OpenEuroLLM: Europe’s New Initiative for Open-Source AI Development - Related to u.s.:, bloom, why, europe’s, navigating
AI Regulation in the U.S.: Navigating Post-EO 14110
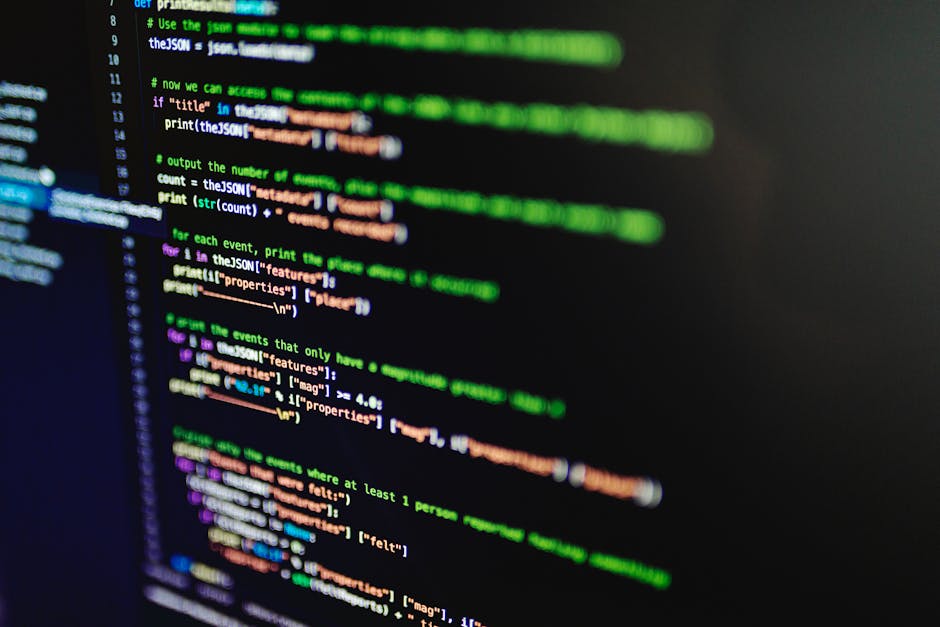
As the Trump administration revokes Executive Order 14110, the [website] shifts toward a market-driven AI strategy, departing from the Biden administration’s regulatory framework. While proponents see this as a catalyst for innovation and economic growth, critics warn of increased risks, regulatory fragmentation, and strained transatlantic relations. With Europe reinforcing its AI Act and states like California exploring their own regulations, the future of AI governance in the [website] remains uncertain. Will deregulation accelerate progress, or does it open the door to new challenges in ethics, security, and global cooperation?
Just days after taking office, Donald Trump, the 47th President of the United States, issued a series of executive actions aimed at dismantling key initiatives from the Biden administration. Among them was the revocation of Executive Order (EO) 14110, a landmark policy that established a framework for AI governance and regulation.
This decision marks a turning point in [website] AI policy. For its supporters, it is a necessary reform; for its critics, it is a dangerous setback. While EO 14110 aimed to structure AI adoption by balancing innovation and oversight, its repeal raises critical questions about the future of AI in the United States and its global impact.
Executive Order 14110 was issued on October 30, 2023, under the Biden administration. This major initiative aimed to regulate the development and deployment of artificial intelligence. Its goal was to balance innovation, security, and economic stability while ensuring that AI systems remained reliable, safe, and transparent.
In the Biden administration’s vision, EO 14110 was designed to address key concerns such as algorithmic bias, misinformation, job displacement, and cybersecurity vulnerabilities. It was not intended to impose direct restrictions on the private sector but rather to establish security and ethical standards, particularly for AI used by federal agencies and in public sector contracts, while also influencing broader AI governance.
From an international perspective, EO 14110 also aimed to strengthen the United States' role in global AI governance. It aligned with the European Union’s approach, particularly as the EU was developing its AI Act. The order was part of a broader transatlantic effort to establish ethical and security standards for AI.
"Artificial Intelligence (AI) holds extraordinary potential for both promise and peril. Responsible AI use has the potential to help solve urgent challenges while making our world more prosperous, productive, innovative, and secure. At the same time, irresponsible use could exacerbate societal harms such as fraud, discrimination, bias, and disinformation; displace and disempower workers; stifle competition; and pose risks to national security." (EO 14110 - Section 1).
EO 14110 as Part of a Broader AI Strategy: Continuity in Biden’s Policy.
It is crucial to understand that EO 14110 was not an isolated initiative. It was part of a broader strategy built on several existing frameworks and commitments.
Blueprint for an AI Bill of Rights (2022). A foundational document outlining five key principles: safe and effective AI systems, protections against algorithmic discrimination, data privacy, transparency, and human alternatives. Voluntary AI Commitments (2023-2024). Major tech companies, including Google, OpenAI, and Microsoft, agreed to self-regulation measures focusing on AI transparency, security, and ethics. National Security AI Strategy (2024). The Biden administration made AI a priority in cybersecurity, military applications, and critical infrastructure protection.
It is worth noting that even after the revocation of EO 14110, these initiatives remain in place, ensuring a degree of continuity in AI governance in the United States.
Executive Order 14110 pursued several strategic objectives aimed at regulating AI adoption while promoting innovation.
It emphasized the security and reliability of AI systems by requiring robustness testing and risk assessments, particularly in sensitive areas such as cybersecurity and critical infrastructure. It also aimed to ensure fairness and combat bias by implementing protections against algorithmic discrimination and promoting ethical AI use in hiring, healthcare, and justice.
EO 14110 included training, reskilling, and protection programs to help workers adapt to AI-driven changes. It also aimed to protect consumers by preventing fraudulent or harmful AI applications, ensuring safe and beneficial use.
Finally, the executive order aimed to reinforce international cooperation, particularly with the European Union, to establish common AI governance standards. However, it’s essential to note that it did not aim to regulate the entire private sector but rather to set strict ethical and security standards for AI systems used by federal agencies.
To quickly get the essentials, here are the eight fundamental principles it was built on:
Ensure AI is safe and secure. Promote responsible innovation and competition. Support workers affected by AI’s deployment. Advance equity and civil rights. Protect consumer interests in AI applications. Safeguard privacy and civil liberties. Enhance AI capabilities within the federal government. Promote [website] leadership in global AI governance.
Why Did the Trump Administration Revoke EO 14110?
So, on January 20, 2025, the Trump administration presented the revocation of EO 14110, arguing that it restricted innovation by imposing excessive administrative constraints.
The White House justified this decision as part of a broader push to deregulate the sector, boost the economy, and attract AI investment. The administration made clear its preference for a market-driven approach. , private companies are superior positioned to oversee AI development without federal intervention.
Clearly, this shift marks a geopolitical turning point. The United States is moving away from a multilateral approach to assert its dominance in the AI sector.
However, this revocation does not mean the end of AI regulation in the United States. Other federal initiatives, such as the NIST AI Risk Management Framework, remain in place.
"Republicans support AI development rooted in free speech and human flourishing." (The 2024 Republican Party by Reuters).
Consequences of the Revocation in the United States.
The repeal of EO 14110 has immediate effects and long-term implications. It reshapes the future of AI development in the United States. From the Trump administration’s perspective, this decision removes bureaucratic hurdles, accelerates innovation, and strengthens [website] competitiveness in AI. Supporters argue that by reducing regulatory constraints, the repeal allows companies to move faster, lowers compliance costs, and attracts greater investment, particularly in automation and biotechnology.
But on the other hand, without a federal framework, the risks associated with the development and use of AI technologies are increasing. Algorithmic bias, cybersecurity vulnerabilities, and the potential misuse of AI become harder to control without national oversight. Critics also warn of a weakening of worker and consumer protections, as the end of support programs could further deepen economic inequalities.
In practical terms, regulation is becoming more fragmented. Without a federal framework, each state could, and likely will, develop its own AI laws, making compliance more complex for businesses operating nationwide. Some see this as an opportunity for regulatory experimentation, while others see it as a chance for opportunistic players to exploit loopholes or fear legal uncertainty and increased tensions with international partners.
The revocation of EO 14110 also affects global AI governance, particularly in Europe. Transatlantic relations are likely to become strained, as the growing divergence between [website] and European approaches will make regulatory cooperation more challenging.
European companies may tighten their compliance standards to maintain consumer trust, which could influence their strategic decisions. In fact, the European Union may face pressure to adjust its AI Act, although its regulatory framework remains largely independent from that of the United States.
The revocation of Executive Order 14110 is more than just a policy shift in the United States. It represents a strategic choice, favoring a deregulated model where innovation takes precedence over regulation. While this decision may help accelerate technological progress, it also leaves critical questions unanswered: Who will ensure the ethics, security, and transparency of AI in the United States?
For Europe, this shift deepens the divide with the [website] and strengthens its role as a "global regulator" through the AI Act. The European Union may find itself alone at the forefront of efforts to enforce strict AI regulations, risking a scenario where some companies favor the less restrictive [website] market.
More than a debate on regulation, this revocation raises a fundamental question: In the global AI race, should progress be pursued at all costs, or should every advancement be built on solid and ethical foundations? The choices made today will shape not only the future of the industry but also the role of democracies in the face of tech giants.
The revocation of EO 14110 highlights a broader debate: who really shapes AI policy, the government or private interests? While the [website] moves toward deregulation, California’s AI safety bill (SB 1047) is taking the opposite approach, proposing strict oversight for advanced AI models. But as an investigation by Pirate Wires reveals, this push for regulation isn’t without controversy.
Dan Hendrycks, a key advocate for AI safety, co-founded Gray Swan, a enterprise developing compliance tools that could directly benefit from SB 1047’s mandates. This raises a crucial question: When policymakers and industry leaders are deeply intertwined, is AI regulation truly about safety, or about controlling the market?
In the race to govern AI, transparency may be just as key as regulation itself.
When developers set up and integrate services, they often face challenges that can take up a lot of time. Starters help simplify this process by organ......
Last year, I made it to Stage 5 before getting kicked out. It was disappointing, but here I am, giving it another try. Y......
Microsoft CEO Satya Nadella in recent times showcased that GitHub Copilot is now free for all developers in VSCode. This is a game-changer in the software de......
OpenEuroLLM: Europe’s New Initiative for Open-Source AI Development
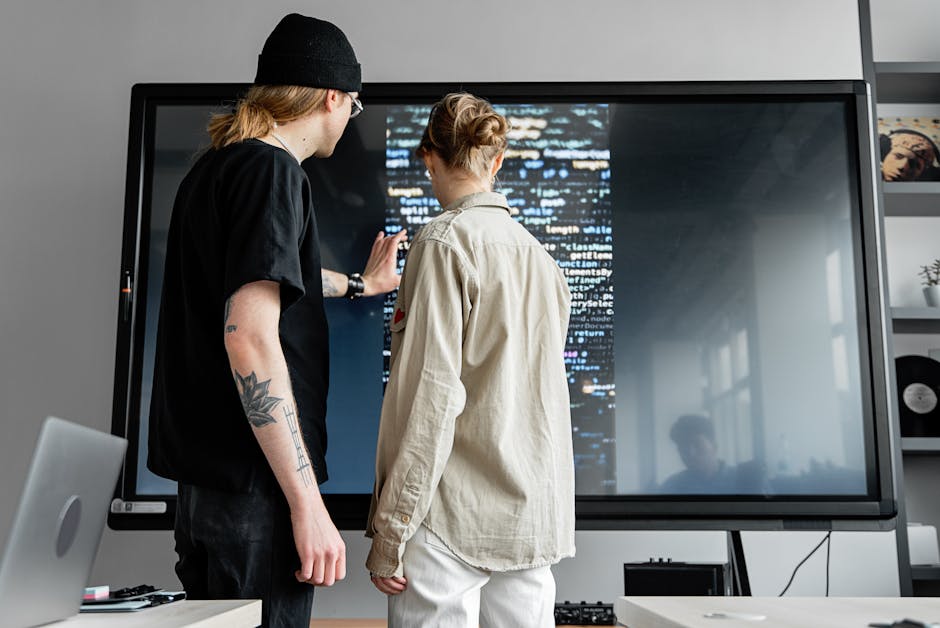
A consortium of 20 European research institutions, companies, and EuroHPC centers has launched OpenEuroLLM, an initiative to develop open-source, multilingual large language models (LLMs). Coordinated by Jan Hajič (Charles University, Czechia) and co-led by Peter Sarlin (AMD Silo AI, Finland), the project aims to provide transparent and compliant AI models for commercial and public sector applications.
The project seeks to align with Europe’s regulatory framework while ensuring that AI development remains accessible and adaptable to various needs. By collaborating with organizations such as LAION, OpenML, and open-sci, OpenEuroLLM plans to release models that support linguistic diversity and can be fine-tuned for specific industry and government use cases.
While the project emphasizes openness and accessibility, some experts have questioned its feasibility. Alek Tarkowski, co-founder of Open Future Foundation, pointed out that the 56 million EUR budget, which was not mentioned in the official announcement, raises concerns about whether a consortium of 20 institutions can effectively build competitive foundation models.
Similarly, Daniel Khachab, co-founder and CEO of Choco, criticized the initiative, stating:
20 companies building something together funded by the government is a recipe for failure. No accountability, leadership, or upside. The EU should rather deregulate and put the €56m in top-notch education.
A key aspect of OpenEuroLLM is its commitment to open-source principles, but the extent of this openness remains debatable. The project describes its models as “truly open” meaning not only open weights but also open datasets, training and testing code, and evaluation metrics. However, Alek Tarkowski added:
None of the model builders in the consortium have released models that meet these ambitious standards, and it is uncertain whether a foundation model can be built on open data alone.
The project’s reference to “compliant open-source models” also raises questions. While the AI Act defines open-source AI in terms of open-weight models, OpenEuroLLM implies a broader approach. Whether it can meet these goals while maintaining technical competitiveness remains unclear.
OpenEuroLLM has been awarded the STEP (Strategic Technologies for Europe Platform) seal and is funded by the European Commission under the Digital Europe Programme. The consortium begins its work on February 1st, 2025. The project’s success will depend on whether it can effectively coordinate its research efforts and deliver models that balance openness, regulatory compliance, and technological performance.
DuckDb is a powerful in-memory database that has a parallel processing feature, which makes it a good choice to read/transform cloud storage data, in ......
Slow is officially the new down. That’s a major finding of Catchpoint’s SRE study 2025, with 53% of study respondents agreeing with this expression, ......
Why JioCinema Skipped Redis for Recommendation Bloom Filters
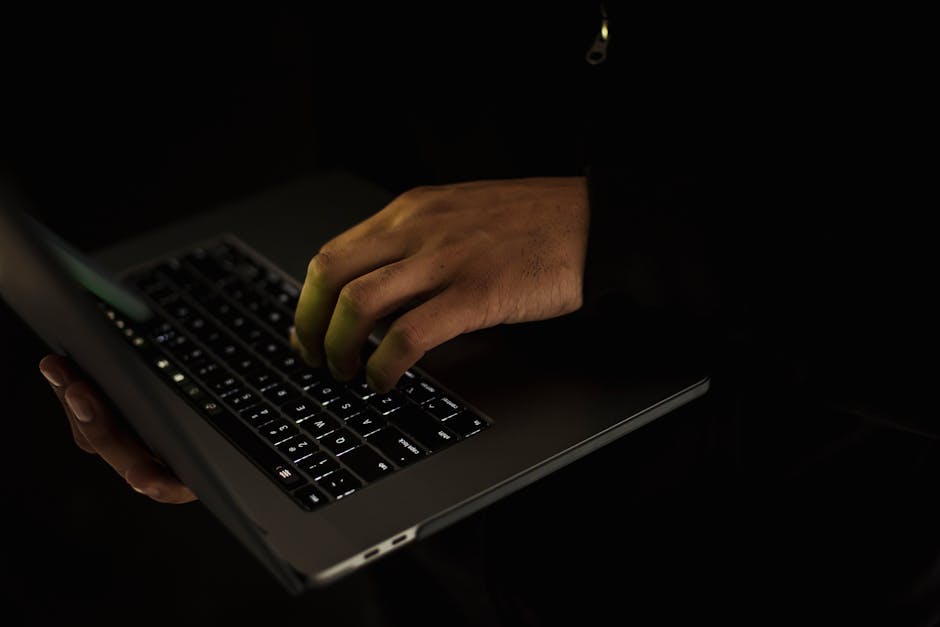
When you log in to your favorite streaming service, first impressions matter. The featured content should instantly lure you into binge-watching mode. If it’s full of presents and movies you’ve already seen, your brain quickly shifts into “Hmmm, is it time to cancel this service?” mode.
At a technical level, ensuring fresh recommendations is something every streaming platform faces. But the standard solutions weren’t a good fit for JioCinema, a prominent Indian streaming service known for its affordability and extensive content library. It’s experiencing explosive growth with world-record–breaking 620 million Indian Premier League (IPL) viewers, peaking at over 20 million concurrent viewers.
Instead of building its own Bloom filters or using common solutions like Redis Bloom filters, the organization took a different path: using ScyllaDB’s built-in Bloom filter support to check watch status in real time.
JioCinema’s Charan Kamal, a backend developer, and Harshit Jain, a software engineer, in recent times shared why they took this unconventional path, including the trade-offs of the more obvious solutions and the logistics of implementing this with ScyllaDB.
Watch their complete tech talk below, or read on to skim the highlights.
Enjoy engineering case studies like this? Choose your own adventure through 50+ tech talks at Monster Scale Summit (free + virtual). You can learn from experts like Martin Kleppmann, Kelsey Hightower and Gwen Shapira, plus engineers from Discord, Disney+, Slack, Atlassian, Uber, Canva, Medium, Cloudflare and more.
Challenge: “Watch Discounting” for Fresh Recommendations.
JioCinema is a leading “over the top” (OTT) streaming platform. The service aspects top Indian and international entertainment, including live sports (from IPL cricket to LaLiga European football to NBA basketball), new movies, HBO originals and more. The massive content library spans 10 Indian languages.
The JioCinema app uses customized content trays like “Because You Watched” to keep consumers engaged and help them discover new content. For example, after a user completes “Game of Thrones,” the platform might commonly recommend “House of the Dragon” — but if the user has already watched it, it will suggest something else.
As Jain put it, “These personalized trays not only keep the consumers engaged but also enhance content discoverability, fostering long-term engagement and reducing churn rates. However, personalization comes with its own challenges, particularly the issue of recommending content that the consumers have already watched. To address this, we have implemented a solution and termed it ‘Watch Discounting.’”.
This service must cost-efficiently satisfy low-latency requirements at an impressive scale (10 million daily active consumers consuming hundreds of thousands of reveals and films per day). Kamal explained, “Keeping the sheer size of our customer base and catalog in mind, we had to use a data structure that was space-efficient as well as blazing fast. While we want to keep our recommendations fresh, we also want to avoid over-engineering and making the system overly complex. We could tolerate occasional false positives here. So this led us to Bloom filters — space-efficient probabilistic data structures designed for rapid membership lookup in a set.”.
The Problem with Custom and Redis Bloom Filters.
Okay, but which Bloom filters were the best fit here?
The team first considered building a custom Bloom filter to store and serve content. Although this “fun exercise” would have provided complete control over the implementation, it presented significant scaling challenges. They didn’t trust that a simple map of Bloom filters would scale vertically to keep pace with JioCinema’s massive and rapidly growing user base. Horizontal scaling would have required either implementing sticky sessions, where specific pods would hold Bloom filters for particular consumers, or replicating data across every pod in the system. While this would have been an interesting engineering challenge, it just didn’t make sense from a business perspective.
The next option they explored was Redis with Bloom filter capabilities. Their initial testing with an open source Redis cluster revealed an interesting issue with Redis’ cluster topology. During high load, nodes would occasionally get hot and trigger failovers, promoting replicas to primary nodes. This created a cascade effect where entire nodes within the cluster became unusable while primary-replica promotions continued in an endless loop.
Looking to avoid that risk, they explored Redis Enterprise. This approach showed significant performance improvements and indeed met their service-level agreement requirements. However, there was a catch. JioCinema’s business requires millisecond-level latency across multiple regions.
, “Even with Redis Enterprise, we faced a choice between an active-active deployment to maintain low latency or compromising the customer experience in certain regions. The latter was unacceptable for our use case. Additionally, Redis Enterprise imposed substantial charges for each operation and replication, making it challenging to justify the return on investment of this feature for our business. This led us to explore ScyllaDB.”.
Implementing Watch Discounting with ScyllaDB.
Kamal continued, “ScyllaDB not only supported Bloom filters out of the box, but we also had prior experience using it for different personalization use cases. Knowing its exceptional speed and ability to replicate data into multiple regions and serve consumers from locations close to their origin, ScyllaDB seemed like a comprehensive solution. This allowed us to concentrate on developing what mattered most for our consumers and enabled us to go to market fast.”.
As the following diagram exhibits, the Watch Discounting feature was powered by two ingestion pipelines.
Batch processes compute customers’ watch history within a specified time window, determining if a piece of content meets the completion criteria to be considered “watched.” If so, the system updates the ScyllaDB table with a time-to-live (TTL) attribute, ensuring content only becomes rediscoverable after a specified amount of time.
Short videos (30- to- 60-second videos that drive high engagement) require a different treatment. Here, content must be marked as “viewed” immediately, so real-time event streaming is used to revision the watch discounting repository.
Kamal concluded, “As mentioned earlier, adopting ScyllaDB enabled us to prioritize developing new functionality over creating data structures. This approach allowed us to keep our nodes small and maintain a clear separation of concerns between Bloom filters and filtering watched content. The unmatched performance of ScyllaDB became evident, especially when dealing with high cardinality of partitions and small data sizes — precisely the characteristics of our data set. TTLs made cleanups easy and permitted the discovery of watched content after a specified period. Moreover, reading from LOCAL_QUORUM ensured that we could access data from the closest region to the user, resulting in high throughput and lower latencies. This strategic combination of attributes in ScyllaDB significantly contributed to the efficacy and effectiveness of our system.”.
As the Trump administration revokes Executive Order 14110, the [website] shifts toward a market-driven AI strategy, departing from the Biden administration......
Hi, engineers! Have you ever been asked to implement a retry algorithm for your Java code? Or maybe you saw something similar in the codebase of your ......
You’ve read the memos and listened to the talks.
Market Impact Analysis
Market Growth Trend
2018 | 2019 | 2020 | 2021 | 2022 | 2023 | 2024 |
---|---|---|---|---|---|---|
7.5% | 9.0% | 9.4% | 10.5% | 11.0% | 11.4% | 11.5% |
Quarterly Growth Rate
Q1 2024 | Q2 2024 | Q3 2024 | Q4 2024 |
---|---|---|---|
10.8% | 11.1% | 11.3% | 11.5% |
Market Segments and Growth Drivers
Segment | Market Share | Growth Rate |
---|---|---|
Enterprise Software | 38% | 10.8% |
Cloud Services | 31% | 17.5% |
Developer Tools | 14% | 9.3% |
Security Software | 12% | 13.2% |
Other Software | 5% | 7.5% |
Technology Maturity Curve
Different technologies within the ecosystem are at varying stages of maturity:
Competitive Landscape Analysis
Company | Market Share |
---|---|
Microsoft | 22.6% |
Oracle | 14.8% |
SAP | 12.5% |
Salesforce | 9.7% |
Adobe | 8.3% |
Future Outlook and Predictions
The Regulation Navigating Post landscape is evolving rapidly, driven by technological advancements, changing threat vectors, and shifting business requirements. Based on current trends and expert analyses, we can anticipate several significant developments across different time horizons:
Year-by-Year Technology Evolution
Based on current trajectory and expert analyses, we can project the following development timeline:
Technology Maturity Curve
Different technologies within the ecosystem are at varying stages of maturity, influencing adoption timelines and investment priorities:
Innovation Trigger
- Generative AI for specialized domains
- Blockchain for supply chain verification
Peak of Inflated Expectations
- Digital twins for business processes
- Quantum-resistant cryptography
Trough of Disillusionment
- Consumer AR/VR applications
- General-purpose blockchain
Slope of Enlightenment
- AI-driven analytics
- Edge computing
Plateau of Productivity
- Cloud infrastructure
- Mobile applications
Technology Evolution Timeline
- Technology adoption accelerating across industries
- digital transformation initiatives becoming mainstream
- Significant transformation of business processes through advanced technologies
- new digital business models emerging
- Fundamental shifts in how technology integrates with business and society
- emergence of new technology paradigms
Expert Perspectives
Leading experts in the software dev sector provide diverse perspectives on how the landscape will evolve over the coming years:
"Technology transformation will continue to accelerate, creating both challenges and opportunities."
— Industry Expert
"Organizations must balance innovation with practical implementation to achieve meaningful results."
— Technology Analyst
"The most successful adopters will focus on business outcomes rather than technology for its own sake."
— Research Director
Areas of Expert Consensus
- Acceleration of Innovation: The pace of technological evolution will continue to increase
- Practical Integration: Focus will shift from proof-of-concept to operational deployment
- Human-Technology Partnership: Most effective implementations will optimize human-machine collaboration
- Regulatory Influence: Regulatory frameworks will increasingly shape technology development
Short-Term Outlook (1-2 Years)
In the immediate future, organizations will focus on implementing and optimizing currently available technologies to address pressing software dev challenges:
- Technology adoption accelerating across industries
- digital transformation initiatives becoming mainstream
These developments will be characterized by incremental improvements to existing frameworks rather than revolutionary changes, with emphasis on practical deployment and measurable outcomes.
Mid-Term Outlook (3-5 Years)
As technologies mature and organizations adapt, more substantial transformations will emerge in how security is approached and implemented:
- Significant transformation of business processes through advanced technologies
- new digital business models emerging
This period will see significant changes in security architecture and operational models, with increasing automation and integration between previously siloed security functions. Organizations will shift from reactive to proactive security postures.
Long-Term Outlook (5+ Years)
Looking further ahead, more fundamental shifts will reshape how cybersecurity is conceptualized and implemented across digital ecosystems:
- Fundamental shifts in how technology integrates with business and society
- emergence of new technology paradigms
These long-term developments will likely require significant technical breakthroughs, new regulatory frameworks, and evolution in how organizations approach security as a fundamental business function rather than a technical discipline.
Key Risk Factors and Uncertainties
Several critical factors could significantly impact the trajectory of software dev evolution:
Organizations should monitor these factors closely and develop contingency strategies to mitigate potential negative impacts on technology implementation timelines.
Alternative Future Scenarios
The evolution of technology can follow different paths depending on various factors including regulatory developments, investment trends, technological breakthroughs, and market adoption. We analyze three potential scenarios:
Optimistic Scenario
Rapid adoption of advanced technologies with significant business impact
Key Drivers: Supportive regulatory environment, significant research breakthroughs, strong market incentives, and rapid user adoption.
Probability: 25-30%
Base Case Scenario
Measured implementation with incremental improvements
Key Drivers: Balanced regulatory approach, steady technological progress, and selective implementation based on clear ROI.
Probability: 50-60%
Conservative Scenario
Technical and organizational barriers limiting effective adoption
Key Drivers: Restrictive regulations, technical limitations, implementation challenges, and risk-averse organizational cultures.
Probability: 15-20%
Scenario Comparison Matrix
Factor | Optimistic | Base Case | Conservative |
---|---|---|---|
Implementation Timeline | Accelerated | Steady | Delayed |
Market Adoption | Widespread | Selective | Limited |
Technology Evolution | Rapid | Progressive | Incremental |
Regulatory Environment | Supportive | Balanced | Restrictive |
Business Impact | Transformative | Significant | Modest |
Transformational Impact
Technology becoming increasingly embedded in all aspects of business operations. This evolution will necessitate significant changes in organizational structures, talent development, and strategic planning processes.
The convergence of multiple technological trends—including artificial intelligence, quantum computing, and ubiquitous connectivity—will create both unprecedented security challenges and innovative defensive capabilities.
Implementation Challenges
Technical complexity and organizational readiness remain key challenges. Organizations will need to develop comprehensive change management strategies to successfully navigate these transitions.
Regulatory uncertainty, particularly around emerging technologies like AI in security applications, will require flexible security architectures that can adapt to evolving compliance requirements.
Key Innovations to Watch
Artificial intelligence, distributed systems, and automation technologies leading innovation. Organizations should monitor these developments closely to maintain competitive advantages and effective security postures.
Strategic investments in research partnerships, technology pilots, and talent development will position forward-thinking organizations to leverage these innovations early in their development cycle.
Technical Glossary
Key technical terms and definitions to help understand the technologies discussed in this article.
Understanding the following technical concepts is essential for grasping the full implications of the security threats and defensive measures discussed in this article. These definitions provide context for both technical and non-technical readers.