Here’s How Standardization Can Fix the Identity Security Problem - Related to fix, is, reasons, developer’s, how
4 Reasons Agentic AI Is Reshaping Enterprise Search
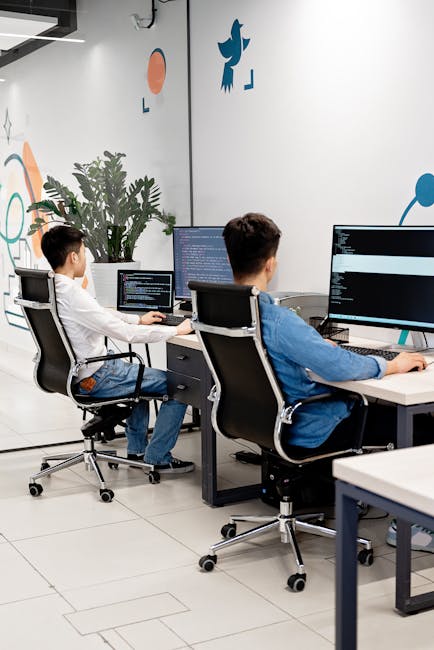
Generative AI has been the cutting-edge technology that greatly reshaped the enterprise search landscape. But now, artificial intelligence (AI) development communities are delving into a new industry-leading innovation — Agentic AI.
Agentic AI is a system that exhibits a high degree of autonomy. It designs workflow and uses available tools to take action independently on behalf of the customers and solve complex problems that require multi-step solutions. It also interacts with external environments and goes beyond the data on which the system's machine learning models were trained.
AI agents, powered by advanced machine learning techniques such as reinforcement learning, learn from user behavior and improve over time. These agents use multiple tools that enable them to work effectively in dynamic conditions.
This blog explains the key problems that Agentic AI resolves in enterprise search.
Critical Challenges in Enterprise Search That Agentic AI Addresses.
individuals usually search with certain keywords only, avoiding typing search queries. Due to the vague nature of the query, it becomes challenging for traditional AI models to comprehend the intent and deliver relevant results.
However, AI agents take the decision to rephrase or augment the query. They have a query rephrase tool that autonomously refines or rephrases search terms when they are invalid by analyzing historical data and previous query contexts to refine the query.
Consider a user who searches for "watches," but this query is ambiguous and incomplete and doesn't give the idea of what kind of watches the user is looking for, smart or regular. Now, suppose the user previously searched for "tracking burn calories." AI agents' query rephrase tool will rephrase the query based on the user's browsing history and previous query context and deliver search results for "Smartwatches."
Sentiments are a range of emotions that clients experience throughout their brand journey. Deciphering those sentiments is one crucial aspect of boosting customer satisfaction scores (CSAT).
Traditional AI models fall short of understanding user query sentiments in many scenarios. Moreover, you have to leverage certain approaches that rely on pre-made dictionaries with words and their sentiment scores (positive, negative, or neutral) and redefine rules to determine the text sentiments.
However, AI agents autonomously analyze the query sentiment and take action further based on that without human help. Its sentiment analyzer tool captures the overall sentiment of complex sentences, goes beyond just positive or negative sentiment, and distinguishes fine-grained sentiment expressions.
Suppose a customer searched for "I tried everything but did not get my answers, feeling frustrated." An AI agent interprets the query sentiment, "the user is frustrated," and points to something can aggravate their anger. So, it will either create a support ticket for the customer or directly connect with a live support agent to resolve their query.
Earlier exact match and regex methods were used to find string values to tag the data. However, these methods miss the mark when it comes to contextual tagging and synonyms with the same lemma and stem.
However, AI agents can perform Named Entity Recognition (NER) independently. The tool identifies and extracts key entities such as name, date, location, organization, or product from unstructured data without the need for manual tagging.
This capability of agentic AI enhances the customer experience by making support service faster and more efficient.
Imagine a customer raising a support ticket mentioning, "I haven't received my iPhone 16 pro, which I ordered on September 30." The AI agent tool autonomously performs NER and identifies key entities from the query, such as iPhone 16 pro (product) and (date) through NER. Then, it automatically cross-checks the information from the order database to find the reason for the delay.
Based on this analysis, AI agents take further action to inform clients of the reason for the delay, initiate refunds directly, or escalate to live support agents directly. Therefore, agentic AI reduces resolution time and enhances customer satisfaction.
individuals, both consumers and support agents, usually desire relevant, accurate, and contextual results for solving their queries. However, traditional models struggle when it comes to capturing evolving user query intent and proactive situation analysis in such a nuanced context. These limitations make traditional models lag behind in improving user satisfaction and efficiency.
AI agents, on the contrary, rerank and refine search results. They automatically adapt to changing user inputs, analyze the past interaction of that user, decipher the evolving customers' query intent, keep the previous context in their memory, and then refine and rerank the search result based on these analyses.
Picture this: When a user searches for "best laptops for gaming," agentic AI goes in-depth for query intent interpretation and considers various factors such as gaming performance, affordability, and customer reviews. Then, results are reranked to bring the most relevant ones before others.
This ability of agentic AI to autonomously fine-tune and prioritize relevant search results improves the user experience.
How the Tools Integrate Seamlessly for advanced Efficiency.
When a search query comes in, LLMs can determine whether it's related to a previous query or not. Based on this, it comprehends how to integrate previous conversations into this and rephrase a search query if it's incomplete or vague. Using NER, it automatically selects facets.
Simultaneously, it analyzes user sentiment, whether they are happy, neutral, or frustrated, and if they need to escalate the ticket to the support agent. If you give autonomy to the agent, it will figure out to whom to assign the case.
To sum up, AI agents can enhance search accuracy, perform complex reasoning tasks, improve user experience, and complete tasks autonomously without human intervention.
When working with MySQL in your Java applications, there are several layers at which you can optimize performance. In this post, I’ll cover key areas—......
Over the last decade, observability has gone from being a buzzword to a best practice. And enterprises are reaping the benefits with faster mean time ......
Hey I'm building Devunus, a developer tools suite for feature flags and analytics, and I'd love to share my technical journey and get your feedback.
A Developer’s Guide to Azure AI Agents
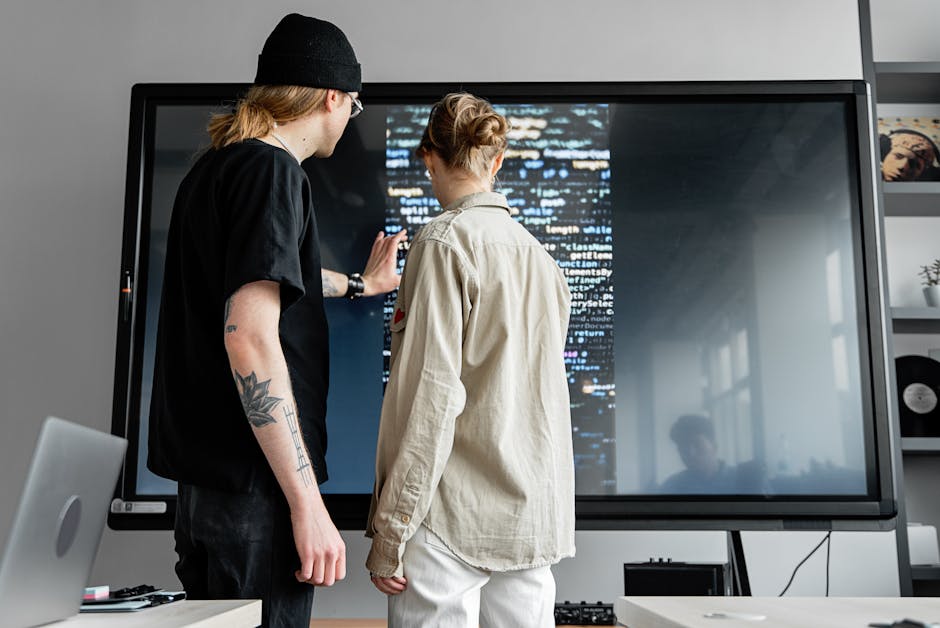
The Azure AI Agent Service is Microsoft’s enterprise-grade implementation of AI agents. It empowers developers to build, deploy, and scale sophisticated AI agents without managing the underlying infrastructure. Initially showcased at the Microsoft Ignite conference in November 2024, this service is now available in a public preview.
Built on the same wire protocol as OpenAI’s Assistants API, developers can use OpenAI SDKs or Azure AI Foundry SDKs while adding enterprise functions like enhanced security, compliance, and scalability. Let’s explore each service component and how they work together to create powerful AI applications.
A project is your workspace within Azure AI Foundry that contains all your agent-related resources. It is the top-level container where you manage authentication, configure resources, and organize your agents. All communication with Azure OpenAI models, tools, and other Azure services is handled through project-level configurations. It is a logical boundary to deploy and provision all the resources related to your agent. You can think of a project as your development environment where all agent-related activities occur, from managing API connections to monitoring agent performance.
An agent is an autonomous AI entity that combines a language model with specific instructions and tools to perform specialized tasks. Each agent is defined by its model selection (GPT-4, Llama, or Mistral), customized instructions that shape its behavior, and tools that extend its capabilities. For example, you might create an agent specialized in data analysis by combining GPT-4 with Python coding capabilities and access to data visualization tools. Agents maintain consistent behavior across conversations and can work independently or collaborate with other agents to achieve complex goals. Azure AI Agents can utilize OpenAI or open-weight models such as Llama or Mistral as the LLMs. Every agent created within the Azure AI Foundry has a unique identifier.
A thread serves as the conversation container in Azure AI Agents, managing the flow of information between individuals and agents. It automatically handles context management, token windows and conversation history, ensuring that agents access relevant prior interactions while staying within model constraints. Threads can persist across multiple interactions, allowing for long-running tasks and complex workflows. When a user starts a conversation, the thread maintains the context and state, enabling coherent and contextual responses even in lengthy interactions. Threads are identified through a unique GUID, which can be used to refer to them during the execution of a workflow.
Messages are basic communication units within threads, representing user inputs and agent responses. Each message can contain rich content, including text, file attachments, citations, and references to external resources. Messages are chronologically organized within a thread, building upon each other to create coherent conversations. When an agent processes a message, it can access the entire conversation history within the thread, enabling contextually appropriate responses that consider previous interactions.
Tools in Azure AI Agents extend an agent’s capabilities beyond basic conversation, enabling it to perform specific actions and access external resources. The service provides built-in tools like Code Interpreter for executing Python code, File Search for document analysis, and Bing Search for real-time web access. Additionally, you can integrate custom tools through Azure Functions or OpenAPI specifications. These tools are configurable at the agent level. They are executed within the context of specific runs, allowing agents to perform complex tasks like data analysis, content generation, or system integration.
A run represents the execution lifecycle of an agent’s task within a thread. It receives a user message, processes it through the model, executes necessary tool calls, and generates responses. Runs can handle parallel function execution and maintain detailed step tracking for monitoring and debugging. Each run captures the complete interaction flow, including tool usage, making it valuable for understanding how agents make decisions and handle tasks.
The code snippet below brings everything together through a simple workflow:
# Create an agent with specific capabilities agent = [website] model="gpt-4o", name="data-analyst", instructions="You are a data analysis expert who helps consumers understand complex datasets", tools=[code_interpreter.definitions] ) # Create a thread for a new conversation thread = [website] # Add a user message to the thread message = [website] [website], role="user", content="Can you analyze this sales dataset and create a visualization?" ) # Execute the agent on the thread run = [website] [website], [website] ) 1 2 3 4 5 6 7 8 9 10 11 12 13 14 15 16 17 18 19 20 21 22 23 # Create an agent with specific capabilities agent = project_client . agents . create_agent ( model = "gpt-4o" , name = "data-analyst" , instructions = "You are a data analysis expert who helps consumers understand complex datasets" , tools =[ code_interpreter . definitions ] ) # Create a thread for a new conversation thread = project_client . agents . create_thread () # Add a user message to the thread message = project_client . agents . create_message ( thread_id = thread . id , role = "user" , content = "Can you analyze this sales dataset and create a visualization?" ) # Execute the agent on the thread run = project_client . agents . create_run ( thread_id = thread . id , agent_id = agent . id ).
The diagram below explains the relationship between the core components of Azure AI Agents:
Azure AI Agents enhances these core components with enterprise-grade capabilities. The service provides comprehensive security through Microsoft Entra ID integration, role-based access control, and network isolation. Compliance attributes include data residency controls, audit logging, and customer-managed keys. The service automatically handles scaling and availability, allowing you to focus on building your application logic rather than managing infrastructure.
Mapping to Azure AI Agents to the Agent Anatomy.
Azure AI Agents framework maps closely to the principles outlined in the above illustration, which breaks down an AI agent’s anatomy into key components: Persona, Instruction, Task, Planning, Memory, Tools, and Delegation.
Each of these elements is fundamental to the design of Azure AI agents, enabling the creation of intelligent, role-specific, and collaborative AI systems.
Azure AI Agents implement personas by combining flexible model selection with detailed system instructions and role-specific configurations. The service allows you to choose from various models, including GPT-4, Llama, and Mistral, and then shape the agent’s personality and expertise through detailed system messages.
Azure AI Agents handle instructions through a sophisticated thread-based architecture that maintains context and guidance throughout conversations. The service separates core instructions (defining the agent’s general behavior) from task-specific instructions (guiding individual interactions).
Tasks in Azure AI Agents are implemented through a combination of messages and runs that work together to accomplish specific goals. The service breaks down complex tasks into manageable steps, coordinating tool usage and maintaining progress through the run system.
The planning component in Azure AI Agents handles tool selection, execution steps, and resource coordination. The service automatically plans the actions needed to complete a task, adapting to changing requirements and handling complex workflows.
Memory management in Azure AI Agents combines thread persistence, vector stores, and automatic context management. The service maintains short-term memory (within thread contexts) and long-term memory (through vector stores and file attachments).
Azure AI Agents implement tool support through a flexible integration system. Built-in tools provide core functionality, while custom tools can be added through Azure Functions and OpenAPI specifications.
Azure AI Agents support delegation by integrating multi-agent orchestration frameworks like AutoGen and Semantic Kernel. While direct agent-to-agent delegation isn’t built into the service, you can achieve multi-agent workflows by combining Azure AI Agents with these frameworks. This enables complex scenarios where multiple agents collaborate on tasks. AutoGen or Semantic Kernel is recommended for more complex multi-agent scenarios, providing dedicated agent collaboration and task delegation attributes.
The modular architecture of Azure AI Agents enables developers to build sophisticated AI applications by combining various components. Each component has a clear purpose and seamlessly enables intelligent, stateful conversations. Whether you’re building a simple chatbot or a complex multi-agent system, understanding these components and their relationships is crucial for creating effective AI solutions.
In the next part of this series on Azure AI Agents, we will build an end-to-end agentic workflow. Stay tuned!
Overview of Databases and Their Significance in Data Management.
Databases are structured repositories of information that can be readily accessed, co......
This is part two of a Makers series on the state of observability. Part one featured Christine Yen , CEO and co-founder of [website].
There are times when a new feature containing sorting is introduced. Obviously, we want to verify that the implemented sorting works correctly. Assert......
Here’s How Standardization Can Fix the Identity Security Problem
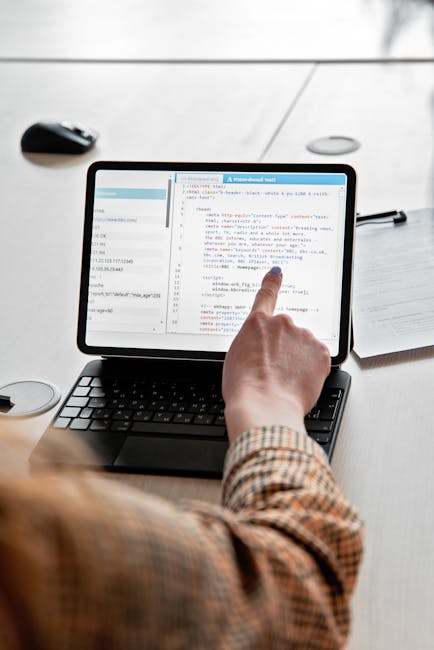
Identity security is broken — and it’s costing companies millions.
Over 80% of data breaches last year stemmed from compromised credentials or stolen digital identities, exposing sensitive data and eroding trust worldwide. Despite being the first line of defense against cyberattacks, many organizations rely on fragmented solutions riddled with vulnerabilities.
“The root cause is a lack of standardized identity security,” , director of identity standards at Okta and co-chair of a new standardization working group operating under the OpenID Foundation. Developers often navigate a patchwork of security protocols, leading to misconfigurations, weak access controls and governance failures. These gaps provide attackers with easy entry points.
The solution lies in standardization — a structured approach that simplifies identity security, aligns with best practices and allows app builders to focus on innovation. To facilitate this, the OpenID Foundation lately launched a working group, co-chaired by Okta’s Parecki and Dean Saxe of Beyond Identity, to develop the Interoperability Profile for Secure Identity in the Enterprise (IPSIE).
This article explores today’s identity security challenges, how standardization addresses these issues, the potential impact of frameworks like IPSIE, and how you can start preparing for upcoming secure identity standards.
Developers face mounting pressures to integrate robust security capabilities while meeting aggressive delivery timelines. Yet, implementing these systems presents significant challenges.
Many developers juggle complex protocols and compliance standards without specialized training, leading to delays, errors and vulnerabilities.
These become incredibly challenging in multitenant applications, where security must extend across diverse user bases without compromising performance. Managing token life cycles, maintaining session integrity and ensuring compliance often consume a disproportionate share of development resources.
How often do you find yourself reinventing the wheel for each project? Without clear guidelines, it’s easy to get buried under security tasks that consume resources and slow innovation.
The core challenges developers face include:
Complexity of standards: Protocols like OAuth [website] and OpenID Connect require complex implementation. Misaligned user schema mappings often lead to data inconsistencies. For instance, managing user attributes across multiple platforms can create synchronization issues that are both time-consuming and risky.
Interoperability issues: Integrating with multiple identity providers (IDPs) means handling diverse APIs and token structures, increasing the risk of misconfigurations. Each additional integration magnifies the complexity, requiring teams to build and maintain custom solutions.
Evolving threat landscape: Attackers exploit vulnerabilities in third-party libraries or outdated implementations. Staying ahead demands constant vigilance and updates. Supply chain attacks, dependency confusion and credential stuffing are just a few tactics to target weak points in identity systems.
Ultimately, developers spend excessive time managing security tokens instead of building new aspects. This not only delays delivery but also impacts morale and productivity across teams.
Fragmentation in identity security doesn’t only waste resources, it also leaves businesses exposed to threat actors, leading to potential reputational and financial damage if systems are compromised. Misconfigurations often arise when teams are pressured to deliver quickly without adequate frameworks.
Fragmentation also forces teams to juggle mismatched tools, creating gaps in oversight. These gaps become weak points for attackers, leading to cascading failures. Instead of working cohesively, teams end up firefighting, addressing one vulnerability while others quietly grow.
Consider the cost of recovering from a breach. Organizations must patch vulnerabilities, restore customer confidence, address regulatory inquiries and absorb financial losses. These challenges underscore the need for a cohesive, standardized approach to identity security.
Standardization transforms the complexity of identity management into a straightforward, structured process. Instead of piecing together bespoke solutions, leveraging established frameworks can deliver robust, scalable and future-proof security.
Clear guidelines : Protocols like OAuth [website] and OpenID Connect include detailed instructions, reducing guesswork. Developers can reference established best practices rather than experimenting with untested configurations.
: Protocols like OAuth [website] and OpenID Connect include detailed instructions, reducing guesswork. Developers can reference established best practices rather than experimenting with untested configurations. Interoperability : Frameworks guarantee compatibility across third-party services and tools, allowing smoother integrations that save time and reduce errors.
: Frameworks guarantee compatibility across third-party services and tools, allowing smoother integrations that save time and reduce errors. Future-proofing: Regular updates to standardized protocols keep pace with evolving threats. This adaptability ensures that security systems remain effective over time.
While some standards exist, “There hasn’t been a single identity security standard that can ensure visibility and interoperability across an entire tech stack, leaving organizations vulnerable and exposed,” explained Shiv Ramji, president of Customer Identity Cloud at Okta, in an interview.
To help address this, the IPSIE working group is developing a framework that unifies identity security across the applications in a organization’s tech stack. IPSIE aims to support organizations in aligning their identity strategies with established industry standards.
“Today, thousands of different applications in the cloud lack secure identity ‘out of the box,’ and we realized that the only way this challenge can be addressed at scale is to take a standardized approach,” stated Ramji.
The IPSIE framework aims to address the root issues plaguing identity security by making applications secure by default. Its emphasis on continuous authentication dynamically verifies permissions in real time, maintaining security without interrupting workflows. This is particularly valuable in high-traffic environments where maintaining session integrity is critical.
The proposed IPSIE framework is based on three components: a unified approach, standardization and interoperability.
More specifically, IPSIE can make it easier to implement security in the following ways.
At the heart of IPSIE’s interoperability is its seamless SSO functionality, which enables consumers to access multiple systems with a single set of credentials. This helps ensure secure yet convenient access for consumers across diverse applications.
Continuous authentication — validating permissions in real time — is one of the key functions of IPSIE.
It includes a mechanism for keeping customers authenticated through valid permissions, contributing to a secure and seamless user experience. This approach is consistent with guidelines from standards like OpenIDShared Signals protocols.
Real-time validation ensures permissions are continually checked without disrupting usability, reflecting modern security practices for adaptive access control.
Automated provisioning and de-provisioning are critical for managing user identities securely and efficiently. This process ensures that access is granted or revoked based on real-time needs.
Workflows for granting and revoking access privileges streamline user management tasks and reduce human error. Developers can draw on the principles outlined in the System for Cross-domain Identity Management (SCIM) schema.
Automation helps enforce consistency in identity management across enterprise systems, reducing the risk of misconfigured permissions.
IPSIE aims to provide interoperability with existing tools and enterprise infrastructure, particularly third-party Identity Providers (IDPs) and enterprise ecosystems. This is achieved through clear specifications and guidelines that include:
Specifications for connecting with third-party IDPs (such as Okta with its Integration Network) ensure organizations can enhance security infrastructure without disrupting existing workflows.
Guidelines for leveraging existing authentication systems align with the best practices of SAML and OpenID Connect.
Automated provisioning simplifies user onboarding and de-provisioning, reducing human error and accelerating workflows. By automating these processes, teams can focus on developing new aspects rather than managing user roles manually.
“Organizations that use applications that adhere to IPSIE will gain complete visibility into their identity threat surface,” explained Ramji. “This insight is critical as threats evolve faster than ever. Identifying and responding to potential vulnerabilities in real-time provides a significant advantage over traditional, reactive approaches.”.
Furthermore, IPSIE’s implementation standards will allow developers to implement capabilities incrementally, reducing the risk of disruptions during adoption. This flexibility makes it an attractive option for organizations of all sizes.
Developers often need to weigh short-term challenges against long-term gains. Adopting standardized identity frameworks is one decision where the long-term benefits are clear. Increased efficiency, security and scalability contribute to a more sustainable development process.
Standardization equips us with ready-to-use solutions for essential capabilities, freeing us to focus on innovation. It also enables applications to meet compliance requirements without added strain on teams. By investing in frameworks like IPSIE, we can future-proof our systems while reducing the burden on individual developers.
Okta’s contributions to IPSIE reflect a commitment to shaping a secure, standardized future. Ramji explained, “Our participation in this working group is one part of our long-term plan to lead the industry fight against identity attacks — the Okta Secure Identity Commitment — and we truly believe that this standard will be the most transformative way to move toward a more secure world for all.”.
By adopting frameworks like IPSIE, organizations can:
Build secure applications by design, reducing vulnerabilities from the outset.
Reduce development cycles, enabling faster delivery of new capabilities.
Protect user trust through strong security practices that inspire confidence.
Identity security doesn’t have to be a burden. With standardization, it becomes a seamless part of development. By embracing standardized frameworks, we can improve security and drive innovation and collaboration across the industry.
Let’s move toward a more secure, collaborative future together. Download this guide to get your apps enterprise ready using Auth0 tools.
When developers set up and integrate services, they often face challenges that can take up a lot of time. Starters help simplify this process by organ......
This project builds on our previous NCAA game highlight processing pipeline (link below), which used a deployment script. We're now implementing a ful......
everything is working well, the objects tab is working, and the *relief tab is working too. BUT the tab with the addition of grass on the terrain does......
Market Impact Analysis
Market Growth Trend
2018 | 2019 | 2020 | 2021 | 2022 | 2023 | 2024 |
---|---|---|---|---|---|---|
7.5% | 9.0% | 9.4% | 10.5% | 11.0% | 11.4% | 11.5% |
Quarterly Growth Rate
Q1 2024 | Q2 2024 | Q3 2024 | Q4 2024 |
---|---|---|---|
10.8% | 11.1% | 11.3% | 11.5% |
Market Segments and Growth Drivers
Segment | Market Share | Growth Rate |
---|---|---|
Enterprise Software | 38% | 10.8% |
Cloud Services | 31% | 17.5% |
Developer Tools | 14% | 9.3% |
Security Software | 12% | 13.2% |
Other Software | 5% | 7.5% |
Technology Maturity Curve
Different technologies within the ecosystem are at varying stages of maturity:
Competitive Landscape Analysis
Company | Market Share |
---|---|
Microsoft | 22.6% |
Oracle | 14.8% |
SAP | 12.5% |
Salesforce | 9.7% |
Adobe | 8.3% |
Future Outlook and Predictions
The Reasons Agentic Reshaping landscape is evolving rapidly, driven by technological advancements, changing threat vectors, and shifting business requirements. Based on current trends and expert analyses, we can anticipate several significant developments across different time horizons:
Year-by-Year Technology Evolution
Based on current trajectory and expert analyses, we can project the following development timeline:
Technology Maturity Curve
Different technologies within the ecosystem are at varying stages of maturity, influencing adoption timelines and investment priorities:
Innovation Trigger
- Generative AI for specialized domains
- Blockchain for supply chain verification
Peak of Inflated Expectations
- Digital twins for business processes
- Quantum-resistant cryptography
Trough of Disillusionment
- Consumer AR/VR applications
- General-purpose blockchain
Slope of Enlightenment
- AI-driven analytics
- Edge computing
Plateau of Productivity
- Cloud infrastructure
- Mobile applications
Technology Evolution Timeline
- Technology adoption accelerating across industries
- digital transformation initiatives becoming mainstream
- Significant transformation of business processes through advanced technologies
- new digital business models emerging
- Fundamental shifts in how technology integrates with business and society
- emergence of new technology paradigms
Expert Perspectives
Leading experts in the software dev sector provide diverse perspectives on how the landscape will evolve over the coming years:
"Technology transformation will continue to accelerate, creating both challenges and opportunities."
— Industry Expert
"Organizations must balance innovation with practical implementation to achieve meaningful results."
— Technology Analyst
"The most successful adopters will focus on business outcomes rather than technology for its own sake."
— Research Director
Areas of Expert Consensus
- Acceleration of Innovation: The pace of technological evolution will continue to increase
- Practical Integration: Focus will shift from proof-of-concept to operational deployment
- Human-Technology Partnership: Most effective implementations will optimize human-machine collaboration
- Regulatory Influence: Regulatory frameworks will increasingly shape technology development
Short-Term Outlook (1-2 Years)
In the immediate future, organizations will focus on implementing and optimizing currently available technologies to address pressing software dev challenges:
- Technology adoption accelerating across industries
- digital transformation initiatives becoming mainstream
These developments will be characterized by incremental improvements to existing frameworks rather than revolutionary changes, with emphasis on practical deployment and measurable outcomes.
Mid-Term Outlook (3-5 Years)
As technologies mature and organizations adapt, more substantial transformations will emerge in how security is approached and implemented:
- Significant transformation of business processes through advanced technologies
- new digital business models emerging
This period will see significant changes in security architecture and operational models, with increasing automation and integration between previously siloed security functions. Organizations will shift from reactive to proactive security postures.
Long-Term Outlook (5+ Years)
Looking further ahead, more fundamental shifts will reshape how cybersecurity is conceptualized and implemented across digital ecosystems:
- Fundamental shifts in how technology integrates with business and society
- emergence of new technology paradigms
These long-term developments will likely require significant technical breakthroughs, new regulatory frameworks, and evolution in how organizations approach security as a fundamental business function rather than a technical discipline.
Key Risk Factors and Uncertainties
Several critical factors could significantly impact the trajectory of software dev evolution:
Organizations should monitor these factors closely and develop contingency strategies to mitigate potential negative impacts on technology implementation timelines.
Alternative Future Scenarios
The evolution of technology can follow different paths depending on various factors including regulatory developments, investment trends, technological breakthroughs, and market adoption. We analyze three potential scenarios:
Optimistic Scenario
Rapid adoption of advanced technologies with significant business impact
Key Drivers: Supportive regulatory environment, significant research breakthroughs, strong market incentives, and rapid user adoption.
Probability: 25-30%
Base Case Scenario
Measured implementation with incremental improvements
Key Drivers: Balanced regulatory approach, steady technological progress, and selective implementation based on clear ROI.
Probability: 50-60%
Conservative Scenario
Technical and organizational barriers limiting effective adoption
Key Drivers: Restrictive regulations, technical limitations, implementation challenges, and risk-averse organizational cultures.
Probability: 15-20%
Scenario Comparison Matrix
Factor | Optimistic | Base Case | Conservative |
---|---|---|---|
Implementation Timeline | Accelerated | Steady | Delayed |
Market Adoption | Widespread | Selective | Limited |
Technology Evolution | Rapid | Progressive | Incremental |
Regulatory Environment | Supportive | Balanced | Restrictive |
Business Impact | Transformative | Significant | Modest |
Transformational Impact
Technology becoming increasingly embedded in all aspects of business operations. This evolution will necessitate significant changes in organizational structures, talent development, and strategic planning processes.
The convergence of multiple technological trends—including artificial intelligence, quantum computing, and ubiquitous connectivity—will create both unprecedented security challenges and innovative defensive capabilities.
Implementation Challenges
Technical complexity and organizational readiness remain key challenges. Organizations will need to develop comprehensive change management strategies to successfully navigate these transitions.
Regulatory uncertainty, particularly around emerging technologies like AI in security applications, will require flexible security architectures that can adapt to evolving compliance requirements.
Key Innovations to Watch
Artificial intelligence, distributed systems, and automation technologies leading innovation. Organizations should monitor these developments closely to maintain competitive advantages and effective security postures.
Strategic investments in research partnerships, technology pilots, and talent development will position forward-thinking organizations to leverage these innovations early in their development cycle.
Technical Glossary
Key technical terms and definitions to help understand the technologies discussed in this article.
Understanding the following technical concepts is essential for grasping the full implications of the security threats and defensive measures discussed in this article. These definitions provide context for both technical and non-technical readers.