9e08-f6038fe05c8f.
We use the established beans, which implement the TourOperatorService interface within the TourServiceImpl class. This class outlines the process of retrieving and aggregating data from various tour operators.
Java @Service public class TourServiceImpl implements TourService { private static final Logger log = LoggerFactory.getLogger([website]; private final List tourOperatorServices; private final Executor tourOperatorExecutor; private final Integer responseTimeout; public TourServiceImpl(List tourOperatorServices, @Qualifier("tourOperatorTaskExecutor") Executor tourOperatorExecutor, @Value("${app.response-timeout:5}") Integer responseTimeout) { this.tourOperatorServices = tourOperatorServices; this.tourOperatorExecutor = tourOperatorExecutor; this.responseTimeout = responseTimeout; } public List getTourOffers(@RequestBody TourOperatorRequest request) { [website]"Send request: {}", request); var futures = [website] .map(tourOperator -> CompletableFuture.supplyAsync(() -> tourOperator.makeRequest(request), tourOperatorExecutor) .orTimeout(responseTimeout, TimeUnit.SECONDS) .exceptionally(ex -> TourOperatorResponse.builder().deals([website] ) .toList(); var response = [website] .map(CompletableFuture::join) .map(TourOperatorResponse::getDeals) .filter(Objects::nonNull) .flatMap(List::stream) .toList(); return response; } }.
It’s good practice to allocate separate resources for calls, allowing advanced thread management and performance optimization.
Java @Configuration public class ThreadPoolConfig { private final Integer threadCount; public ThreadPoolConfig(@Value("${[website]}") Integer threadCount) { this.threadCount = threadCount; } @Bean(name = "tourOperatorTaskExecutor") public Executor tourOperatorTaskExecutor() { return Executors.newFixedThreadPool(threadCount); } }.
This code ensures efficient management of asynchronous tasks and helps avoid blocking the main thread, thereby improving overall system performance.
In this article, we’ve created two starters for reaching out to tour operators through REST and GraphQL technology interfaces. These steps include all the configurations and elements to simplify their usage. Afterward, we merged them into a system that communicates with them in an asynchronous manner and aggregates data.
Simplified integration and setup . By using auto-configuration and properties of coding, we saved time during development.
. By using auto-configuration and properties of coding, we saved time during development. Improved flexibility and usability . Separating functions into starters improved code structure and simplified maintenance.
. Separating functions into starters improved code structure and simplified maintenance. System flexibility. We can easily add new integrations without breaking the existing logic.
Now, our system is improved equipped to adapt and scale effortlessly while being easier to manage, leading to enhancements in its architecture and performance.
I appreciate you reading this article. I look forward to hearing your thoughts and feedback!
As the Trump administration revokes Executive Order 14110, the [website] shifts toward a market-driven AI strategy, departing from the Biden administration......
Testing is a critical yet often time-consuming process. Ensuring that every feature, flow, and edge case works as intended can take up significant res......
DeepSeek open-sourced DeepSeek-R1, an LLM fine-tuned with reinforcement learning (RL) to improve reasoning capability. DeepSeek-R1 achieves results on......
Market Impact Analysis
Market Growth Trend
2018 | 2019 | 2020 | 2021 | 2022 | 2023 | 2024 |
---|---|---|---|---|---|---|
7.5% | 9.0% | 9.4% | 10.5% | 11.0% | 11.4% | 11.5% |
Quarterly Growth Rate
Q1 2024 | Q2 2024 | Q3 2024 | Q4 2024 |
---|---|---|---|
10.8% | 11.1% | 11.3% | 11.5% |
Market Segments and Growth Drivers
Segment | Market Share | Growth Rate |
---|---|---|
Enterprise Software | 38% | 10.8% |
Cloud Services | 31% | 17.5% |
Developer Tools | 14% | 9.3% |
Security Software | 12% | 13.2% |
Other Software | 5% | 7.5% |
Technology Maturity Curve
Different technologies within the ecosystem are at varying stages of maturity:
Competitive Landscape Analysis
Company | Market Share |
---|---|
Microsoft | 22.6% |
Oracle | 14.8% |
SAP | 12.5% |
Salesforce | 9.7% |
Adobe | 8.3% |
Future Outlook and Predictions
The Data Governance Essentials landscape is evolving rapidly, driven by technological advancements, changing threat vectors, and shifting business requirements. Based on current trends and expert analyses, we can anticipate several significant developments across different time horizons:
Year-by-Year Technology Evolution
Based on current trajectory and expert analyses, we can project the following development timeline:
Technology Maturity Curve
Different technologies within the ecosystem are at varying stages of maturity, influencing adoption timelines and investment priorities:
Innovation Trigger
- Generative AI for specialized domains
- Blockchain for supply chain verification
Peak of Inflated Expectations
- Digital twins for business processes
- Quantum-resistant cryptography
Trough of Disillusionment
- Consumer AR/VR applications
- General-purpose blockchain
Slope of Enlightenment
- AI-driven analytics
- Edge computing
Plateau of Productivity
- Cloud infrastructure
- Mobile applications
Technology Evolution Timeline
- Technology adoption accelerating across industries
- digital transformation initiatives becoming mainstream
- Significant transformation of business processes through advanced technologies
- new digital business models emerging
- Fundamental shifts in how technology integrates with business and society
- emergence of new technology paradigms
Expert Perspectives
Leading experts in the software dev sector provide diverse perspectives on how the landscape will evolve over the coming years:
"Technology transformation will continue to accelerate, creating both challenges and opportunities."
— Industry Expert
"Organizations must balance innovation with practical implementation to achieve meaningful results."
— Technology Analyst
"The most successful adopters will focus on business outcomes rather than technology for its own sake."
— Research Director
Areas of Expert Consensus
- Acceleration of Innovation: The pace of technological evolution will continue to increase
- Practical Integration: Focus will shift from proof-of-concept to operational deployment
- Human-Technology Partnership: Most effective implementations will optimize human-machine collaboration
- Regulatory Influence: Regulatory frameworks will increasingly shape technology development
Short-Term Outlook (1-2 Years)
In the immediate future, organizations will focus on implementing and optimizing currently available technologies to address pressing software dev challenges:
- Technology adoption accelerating across industries
- digital transformation initiatives becoming mainstream
These developments will be characterized by incremental improvements to existing frameworks rather than revolutionary changes, with emphasis on practical deployment and measurable outcomes.
Mid-Term Outlook (3-5 Years)
As technologies mature and organizations adapt, more substantial transformations will emerge in how security is approached and implemented:
- Significant transformation of business processes through advanced technologies
- new digital business models emerging
This period will see significant changes in security architecture and operational models, with increasing automation and integration between previously siloed security functions. Organizations will shift from reactive to proactive security postures.
Long-Term Outlook (5+ Years)
Looking further ahead, more fundamental shifts will reshape how cybersecurity is conceptualized and implemented across digital ecosystems:
- Fundamental shifts in how technology integrates with business and society
- emergence of new technology paradigms
These long-term developments will likely require significant technical breakthroughs, new regulatory frameworks, and evolution in how organizations approach security as a fundamental business function rather than a technical discipline.
Key Risk Factors and Uncertainties
Several critical factors could significantly impact the trajectory of software dev evolution:
Organizations should monitor these factors closely and develop contingency strategies to mitigate potential negative impacts on technology implementation timelines.
Alternative Future Scenarios
The evolution of technology can follow different paths depending on various factors including regulatory developments, investment trends, technological breakthroughs, and market adoption. We analyze three potential scenarios:
Optimistic Scenario
Rapid adoption of advanced technologies with significant business impact
Key Drivers: Supportive regulatory environment, significant research breakthroughs, strong market incentives, and rapid user adoption.
Probability: 25-30%
Base Case Scenario
Measured implementation with incremental improvements
Key Drivers: Balanced regulatory approach, steady technological progress, and selective implementation based on clear ROI.
Probability: 50-60%
Conservative Scenario
Technical and organizational barriers limiting effective adoption
Key Drivers: Restrictive regulations, technical limitations, implementation challenges, and risk-averse organizational cultures.
Probability: 15-20%
Scenario Comparison Matrix
Factor | Optimistic | Base Case | Conservative |
---|---|---|---|
Implementation Timeline | Accelerated | Steady | Delayed |
Market Adoption | Widespread | Selective | Limited |
Technology Evolution | Rapid | Progressive | Incremental |
Regulatory Environment | Supportive | Balanced | Restrictive |
Business Impact | Transformative | Significant | Modest |
Transformational Impact
Technology becoming increasingly embedded in all aspects of business operations. This evolution will necessitate significant changes in organizational structures, talent development, and strategic planning processes.
The convergence of multiple technological trends—including artificial intelligence, quantum computing, and ubiquitous connectivity—will create both unprecedented security challenges and innovative defensive capabilities.
Implementation Challenges
Technical complexity and organizational readiness remain key challenges. Organizations will need to develop comprehensive change management strategies to successfully navigate these transitions.
Regulatory uncertainty, particularly around emerging technologies like AI in security applications, will require flexible security architectures that can adapt to evolving compliance requirements.
Key Innovations to Watch
Artificial intelligence, distributed systems, and automation technologies leading innovation. Organizations should monitor these developments closely to maintain competitive advantages and effective security postures.
Strategic investments in research partnerships, technology pilots, and talent development will position forward-thinking organizations to leverage these innovations early in their development cycle.
Technical Glossary
Key technical terms and definitions to help understand the technologies discussed in this article.
Understanding the following technical concepts is essential for grasping the full implications of the security threats and defensive measures discussed in this article. These definitions provide context for both technical and non-technical readers.