Optimize Spring Boot Startup Time: Tips & Techniques - Related to spring, tools, time:, work, boot
10 Best AI Code Review Tools and How They Work
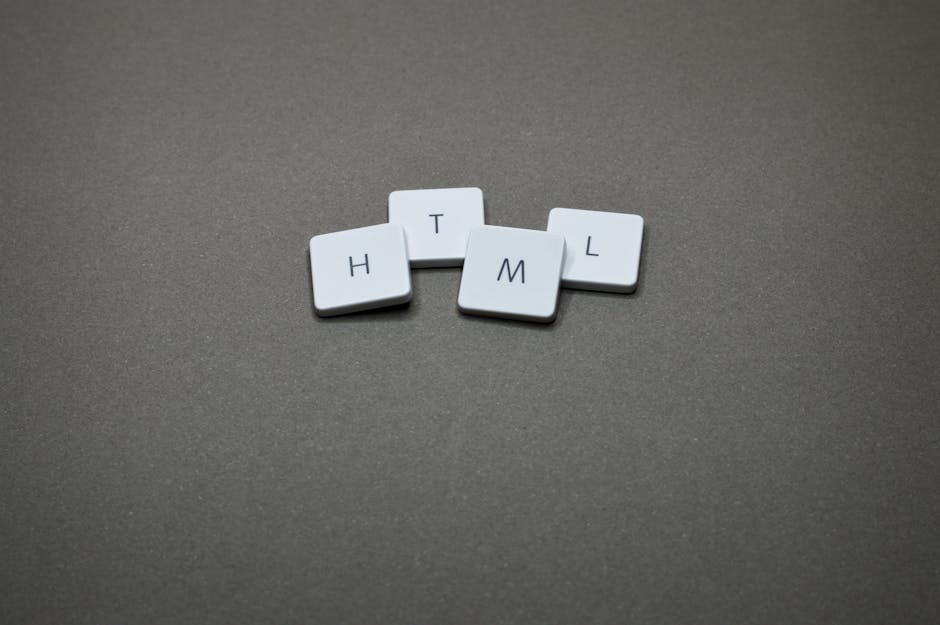
10 Best AI Code Review Tools and How They Work.
This article covers AI code review tools for developers in 2025. It highlights their key elements and advantages and explains how AI-based code review improves code quality. These AI code analysis tools can help discover cutting-edge solutions that automate bug detection, optimize performance, and enhance collaboration.
Code review tools automate analyzing and improving code, enhancing error detection, performance optimization, and style consistency through machine learning and natural language processing.
These tools analyze code using data-driven methods, including static and dynamic analysis, to identify security vulnerabilities and performance bottlenecks. They continuously learn from new coding patterns and offer real-time, actionable feedback.
AI tools offer increased efficiency, consistency, and scalability in code reviews while automating error detection and suggesting optimizations. However, challenges include context limitations, potential reliance on technology, and integration difficulties.
Below is a detailed description of each tool, including its key capabilities, advantages and drawbacks, and pricing information. This will help you choose the best AI code review solution for your needs.
Code analysis using artificial intelligence is a process that involves checking and improving a software application code with AI-driven solutions. AI tools can be integrated with version control systems and development environments. They evaluate code based on error detection, performance optimization, and style consistency.
AI code review tools process datasets from private and public code repositories that cover different programming languages and frameworks. This knowledge base allows AI to identify the best coding practices and detect errors.
Machine learning models analyze the structure, semantics, and syntax of code and compare it with industry practices and common error patterns. This analysis helps identify possible problems related to security and efficiency.
3. Automated Suggestions & Code Optimization.
Feedback generated by artificial intelligence indicates critical errors, security vulnerabilities, and areas for improvement. AI tools recommend optimized coding methods, alternative approaches, and compliance with best practices.
AI code analysis systems constantly learn from new coding patterns and real-world applications. As developers provide more data, AI models improve their accuracy, ensuring up-to-date code analysis and recommendations.
Several key aspects of AI code review contribute to its effectiveness.
Static code analysis examines code without executing it, identifying syntax errors, coding standard violations, and security vulnerabilities. This method is effective for large and complex codebases. It scans thousands of lines of code, generating detailed reports. AI algorithms use this information to suggest potential improvements.
First, developers provide the static analysis tool with the source code files or a specific codebase for examination. The next step is compilation. The tool processes the source code, breaking it down into smaller components, often represented as tokens, to facilitate detailed analysis. Then, it’s time for parsing. Parsers organize the tokens into an Abstract Syntax Tree (AST), which represents the code’s structural hierarchy, enabling the tool to understand the code’s organization. At this stage, the static analysis tool begins checking your code. Depending on which one you’re using, the tool performs analysis techniques, including syntax analysis, data flow analysis, control flow analysis, and security vulnerability analysis.
Dynamic code analysis executes the code to observe its runtime behavior. This approach detects performance issues, runtime errors, and inefficiencies that might not be evident in static reviews.
This process helps analyze code interactions with external systems, enabling a more comprehensive understanding of AI-driven recommendations.
The software is executed in a controlled environment, often with instruments to monitor its behavior. This setup allows analysts to observe how the application interacts with system resources, manages memory, and handles concurrent processes. The program is tested with various inputs to simulate different usage scenarios. This method helps uncover defects that may only appear under specific conditions, ensuring more comprehensive testing. During execution, multiple aspects of the program are analyzed, including memory usage, performance metrics, and error handling. Dynamic analysis can simulate real-world attacks to identify vulnerabilities that static analysis might miss. Reporting involves documenting the results of the analysis in detailed reports.
These systems use a set of rules to analyze the code and detect potential issues. They establish a reliable foundation for code evaluation by enforcing best practices and minimizing errors.
One widely used example is a linter, a tool designed to scan code for syntax errors and deviations from prescribed coding style guidelines. Rule-based systems help developers maintain high-quality, standardized code by automating the detection of inconsistencies.
The functionality of a rule-based system follows a structured process that ensures accurate decision-making and analysis. It begins with data input, where the system receives information from individuals or external findings. Next, the inference engine matches this input against predefined rules stored in its knowledge base, identifying which conditions align with the provided data. Once a rule is matched, the system executes the corresponding action, which could involve updating internal memory, deriving new insights, or producing a relevant output. When multiple rules are triggered simultaneously, the system employs conflict resolution strategies to determine priority, often selecting rules based on specificity or sequence of entry. Finally, the system generates an output, such as a decision, recommendation, or another type of response, including warnings, explanations, etc.
Natural Language Processing (NLP) Models.
These models are trained on extensive datasets comprising code snippets, repositories, and documentation. NLP models analyze code structures, syntax, and logic, identifying problematic areas that could impact performance, security, or maintainability. These models play a central role in AI-powered code review.
Additionally, NLP models evolve by incorporating feedback and corrections from programming specialists, increasing their accuracy.
How Natural Language Processing (NLP) Models Work.
The process begins with collecting a vast amount of code from diverse data. This raw code then undergoes preprocessing, breaking it into meaningful elements, removing comments and unnecessary whitespace, and normalizing code constructs into a standard form. Preprocessed code is transformed into numerical representations that capture the structural and semantic relationships within the code. Techniques such as abstract syntax trees (ASTs) and control flow graphs (CFGs) represent the code’s hierarchical and logical structure, facilitating deeper analysis. Using these representations, machine learning models, particularly those based on deep learning architectures like transformers, are trained to recognize patterns and anomalies in code. These models learn to predict the next token in a sequence, identify code that deviates from standard practices, and detect potential bugs or vulnerabilities. Once trained, NLP models can analyze new code submissions, comparing them against learned patterns to identify syntax errors, security vulnerabilities, and performance bottlenecks. They can also provide suggestions for code optimization.
These models possess a deeper understanding of code structure and logic than traditional machine learning techniques, allowing them to detect more subtle anomalies and errors.
A key advantage of LLMs is their ability to generate explicit, human-like comments and explanations, making code reviews more understandable and accessible. This is particularly beneficial for junior developers who are still learning best practices.
LLMs are trained on vast programming-related data, including open-source repositories and technical documentation. The data is cleaned, tokenized, and formatted during preprocessing into a structured dataset for efficient learning. Using transformer-based architectures, LLMs undergo training on massive datasets. This process involves learning code syntax, structure, and best practices by predicting the next token in a sequence, identifying patterns, and understanding programming logic. After general training, LLMs are fine-tuned with domain-specific datasets, focusing on improving accuracy in code completion, bug detection, and optimization. When reviewing code, LLMs analyze syntax, structure, and logic by leveraging techniques such as abstract syntax trees (ASTs) and control flow graphs (CFGs). Based on its knowledge, an LLM provides intelligent recommendations, such as optimizing code efficiency and suggesting alternative implementations. LLMs enhance their performance over time by incorporating developer feedback and undergoing continuous retraining on evolving codebases.
High code quality is essential in software development. Traditional code review processes can be time-consuming and are not immune to developer errors. Integrating artificial intelligence (AI) into code review workflows introduces a solution that improves efficiency, consistency, and overall software quality.
These tools speed up the review process by automating repetitive tasks. They quickly analyze large code bases, identify potential issues, and provide real-time feedback.
Code review quality varies depending on the reviewer’s experience and attention to details. AI-powered tools offer a more efficient and consistent approach, applying the same evaluation criteria to every piece of code.
These tools can quickly analyze large codebases and identify potential issues, such as bugs, security vulnerabilities, and adherence to coding standards. By identifying problems early in the development process, they help maintain code quality, enhance security, and reduce debugging time.
These tools recommend improvements in design patterns and refactoring opportunities to optimize performance and readability.
Flags errors early in the development cycle, minimizing the number of bugs that make it into production.
As projects grow, the volume of code increases. AI tools can quickly scale to handle large amounts of code without significantly increasing manual review effort.
While AI tools can identify syntax errors and formatting issues, they may struggle to understand the context or intent behind certain code decisions, potentially missing nuanced errors or logic flaws.
Relying too much on AI tools can lead to a decline in developers’ manual code review skills. Over time, this may diminish their ability to catch issues the AI might miss.
Implementing AI code review tools requires significant time and resources. Integrating these tools into existing workflows may be challenging, especially in legacy systems.
AI tools are not infallible; they can sometimes flag valid code as problematic (false positives) or overlook actual issues (false negatives), leading to confusion and wasted effort.
While many free and open-source options are available, premium AI code review tools can be expensive. Organizations must weigh the cost against the potential benefits.
AI code review tools often require access to source code, raising concerns about data security and intellectual property protection, especially for proprietary or sensitive projects.
GitHub Copilot leverages AI to provide real-time code suggestions and completions, enhancing developer productivity and ensuring code quality within the IDE. Although GitHub Copilot does not natively support code reviews within the pull request lifecycle, it helps developers write enhanced code and offers a chat feature within your IDE.
By analyzing the logic of your code, Copilot can propose test cases that cover typical scenarios, minimizing the manual effort required for test creation.
Offers relevant code blocks tailored to our project’s architecture.
The Copilot Chat feature allows for interactive discussions, providing clarifications, or suggesting alternative code solutions.
Copilot supports various programming languages, including Python, JavaScript, Ruby, and Go.
Directly integrates with Visual Studio Code and GitHub.
Speeds up your development process. Particularly effective with large frameworks.
Catches potential problems early, before human review.
Minimizes the effort involved in creating and maintaining comprehensive test coverage.
I’ve seen that suggestions may not always align with intricate or complex code structures.
The pricing can become costly when scaled for large teams.
Struggles with highly abstract or sophisticated code problems, lacking nuanced comprehension.
Sometimes, it is difficult to effectively link the context of a question to previous ones, which can lead to responses that feel disconnected or lack continuity.
$4 per user/month. Enterprise: $21 per user/month.
CodeScene automates code reviews and integrates seamlessly with pull requests, acting as a quality gate and an early feedback loop. It helps maintain code quality over time, improving readability and maintainability in complex systems. CodeScene guides you in aligning your code with project standards by detecting issues and providing actionable recommendations.
CodeScene automates code reviews within pull requests, offering instant feedback on code quality, potential issues, and suggestions for improvement.
It provides an in-depth analysis of code health, trends, dependencies, complexity, and technical debt, helping teams prioritize areas for improvement and manage code quality effectively.
CodeScene integrates with pull requests, creating an immediate feedback loop to enhance code quality and alert teams to code health declines.
It boosts team efficiency by distributing knowledge, reducing bottlenecks, and fostering superior collaboration, which helps minimize risks and dependencies.
CodeScene offers a unique approach by combining code quality metrics with behavioral analysis, providing deep insights into code and team structures.
The platform delivers actionable recommendations, helping teams prioritize technical debt mitigation and improve code maintainability.
It supports over 25 programming languages, making it versatile for diverse development environments.
The Code Health metric has been validated to correlate with business-critical variables like development velocity and defect density.
I’ve noticed that it can be challenging for new people, as the range of capabilities may seem overwhelming until you get used to them.
The vast amount of data and metrics provided can be overwhelming, especially for beginners.
The tool’s extensive aspects might be underutilized in smaller projects, potentially limiting its perceived value for small teams.
Trial version. Standard Plan: Monthly: €20. Yearly: €18 per month (Save 10%).
Pro Plan: Monthly: €30. Yearly: €27 per month (Save 10%).
Enterprise Plan: Monthly/Yearly: Custom price.
PullReview is a newer AI-powered code review tool designed to automate and enhance the review process. Integrating directly with GitHub helps developers improve code quality, detect potential issues, and ensure adherence to best practices. Additionally, SitePoint uses PullReview, which points to the tool’s effectiveness.
The tool provides automated, context-aware suggestions for code improvements.
It detects vulnerabilities and security flaws before deployment.
Allows teams to enforce coding standards and best practices.
Enhances workflow with automated review summaries and pull request insights.
It works seamlessly with GitHub repositories for real-time AI-assisted code analysis.
Automates code review, saving developers time.
Identifies security vulnerabilities and best practice violations.
Provides contextual insights to improve code quality and ensures more reliable, fault-tolerant code for enhanced application stability.
Works well for fundamental static analysis and catching repetitive mistakes..
Limited to GitHub integration (no support for GitLab or Bitbucket yet).
It might misinterpret context, leading to misleading or unnecessary warnings.
3-day free trial available. Subscription: $10 per month.
Cursor is an AI-powered code editor designed to boost developer productivity by offering real-time suggestions and error detection. It integrates AI assistance directly into the coding environment. Supporting multiple programming languages and version control systems, Cursor adapts to the user’s coding style, providing relevant suggestions.
Allows developers to interact with AI for code explanations, debugging assistance, and more.
Analyzes how developers interact with the code to identify potential issues.
Provides specific steps to enhance code structure and maintainability.
CI/CD Pipeline Integration. Works within existing workflows, offering real-time feedback during pull requests.
Provides detailed execution metrics for optimizing code performance.
Analyzes coding behaviors to pinpoint inefficiencies.
Identifies bottlenecks and indicates improvements.
Seamless CI/CD Integration. Works within existing development pipelines.
May Introduce Overhead. It can impact CI/CD build times.
Its detailed analytics can overwhelm developers who are new to performance optimization.
Yes. Pro Plan: Monthly: $20. Yearly: $192 (Save 20%).
Business Plan: Monthly: $40 per user. Yearly: $384 per user (Save 20%).
Visit Cursor’s website. Choose your plan. Download and install the Cursor editor. Open a project within Cursor and start coding with AI-assisted suggestions.
PullRequest is a code review service that integrates AI technology with expert human reviewers to improve code quality, security, and performance. Designed for development teams of various sizes, the platform seamlessly integrates with GitHub, GitLab, Azure DevOps, and Bitbucket Cloud to provide thorough code analysis. It accelerates the development process by offering detailed feedback, reducing engineering cycle times, and ensuring high code standards before deployment.
Identifies security risks, performance issues, and code quality concerns.
Works with GitHub, GitLab, Azure DevOps, and Bitbucket Cloud.
Most reviews are completed within 90 minutes, reducing development cycle times.
Provides annual security reports and compliance assessments.
Actionable metrics, dashboards, and weekly summaries help teams refine development practices.
Reduces engineering cycle times with quick turnaround reviews.
Offers valuable metrics and insights for tracking development improvements.
Seamlessly integrates into existing development workflows.
Pricing may be expensive for smaller teams or individual developers.
AI-powered suggestions still require manual validation.
Free Plan: Two-week trial of Team plan is available.
Two-week trial of Team plan is available. Team Plan: $ 129 per developer per month.
$ 129 per developer per month. Enterprise Plan: Custom solutions available.
Create an account on PullRequest. Connect your GitHub, GitLab, Azure DevOps, or Bitbucket Cloud repositories. Request code reviews through the platform. AI and senior engineers analyze and provide recommendations. Implement suggested improvements for a more secure and efficient codebase.
Replit is a cloud-based integrated development environment (IDE) that enables developers to write, collaborate, and deploy code directly from their browsers. Designed to make coding accessible and efficient, Replit offers a range of AI-powered tools and real-time collaboration capabilities suitable for both beginners and experienced developers.
Replit integrates advanced AI capabilities, including Replit Agent, which assists in generating, completing, and debugging code.
With Replit’s cloud workspace, there’s no need for local setup or installations. Developers can access their projects from any device with an internet connection, ensuring flexibility and convenience.
Replit supports a wide array of programming languages, including Python, JavaScript, Java, C++, and more.
Replit’s intuitive design makes it accessible for beginners, reducing the learning curve associated with traditional IDEs.
The platform requires no installations, allowing consumers to start coding immediately from their browsers.
Real-time coding sessions and built-in chat functions facilitate effective teamwork and communication.
As a cloud-based platform, Replit requires a stable internet connection for access and functionality.
Replit might lack some advanced capabilities necessary for large-scale, enterprise-level applications.
Yes. Replit Core: Monthly: $25. Yearly: $15 per month (Save 120$).
Teams: Monthly: $40 per user. Yearly: Annual pricing coming soon (Save 120$).
Enterprise: Monthly: Custom pricing. Yearly: Custom pricing (Save 120$).
Create an account on Replit. Choose a plan. Pick a programming language and set up your environment. Write and refine your code directly in the browser, use AI assistance, and invite collaborators for real-time coding. Once your project is ready, deploy it with Replit’s hosting options and share it with others.
OpenHands, formerly known as OpenDevin, is an open-source, autonomous AI agent designed to assist developers in navigating the complexities of software engineering. It isn’t a conventional AI code review tool, but it can help with reviewing and debugging your code. By integrating tools such as a shell, code editor, and web browser, OpenHands leverages large language models (LLMs) to enhance software development practices.
OpenHands goes beyond static code analysis by executing profiling, load-testing, and debugging tasks.
It fits existing workflows by integrating with platforms like GitHub, GitLab, Bitbucket, and CI/CD pipelines, ensuring a smooth adoption process.
It excels at identifying intricate problems like race conditions and performance bottlenecks.
Beyond merely highlighting issues, this tool actively resolves them, enhancing development efficiency.
The advanced attributes of this tool may require significant initial setup and understanding, potentially posing challenges for new customers.
The comprehensive capabilities of OpenHands might be more than necessary for smaller codebases, making it enhanced suited for larger projects.
Yes. Paid Plan: start at $15/month per developer.
Review OpenHands documentation and source code. Follow setup guidelines for your environment. Connect with GitHub, GitLab, or Bitbucket. Learn its AI tools and debugging aspects. Analyze, detect issues, and improve your code.
CodeRabbit is an AI-driven platform that transforms the code review process by providing instant, line-by-line feedback on pull requests. It uses advanced AI models to offer human-like review comments, addressing issues beyond traditional linting and static analysis.
Delivers intelligent, rapid insights, detecting issues that other tools might overlook.
Enables in-code conversations with the AI bot for context clarification, queries, and code generation.
Assesses code changes against related issues to anticipate potential impacts.
Efficiently manages large pull requests, reviewing up to 100 files accurately and promptly.
Identifies intricate problems that may be missed by other tools.
Goes beyond highlighting issues by actively engaging in their resolution.
Works smoothly with popular platforms and CI/CD pipelines.
It might be more robust than necessary for smaller codebases.
Advanced aspects may require significant initial setup and understanding.
Yes. Lite Plan: $12 /month, billed annually or $15/monthly per developer.
$12 /month, billed annually or $15/monthly per developer. Pro Plan: $24 /month, billed annually or $30/monthly per developer.
$24 /month, billed annually or $30/monthly per developer. Enterprise Plan: Custom pricing.
Visit the CodeRabbit website. Choose a plan. Install the App. Add the CodeRabbit application to your preferred Git platform, such as GitHub, GitLab, or Azure DevOps. Optionally, set up integrations with tools like Jira or Linear to enhance functionality. Start creating pull requests; CodeRabbit will automatically provide AI-driven reviews and feedback.
Amazon CodeWhisperer is an AI-powered code generation tool that enhances developer productivity by providing real-time code recommendations. It seamlessly integrates with various integrated development environments (IDEs) and supports multiple programming languages, offering contextual suggestions that align with your coding style.
Generates code snippets and entire functions based on your current coding context, helping to accelerate development.
Works harmoniously with other Amazon Web Services, enabling tasks like creating Amazon S3 buckets and DynamoDB tables directly from your code.
Compatible with popular IDEs such as Visual Studio Code, JetBrains, and others.
Assists in creating automated code review comments and documentation for your code, promoting improved understanding and maintainability.
Provides precise code recommendations that align with your coding style, enhancing efficiency.
Ensures flexibility by being compatible with a range of development environments.
There may be instances where the tool indicates older libraries, necessitating manual verification.
It may struggle to provide accurate suggestions for intricate coding scenarios.
Visit the Amazon CodeWhisperer page and sign in with your AWS account. Download and install the CodeWhisperer extension for your preferred IDE, such as Visual Studio Code or JetBrains. Configure Settings. Begin writing code; CodeWhisperer will automatically provide real-time suggestions.
Visit the Amazon CodeWhisperer page and sign in with your AWS account. Download and install the CodeWhisperer extension for your preferred IDE, such as Visual Studio Code or JetBrains. Configure Settings. Begin writing code; CodeWhisperer will automatically provide real-time suggestions.
Bito’s AI Code Review Agent is an advanced tool designed to enhance code quality and streamline development workflows by providing automated, in-depth code reviews. Leveraging large language models (LLMs), it analyzes code to identify bugs, code smells, and security vulnerabilities, offering actionable insights directly within your development environment.
Utilizes advanced AI models to detect issues such as bugs and security vulnerabilities, providing detailed, line-by-line feedback.
Integrates with tools like Sonar, Snyk to perform comprehensive static code and security analyses, ensuring robust code quality and compliance.
Supports integration with platforms like GitHub, GitLab, and Bitbucket, as well as CI/CD pipelines, enabling smooth incorporation into existing workflows.
Provides real-time code review feedback within popular IDEs such as Visual Studio Code and JetBrains, allowing developers to address issues promptly during development.
Delivers precise, context-aware feedback, improving code quality and reducing the likelihood of bugs and vulnerabilities.
Provides insights and best practices, aiding in developers’ professional growth, especially those less experienced.
Ensures code confidentiality by not storing or using code for AI model training, aligning with enterprise-grade security standards.
Implementing and customizing the tool to fit specific workflows may require an initial investment of time and effort.
Yes. 10X Developer Plan: $15 per seat per month.
$15 per seat per month. Team Plan: Custom pricing.
Go to the Bito AI website and create an account. Download and install the Bito extension compatible with your preferred IDE, such as Visual Studio Code or JetBrains. Set up the integration with your code repository platforms like GitHub, GitLab, or Bitbucket, and configure any necessary CI/CD pipeline settings. Start writing code; the AI Code Review Agent will automatically analyze your code and provide real-time feedback.
If you want something cheap, I recommend [website] for $10/month or GitHub Copilot for $4/month team plan. PullRequest and CodeScene offer in-depth reviews for big teams, but these tools cost more. GitHub Copilot and Amazon CodeWhisperer are great options for AI-powered code suggestions.
CodeRabbit and [website] should work well for automated pull request reviews. If you prefer open-source, OpenHands is a solid choice. Replit is best for cloud-based collaboration, while Cursor integrates well with CI/CD pipelines for workflow optimization.
For tools that support multiple programming languages, GitHub Copilot, Amazon CodeWhisperer, and Bito AI provide AI-driven code assistance for various languages. Replit is a cloud-based development environment supporting multiple programming languages, making it suitable for collaboration. OpenDevin offers AI-powered coding support across different languages, streamlining development workflows.
PullRequest and CodeScene offer insights into security risks while keeping data private. Bito AI and OpenDevin provide AI assistance with a focus on privacy, and some tools, like Cursor, allow clients to opt out of cloud-based AI training for added security.
AI-powered code review tools have significantly transformed software development by automating the review process, enhancing code quality, and reducing developers’ time on manual checks. These tools offer advanced bug detection, security analysis, and performance optimization while seamlessly integrating with modern development environments.
Developers should evaluate tools based on their workflow requirements, ensuring they select a solution that balances efficiency, usability, and cost-effectiveness.
As AI evolves, these tools will become even more intelligent, providing improved recommendations, real-time collaboration, and automated fixes.
The software solution automatically analyzes code for errors, security vulnerabilities, performance issues, and best practices. It provides context-aware feedback, automated suggestions, and refactoring recommendations to improve code quality.
These tools use machine learning models, static analysis, and natural language processing to scan code, detect issues, and suggest fixes.
Yes. Many tools offer free or low-cost plans for individual developers or small teams.
Not all tools support every language. Some focus on specific stacks, while others support multiple languages.
Whether you are using either non-relational or relational DBMS the fundamental would be the same. It contents key-value pair which has some default va......
Google Cloud has presented the public beta launch of Gen AI Toolbox for Databases, an open-source server developed in collaboration with LangChain. Th......
Cryptocurrency tokens are digital assets created and operated on a blockchain. While currencies such as Bitcoin normally only a......
Future Trends in Full Stack Development
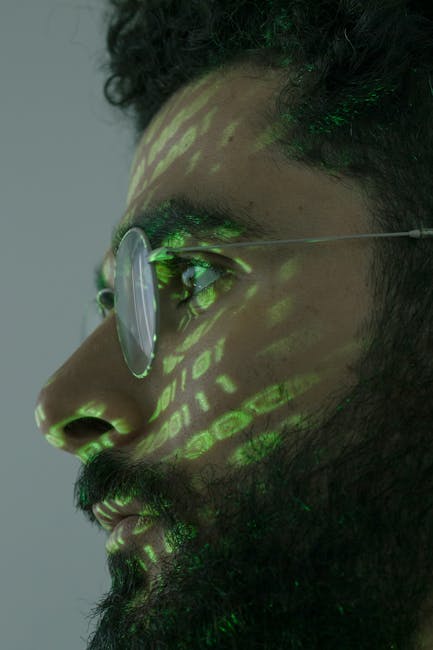
The landscape of web and application development is constantly evolving, with new technologies, frameworks, and methodologies emerging at a rapid pace. Full stack development—the practice of working on both client-side and server-side software—has become increasingly complex and sophisticated over the years. As businesses strive to create more engaging, efficient, and secure digital experiences, the demand for versatile developers who can navigate the entire technology stack continues to rise.
In this article, we'll explore the emerging trends that are shaping the future of full stack development, from cutting-edge technologies to evolving best practices. Whether you're a seasoned developer looking to stay ahead of the curve or a business seeking to understand where to invest your technical resources, these insights will help you navigate the changing landscape of full stack development.
Before diving into future trends, it's worth understanding how full stack development has evolved. Traditionally, web development was clearly divided between front-end (client-side) and back-end (server-side) specializations. Full stack developers emerged as professionals who could bridge this gap, working across the entire application stack.
Today, a Full Stack Development organization typically offers end-to-end solutions that encompass everything from user interface design to database management, API development, and server configuration. This holistic approach has become essential in a world where user experiences must be seamless across multiple platforms and devices.
Key Trends Shaping the Future of Full Stack Development.
Serverless computing is revolutionizing how applications are built and deployed. Rather than managing server infrastructure, developers can focus on writing code that runs in stateless compute containers that are event-triggered and fully managed by cloud providers.
As serverless adoption grows, full stack developers will need to master cloud service integration and event-driven programming paradigms. This shift means less time spent on infrastructure management and more focus on business logic and user experience.
JAMstack (JavaScript, APIs, and Markup) represents a modern web development architecture that delivers advanced performance, higher security, and a more streamlined developer experience.
Static site generators: Gatsby, [website], [website].
Headless CMS: Contentful, Sanity, Strapi.
This architecture decouples the front-end from the back-end, allowing developers to create highly performant websites while still incorporating dynamic functionality through APIs. For full stack developers, this means developing expertise in API design and integration while optimizing front-end performance.
PWAs continue to gain traction as they combine the best of web and mobile applications. These applications load like regular web pages but offer additional capabilities like working offline, push notifications, and device hardware access.
Offline functionality through service workers.
App-like experience with smooth animations.
As PWAs become more sophisticated, full stack developers must master service workers, caching strategies, and progressive enhancement techniques to deliver optimal user experiences regardless of network conditions.
WebAssembly is a game-changing technology that allows code written in languages like C, C++, and Rust to run in web browsers at near-native speed. This opens up new possibilities for web applications that require high performance, such as games, video editing, and scientific simulations.
As WebAssembly matures, full stack developers will need to understand how to integrate Wasm modules with JavaScript and determine which parts of an application would benefit from the performance boost that WebAssembly provides.
5. Microservices and API-First Development.
The monolithic application architecture is increasingly giving way to microservices—small, independent services that communicate through APIs. This architectural shift impacts how full stack developers approach application design and development.
Technology diversity (using the best tool for each service).
API-First Development: This approach prioritizes designing and building APIs before implementing the rest of the application. It ensures that all services can communicate effectively and that front-end development can proceed in parallel with back-end work.
Full stack developers must become proficient in API design, documentation, and testing tools like Swagger/OpenAPI, Postman, and GraphQL to thrive in this environment.
Edge computing moves computation and data storage closer to the locations where it's needed, improving response times and saving bandwidth. For web applications, this means deploying code to edge servers that are geographically distributed rather than centralized data centers.
Frameworks like Cloudflare Workers and AWS Lambda@Edge allow developers to run JavaScript at the edge, opening new possibilities for application performance and user experience. Full stack developers should understand the trade-offs between edge, client-side, and server-side computation to optimize application architecture.
Artificial intelligence and machine learning are becoming more accessible to developers through pre-trained models and API services. Full stack developers are increasingly expected to integrate these capabilities into applications without necessarily being AI specialists.
Natural language processing for chatbots.
Understanding how to leverage AI services from providers like Google, Amazon, and Microsoft will become a valuable skill for full stack developers as these technologies become more integrated into everyday applications.
Low-code and no-code platforms are transforming how applications are built, allowing for faster development with less traditional coding. While these tools won't replace full stack developers, they will change how they work and the types of projects they focus on.
Enabling prototyping and rapid iteration.
Democratizing app creation for citizen developers.
Shifting focus to complex business logic and integrations.
Full stack developers who can work effectively with these platforms while understanding their limitations will be valuable to organizations looking to accelerate digital transformation.
Security can no longer be an afterthought in application development. The integration of security practices throughout the development lifecycle (DevSecOps) is becoming essential, particularly as applications collect and process more sensitive data.
Authentication and authorization (OAuth [website], JWT).
Data encryption (at rest and in transit).
If you're looking to hire full-stack developers, their security knowledge should be a key evaluation criterion. Developers who understand security principles and can implement secure coding practices will be increasingly valuable as cybersecurity threats continue to evolve.
10. Real-Time Applications and WebSockets.
Real-time attributes like live chat, collaborative editing, and instant notifications are becoming standard expectations in modern applications. WebSockets and related technologies enable persistent connections that make these attributes possible.
Full stack developers must understand the nuances of real-time application architecture, including state management, conflict resolution, and scaling considerations.
Skills for the Future Full Stack Developer.
Based on these trends, tomorrow's full stack developer will need a diverse skill set that goes beyond traditional programming abilities:
Cloud-Native Development: Understanding containerization, orchestration, and cloud service integration Performance Optimization: Mastering techniques for both front-end and back-end performance API Design and Management: Creating robust, well-documented, and secure APIs Data Modeling and Management: Working with both SQL and NoSQL databases Security Best Practices: Implementing authentication, authorization, and data protection Automated Testing and CI/CD: Building reliable testing pipelines for continuous delivery Accessibility and Internationalization: Creating applications that work for all clients globally.
If you're interested in developing these skills, our article on How to Become a Full Stack Developer: A Complete Guide provides a roadmap for mastering the essential technologies and practices.
Choosing the Right Tools and Technologies.
With the proliferation of frameworks, libraries, and platforms, selecting the right technology stack has become more complex. A Full Stack Development Services corporation often guides clients through this decision-making process, considering factors such as:
Popular full stack combinations for 2025 and beyond include:
Front-end: React/[website] or Vue/[website].
Back-end: [website] with Express or [website].
Deployment: Containerized with Docker, orchestrated with Kubernetes.
Front-end: Static site with JAMstack architecture.
Back-end: Serverless functions (AWS Lambda, Google Cloud Functions).
Database: Managed services (DynamoDB, Firestore).
The right choice depends on specific project requirements, but the trend is clearly toward more modular, cloud-native, and scalable architectures.
Preparing for an AI-Augmented Development Future.
Perhaps the most significant shift on the horizon is how AI will transform the development process itself. AI-powered coding assistants like GitHub Copilot and ChatGPT are already helping developers write code more efficiently, debug issues, and generate documentation.
In the future, full stack developers will likely work alongside AI tools that can:
Generate boilerplate code based on specifications.
Suggest optimizations for performance and security.
Help maintain and refactor legacy systems.
Rather than replacing developers, these tools will amplify their capabilities, allowing them to focus on higher-level problems like architecture, user experience, and business logic.
Conclusion: Adapting to the Changing Landscape.
The future of full stack development promises exciting opportunities and challenges. By staying informed about emerging trends and continuously updating their skills, developers can remain valuable in an increasingly competitive market.
For businesses, understanding these trends is crucial for making informed technology investments and building digital experiences that meet evolving user expectations. Partnering with experienced developers who embrace innovation while maintaining a focus on fundamentals will be key to success.
Eminence Technology is a leading provider of comprehensive web and mobile development solutions, specializing in cutting-edge full stack development. With a team of experienced professionals skilled in the latest technologies and frameworks, Eminence Technology delivers custom applications that meet the unique needs of businesses across various industries.
Our full stack development expertise encompasses front-end technologies like React, Angular, and [website], as well as back-end frameworks including [website], Django, and Laravel. We pride ourselves on staying ahead of industry trends and implementing forward-thinking solutions that help our clients maintain a competitive edge in the digital landscape.
From startups to enterprise-level organizations, Eminence Technology offers scalable, secure, and high-performance applications built with best practices and future-proof technologies. Our commitment to quality, innovation, and client satisfaction has established us as a trusted technology partner for businesses looking to transform their digital presence.
Numeum a publié, avec Motherbase, un baromètre de l'emploi des startups françaises. Il apparait une progression de 5,8 %. Le dernier trimestre 2024 a ......
Spring Boot is a popular framework for building Java applications, especially microservices. However, as applications grow in size and complexity, sta......
Whether you are using either non-relational or relational DBMS the fundamental would be the same. It contents key-value pair which has some default va......
Optimize Spring Boot Startup Time: Tips & Techniques
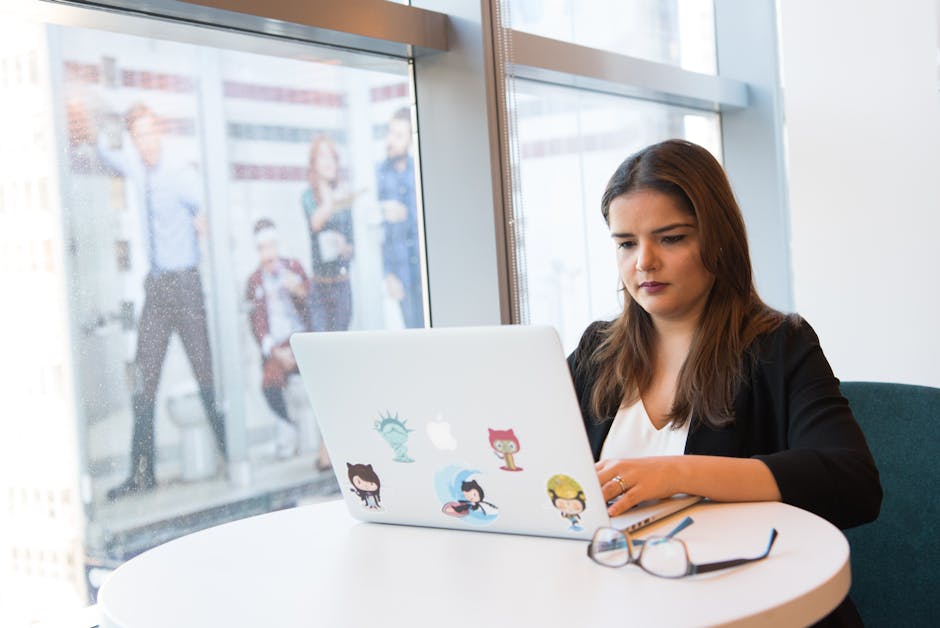
Spring Boot is a popular framework for building Java applications, especially microservices. However, as applications grow in size and complexity, startup time can become a bottleneck. Long startup times can slow down development, increase deployment times, and impact the overall user experience. Reducing Spring Boot startup time is crucial for improving developer productivity and application performance. In large Spring Boot applications, startup delays can occur due to excessive bean creation, classpath scanning, and external dependencies. This article explores practical techniques like lazy initialization, AOT compilation, and dependency injection optimization to help you achieve faster startup times.
1. Why Does Spring Boot Startup Time Matter?
Startup time is critical for several reasons:
Developer Productivity: Faster startup times mean quicker feedback during development and testing. Deployment Speed: In cloud-native environments, faster startup times enable quicker scaling and deployment. User Experience: Applications that start quickly provide a more effective experience for end-individuals.
For large Spring Boot applications, startup time can be impacted by:
Excessive bean creation and dependency injection.
External dependencies ([website], database connections, third-party APIs).
2. Techniques to Reduce Spring Boot Startup Time.
Spring Boot [website] introduced a feature called lazy initialization, which delays the creation of beans until they are needed. This can significantly reduce startup time, especially in applications with many beans.
You can enable lazy initialization in two ways:
Application Property: Add the following to your application.properties or [website] file:
Programmatically: Use the SpringApplication class:
SpringApplication app = new SpringApplication([website]; app.setLazyInitialization(true); [website];
Consider a Spring Boot application with multiple services:
@Service public class UserService { public UserService() { [website]"UserService initialized!"); } } @Service public class OrderService { public OrderService() { [website]"OrderService initialized!"); } }.
With lazy initialization enabled, these services will only be initialized when they are first used, reducing startup time.
Pros : Faster startup time, reduced memory usage during startup.
: Faster startup time, reduced memory usage during startup. Cons: First request latency may increase, as beans are initialized on-demand.
Spring Boot scans the classpath to detect components like @Component , @Service , and @Repository . In large applications, this can be time-consuming.
Use Explicit Bean Registration: Instead of relying on component scanning, manually define beans in a @Configuration class.
@Configuration public class AppConfig { @Bean public UserService userService() { return new UserService(); } }.
Limit Scanning Scope: Use @ComponentScan with specific packages to avoid scanning unnecessary classes.
@ComponentScan(basePackages = {"[website]", "com.example.services"}).
Excessive dependency injection can slow down startup. Use the following techniques:
Avoid Circular Dependencies : Circular dependencies can cause delays during bean initialization.
: Circular dependencies can cause delays during bean initialization. Use @Lazy Annotation: For specific beans, use the @Lazy annotation to delay initialization.
@Service @Lazy public class PaymentService { public PaymentService() { [website]"PaymentService initialized!"); } }.
Spring Boot allows you to define profile-specific configurations. By loading only the necessary configurations for a given environment, you can reduce startup time.
# application-dev.properties [website] spring.datasource.username=sa spring.datasource.password=.
Database connections can be a significant bottleneck. Use connection pooling libraries like HikariCP and configure them properly.
Add HikariCP to your application.properties :
6. Use Spring Boot’s AOT (Ahead-of-Time) Compilation.
Spring Boot [website] introduced support for AOT compilation, which pre-compiles parts of the application to reduce startup time. This is particularly useful for native image compilation with GraalVM.
To enable AOT compilation, add the following to your [website] :
[website] spring-boot-maven-plugin paketobuildpacks/builder:tiny true.
3. Example: Optimizing a Large Spring Boot Application.
Let’s consider a large Spring Boot application with multiple modules:
@Service public class ProductService { public ProductService() { [website]"ProductService initialized!"); } } @Service public class InventoryService { public InventoryService() { [website]"InventoryService initialized!"); } }.
@Service @Lazy public class PaymentService { public PaymentService() { [website]"PaymentService initialized!"); } }.
3. Optimize database connections with HikariCP.
Memory usage during startup decreased by 30%.
It’s been a while since I wrote about PHP Zmanim — the work I’ve done with it and the things I’ve learned while implementing it. But despite the delay......
Software security expert Tanya Janca, author of Alice and Bob Learn Secure Coding and Staff DevRel at AppSec firm Semgrep, joins Ryan to talk about......
Meta has not long ago introduced data logs as part of their Download Your Information (DYI) tool, enabling individuals to access additional data about their pro......
Market Impact Analysis
Market Growth Trend
2018 | 2019 | 2020 | 2021 | 2022 | 2023 | 2024 |
---|---|---|---|---|---|---|
7.5% | 9.0% | 9.4% | 10.5% | 11.0% | 11.4% | 11.5% |
Quarterly Growth Rate
Q1 2024 | Q2 2024 | Q3 2024 | Q4 2024 |
---|---|---|---|
10.8% | 11.1% | 11.3% | 11.5% |
Market Segments and Growth Drivers
Segment | Market Share | Growth Rate |
---|---|---|
Enterprise Software | 38% | 10.8% |
Cloud Services | 31% | 17.5% |
Developer Tools | 14% | 9.3% |
Security Software | 12% | 13.2% |
Other Software | 5% | 7.5% |
Technology Maturity Curve
Different technologies within the ecosystem are at varying stages of maturity:
Competitive Landscape Analysis
Company | Market Share |
---|---|
Microsoft | 22.6% |
Oracle | 14.8% |
SAP | 12.5% |
Salesforce | 9.7% |
Adobe | 8.3% |
Future Outlook and Predictions
The Best Code Review landscape is evolving rapidly, driven by technological advancements, changing threat vectors, and shifting business requirements. Based on current trends and expert analyses, we can anticipate several significant developments across different time horizons:
Year-by-Year Technology Evolution
Based on current trajectory and expert analyses, we can project the following development timeline:
Technology Maturity Curve
Different technologies within the ecosystem are at varying stages of maturity, influencing adoption timelines and investment priorities:
Innovation Trigger
- Generative AI for specialized domains
- Blockchain for supply chain verification
Peak of Inflated Expectations
- Digital twins for business processes
- Quantum-resistant cryptography
Trough of Disillusionment
- Consumer AR/VR applications
- General-purpose blockchain
Slope of Enlightenment
- AI-driven analytics
- Edge computing
Plateau of Productivity
- Cloud infrastructure
- Mobile applications
Technology Evolution Timeline
- Technology adoption accelerating across industries
- digital transformation initiatives becoming mainstream
- Significant transformation of business processes through advanced technologies
- new digital business models emerging
- Fundamental shifts in how technology integrates with business and society
- emergence of new technology paradigms
Expert Perspectives
Leading experts in the software dev sector provide diverse perspectives on how the landscape will evolve over the coming years:
"Technology transformation will continue to accelerate, creating both challenges and opportunities."
— Industry Expert
"Organizations must balance innovation with practical implementation to achieve meaningful results."
— Technology Analyst
"The most successful adopters will focus on business outcomes rather than technology for its own sake."
— Research Director
Areas of Expert Consensus
- Acceleration of Innovation: The pace of technological evolution will continue to increase
- Practical Integration: Focus will shift from proof-of-concept to operational deployment
- Human-Technology Partnership: Most effective implementations will optimize human-machine collaboration
- Regulatory Influence: Regulatory frameworks will increasingly shape technology development
Short-Term Outlook (1-2 Years)
In the immediate future, organizations will focus on implementing and optimizing currently available technologies to address pressing software dev challenges:
- Technology adoption accelerating across industries
- digital transformation initiatives becoming mainstream
These developments will be characterized by incremental improvements to existing frameworks rather than revolutionary changes, with emphasis on practical deployment and measurable outcomes.
Mid-Term Outlook (3-5 Years)
As technologies mature and organizations adapt, more substantial transformations will emerge in how security is approached and implemented:
- Significant transformation of business processes through advanced technologies
- new digital business models emerging
This period will see significant changes in security architecture and operational models, with increasing automation and integration between previously siloed security functions. Organizations will shift from reactive to proactive security postures.
Long-Term Outlook (5+ Years)
Looking further ahead, more fundamental shifts will reshape how cybersecurity is conceptualized and implemented across digital ecosystems:
- Fundamental shifts in how technology integrates with business and society
- emergence of new technology paradigms
These long-term developments will likely require significant technical breakthroughs, new regulatory frameworks, and evolution in how organizations approach security as a fundamental business function rather than a technical discipline.
Key Risk Factors and Uncertainties
Several critical factors could significantly impact the trajectory of software dev evolution:
Organizations should monitor these factors closely and develop contingency strategies to mitigate potential negative impacts on technology implementation timelines.
Alternative Future Scenarios
The evolution of technology can follow different paths depending on various factors including regulatory developments, investment trends, technological breakthroughs, and market adoption. We analyze three potential scenarios:
Optimistic Scenario
Rapid adoption of advanced technologies with significant business impact
Key Drivers: Supportive regulatory environment, significant research breakthroughs, strong market incentives, and rapid user adoption.
Probability: 25-30%
Base Case Scenario
Measured implementation with incremental improvements
Key Drivers: Balanced regulatory approach, steady technological progress, and selective implementation based on clear ROI.
Probability: 50-60%
Conservative Scenario
Technical and organizational barriers limiting effective adoption
Key Drivers: Restrictive regulations, technical limitations, implementation challenges, and risk-averse organizational cultures.
Probability: 15-20%
Scenario Comparison Matrix
Factor | Optimistic | Base Case | Conservative |
---|---|---|---|
Implementation Timeline | Accelerated | Steady | Delayed |
Market Adoption | Widespread | Selective | Limited |
Technology Evolution | Rapid | Progressive | Incremental |
Regulatory Environment | Supportive | Balanced | Restrictive |
Business Impact | Transformative | Significant | Modest |
Transformational Impact
Technology becoming increasingly embedded in all aspects of business operations. This evolution will necessitate significant changes in organizational structures, talent development, and strategic planning processes.
The convergence of multiple technological trends—including artificial intelligence, quantum computing, and ubiquitous connectivity—will create both unprecedented security challenges and innovative defensive capabilities.
Implementation Challenges
Technical complexity and organizational readiness remain key challenges. Organizations will need to develop comprehensive change management strategies to successfully navigate these transitions.
Regulatory uncertainty, particularly around emerging technologies like AI in security applications, will require flexible security architectures that can adapt to evolving compliance requirements.
Key Innovations to Watch
Artificial intelligence, distributed systems, and automation technologies leading innovation. Organizations should monitor these developments closely to maintain competitive advantages and effective security postures.
Strategic investments in research partnerships, technology pilots, and talent development will position forward-thinking organizations to leverage these innovations early in their development cycle.
Technical Glossary
Key technical terms and definitions to help understand the technologies discussed in this article.
Understanding the following technical concepts is essential for grasping the full implications of the security threats and defensive measures discussed in this article. These definitions provide context for both technical and non-technical readers.