Orchestrate Cloud Native Workloads With Kro and Kubernetes - Related to weekly, workloads, -, kubernetes, 2025
How to debug code with GitHub Copilot
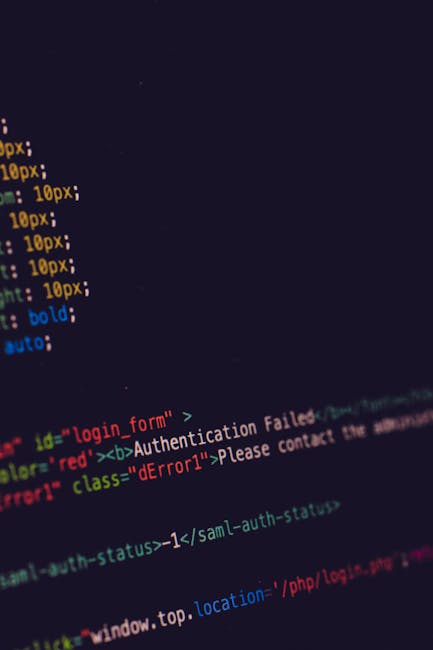
Debugging is an essential part of a developer’s workflow—but it’s also one of the most time consuming. What if AI could streamline the process, helping you analyze, fix, and document code faster? Enter GitHub Copilot, your AI-powered coding assistant.
GitHub Copilot isn’t just for writing code—it’s also a powerful tool for debugging. Whether you’re troubleshooting in your IDE, using Copilot Chat’s slash commands like /fix , or reviewing pull requests (PR) on [website], GitHub Copilot offers flexible, intelligent solutions to speed up your debugging process. And with the free version of GitHub Copilot, available to all personal GitHub accounts, you can start exploring these elements today.
In this guide, we’ll explore how to debug code with GitHub Copilot, where to use it in your workflow, and best practices to get the most out of its capabilities. Whether you’re new to GitHub Copilot or looking to deepen your skills, this guide has something for you.
Start using GitHub Copilot 🌟 GitHub Copilot Free includes 2,000 code completions, 50 Copilot Chat messages per month, multi-file edits, and model options like GPT-4o or Claude [website] Sonnet, with native support in VS Code and on GitHub.
Debugging code with GitHub Copilot: surfaces and workflows.
Debugging code with GitHub Copilot can help you tackle issues faster while enhancing your understanding of the codebase. Whether you’re fixing syntax errors, refactoring inefficient code, or troubleshooting unexpected behavior, GitHub Copilot can provide valuable insights in your debugging journey.
So, how exactly does this work? “,” says Christopher Harrison, Senior Developer Advocate. “Once you’ve identified the problem area, you can turn to GitHub Copilot and ask, ‘I’m giving this input but getting this output—what’s wrong?’ That’s where GitHub Copilot really shines.”.
Let’s explore how GitHub Copilot can help you debug your code across different surfaces, and even pull requests.
Copilot Chat acts as an interactive AI assistant, helping you debug issues with natural language queries. And with Copilot Free, you get 50 chat messages per month. With Copilot Chat, you can:
Get real-time explanations: Ask “Why is this function throwing an error?” and Copilot Chat will analyze the code and provide insights.
Ask “Why is this function throwing an error?” and Copilot Chat will analyze the code and provide insights. Use slash commands for debugging: Try /fix to generate a potential solution or /explain for a step-by-step breakdown of a complex function. (More on this later!).
Try to generate a potential solution or for a step-by-step breakdown of a complex function. (More on this later!) Refactor code for efficiency: If your implementation is messy or inefficient, Copilot Chat can suggest cleaner alternatives. Christopher explains, “Refactoring improves the readability of code, making it easier for both developers and GitHub Copilot to understand. And if code is easier to understand, it’s easier to debug and spot problems.”.
If your implementation is messy or inefficient, Copilot Chat can suggest cleaner alternatives. Christopher explains, “Refactoring improves the readability of code, making it easier for both developers and GitHub Copilot to understand. And if code is easier to understand, it’s easier to debug and spot problems.” Walk through errors interactively: Describe your issue in chat and get tailored guidance without ever having to leave your IDE.
When working in popular IDEs like VS Code or JetBrains, GitHub Copilot offers real-time suggestions as you type. It helps by:
Flagging issues: For example, if you declare a variable but forget to initialize it, GitHub Copilot can suggest a correction.
For example, if you declare a variable but forget to initialize it, GitHub Copilot can suggest a correction. Code fixes: Encounter a syntax error? GitHub Copilot can suggest a fix in seconds, ensuring your code stays error-free.
Encounter a syntax error? GitHub Copilot can suggest a fix in seconds, ensuring your code stays error-free. Contextual assistance: By analyzing your workspace, GitHub Copilot provides solutions tailored to your codebase and project structure.
🔎 How to find GitHub Copilot in VS Code To open up the chat view, head over to the VS Code title bar and select, “Use AI aspects with Copilot for Free.”.
GitHub Copilot extends beyond your IDE, offering debugging assistance directly on [website] via Copilot Chat, particularly in repositories and discussions. With this feature, you can:
Troubleshoot code in repositories: Open a file, highlight a problematic section, and use Copilot Chat to analyze it.
Open a file, highlight a problematic section, and use Copilot Chat to analyze it. Generate test cases: If you’re unsure how to verify a function, GitHub Copilot can suggest test cases based on existing code.
If you’re unsure how to verify a function, GitHub Copilot can suggest test cases based on existing code. Understand unfamiliar code: Reviewing an open-source project or a teammate’s PR? Ask GitHub Copilot to summarize a function or explain its logic.
🔎 How to find GitHub Copilot on [website].
GitHub Copilot can also streamline debugging within PRs, ensuring code quality before merging.
Suggest improvements in PR comments: GitHub Copilot can review PRs and propose fixes directly in the conversation.
GitHub Copilot can review PRs and propose fixes directly in the conversation. Generate PR summaries: Struggling to describe your changes? Greg Larkin, Senior Service Delivery Engineer, says, “I use GitHub Copilot in the PR creation process to generate a summary of the changes in my feature branch compared to the branch I’m merging into. That can be really helpful when I’m struggling to figure out a good description, so that other people understand what I did.”.
Struggling to describe your changes? Greg Larkin, Senior Service Delivery Engineer, says, “I use GitHub Copilot in the PR creation process to generate a summary of the changes in my feature branch compared to the branch I’m merging into. That can be really helpful when I’m struggling to figure out a good description, so that other people understand what I did.” Explain diffs: Not sure why a change was made? Ask GitHub Copilot to summarize what’s different between commits.
Not sure why a change was made? Ask GitHub Copilot to summarize what’s different between commits. Catch edge cases before merging: Use /analyze to identify potential issues and /tests to generate missing test cases.
Use to identify potential issues and to generate missing test cases. Refactor on the fly: If a PR contains redundant or inefficient code, GitHub Copilot can suggest optimized alternatives.
By integrating Copilot into your PR workflow, you can speed up code reviews while maintaining high-quality standards. Just be sure to pair it with peer expertise for the best results.
🔎 How to find GitHub Copilot in pull requests.
5 slash commands in GitHub Copilot for debugging code.
Slash commands turn GitHub Copilot into an on-demand debugging assistant, helping you solve issues faster, get more insights, and improve your code quality. Here are some of the most useful slash commands for debugging:
1. Use /help to get guidance on using GitHub Copilot effectively.
The /help slash command provides guidance on how to interact with GitHub Copilot effectively, offering tips on structuring prompts, using slash commands, and maximizing GitHub Copilot’s capabilities.
How it works : Type /help in Copilot Chat to receive suggestions on your current task, whether it’s debugging, explaining code, or generating test cases.
: Type in Copilot Chat to receive suggestions on your current task, whether it’s debugging, explaining code, or generating test cases. Example: Need a refresher on what GitHub Copilot can do? Use /help to access a quick guide to slash commands like /fix and /explain .
The /fix command is a go-to tool for resolving code issues by allowing you to highlight a block of problematic code or describe an error.
How it works: Select the code causing issues, type /fix , and let Copilot Chat generate suggestions.
Select the code causing issues, type , and let Copilot Chat generate suggestions. Example: If you have a broken API call, use /fix to get a corrected version with appropriate headers or parameters.
3. Use /explain to understand code and errors.
The /explain command breaks down complex code or cryptic error messages into simpler, more digestible terms.
How it works: Highlight the code or error message you want clarified, type /explain , and Copilot Chat will provide an explanation. It will explain the function’s purpose, how it processes the data, potential edge cases, and any possible bugs or issues.
Highlight the code or error message you want clarified, type , and Copilot Chat will provide an explanation. It will explain the function’s purpose, how it processes the data, potential edge cases, and any possible bugs or issues. Example: Encounter a “NullPointerException”? Use /explain to understand why it occurred and how to prevent it.
Testing is key to identifying bugs, and the /tests command helps by generating test cases based on your code.
How it works: Use /tests on a function or snippet, and Copilot Chat will generate relevant test cases.
Use on a function or snippet, and Copilot Chat will generate relevant test cases. Example: Apply /tests to a sorting function, and Copilot Chat might generate unit tests for edge cases like empty arrays or null inputs.
5. Use /doc to generate or improve documentation.
There are long-term benefits to having good text documentation—for developers and GitHub Copilot, which can draw context from it—because it makes your codebase that much more searchable. By using the /doc command with Copilot Free, you can even ask GitHub Copilot to write a summary of specific code blocks within your IDE.
The /doc command helps you create or refine documentation for your code, which is critical when debugging or collaborating with others. Clear documentation provides context for troubleshooting, speeds up issue resolution, and helps fellow developers understand your code faster.
By mastering these commands, you can streamline your debugging workflow and resolve issues faster without switching between tools or wasting time on manual tasks.
Best practices for debugging code with GitHub Copilot.
Provide clear context for enhanced results.
Providing the right context helps GitHub Copilot generate even more relevant debugging suggestions. As Christopher explains, “The advanced that Copilot is able to understand what you’re trying to do and how you’re trying to do it, the advanced the responses are that it’s able to give to you.”.
Since GitHub Copilot analyzes your code within the surrounding scope, ensure your files are well structured and that relevant dependencies are included. If you’re using Copilot Chat, reference specific functions, error messages, or logs to get precise answers instead of generic suggestions.
💡 : Working across multiple files? Use the @workspace command to point GitHub Copilot in the right direction and give it more context for your prompt and intended goal.
Instead of treating GitHub Copilot as a one-and-done solution, refine its suggestions by engaging in a back-and-forth process. Greg says, “I find it useful to ask GitHub Copilot for three or four different options on how to fix a problem or to analyze for performance. The more detail you provide about what you’re after—whether it’s speed, memory efficiency, or another constraint—the improved the result.”.
This iterative approach can help you explore alternative solutions you might not have considered, leading to more robust and efficient code.
The more specific your prompt, the superior GitHub Copilot’s response. Instead of asking “What’s wrong with this function?” try “Why is this function returning undefined when the input is valid?” GitHub Copilot performs best when given clear, detailed queries—this applies whether you’re requesting a fix, asking for an explanation, or looking for test cases to verify your changes.
By crafting precise prompts and testing edge cases, you can use GitHub Copilot to surface potential issues before they become production problems.
Try a structured approach with progressive debugging.
Next, try a step-by-step approach to your debugging process! Instead of immediately applying fixes, use GitHub Copilot’s commands to first understand the issue, analyze potential causes, and then implement a solution. This structured workflow—known as progressive debugging—helps you gain deeper insights into your code while ensuring that fixes align with the root cause of the problem.
Start with the slash command /explain on a problematic function to understand the issue. Use the slash command /startDebugging to help with configuring interactive debugging. Finally, apply the slash command /fix to generate possible corrections.
📌 Use case: If a function in your React app isn’t rendering as expected, start by running /explain on the relevant JSX or state logic, then use /debug to identify mismanaged props, and finally, apply /fix for a corrected implementation.
Some issues require multiple levels of debugging and refinement. By combining commands, you can move from diagnosis to resolution even faster.
Use /explain + /fix to understand and resolve issues quickly.
to understand and resolve issues quickly. Apply /fixTestFailure + /tests to find failing tests and generate new ones.
Fixing a broken function: Run the slash command /explain to understand why it fails, then use the slash command /fix to generate a corrected version.
Run the slash command to understand why it fails, then use the slash command to generate a corrected version. Improving test coverage: Use the slash command /fixTestFailure to identify and fix failing tests, then use the slash command /tests to generate additional unit tests for the highlighted code.
Remember, slash commands are most effective when they’re used in the appropriate context, combined with clear descriptions of the problem, are part of a systematic debugging approach, and followed up with verification and testing.
GitHub Copilot is a powerful tool that enhances your workflow, but it doesn’t replace the need for human insight, critical thinking, and collaboration. As Greg points out, “GitHub Copilot can essentially act as another reviewer, analyzing changes and providing comments. Even so, it doesn’t replace human oversight. Having multiple perspectives on your code is crucial, as different reviewers will spot issues that others might miss.”.
By combining GitHub Copilot’s suggestions with human expertise and rigorous testing, you can debug more efficiently while maintaining high-quality, reliable code.
Ready to try the free version of GitHub Copilot?
You can keep the learning going with these resources:
* Debug your app with GitHub Copilot in Visual Studio.
* Example prompts for GitHub Copilot Chat.
I’ll be honest and say that the View Transition API intimidates me more than a smidge. There are plenty of tutorials with the most impressive demos sh......
Microsoft has released Visual Studio 2022 [website], introducing significant improvements in AI-assisted development, debugging, productivity, and cloud ......
Shane Hastie: Good day folks. This is Shane Hastie for the InfoQ Engineering Culture Podcast. Today I'm sitting down with Courtney Nash. C......
Weekly Updates - Feb 28, 2025
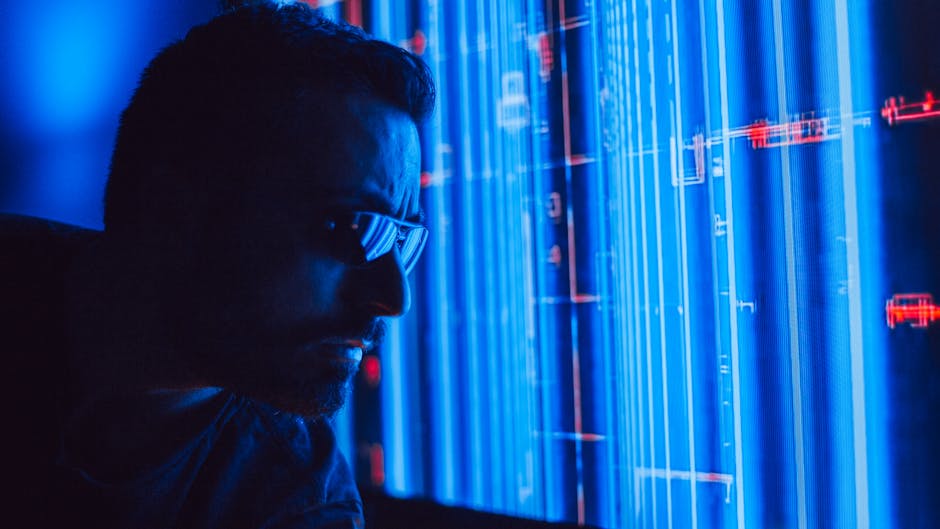
At Couchbase, ‘The Developer Data Platform for Critical Applications in Our AI World’, we have plenty to share with you on happenings in our ecosystem.
⭐ Announcing General Availability of the Quarkus SDK for Couchbase - We’re excited to announce the General Availability (GA) of the Couchbase Quarkus SDK [website], now officially ready for production use! This release brings native integration with the Quarkus framework, enhancing developer productivity and application performance. A standout feature of this release is support for GraalVM native image generation, enabling ultrafast startup times and optimized runtime performance. Learn more >>.
✔️ Integrate Groq’s Fast LLM Inferencing With Couchbase Vector Search - In this post Shivay Lamba explores how you integrate Groq’s fast LLM inferencing capabilities with Couchbase Vector Search to create fast and efficient RAG applications. He also compares the performance of different LLM solutions like OpenAI, Gemini and how they compare with Groq’s inference speeds. Find out more >>.
🤝 Couchbase and NVIDIA Team Up to Help Accelerate Agentic Application Development Couchbase is working with NVIDIA to help enterprises accelerate the development of agentic AI applications by adding support for NVIDIA AI Enterprise including its development tools, Neural Models framework (NeMo) and NVIDIA Inference Microservices (NIM). Capella adds support for NIM within its AI Model Services and adds access to the NVIDIA NeMo Framework for building, training, and tuning custom language models. The framework supports data curation, training, model customization, and RAG workflows for enterprises. Read on >>.
I’ve often presented that a beautiful desktop environment can make or break a distribution. Sure, there are plenty of people who don’t care what their desk......
Modern web development often involves multiple JavaScript files, dependencies from npm, and the need for efficient perform......
How Would You Design a Scalable and Maintainable Event Ticketing API?
I’m working on designing a mock event ticketing API, and I want ......
Orchestrate Cloud Native Workloads With Kro and Kubernetes
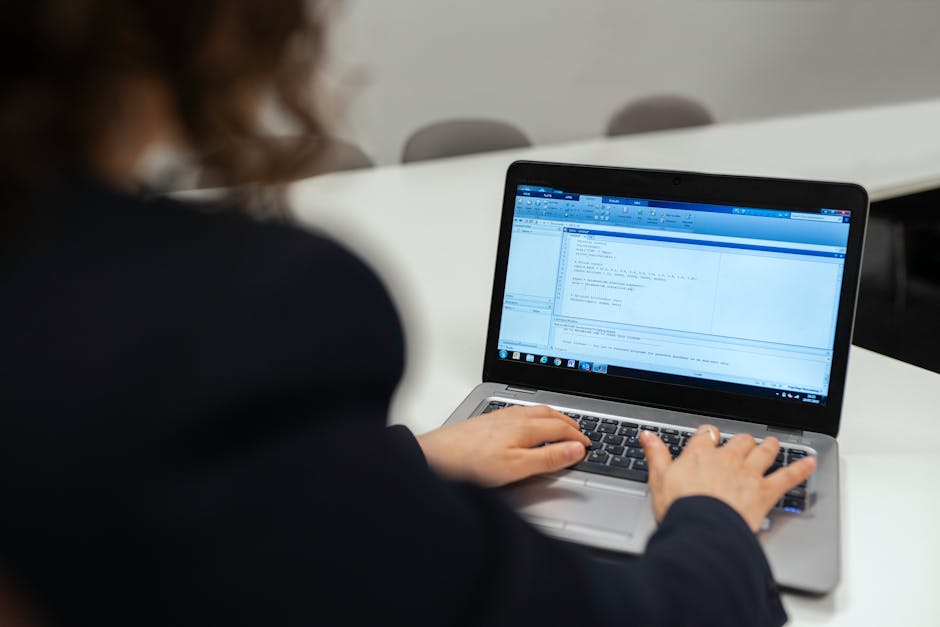
In the first part of this series, I introduced the background of Kube Resource Orchestrator (Kro). In this installment, we will define a Resource Graph Definition for WordPress and deploy multiple instances by creating them as Kro applications.
To understand and appreciate the power of Kro, imagine a managed hosting corporation specializing in deploying and managing WordPress sites for a diverse range of consumers — each with unique branding, custom domains and specific performance requirements. This corporation needs a consistent definition of WordPress deployment while changing only a few parameters per customer. Kro is a perfect match for this use case.
By leveraging RGD as a centralized blueprint for WordPress deployments, the firm can ensure that every site adheres to a consistent and optimized configuration while allowing individual customizations. This separation means the core setup — covering components like database configurations, persistent storage and ingress rules — is maintained in one robust, reusable definition, simplifying updates and security patches across all sites.
At the same time, individual application instances can be tailored with customer-specific settings such as unique credentials and custom domains, enabling rapid onboarding and reducing the risk of manual errors. This approach not only streamlines operations but also enhances scalability and reliability, making it easier for the hosting provider to manage a growing portfolio of WordPress sites efficiently.
This tutorial will define the WordPress workload as an RGD that encapsulates all the required Kubernetes resources, such as secrets, volumes, deployments, services and ingress. We will then define two instances representing different consumers or tenants of this hosting corporation.
For completeness, this tutorial has all the steps from start to finish to explore Kro.
curl -LO [website] sudo install minikube-darwin-arm64 /usr/local/bin/minikube 1 2 curl - LO https :// github . com / kubernetes / minikube / releases / latest / download / minikube - darwin - arm64 sudo install minikube - darwin - arm64 / usr / local / bin / minikube.
Let’s launch Minikube and configure storage and ingress. We will use Rancher Local Path as the storage provider.
minikube start minikube addons enable ingress 1 2 minikube start minikube addons enable ingress.
First, fetch the latest release version of Kro, and then install it as a Helm chart into its own namespace:
export KRO_VERSION=$(curl -sL \ [website] | \ jq -r '.tag_name | ltrimstr("v")' ) helm install kro oci://[website] \ --namespace kro \ --create-namespace \ --version=${KRO_VERSION} 1 2 3 4 5 6 7 8 9 export KRO_VERSION =$( curl - sL \ https :// api . github . com / repos / kro - run / kro / releases / latest | \ jq - r '.tag_name | ltrimstr("v")' ) helm install kro oci :// ghcr . io / kro - run / kro / kro \ -- namespace kro \ -- create - namespace \ -- version =${ KRO_VERSION }.
This will create a CRD in our Kubernetes cluster.
Step 3 — Deploy the WordPress Application With Kro.
Create a YAML file containing the ResourceGraphDefinition. This file aggregates all the Kubernetes objects required for a WordPress deployment, including MySQL components, PersistentVolumeClaims, Deployments, Services and optionally an Ingress resource:
In the above WordPress RGD, the definition is structured into two main parts: the schema and the resource templates.
The schema specifies key parameters for your WordPress deployment, such as the application name, the MySQL password (Base64 encoded), the storage class and whether an Ingress should be enabled. Operators can customize these values without directly editing multiple Kubernetes objects.
The resource templates then use these schema values to dynamically generate all necessary Kubernetes resources, including Secrets for storing MySQL credentials, PersistentVolumeClaims for both MySQL and WordPress data, Deployments and Services for running MySQL and WordPress pods, and optionally an Ingress for external access.
This unified approach simplifies the deployment process by aggregating multiple interdependent components into a single logical unit. It also ensures consistency and proper sequencing during resource creation. As a result, managing a complex application like WordPress becomes more efficient, predictable and less error-prone, as any changes to configuration parameters automatically propagate across all relevant resources.
The above step results in a new RGD called wp-app.
Step 4 — Deploy Two Application Instances.
Create another YAML file (for example, [website] that instantiates your ResourceGraphDefinition. Here, two WordPress applications are defined with custom names, MySQL passwords, storage settings and Ingress enabled:
Separating the RGD from individual application instances offers significant advantages, particularly for a managed hosting enterprise deploying WordPress sites for multiple clients with custom domains.
The individual application instances based on the RGD allow for customer-specific customizations, such as unique MySQL credentials, storage configurations and custom domain settings, without the need to modify the underlying blueprint. This separation simplifies maintenance, speeds up the onboarding process for new consumers and minimizes the risk of errors since the core configuration is defined once and then parameterized per instance.
Notice how we changed only the required parameters. If you want to extend this, change the Ingress hostname as a parameter.
After deploying the applications, they should become active and synchronized.
These applications are translated into various Kubernetes resources by the Kro controller.
We can access the WordPress sites after adding the HOST DNS entries and modifying the Header through an extension like Mod Header for Chrome. Don’t forget to launch Minikube Tunnel before accessing the sites.
I hope this tutorial gave you a comprehensive overview of Kro and the workflow involved in using it.
Around 30,000 websites and applications are hacked every day*, and the developer is often to blame.
The vast majority of breaches occur due to miscon......
Editor's Note: The following is an infographic written for and 's 2025 Trend research, Developer Experience: The Coalescence of Develo......
Whether you were building a web site or an application, hosting choices used to be about bandwidth, latency, security and availability (as well as cos......
Market Impact Analysis
Market Growth Trend
2018 | 2019 | 2020 | 2021 | 2022 | 2023 | 2024 |
---|---|---|---|---|---|---|
7.5% | 9.0% | 9.4% | 10.5% | 11.0% | 11.4% | 11.5% |
Quarterly Growth Rate
Q1 2024 | Q2 2024 | Q3 2024 | Q4 2024 |
---|---|---|---|
10.8% | 11.1% | 11.3% | 11.5% |
Market Segments and Growth Drivers
Segment | Market Share | Growth Rate |
---|---|---|
Enterprise Software | 38% | 10.8% |
Cloud Services | 31% | 17.5% |
Developer Tools | 14% | 9.3% |
Security Software | 12% | 13.2% |
Other Software | 5% | 7.5% |
Technology Maturity Curve
Different technologies within the ecosystem are at varying stages of maturity:
Competitive Landscape Analysis
Company | Market Share |
---|---|
Microsoft | 22.6% |
Oracle | 14.8% |
SAP | 12.5% |
Salesforce | 9.7% |
Adobe | 8.3% |
Future Outlook and Predictions
The Debug Code Github landscape is evolving rapidly, driven by technological advancements, changing threat vectors, and shifting business requirements. Based on current trends and expert analyses, we can anticipate several significant developments across different time horizons:
Year-by-Year Technology Evolution
Based on current trajectory and expert analyses, we can project the following development timeline:
Technology Maturity Curve
Different technologies within the ecosystem are at varying stages of maturity, influencing adoption timelines and investment priorities:
Innovation Trigger
- Generative AI for specialized domains
- Blockchain for supply chain verification
Peak of Inflated Expectations
- Digital twins for business processes
- Quantum-resistant cryptography
Trough of Disillusionment
- Consumer AR/VR applications
- General-purpose blockchain
Slope of Enlightenment
- AI-driven analytics
- Edge computing
Plateau of Productivity
- Cloud infrastructure
- Mobile applications
Technology Evolution Timeline
- Technology adoption accelerating across industries
- digital transformation initiatives becoming mainstream
- Significant transformation of business processes through advanced technologies
- new digital business models emerging
- Fundamental shifts in how technology integrates with business and society
- emergence of new technology paradigms
Expert Perspectives
Leading experts in the software dev sector provide diverse perspectives on how the landscape will evolve over the coming years:
"Technology transformation will continue to accelerate, creating both challenges and opportunities."
— Industry Expert
"Organizations must balance innovation with practical implementation to achieve meaningful results."
— Technology Analyst
"The most successful adopters will focus on business outcomes rather than technology for its own sake."
— Research Director
Areas of Expert Consensus
- Acceleration of Innovation: The pace of technological evolution will continue to increase
- Practical Integration: Focus will shift from proof-of-concept to operational deployment
- Human-Technology Partnership: Most effective implementations will optimize human-machine collaboration
- Regulatory Influence: Regulatory frameworks will increasingly shape technology development
Short-Term Outlook (1-2 Years)
In the immediate future, organizations will focus on implementing and optimizing currently available technologies to address pressing software dev challenges:
- Technology adoption accelerating across industries
- digital transformation initiatives becoming mainstream
These developments will be characterized by incremental improvements to existing frameworks rather than revolutionary changes, with emphasis on practical deployment and measurable outcomes.
Mid-Term Outlook (3-5 Years)
As technologies mature and organizations adapt, more substantial transformations will emerge in how security is approached and implemented:
- Significant transformation of business processes through advanced technologies
- new digital business models emerging
This period will see significant changes in security architecture and operational models, with increasing automation and integration between previously siloed security functions. Organizations will shift from reactive to proactive security postures.
Long-Term Outlook (5+ Years)
Looking further ahead, more fundamental shifts will reshape how cybersecurity is conceptualized and implemented across digital ecosystems:
- Fundamental shifts in how technology integrates with business and society
- emergence of new technology paradigms
These long-term developments will likely require significant technical breakthroughs, new regulatory frameworks, and evolution in how organizations approach security as a fundamental business function rather than a technical discipline.
Key Risk Factors and Uncertainties
Several critical factors could significantly impact the trajectory of software dev evolution:
Organizations should monitor these factors closely and develop contingency strategies to mitigate potential negative impacts on technology implementation timelines.
Alternative Future Scenarios
The evolution of technology can follow different paths depending on various factors including regulatory developments, investment trends, technological breakthroughs, and market adoption. We analyze three potential scenarios:
Optimistic Scenario
Rapid adoption of advanced technologies with significant business impact
Key Drivers: Supportive regulatory environment, significant research breakthroughs, strong market incentives, and rapid user adoption.
Probability: 25-30%
Base Case Scenario
Measured implementation with incremental improvements
Key Drivers: Balanced regulatory approach, steady technological progress, and selective implementation based on clear ROI.
Probability: 50-60%
Conservative Scenario
Technical and organizational barriers limiting effective adoption
Key Drivers: Restrictive regulations, technical limitations, implementation challenges, and risk-averse organizational cultures.
Probability: 15-20%
Scenario Comparison Matrix
Factor | Optimistic | Base Case | Conservative |
---|---|---|---|
Implementation Timeline | Accelerated | Steady | Delayed |
Market Adoption | Widespread | Selective | Limited |
Technology Evolution | Rapid | Progressive | Incremental |
Regulatory Environment | Supportive | Balanced | Restrictive |
Business Impact | Transformative | Significant | Modest |
Transformational Impact
Technology becoming increasingly embedded in all aspects of business operations. This evolution will necessitate significant changes in organizational structures, talent development, and strategic planning processes.
The convergence of multiple technological trends—including artificial intelligence, quantum computing, and ubiquitous connectivity—will create both unprecedented security challenges and innovative defensive capabilities.
Implementation Challenges
Technical complexity and organizational readiness remain key challenges. Organizations will need to develop comprehensive change management strategies to successfully navigate these transitions.
Regulatory uncertainty, particularly around emerging technologies like AI in security applications, will require flexible security architectures that can adapt to evolving compliance requirements.
Key Innovations to Watch
Artificial intelligence, distributed systems, and automation technologies leading innovation. Organizations should monitor these developments closely to maintain competitive advantages and effective security postures.
Strategic investments in research partnerships, technology pilots, and talent development will position forward-thinking organizations to leverage these innovations early in their development cycle.
Technical Glossary
Key technical terms and definitions to help understand the technologies discussed in this article.
Understanding the following technical concepts is essential for grasping the full implications of the security threats and defensive measures discussed in this article. These definitions provide context for both technical and non-technical readers.