Podcast: Resilience, Observability and Unintended Consequences of Automation - Related to psychological, podcast:, a, unintended, safety
Load balancing Cypress tests without Cypress Cloud
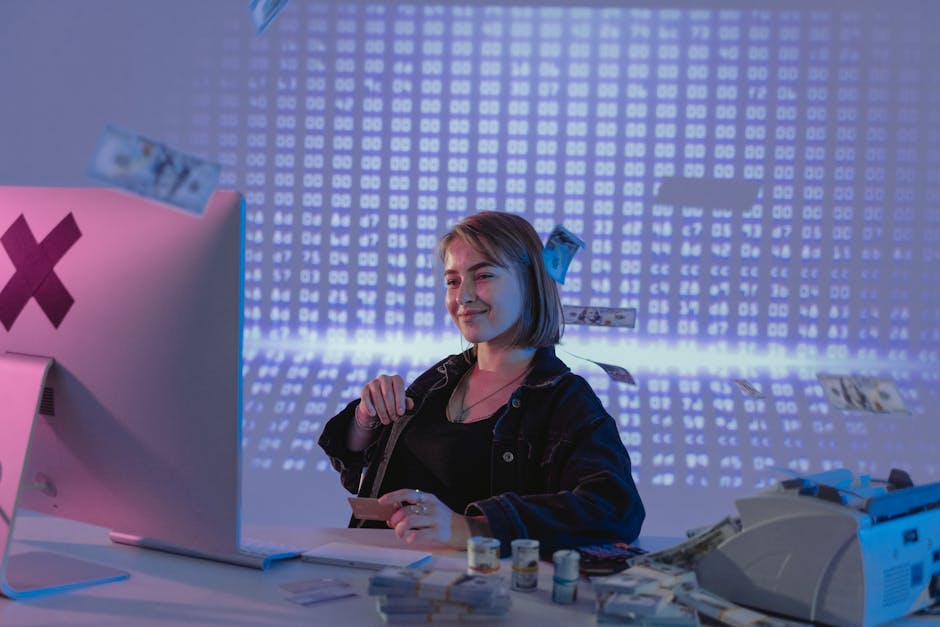
lately I've been asked to work on a solution of efficiently running Cypress component tests on pull requests without taking a lot of time. At first, my standing solution was to just evenly spread out the files against a number of parallel jobs on GitHub Actions workflows, but there is a big discrepancy between the slowest job and the average job times. Thus, we've been wondering if there is a smarter way of evening out the runtimes.
With that, I created a new plugin of cypress-load-balancer, which allows us to solve that problem. This plugin saves the durations of the tests it runs and calculates an average, which then then can be passed into a script; that script uses an algorithm to perform load balancing for a number of job runners.
In computing, load balancing is the process of distributing a set of tasks over a set of resources (computing units), with the aim of making their overall processing more efficient. Load balancing can optimize response time and avoid unevenly overloading some compute nodes while other compute nodes are left idle.
The general approach of using a load balancer for tests.
This is the basic idea of steps that need to occur to utilize results from load balancing properly. A persistent load balancing map file known as [website] is saved on the host machine. The load balancer will reference that file and perform calculations to assign tests across a given number of runners. After all parallel test jobs complete, they will create a key-value list of test file names to their execution time; these results can then be merged back to the main spec map file, recalculate a new average duration per each test file, and then overwrite the original file on the host machine. Then the spec map can be consumed on the next test runs, and run through this process all over and over again.
For this tool, here are the general steps:
Install and configure the plugin in the Cypress config. When Cypress runs, it will be able to locally save the results of the spec executions per each runner, depending on e2e or component tests. Initialize the load balancer main map file in a persisted location that can be easily restored from cache. This means the main file needs to be in a place outside of the parallelized jobs to can be referenced by the parallelized jobs in order to save new results. Execute the load balancer against a number of runners. The output is able to be used for all parallelized jobs to instruct them which specs to execute. Execute each parallelized job that starts the Cypress testrunner with the list of spec files to run across each runner. When the parallelized jobs complete, collect and save the output of the load balancing files from each job in a temporary location. After all parallelized test jobs complete, merge their load balancing map results back to the persisted map file and cached for later usage. This is where the persisted file on the host machine gets overwritten with new results to improved perform on the next runs. (In a GitHub Actions run, this means on pull request merge, the load balancing files from the base branch and the head branch need to be merged, then cached down to the base branch.).
So, for Docker Compose, a persistent volume needs to exist for the host [website] to be saved. It can then run the load balancing script, and execute a number of parallelized containers to run those separated Cypress tests. When each test job completes, the duration of each test can be merged back to the original file and re-calculate a new average.
For GitHub Actions, it's a bit more complex. More on that later.
How does it work for Cypress automated tests?
The current installation guide as of February 2025 is as such:
Install the package to your project: npm install --save-dev cypress-load-balancer yarn add -D cypress-load-balancer Add the following to your .gitignore and other ignore files: .cypress_load_balancer In your Cypress configuration file, add the plugin separately to your e2e configuration and also component.
This will register load balancing for separate testing types import { addCypressLoadBalancerPlugin } from " cypress-load-balancer " ; defineConfig ({ e2e : { setupNodeEvents ( on , config ) { addCypressLoadBalancerPlugin ( on ); } }, component : { setupNodeEvents ( on , config ) { addCypressLoadBalancerPlugin ( on ); } } });
Cypress tests are run for e2e or component testing types.
When the run completes, the durations and averages of all executed tests are added to [website] .
. The [website] can now be used by the included executable, cypress-load-balancer , to perform load balancing against the current Cypress configuration and tests that were executed. The tests are sorted from slowest to fastest and then assigned out per runner to get them as precise as possible to each other in terms of execution time. For example, with 3 runners and e2e tests: npx cypress-load-balancer --runners 3 --testing-type e2e.
can now be used by the included executable, , to perform load balancing against the current Cypress configuration and tests that were executed. The tests are sorted from slowest to fastest and then assigned out per runner to get them as precise as possible to each other in terms of execution time. For example, with 3 runners and e2e tests: The script will output an array of arrays of spec files balanced across 3 runners.
There are included scripts with npx cypress-load-balancer :
shell $: npx cypress-load-balancer --help cypress-load-balancer Performs load balancing against a set of runners and Cypress specs Commands: cypress-load-balancer Performs load balancing against a set of runners and Cypress specs [default] cypress-load-balancer initialize Initializes the load balancing map file and directory. cypress-load-balancer merge Merges load balancing map files together back to an original map. Options: --version Show version number [boolean] -r, --runners The count of executable runners to use [number] [required] -t, --testing-type The testing type to use for load balancing [string] [required] [choices: "e2e", "component"] -F, --files An array of file paths relative to the current working directory to use for load balancing. Overrides finding Cypress specs by configuration file. If left empty, it will utilize a Cypress configuration file to find test files to use for load balancing. The Cypress configuration file is implied to exist at the base of the directory unless set by "[website]" [array] [default: []] --format, --fm Transforms the output of the runner jobs into various formats. "--transform spec": Converts the output of the load balancer to be as an array of "--spec {file}" formats "--transform string": Spec files per runner are joined with a comma; example: "tests/[website],tests/[website]" "--transform newline": Spec files per runner are joined with a newline; example: "tests/[website] tests/[website]" [choices: "spec", "string", "newline"] --set-gha-output, --gha Sets the output to the GitHub Actions step output as "cypressLoadBalancerSpecs" [boolean] -h, --help Show help [boolean] Examples: Load balancing for 6 runners against cypressLoadBalancer -r 6 -t "component" testing with implied Cypress component configuration of `./[website]` Load balancing for 3 runners against cypressLoadBalancer -r 3 -t e2e -F "e2e" testing with specified file paths cypress/e2e/[website] cypress/e2e/[website] cypress/e2e/[website] Enter fullscreen mode Exit fullscreen mode.
I included two workflows in the package that show how this can work for tests executed on pull requests.
get-specs: A cached load balancing map is attempted to be restored. It tries for the current target branch, then for the source branch, and if none can be found, it initializes a basic map of the files to be run. Load balancing is performed based on the user's input of the number of jobs to use. It outputs an array of specs for each runner.
cypress_run_e2e: These are the parallelized jobs that run a subset of the files obtained from the load balancer output. When this job completes, it produces a temporary [website] of just those files, and uploads the artifact.
merge_cypress_load_balancing_maps: After all parallel jobs complete, download their artifacts of their temporary [website] files, merge them to the branch's map file, and then cache and upload it. This is how it can be saved per branch.
yml name: Testing load balancing Cypress E2E tests on: pull_request: workflow_dispatch: inputs: runners: type: number description: Number of runners to use for parallelization required: false default: 3 debug: type: boolean description: Enables debugging on the job and on the cypress-load-balancer script. env: runners: ${{ inputs.runners || 3}} CYPRESS_LOAD_BALANCER_DEBUG: ${{ [website] || false }} jobs: get_specs: runs-on: [website] outputs: e2e_specs: ${{ [website] }} steps: - name: Checkout uses: actions/checkout@v4 - uses: actions/setup-node@v4 with: node-version: ${{ env.NODE_VERSION }} - run: | yarn install yarn build - name: Get cached load-balancing map id: cache-restore-load-balancing-map uses: actions/cache/restore@v4 with: fail-on-cache-miss: false path: .cypress_load_balancer/[website] key: cypress-load-balancer-map-${{ github.head_ref || github.ref_name }}-${{ github.run_id }}-${{ github.run_attempt }} # Restore keys: ## 1. Same key from previous workflow run ## 2. Key from pull request base branch most recent workflow. Used for the "base" map, if one exists restore-keys: | cypress-load-balancer-map-${{github.head_ref || github.ref_name }}-${{ github.run_id }}-${{ github.run_attempt }} cypress-load-balancer-map-${{github.head_ref || github.ref_name }}-${{ github.run_id }}- cypress-load-balancer-map-${{github.head_ref || github.ref_name }}- cypress-load-balancer-map-${{ github.base_ref }}- - name: Perform load balancing for E2E tests id: e2e-cypress-load-balancer #TODO: this can eventually be replaced with a GitHub action. The executable should be used for Docker and other CI/CD tools run: npx cypress-load-balancer -r ${{ env.runners }} -t e2e --fm string --gha #run: echo "specs=$(echo $(npx cypress-load-balancer -r ${{ env.runners }} -t e2e --fm string | tail -1))" >> $GITHUB_OUTPUT - name: "DEBUG: read restored cached [website] file" if: ${{ env.CYPRESS_LOAD_BALANCER_DEBUG == 'true' }} run: cat .cypress_load_balancer/[website] cypress_run_e2e: runs-on: [website] needs: get_specs strategy: fail-fast: false matrix: spec: ${{ fromJson(needs.get_specs.outputs.e2e_specs) }} steps: - name: Generate uuid to use uploading a unique load balancer map artifact id: generate-uuid run: echo uuid="$(uuidgen)" >> $GITHUB_OUTPUT - uses: actions/checkout@v4 - uses: actions/setup-node@v4 with: node-version: ${{ env.NODE_VERSION }} - name: Cypress run e2e tests uses: cypress-io/github-action@v6 with: browser: electron build: yarn build spec: ${{ [website] }} # Fix for [website] config: videosFolder=/tmp/cypress-videos - name: Upload temp load balancer map if: always() uses: actions/upload-artifact@v4 with: name: ${{[website] }}-cypress-load-balancer-map-temp-from-parallel-job path: .cypress_load_balancer/[website] merge_cypress_load_balancing_maps: runs-on: [website] needs: [get_specs, cypress_run_e2e] if: ${{ [website] == 'success' }} steps: - name: Checkout uses: actions/checkout@v4 - uses: actions/setup-node@v4 with: node-version: ${{ env.NODE_VERSION }} - run: | yarn install yarn build - name: Get cached load-balancing map id: cache-restore-load-balancing-map uses: actions/cache/restore@v4 with: fail-on-cache-miss: false path: .cypress_load_balancer/[website] key: cypress-load-balancer-map-${{ github.head_ref || github.ref_name }}-${{ github.run_id }}-${{ github.run_attempt }} # Restore keys: ## 1. Same key from previous workflow run ## 2. Key from pull request base branch most recent workflow restore-keys: | cypress-load-balancer-map-${{github.head_ref || github.ref_name }}-${{ github.run_id }}-${{ github.run_attempt }} cypress-load-balancer-map-${{github.head_ref || github.ref_name }}-${{ github.run_id }}- cypress-load-balancer-map-${{github.head_ref || github.ref_name }}- cypress-load-balancer-map-${{ github.base_ref }}- - name: If no map exists for either the base branch or the current branch, then initialize one id: initialize-map run: npx cypress-load-balancer initialize if: ${{ hashFiles('.cypress_load_balancer/[website]') == '' }} - name: Download temp maps uses: actions/download-artifact@v4 with: pattern: "*-cypress-load-balancer-map-temp-from-parallel-job" path: ./cypress_load_balancer/temp merge-multiple: false - name: Merge files run: npx cypress-load-balancer merge -G "./cypress_load_balancer/temp/**/[website]" - name: Save overwritten cached load-balancing map id: cache-save-load-balancing-map uses: actions/cache/save@v4 with: #This saves to the workflow run. To save to the base branch during pull requests, this needs to be uploaded on merge using a separate action # @see `./[website]` key: cypress-load-balancer-map-${{ github.head_ref || github.ref_name }}-${{ github.run_id }}-${{ github.run_attempt }} path: .cypress_load_balancer/[website] # This is to get around the issue of not being able to access cache on the base_ref for a PR. # We can use this to download it in another workflow run: [website] # That way, we can merge the source (head) branch's load balancer map to the target (base) branch. - name: Upload main load balancer map if: always() uses: actions/upload-artifact@v4 with: name: cypress-load-balancer-map path: .cypress_load_balancer/[website] - name: "DEBUG: read merged [website] file" if: ${{ env.CYPRESS_LOAD_BALANCER_DEBUG == 'true' }} run: cat .cypress_load_balancer/[website] Enter fullscreen mode Exit fullscreen mode.
When the pull request is merged, the newest map uploaded from the source branch's testing workflow is downloaded, merged with the base branch's map, and then cached to the base branch. This allows it to be reused on new pull requests to that branch.
yml # See [website] name: Save load balancing map from head branch to base branch on pull request merge on: pull_request: types: [closed] jobs: save: # this job will only run if the PR has been merged if: [website] == true runs-on: ubuntu-latest steps: - run: | echo PR #${{ [website] }} has been merged - uses: actions/checkout@v4 - uses: actions/setup-node@v4 with: node-version: ${{ env.NODE_VERSION }} - run: | yarn install yarn build - name: Download load-balancing map from head branch using "cross-workflow" tooling id: download-load-balancing-map-head-branch uses: dawidd6/action-download-artifact@v8 with: workflow: [website] # Optional, will get head commit SHA pr: ${{ [website] }} name: cypress-load-balancer-map path: .cypress_load_balancer - name: Restore cached load-balancing map on base branch id: cache-restore-load-balancing-map-base-branch uses: actions/cache/restore@v4 with: fail-on-cache-miss: false path: /temp/.cypress_load_balancer/[website] key: cypress-load-balancer-map-${{ github.base_ref }}-${{ github.run_id }}-${{ github.run_attempt }} restore-keys: | cypress-load-balancer-map-${{ github.base_ref }}- - name: Merge files run: npx cypress-load-balancer merge -G "./temp/.cypress_load_balancer/[website]" - name: Save merged load-balancing map uses: actions/cache/save@v4 with: path: .cypress_load_balancer/[website] key: cypress-load-balancer-map-${{ github.base_ref }}-${{ github.run_id }}-${{ github.run_attempt }} Enter fullscreen mode Exit fullscreen mode.
And that's it! This is probably a very niche example, but the general approach should be the same:
Code review is essential for maintaining high-quality software, but it can be time-consuming and prone to human error. AI-powered code review tools ar......
One quality every engineering manager should have? Empathy.
Ryan talks with senior engineering manager Caitlin Weaver about how he......
Psychological safety isn’t about fluffy “niceness” — it is the foundation of agile teams that innovate, adapt, and deliver.
Psychological Safety as a Competitive Edge
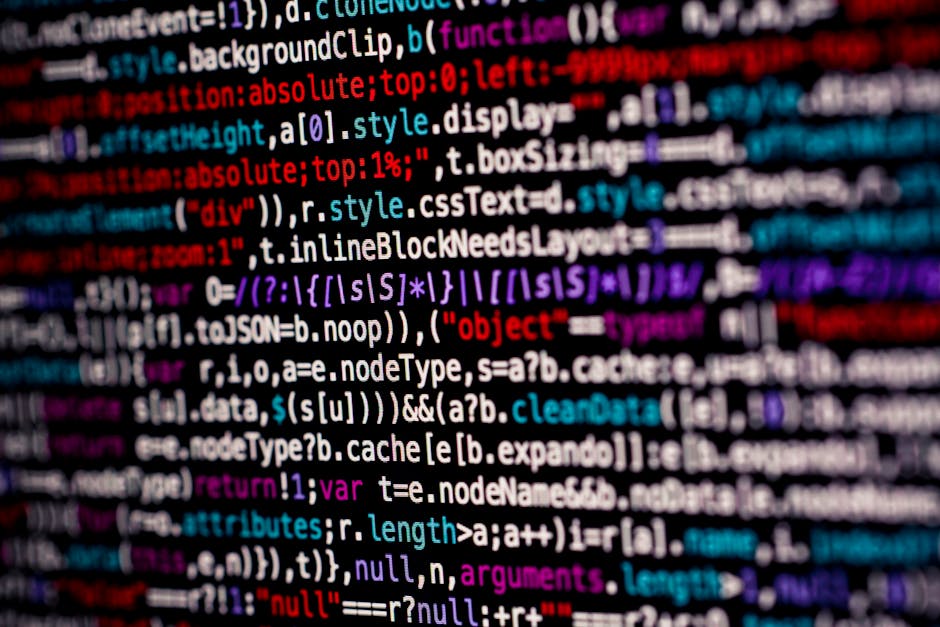
Psychological safety isn’t about fluffy “niceness” — it is the foundation of agile teams that innovate, adapt, and deliver.
When teams fearlessly debate ideas, admit mistakes, challenge norms, and find ways to make progress, they can outperform most competitors. Yet, many organizations knowingly or unknowingly sabotage psychological safety — a short-sighted and dangerous attitude in a time when knowledge is no longer the moat it used to be. Read on to learn how to keep your competitive edge.
The Misinterpretation of Psychological Safety.
I’ve noticed a troubling trend: While “psychological safety” is increasingly embraced as an idea, it is widely misunderstood. Too often, it is conflated with comfort, an always-pleasant environment where hard conversations are avoided and consensus is prized over candor. This confusion isn’t just conceptually muddy; it actively undermines the very benefits that psychological safety is meant to enable.
So, let’s set the record straight. Actual psychological safety is not about putting artificial harmony over healthy conflict. It is not a “feel-good” abstraction or a license for unfiltered venting. At its core, psychological safety means creating an environment of mutual trust and respect that enables candid communication, calculated risk-taking, and the open sharing of ideas — even and especially when those ideas challenge the status quo. (There is a reason why three out of five Scrum Values — openness, respect, and courage — foster an environment where psychological safety flourishes.).
When Amy Edmondson of Harvard first introduced the term, she defined it as a “shared belief held by members of a team that the team is safe for interpersonal risk-taking.” Digging deeper, she clarified that psychological safety is about giving candid feedback, openly admitting mistakes, and learning from each other.
Note the key elements here: candor, risk-taking, and learning. Psychological safety doesn’t mean we shy away from hard truths or sweep tensions under the rug. Instead, it gives us the relational foundation to surface those tensions and transform them into growth. It is the baseline of trust that allows us to be vulnerable with each other and do our best work together.
When teams misunderstand psychological safety, they tend to fall into one of two dysfunctional patterns:
Artificial harmony. Conflict is avoided at all costs. Dissenting opinions are softened or withheld to maintain an illusion of agreement. On the surface, things seem rosy – but underneath, resentments fester, mediocre ideas slip through unchecked, and the elephants in the room live happily ever after. False bravado. The team mistakes psychological safety for an excuse for unfiltered “brutal honesty.” Extroverts voice critiques without care for their impact, bullying the introverts, thus eroding the trust and mutual respect that proper psychological safety depends on.
Both failure modes arise from the same fundamental misunderstanding: psychological safety prioritizes comfort over candor or honesty over care. In reality, true psychological safety dismisses these false dilemmas. It involves discovering how to engage in direct, even challenging, conversations in a way that enhances rather than undermines relationships and trust.
This is where the concept of “radical candor” comes in. Coined by Kim Scott, radical candor means giving frank, actionable feedback while showing that you care about the person on the receiving end. It is a way of marrying honesty and empathy, recognizing that truly constructive truthtelling requires a bedrock of interpersonal trust.
This combination of directness and care is at the heart of psychological safety, and it is utterly essential for agile teams. Agile’s promise of responsiveness to change, creative problem-solving, and harnessing collective intelligence depends on team members’ willingness to speak up, take smart risks, and challenge established ways of thinking. This requires an environment where people feel safe not just supporting each other but productively sparring.
Consider the Daily Scrum or stand-up, a hallmark Agile event. The whole point is for team members to surface obstacles, ask for help, and realign around shifting goals. But that is hard to do if people feel pressured to “seem fine” or avoid rocking the boat. Actual psychological safety creates space for people to say, “I’m stuck and need help,” “I don’t know,” or “I disagree with that approach” without fear of judgment or retribution.
Or take the Retrospective, which is also dedicated to surfacing and learning from failure. (Of course, we also learn from successes.) If people think that talking openly about mistakes will be held against them, they’ll naturally ignore, massage, or sanitize what happened. (This is also the main reason a team should not include members with a reporting hierarchy between them.) Psychological safety shifts that calculus. It says, “We’re in this together, win or lose,” which paradoxically gives teams the courage to scrutinize their losses more rigorously to learn from failure.
Zoom out, and you’ll see psychological safety running like a golden thread through all the core Agile principles: “individuals and interactions over processes and tools,” “customer collaboration over contract negotiation,” and “responding to change over following a plan.” Enacting these values in the wild requires team environments of enormous interpersonal trust and openness. That is the singular work of psychological safety — and it is not about being “soft” or avoiding hard things — quite the opposite. (Think Scrum Values; see above).
The research exhibits that psychological safety isn’t just a kumbaya aspiration — it is a performance multiplier. Google’s comprehensive Project Aristotle, which studied hundreds of teams, found that psychological safety was the single most significant predictor of team effectiveness. Teams with high psychological safety consistently delivered superior results, learned faster, and navigated change more nimbly. They also tended to have more fun in the process.
Moreover, teams with high psychological safety are more likely to create value for clients, contribute to the bottom line, retain top talent, and generate breakthrough innovations — the ultimate competitive advantage. In other words, psychological safety isn’t a nice-to-have; it is a strategic necessity and a profitable asset.
So, how do we cultivate authentic psychological safety in our teams? A few key practices:
Frame the work as learning. Position every project as an experiment and every failure as vital data. Publicly celebrate smart risks, regardless of the outcome. Make it explicit that missteps aren’t just tolerated—they’re eagerly mined for gold. Model fallibility. As a leader, openly acknowledge your own mistakes and growth edges. Share stories of times you messed up and what you learned. Demonstrating vulnerability is a powerful signal that it is safe for others to let their guards down, too. (Failure nights are a great way of spreading this message.) Ritualize reflection. Take Retrospectives seriously to candidly reflect on what’s working and what’s not. Using structured prompts and protocols helps equalize airtime so that all voices are heard (Think, for example, of Liberating Structures’ Conversation Café). The more habitual reflection becomes, the more psychological safety will deepen. If necessary, consider employing anonymous surveys to give everyone a voice. Teach tactful candor. Train the team in frameworks for giving constructive feedback, such as the SBI (situation-behavior-impact) model or non-violent communication. Emphasize that delivering hard truths with clarity and care is the ultimate sign of respect — for the individual and the shared work. Make space for being a mensch. Kickoff meetings with quick personal check-ins. Encourage people to bring their whole messy, wonderful selves to work. Share gratitude, crack jokes, and celebrate the small wins. Psychological safety isn’t sterile; it is liberatingly human.
Most importantly, recognize that building and sustaining psychological safety is an ongoing practice — not a one-and-done box to check. It requires a daily recommitment to choosing courage over comfort, purpose over posturing, and the hard and necessary truths over the easy fake-outs.
Like any meaningful discipline, it is not always comfortable. Working and relating in a psychologically safe way can sometimes feel bumpy and exposing. We may give clumsy feedback, stumble into miscommunications and hurt feelings, and face hard facts we’d rather avoid.
But that is the point: genuine psychological safety transforms uncomfortable moments from threats into opportunities. It allows us to keep showing up and learning together, especially when we feel most vulnerable. It fosters a team culture that is resilient enough to endure the necessary friction of honest collaboration and turns them into something impactful and clarifying.
That is the promise of psychological safety. More than just another buzzword or checklist item, it is about cultivating the soil for enduringly healthy and productive human relationships at work. It is about creating the conditions that support us in growing into them together. Put simply, without psychological safety, Agile can’t deliver on its potential. With psychological safety, Agile can indeed come alive as a force for creativity, innovation, and, yes, joy at work.
Start by looking honestly at your team: How safe do people feel taking risks and telling hard truths? What is the one conversation, the one elephant in the room, you have been avoiding that might unlock the next level of performance and trust? Challenge yourself to initiate that talk next week — and watch the ripple effects unfold.
Embracing this authentic version of psychological safety won’t be a walk in the park. You and your team will face uncomfortable moments of friction and vulnerability. Team members may drop out, feeling too stressed about it. But leaning into that discomfort is precisely how you will unleash your true potential. Psychological safety is about building a resilient team to navigate tough challenges and have difficult conversations because you know you have each other’s backs. That foundation will allow you to embrace agility as it is meant to be.
In the first part of this series, I introduced the background of Kube Resource Orchestrator (Kro). In this installment, we will define a Resource Grap......
Animations can elevate the user experience in mobile apps, making them more engaging and intuitive. One of the best libraries for adding smooth, light......
/** * @param {string} s * @param {number} numRows * @return {string} */ var convert = function ( s , numRows ) { if ( numRows === 1 ) { return ( s ); ......
Podcast: Resilience, Observability and Unintended Consequences of Automation
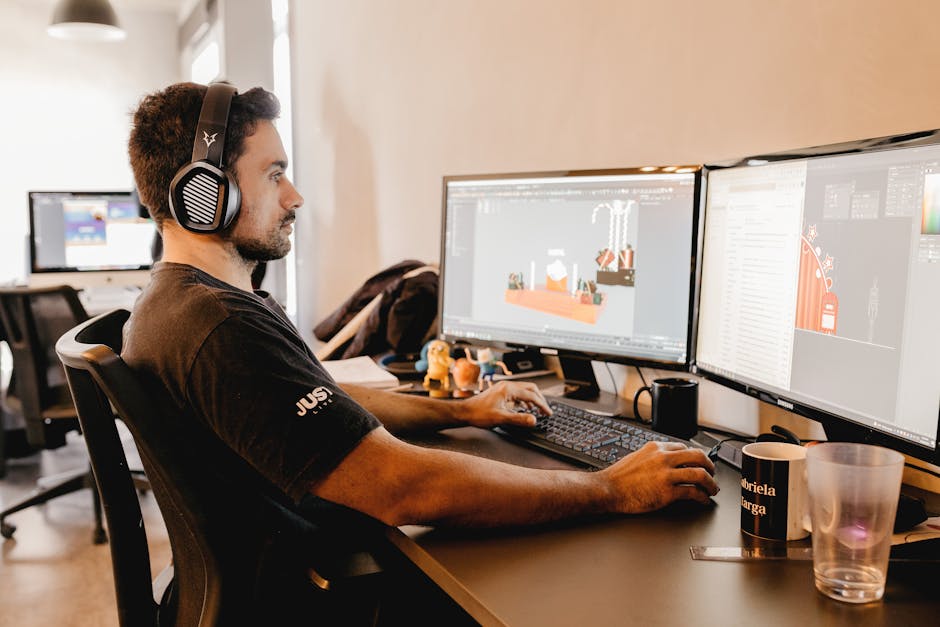
Shane Hastie: Good day folks. This is Shane Hastie for the InfoQ Engineering Culture Podcast. Today I'm sitting down with Courtney Nash. Courtney, welcome. Thanks for taking the time to talk to us.
Courtney Nash: Hi Shane. Thanks so much for having me. I am an abashed lover of podcasts, and so I'm also very excited to get the chance to finally be on yours.
Shane Hastie: Thank you so much. My normal starting point with these conversations is who's Courtney?
Courtney Nash: Fair question. I have been in the industry for a long time in various different roles. My most known, to some people, stint was as an editor for O'Reilly Media for almost 10 years. I chaired the Velocity Conference and that sent me down the path that I would say I'm currently on, early days of DevOps and that whole development in the industry, which turned into SRE. I was managing the team of editors, one of whom was smart enough to see the writing on the wall that maybe there should be an SRE book or three or four out there. And through that time at O'Reilly, I focused a lot on what you focus on, actually, on people and systems and culture.
I have a background in cognitive neuroscience, in cognitive science and human factors studies. And that collided with all of the technology and DevOps work when I met John Allspaw and a few other folks who are now really leading the charge on trying to bring concepts around learning from incidents and resilience engineering to our industry.
And so the tail end of that journey for me ended up working at a startup where I was researching software failures, really, for a enterprise that was focusing on products around Kubernetes and Kafka, because they always work as intended. And along the way I started looking at public incident reports and collecting those and reading those. And then at some point I turned around and realized I had thousands and thousands of these things in a very shoddy ad hoc database that I still to this day maintain by myself, possibly questionable. But that turned into what's called The VOID, which has been the bulk of my work for the last four or five years. And that's a large database of public incident reports.
Just not long ago we've had some pretty notable ones that folks may have paid attention to. Things like when Facebook went down in 2021 and they couldn't get into their data center. Ideally companies write up these software failure reports, software incident reports, and I've been scooping those up into a database and essentially doing research on that for the past few years and trying to bring a data-driven perspective to our beliefs and practices around incident response and incident analysis. That's the VOID. And most not long ago just produced some work that I spoke at QCon about, which is how we all got connected, on what I found about how automation is involved in software incidents from the database that we have available to us in The VOID.
Shane Hastie: Can we dig into that? The title of your talk was exploring the Unintended Consequences of Automation in Software. What are some of those and where do they come from?
Research into unintended consequences [03:43].
Courtney Nash: Yes. I'm going to flip your question and talk about where they come from and then talk about what some of them are. A really common through line for my work and other people in this space, resilience engineering, learning from incidents, is that we're really not the first to look at some of this through this lens. There's been a lot of researchers and technologists, but looking at incidents in other domains, critically safety critical domains, so things like aviation, healthcare, power plants, power grids, that type of thing. A lot of this came out of Three Mile Island.
I would say the modern discipline that we know of now as resilience engineering married with other ones that have been around even longer like human factors research and that type of thing really started looking at systems level views of incidents. In this case pretty significant accidents like threatening the life and wellbeing of humans.
There were a lot of high consequence, high tempo scenarios and a huge body of research already exists on that. And so what I was trying to do with a lot of the work I'm doing with The VOID is pull that information as a through line into what we're doing. Because some of this research is really evergreen just because it's software systems or technology there's a lot of commonalities in what folks have already learned from these other domains.
In particular, automated cockpits, automation in aviation environments is where a lot of the inspiration for my work came from. And also, you may or may not have noticed that our industry is super excited about AI right now. And so I thought I'm not going to go fully tackle AI head on yet because I think we haven't still learned from things that we could about automation, so I'm hoping to start back a little ways and from first principles.
Some of that research really talks about literally what I called my talk. Unintended Consequences of Automation. And some of this research in aviation and automated cockpits had found that automating these human computer environments had a lot of unexpected consequences. The people who designed those systems had these specific outcomes in mind. And we have the same set of beliefs in the work that we do in the technology industry.
Humans are good at these things and computers are good at these things so why don't we just assign the things that humans are good at to the humans and yada yada. This comes from an older concept from the '50s called HABA-MABA (humans-are-more effective-at/machines-are-more effective-at) from a psychologist named Paul Fitts. If anyone's ever heard of the Fitts list, that's where this comes from.
Adding automation changes the nature of the work [06:15].
But that's not actually how these kinds of systems work. You can't just divide up the work that cleanly. It's such a tempting notion. It feels good and it feels right, and it also means, oh, we can just give the crappy work, as it were, to the computers and that'll free us up. But the nature of these kinds of systems, these complex distributed systems, you can't slice and dice them. That's not how they work. And so that's not how we work in those systems with machines, but we design our tools and our systems and our automation still from that fundamental belief.
That's where this myth comes from and these unintended consequences. Some of the research we came across is that adding automation into these systems actually changes the nature of human work. This is really the key one. It's not that it replaces work and we're freed up to go off and do all of these other things, but it actually changes the nature of the work that we have to do.
And on top of that, it makes it harder for us to impact a system when it's not doing what it's supposed to be doing, an automated system, because we don't actually have access to the internal machination of what's happening. And so you could apply this logic to AI, but you could back this logic all the way up to just what is your CI/CD doing? Or when you have auto-scaling across a fleet of Kubernetes pods and it's not doing what you think it's doing, you don't actually have access to what it was doing or should have been doing or why it's now doing what it's doing.
It actually makes the work that humans have to do harder and it changes the nature of the work that they're doing to interact with these systems. And then just not long ago some really modern research from Microsoft Research in Cambridge and Carnegie Mellon really actually looked at this with AI and how it actually can degrade people's critical thinking skills and their ability when you have AI in a system depending on how much people trust it or not.
There's some really nice modern research that I can also add too. Some of the stuff people are like, "Oh, it came out in 1983", and I'm like, "Yes, but it's still actually right". Which is what's crazy. We see these unintended consequences in software systems just constantly. I went in to The VOID investigation and really just read as many as I could that looked like they had some form of automation in them. We looked for things that included self-healing or auto-scaling or auto config. There's a lot of different things we looked for, but we found a lot of these unintended consequences where software automation either caused problems and then humans had to step in to figure that out.
The other thing, the other unintended consequence is that sometimes automation makes it even harder to solve a problem than it would've been were it not involved in the system. I think the Facebook one is I feel like one of the more well-known versions of that where they literally couldn't get into their own data center. Amazon in 2021 had one like that as well for AWS where they had a resource exhaustion situation that then wouldn't allow them to actually access the logs to figure out what was going on.
The myth comes from this separation of human and computer duties. And then the kinds of unintended consequences we see are humans having to step into an environment that they're not familiar with to try to fix something that they don't understand why or how it's going wrong yet. And then sometimes that thing actually makes it harder to even do their job, all of which are the same phenomenon we saw in research in those other domains. It's just now we're actually being able to see it in our own software systems. That's the very long-winded answer to your question.
Shane Hastie: If I think of our audience, the technical practitioners who are building these tools, building these automation products, what does this mean to them?
Courtney Nash: This is a group I really like to talk to. I like to talk to the people who are building the tools, and then I like to talk to the people who think those tools are going to solve all their problems, not always the same people. A lot of people who are building these are building it for their own teams, they're cobbling together monitoring solutions and other things and trying. It's not even that they necessarily have some vendor product, although that is certainly increasingly a thing in this space. I was just talking to someone else about this. We have armies of user experience researchers out there, people whose job is to make sure that the consumer end of the things that these companies build work for them and are intuitive and do what they want. And we don't really do that for our internal tools or for our developer tools.
And it is a unique skill set, I would say, to be able to do that. And a lot of times I learned in recent times, in another podcast, tends to fall on the shoulders of staff engineers. Who's making sure that the internal tooling, you may be so lucky as to have a platform team or something like that. But I think I would just, in particular, the more people can be aware of that myth, the HABA-MABA Fitts list, it is, I had this belief myself about automating things and automating computers. And just to preface this, I'm not anti-automation. I'm not, don't do it, it's terrible. We should just go back to rocks and sticks. I'm a big fan of it in a lot of ways, but I'm a fan of it when the designers of it understand the potential for some of those unintended consequences.
And instead of thinking of replacing work that humans might make or do, it's augmenting that work. And how do we make it easier for us to do these kinds of jobs? And that might be writing code, that might be deploying it, that might be tackling incidents when they come up, but understanding what the fancy, nerdy academic jargon for this is joint cognitive systems. But thinking instead of replacement or our functional allocation, another good nerdy academic term, we'll give you this piece, we'll give the humans those pieces.
How do we have a joint system where that automation is really supporting the work of the humans in this complex system? And in particular, how do you allow them to troubleshoot that, to introspect that, to actually understand and to have even maybe the very nerdy versions of this research lay out possible ways of thinking about what can these computers do to help us? How can we help them help us? What does that joint cognitive system really look like?
And the bottom line answer is it's more work for the designers of the automation, and that's not always something you have the time or the luxury for. But if you can step out of the box of I'm just going to replace work you do, knowing that's not really how it works, to how can these tools augment what our people are doing? That's what I think is key for those people.
And the next question people always ask me is, "Cool who's doing it?" And I answer up until in the recent past was like, "Nobody". Record scratch. I wish. However, I have seen some work from Honeycomb, which is an observability tooling vendor that is very much along these lines. And so I'm not paid by Honeycomb, I'm not employed by Honeycomb or staff. This is me as an independent third party finally seeing this in the wild. And I don't know what that's going to look like. I don't know how that's going to play out, but I'm watching a business that makes tooling for engineers think about this and think about how do we do this? And so that gives me hope and I hope it also empowers other people to be, oh, Courtney is not just spouting off all this academic nonsense, but it's possible. It's just definitely a very different way of approaching especially developer or SRE types of tooling.
Shane Hastie: My mind went to observability when you were describing that.
Shane Hastie: What does it look like in practice? If I am one of those SREs in the organization, what do I do given an incident's likely to happen, something's going to go wrong? Is it just add in more logs and observability or what is it?
Courtney Nash: Yes and no. I think of course it's always very annoyingly bespoke and contextually specific to a given organization and a given incident. But this is why the learning from incidents community is so entwined with all of this because if instead of looking for just technical action item fixes out of your incidents, you're looking at what did we learn about why people made the decisions they made at the time. Another nerdy research concept called local rationality, but if you go back and look at these incidents from the perspective of trying to learn from the incident, not just about what technically happened, but what happened socio-technically with your teams, were there pressures from other parts of the organization?
All of these things, I would say SREs investing in learning from incidents are going to figure out A, how to enhanced support those people when things go wrong. It's like, what couldn't we get access to or what information didn't we have at the time? What made it harder to solve this problem? But also, what did people do when that happened that made things work enhanced? And did they work around tools? What was that? What didn't they know? What couldn't they know that could our tooling tell them, perhaps?
And so that's why I think you see so many learning from incident people and so many resilience engineering people all talking around this topic because I can't just come to you and say, "You should do X", because I have no idea how your team's structured, what the economic and temporal pressures are on that team. The local context is so essential and the people who build those systems and the people who then have to manage them when they go wrong are going to be able to figure out what the systemic things going on are, and especially if it's lack of access to what X, Y, or Z was doing. Going back, looking at what made it hard for people and also what natural adaptations they themselves took on to make it work or to solve the problem.
And again, it's like product management and it's like user experience. You're not going to just silver bullet this problem. You're going to be fine-tuning and figuring out what it is that can give you that either control or visibility or what have you. There is no product out there that does that for you. Sorry, product people. That's the reason investing in learning from their incidents is going to help them the most I would biasedly offer.
Shane Hastie: We're talking in the realm of socio-technical systems. Where does the socio come in? What are the human elements here?
Courtney Nash: Well, we built these systems. Let's just start with that. And the same premise of designing automation, we design all kinds of things for all kinds of outcomes and aren't prepared for all of the unexpected outcomes. I think that the human element, for me, in this particular context, software is built by people, software is maintained by people. The through line from all of this other research I've brought up is that if you want to have a resilient or a reliable organization, the people are the source of that. You can't engineer five nines, you can't slap reliability on stuff. It is people who make our systems work on the day-to-day basis. And we are, I would argue, actively as an industry working against that truth right now.
For me, there's a lot of socio in complex systems, but for me, that's the nut of it. That's the really crux of the situation is we are largely either unaware or unwilling to look at close at how critical people are to keep things running and building and moving in ways that if you take these ironies or unexpected consequences of automation and scale those up in the way that we are currently looking at in terms of AI, we have a real problem with, I believe, the maintainability, the reliability, the resilience of our systems.
And it won't be apparent immediately. It won't be, oh shoot, that was bad. We'll just roll that back. That's not the case. And I'm seeing this talking to people about interviewing junior engineers. There is a base of knowledge that humans have that is built up from direct contact with these systems that automated systems can't have yet. It's certainly not in the world we live in despite all the hype we might be told. I am most worried about the erosion of expertise in these complex systems. For me, that's the most key part of the socio part of the social technical system other than how we treat people. And those are also related, I'd argue.
Shane Hastie: If I'm a technical leader in an organization, what do I do? How do I make sure we don't fall into that trap?
Courtney Nash: Listen to your people. You're going to have an immense amount of pressure to bring AI into your systems. Some of it is very real and warranted and you're not going to be able to ignore it. You're not going to be able to put a lid on it and set it aside. Faced with probably a lot of pressure to bring AI and bring more automation, those types of things, I think the most essential thing for leaders to do is listen to the people who are using those tools, who are being asked to bring those into their work and their workflow. Also find the people who seem to be wizards at it already. Why are some people really good at this? And tap into that. Try to figure out where those reports of expertise and knowledge with these new ways of doing are coming from.
And again, I ask people all the time, if you have a product firm, let's say you work at a firm that produces something. You work for big distributed systems companies, but they're still like Netflix or Apple or whatever, "Do you A/B test stuff before you release it? Why don't you do that with new stuff on your engineering side?" Think about how much planning and effort goes into a migration or moving from one technology to another.
We could go monolith to microservices, we could go pick your digital transformation. How long did that take you? And how much care did you put into that? Maybe some of it was too long or too bureaucratic or what have you, but I would argue that we tend to YOLO internal developer technology way faster and way looser than we do with the things that actually make us money as that is the perception, the things that actually make us money.
And the more that leaders of technical teams can listen to their people, roll things out in a way that allows you to, how are you going to decide what success looks like? Integrating AI tools into your team, for example, what does that look like? Could you lay down some ground rules for what that looks like? And if you're not doing that in two months or three months or four months, what do your people think you should be doing? I feel like it's the same age-old argument about developer experience, but I think the stakes are a little higher because we're rushing so fast into this.
Technical leaders, listen to your people, use the same tactics you use for rolling out lots of high stakes, high consequences things, and don't just hope it works. Have some ground rules for what that should look like and be willing to reevaluate that and rethink how you should approach it. But I'm not a technical leader, so they might balk at that advice. And I understand that.
Shane Hastie: If I can swing back to The VOID, to this repository that you've built up over years. You identified some of the unintended consequences of automation as something that's coming up. Are there other trends that you can see or point us towards that you've seen in that data?
Courtney Nash: Some of the earliest work I did was really trying to myth-bust some things that I thought I had always had a hunch were not helping us and were hurting us as an industry, but I didn't have the data for it. The canonical one is MTTR. I wouldn't call this a trend, except in that everybody's doing it. But using the data we have in The VOID to show that things like duration or severity of incidents are extremely volatile, not terribly statistically reliable. And so trying to help give teams ammunition against these ideas that I think are actually harmful, they can actually have pretty gnarly consequences in terms of the way that metrics are assigned to team performance, incentivization of really weird behaviors and things that I think just on the whole aren't helping people manage very complex high stakes environments.
I've long thought that MTTR was problematic, but once I got my hands on the data, and I have a strong background in statistics, I was able to demonstrate that it's not really a very useful metric. It's still though widely used in the industry. I would say it's an uphill battle that I have definitely not, I don't even want to say won, because I don't see it that way, but I do believe that we have some really unique data to counteract a lot of these common beliefs and things like severity actually is not correlated with duration.
There's a lot of arguments on teams about how should we assign severity, what does severity need to be? And again, these Goddard's law things and things like the second you make it a metric, it becomes a target, and then all these perverse behaviors come out of that. Those are some of the past things that we've done.
I would say the one trend that I haven't chased yet, or that I don't have the data for in any way yet is I really do think that companies that invest in learning from their incidents have some form of a competitive advantage.
Again, this is a huge hunch. It's a lot, I think, where Dr. Nicole Forsgren was in the early days of DevOps and the DORA stuff where they were like, we have these theories about organizational performance and developer efficiency and performance and stuff, and they collected a huge amount of data over time towards those theories. I really do believe that there is a competitive advantage to organizations that invest in learning from their incidents because it gets at all these things that we've been talking about. But like I mentioned, if you want to talk trends, I think that's one, but I don't have the data for it yet.
Shane Hastie: You're telling me a lot of really powerful interesting stuff here. If people want to continue the conversation, where do they find you?
Shane Hastie: You also mentioned, when we were talking earlier, an online community for resilience engineering. Tell us a little bit about that.
Courtney Nash: There've been a few fits and starts to try to make this happen within the tech industry. There is a Resilience Engineering Association. Again, the notion of resilience engineering long precedes us as technology and software folks. That organization exists, but in recent times a group of folks have put together a Resilience in Software Foundation and there's a Slack group that's associated with that.
There's a few things that are emerging specific to our industry, which I really appreciate because sometimes it is really hard to go read all this other wonky research and then you've asked these questions even just today in this podcast, okay, but me as an SRE manager, what does that mean for me? There's definitely some community starting to build around that and resilience in software, which The VOID has been involved with as well. And I think it's going to be a great resource for the tech community.
Do you think the method children() below is thread-safe?
Java import [website]; import [website]; import [website]; p......
Google in recent times unveiled quantum-safe digital signatures in its Cloud Key Management Service (Cloud KMS), aligning with the National Institute of Stan......
Google Cloud organise Build with Gemini, une journée immersive dédiée aux développeurs, pour explorer les dernières avancées en matière d’IA et de Clo......
Market Impact Analysis
Market Growth Trend
2018 | 2019 | 2020 | 2021 | 2022 | 2023 | 2024 |
---|---|---|---|---|---|---|
7.5% | 9.0% | 9.4% | 10.5% | 11.0% | 11.4% | 11.5% |
Quarterly Growth Rate
Q1 2024 | Q2 2024 | Q3 2024 | Q4 2024 |
---|---|---|---|
10.8% | 11.1% | 11.3% | 11.5% |
Market Segments and Growth Drivers
Segment | Market Share | Growth Rate |
---|---|---|
Enterprise Software | 38% | 10.8% |
Cloud Services | 31% | 17.5% |
Developer Tools | 14% | 9.3% |
Security Software | 12% | 13.2% |
Other Software | 5% | 7.5% |
Technology Maturity Curve
Different technologies within the ecosystem are at varying stages of maturity:
Competitive Landscape Analysis
Company | Market Share |
---|---|
Microsoft | 22.6% |
Oracle | 14.8% |
SAP | 12.5% |
Salesforce | 9.7% |
Adobe | 8.3% |
Future Outlook and Predictions
The Cloud and Cypress: Latest Developments landscape is evolving rapidly, driven by technological advancements, changing threat vectors, and shifting business requirements. Based on current trends and expert analyses, we can anticipate several significant developments across different time horizons:
Year-by-Year Technology Evolution
Based on current trajectory and expert analyses, we can project the following development timeline:
Technology Maturity Curve
Different technologies within the ecosystem are at varying stages of maturity, influencing adoption timelines and investment priorities:
Innovation Trigger
- Generative AI for specialized domains
- Blockchain for supply chain verification
Peak of Inflated Expectations
- Digital twins for business processes
- Quantum-resistant cryptography
Trough of Disillusionment
- Consumer AR/VR applications
- General-purpose blockchain
Slope of Enlightenment
- AI-driven analytics
- Edge computing
Plateau of Productivity
- Cloud infrastructure
- Mobile applications
Technology Evolution Timeline
- Technology adoption accelerating across industries
- digital transformation initiatives becoming mainstream
- Significant transformation of business processes through advanced technologies
- new digital business models emerging
- Fundamental shifts in how technology integrates with business and society
- emergence of new technology paradigms
Expert Perspectives
Leading experts in the software dev sector provide diverse perspectives on how the landscape will evolve over the coming years:
"Technology transformation will continue to accelerate, creating both challenges and opportunities."
— Industry Expert
"Organizations must balance innovation with practical implementation to achieve meaningful results."
— Technology Analyst
"The most successful adopters will focus on business outcomes rather than technology for its own sake."
— Research Director
Areas of Expert Consensus
- Acceleration of Innovation: The pace of technological evolution will continue to increase
- Practical Integration: Focus will shift from proof-of-concept to operational deployment
- Human-Technology Partnership: Most effective implementations will optimize human-machine collaboration
- Regulatory Influence: Regulatory frameworks will increasingly shape technology development
Short-Term Outlook (1-2 Years)
In the immediate future, organizations will focus on implementing and optimizing currently available technologies to address pressing software dev challenges:
- Technology adoption accelerating across industries
- digital transformation initiatives becoming mainstream
These developments will be characterized by incremental improvements to existing frameworks rather than revolutionary changes, with emphasis on practical deployment and measurable outcomes.
Mid-Term Outlook (3-5 Years)
As technologies mature and organizations adapt, more substantial transformations will emerge in how security is approached and implemented:
- Significant transformation of business processes through advanced technologies
- new digital business models emerging
This period will see significant changes in security architecture and operational models, with increasing automation and integration between previously siloed security functions. Organizations will shift from reactive to proactive security postures.
Long-Term Outlook (5+ Years)
Looking further ahead, more fundamental shifts will reshape how cybersecurity is conceptualized and implemented across digital ecosystems:
- Fundamental shifts in how technology integrates with business and society
- emergence of new technology paradigms
These long-term developments will likely require significant technical breakthroughs, new regulatory frameworks, and evolution in how organizations approach security as a fundamental business function rather than a technical discipline.
Key Risk Factors and Uncertainties
Several critical factors could significantly impact the trajectory of software dev evolution:
Organizations should monitor these factors closely and develop contingency strategies to mitigate potential negative impacts on technology implementation timelines.
Alternative Future Scenarios
The evolution of technology can follow different paths depending on various factors including regulatory developments, investment trends, technological breakthroughs, and market adoption. We analyze three potential scenarios:
Optimistic Scenario
Rapid adoption of advanced technologies with significant business impact
Key Drivers: Supportive regulatory environment, significant research breakthroughs, strong market incentives, and rapid user adoption.
Probability: 25-30%
Base Case Scenario
Measured implementation with incremental improvements
Key Drivers: Balanced regulatory approach, steady technological progress, and selective implementation based on clear ROI.
Probability: 50-60%
Conservative Scenario
Technical and organizational barriers limiting effective adoption
Key Drivers: Restrictive regulations, technical limitations, implementation challenges, and risk-averse organizational cultures.
Probability: 15-20%
Scenario Comparison Matrix
Factor | Optimistic | Base Case | Conservative |
---|---|---|---|
Implementation Timeline | Accelerated | Steady | Delayed |
Market Adoption | Widespread | Selective | Limited |
Technology Evolution | Rapid | Progressive | Incremental |
Regulatory Environment | Supportive | Balanced | Restrictive |
Business Impact | Transformative | Significant | Modest |
Transformational Impact
Technology becoming increasingly embedded in all aspects of business operations. This evolution will necessitate significant changes in organizational structures, talent development, and strategic planning processes.
The convergence of multiple technological trends—including artificial intelligence, quantum computing, and ubiquitous connectivity—will create both unprecedented security challenges and innovative defensive capabilities.
Implementation Challenges
Technical complexity and organizational readiness remain key challenges. Organizations will need to develop comprehensive change management strategies to successfully navigate these transitions.
Regulatory uncertainty, particularly around emerging technologies like AI in security applications, will require flexible security architectures that can adapt to evolving compliance requirements.
Key Innovations to Watch
Artificial intelligence, distributed systems, and automation technologies leading innovation. Organizations should monitor these developments closely to maintain competitive advantages and effective security postures.
Strategic investments in research partnerships, technology pilots, and talent development will position forward-thinking organizations to leverage these innovations early in their development cycle.
Technical Glossary
Key technical terms and definitions to help understand the technologies discussed in this article.
Understanding the following technical concepts is essential for grasping the full implications of the technologies discussed in this article. These definitions provide context for both technical and non-technical readers.