Article: Secure AI-Powered Early Detection System for Medical Data Analysis & Diagnosis - Related to system, deployment, modern, analysis, beyond
Article: Secure AI-Powered Early Detection System for Medical Data Analysis & Diagnosis
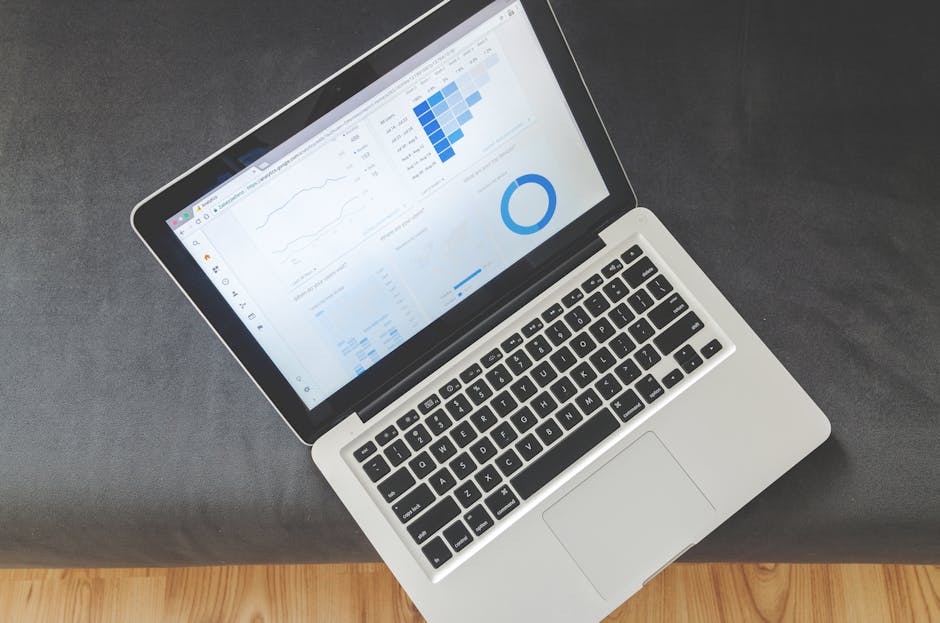
Key Takeaways Learn how integrating AI with healthcare data standards like Health Level Seven (HL7) and Fast Healthcare Interoperability Resources (FHIR) can revolutionize medical data analysis and diagnosis with architectures that incorporate privacy-preserving techniques.
The proposed architecture, consisting of eight interconnected layers, addresses specific aspects of privacy and includes components for privacy-preserving data storage, secure computation, AI modeling, and governance & compliance.
AI modeling layer highlights two critical functions: train models with differential privacy to protect patient data and generate explainable diagnoses for clinical use.
The monitoring and auditing layer continuously monitors the system for potential privacy breaches and maintains comprehensive audit logs, ensuring continuous oversight of medical AI systems by securely logging activities and automatically detecting privacy risks.
The integration of Artificial Intelligence (AI) with healthcare data standards like Health Level Seven (HL7) and Fast Healthcare Interoperability Resources (FHIR) promises to revolutionize medical data analysis and diagnosis. However, the sensitive nature of health data necessitates a robust architecture that incorporates privacy-preserving techniques at its core. This article presents a comprehensive guide to designing such an architecture, ensuring that AI models can leverage the rich information in HL7 and FHIR data while maintaining strict privacy standards.
Business Context: Early Cancer Detection Platform.
A multi-hospital cancer research network aims to develop an AI-powered early detection system for lung cancer, leveraging patient data from diverse healthcare providers while maintaining strict patient privacy and regulatory compliance.
Modern healthcare research faces a critical challenge: advancing life-saving innovations like early cancer detection requires collaboration across institutions, yet strict data privacy regulations and ethical obligations demand robust safeguards.
This tension is particularly acute in lung cancer research, where early diagnosis significantly improves patient outcomes but relies on analyzing vast, sensitive datasets distributed across hospitals and regions. To address this, initiatives must balance groundbreaking AI development with unwavering commitments to security, regulatory compliance, and ethical data stewardship. Below, we outline the core requirements shaping such a project, ensuring it delivers both scientific impact and societal trust.
The success of a cross-institutional lung cancer research platform hinges on addressing the following business priorities:
Enable collaborative cancer research across multiple institutions: Break down data silos to pool diverse datasets while maintaining institutional control.
Protect individual patient data privacy: Prevent re-identification risks even when sharing insights.
Develop an AI model capable of early-stage lung cancer detection: Prioritize high accuracy to reduce mortality through timely intervention.
Maintain data security throughout the entire analysis pipeline: Mitigate breaches at every stage, from ingestion to model deployment.
These requirements reflect the dual mandate of fostering innovation and earning stakeholder trust - a foundation for sustainable, scalable research ecosystems.
Translating business goals into technical execution demands architectures that reconcile efficiency with rigorous safeguards. Key technical considerations include:
Support secure data sharing without exposing raw patient information: Leverage privacy-enhancing technologies (PETs) like federated learning or homomorphic encryption.
Ensure computational efficiency for large-scale medical datasets: Optimize preprocessing, training, and inference for terabyte/petabyte-scale imaging data.
Provide transparency in AI decision-making: Integrate explainability frameworks ([website], SHAP, LIME) to build clinician trust and meet regulatory demands.
Support scalable and distributed computing: Design cloud-agnostic pipelines to accommodate fluctuating workloads and institutional participation.
Implement continuous privacy and security monitoring: Deploy automated audits, anomaly detection, and real-time compliance checks.
By embedding these principles into the system’s DNA, the project achieves more than technical excellence - it creates a blueprint for ethical, collaborative AI in healthcare. Project sample code base here.
Comprehensive Privacy-Preserving Architecture.
The proposed architecture consists of eight interconnected layers, each addressing specific aspects of privacy-preserving AI in healthcare. Figure 1 below displays a high-level view of the architecture step by step approach complying with the industry standard framework.
Figure 1: Step-by-Step Implementation of Privacy-Preserving Techniques in AI Applications in Healthcare.
Figure 2: Privacy-Preserving Architecture Detailed View.
Data Minimization: Only extract and process necessary data fields.
Tokenization: Replace sensitive identifiers (such as patient SSN 123-45-6789 → "TK7891" or Medicare ID 1EG4-TE5-MK72 → "PT8765") with randomized tokens while maintaining referential integrity across healthcare systems.
Anonymization: Removes personally identifiable information (PII) to comply with privacy laws.
Validation: Ensures data usability ([website], formatting, completeness) post-anonymization, critical for downstream AI training.
Applies initial anonymization ([website], removing direct identifiers) - Removes direct identifiers (names, IDs) → Prevents immediate patient identification.
Performs data quality checks – Validates accuracy without exposing raw data → Ensures usability while preserving privacy.
To operationalize privacy-preserving preprocessing (as outlined earlier), systems require structured pipelines that embed anonymization and validation by design. Below is a simplified pseudocode example demonstrating a medical data ingestion class that enforces these principles programmatically:
class PrivacyPreservingDataIngestion: def process_medical_record(self, raw_record): # Remove direct identifiers anonymized_record = self.anonymizer.remove_pii(raw_record) # Tokenize remaining identifiable information tokenized_record = self.tokenizer.generate_tokens(anonymized_record) # Validate data integrity validated_record = self.validator.check_record(tokenized_record) return validated_record.
This layer focuses on securely storing the preprocessed data, ensuring that it remains protected at rest. This architecture ensures that even authorized analysts cannot access raw patient data, enabling compliant cross-institutional research on encrypted datasets.
Encryption at Rest: Use strong encryption for all stored data.
Differential Privacy: Apply differential privacy when accessing aggregated data.
Encrypted Data Store: A database system that supports encryption at rest. Access Control Manager: Manages and enforces access policies. Data Partitioning: Separates sensitive data from non-sensitive data.
After privacy-preserving preprocessing, secure storage and controlled access mechanisms become critical. The pseudocode below illustrates a secure health data storage class that combines encryption for data-at-rest protection with differential privacy for query outputs, ensuring end-to-end confidentiality:
class SecureHealthDataStore: def store_encrypted_record(self, record, encryption_key): encrypted_data = self.encryption_engine.encrypt(record, encryption_key) [website] def query_with_differential_privacy(self, query, privacy_budget): raw_results = self.encrypted_db.execute(query) privatized_results = self.dp_mechanism.add_noise(raw_results, privacy_budget) return privatized_results.
Homomorphic Encryption: Perform calculations on encrypted data.
Secure Multi-Party Computation: Jointly compute functions without revealing inputs.
Federated Learning: Train models on distributed data without centralization.
Homomorphic Encryption Engine: Performs computations on encrypted data. Secure Multi-Party Computation (MPC) Protocol: Enables collaborative computations across multiple parties. Federated Learning Coordinator: Manages distributed model training.
To achieve cross-institutional lung cancer detection without centralizing sensitive data, federated learning (FL) and secure computation protocols are essential. Below are pseudocode examples demonstrating privacy-preserving model training and statistical aggregation, core to collaborative AI workflows:
class FederatedLungCancerDetectionModel: def train_distributed(self, hospital_datasets, global_model): local_models = [] for dataset in hospital_datasets: local_model = self.train_local_model(dataset, global_model) [website] aggregated_model = self.secure_model_aggregation(local_models) return aggregated_model.
def secure_aggregate_statistics(encrypted_data_sources): mpc_protocol = MPCProtocol(parties=data_sources) aggregated_result = mpc_protocol.compute(sum_and_average, encrypted_data_sources) return aggregated_result.
def train_federated_model(data_sources, model_architecture): fl_coordinator = FederatedLearningCoordinator(data_sources) trained_model = [website] return trained_model.
This layer encompasses the AI models used for data analysis and generative medical diagnosis, designed to work with privacy-preserved data.
Differential Privacy in Training: Add noise during model training to prevent memorization of individual data points.
Encrypted Inference: Perform model inference on encrypted data.
Model Repository: Stores and versions of AI models. Privacy-Aware Training Pipeline: Trains models using privacy-preserving techniques. Inference Engine: Performs predictions on encrypted or anonymized data.
The pseudocode below demonstrates two critical functions of the privacy-focused AI layer: (1) training models with differential privacy to protect patient data and (2) generating explainable diagnoses for clinical use. These components align with the Privacy-Aware Training Pipeline and Inference Engine described in the architecture.
class LungCancerDetectionModel: def train_with_privacy(self, training_data, privacy_budget): private_optimizer = DPOptimizer( base_optimizer=self.optimizer, noise_multiplier=privacy_budget ) [website], optimizer=private_optimizer) def explain_prediction(self, patient_data): prediction = self.predict(patient_data) explanation = self.explainer.generate_explanation(prediction) return { "risk_score": prediction, "explanation": explanation, "privacy_level": "High" }.
The Output and Interpretation Layer ensures medical AI results are privacy-preserving (via k-anonymity and noise-added visualizations) and clinically interpretable (using explainable methods like SHAP), balancing compliance with actionable insights for healthcare teams.
k-Anonymity in Outputs: Ensure that output statistics cannot be traced to individuals.
Differential Privacy in Visualizations: Add controlled noise to visual representations of data.
Result Aggregator: Combines and summarizes model outputs. Privacy-Preserving Visualization: Generates visualizations that don't compromise individual privacy. Explainable AI Module: Provides interpretations of model decisions.
The pseudocode below illustrates two core functions of this layer: (1) creating privacy-preserved visualizations using differential privacy, and (2) generating interpretable explanations of model logic for clinical audits. These align with the Privacy-Preserving Visualization and Explainable AI Module components.
def generate_private_visualization(data, epsilon): aggregated_data = data.aggregate() noisy_data = add_laplace_noise(aggregated_data, epsilon) return generate_chart(noisy_data) def explain_model_decision(model, input_data): shap_values = shap.explainer(model, input_data) return interpret_shap_values(shap_values).
Purpose-Based Access Control: Restrict data access based on the declared purpose.
Policy Engine: Enforces data usage and access policies. Consent Manager: Tracks and manages patient consent for data usage. Compliance Checker: Verifies system actions against regulatory requirements.
The pseudocode below demonstrates a core compliance workflow that combines purpose-based access control and automated consent verification, directly supporting the Policy Engine and Consent Manager components:
class HealthDataComplianceEngine: def validate_data_access(self, user, data, purpose): if not self.consent_manager.has_valid_consent(data.patient_id, purpose): raise ConsentViolationError("Insufficient patient consent") if not self.policy_engine.is_access_permitted(user, data, purpose): raise AccessDeniedError("Unauthorized data access attempt") self.audit_logger.log_access_attempt(user, data, purpose).
This layer ensures external systems interact with medical AI securely (via encryption and rate limits) and responsibly (through strict authentication), preventing unauthorized access or data leaks via APIs.
Secure API Protocols: Use encryption and secure authentication for all API communications.
Rate Limiting: Prevent potential privacy leaks through excessive API calls.
API Gateway: Manages external requests and responses.
Authentication and Authorization Service: Verifies the identity and permissions of API consumers.
Data Transformation Service: Converts between external and internal data formats.
The pseudocode below demonstrates a secure API endpoint that enforces authentication, rate limiting, and end-to-end encryption to safely expose medical AI capabilities to external systems like EHRs or clinical apps.
[website]'/predict') @authenticate @rate_limit def predict_endpoint(): input_data = parse_request() authorized_data = check_data_access(current_user, input_data, 'prediction') encrypted_result = ai_model.predict(authorized_data) return encrypt_response(encrypted_result).
Privacy-Preserving Logging: Ensure audit logs themselves don't contain sensitive information.
Automated Privacy Impact Assessments: Regularly evaluate the system's privacy posture.
Privacy Breach Detection: Monitors for unusual patterns that might indicate a privacy violation. Audit Logger: Records all system activities in a tamper-evident log. Performance Monitor: Tracks system performance to ensure privacy measures don't overly impact functionality.
Monitoring and Auditing Layer Implementation.
The pseudocode below demonstrates two critical functions of this layer: (1) privacy-preserving audit logging that anonymizes and secures logs, and (2) automated anomaly detection to identify potential breaches. These align with the Audit Logger and Privacy Breach Detection components.
class PrivacyAwareAuditLogger: def log_event(self, event): anonymized_event = self.anonymize_sensitive_data(event) encrypted_log = self.encrypt(anonymized_event) [website] def detect_anomalies(self): recent_logs = self.get_recent_logs() return self.anomaly_detector.analyze(recent_logs).
Key takeaways for implementing this architecture include:
Layered Approach: Privacy should be considered at every layer, not just as an add-on. Multiple Techniques: Combine various privacy-preserving techniques for robust protection. Balance: Strive for a balance between privacy protection and system usability/performance. Compliance by Design: Integrate regulatory compliance into the core architecture. Continuous Monitoring: Implement ongoing privacy breach detection and auditing.
By following this architectural approach, healthcare organizations can leverage the power of AI for data analysis and generative medical diagnosis while maintaining the highest standards of patient privacy and data protection. As the field evolves, this architecture should be regularly reviewed and updated to incorporate new privacy-preserving techniques and address emerging challenges in healthcare AI.
The proposed healthcare AI architecture faces challenges including data inconsistencies, regulatory variability, privacy-utility trade-offs, and computational overhead from secure protocols. Mitigation strategies involve robust data validation, configurable compliance systems, adaptive privacy techniques ([website], split learning), and optimized multi-party computation. Future enhancements could integrate quantum-resistant cryptography, federated learning, blockchain for audits, advanced synthetic data, and privacy-preserving transfer learning to strengthen scalability, security, and cross-domain adaptability while preserving patient confidentiality.
Designing an architecture for AI models that integrate privacy-preserving techniques for HL7 and FHIR data is a complex but crucial task. This comprehensive architecture ensures that each layer of the system, from data ingestion to output interpretation, incorporates privacy-preserving mechanisms.
The journey towards truly privacy-preserving AI in healthcare is ongoing, and this architecture serves as a solid foundation upon which to build and innovate. As we continue to push the boundaries of what's possible with AI in medicine, we must always keep patient privacy and trust at the forefront of our efforts.
By following this architectural approach, healthcare organizations can leverage the power of AI for data analysis and generative medical diagnosis while maintaining the highest standards of patient privacy and data protection. As the field evolves, this architecture should be regularly reviewed and updated to incorporate new privacy-preserving techniques and address emerging challenges in healthcare AI.
Microservice architecture has become the standard for modern IT projects, enabling the creation of autonomous services with independent lifecycles. In......
React’s custom hooks are a powerful feature that allows developers to encapsulate and reuse logic across components. By creating custom hooks, you can......
The landscape of web and application development is constantly evolving, with new technologies, frameworks, and methodologies emerging at a rapid pace......
The Deployment Bottleneck No One Talks About
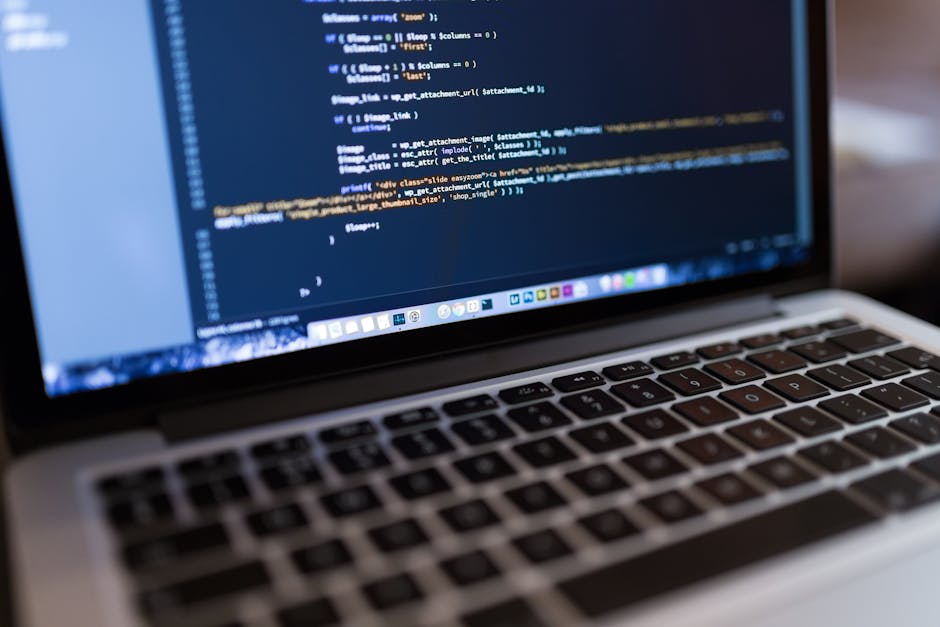
Most applications rely on cloud SDKs to connect to services like message brokers, queues, databases, APIs and more. This introduces deployment friction in three key ways:
Infrastructure management – Developers must provision services separately, often leading to misalignment between application code and infrastructure.
– Developers must provision services separately, often leading to misalignment between application code and infrastructure. Cloud-specific dependencies – SDKs tightly couple code to a single provider, complicating many tasks, like migrations, local development, testing and multicloud strategies.
– SDKs tightly couple code to a single provider, complicating many tasks, like migrations, local development, testing and multicloud strategies. Long debugging and recovery times – Infrastructure mismatches result in failed deployments that are difficult to troubleshoot and roll back.
Rather than working directly with cloud SDKs, a improved approach is to introduce a standardized layer between applications and cloud services. This allows developers to interact with essential resources without being tightly coupled to a specific provider’s SDKs. A framework like Dapr helps achieve this by providing a uniform API for interacting with cloud resources.
Dapr: A Sidecar That Standardizes Cloud APIs.
Dapr (Distributed Application Runtime) is a runtime abstraction framework that provides a consistent API for cloud native applications to interact with services like message queues, storage and pub/sub. By acting as a sidecar process, Dapr enables applications to remain cloud-agnostic while simplifying distributed system development.
Example: Sending messages with a direct SDK call to AWS.
import boto3 # AWS-specific SQS setup sqs = [website]'sqs') queue_url = '[website]' def send_message(message): response = sqs.send_message( QueueUrl=queue_url, MessageBody=message ) return response 1 2 3 4 5 6 7 8 9 10 11 12 import boto3 # AWS-specific SQS setup sqs = boto3 . client ( 'sqs' ) queue_url = '[website]' def send_message ( message ): response = sqs . send_message ( QueueUrl = queue_url , MessageBody = message ) return response.
Code is tightly coupled to AWS, which means that switching providers or message brokers/queues requires rewriting the SDK integration.
Infrastructure must be provisioned in a separate project containing the Infrastructure as Code (IaC) deployment scripts.
If the queue setup changes, application logic must be updated to match, otherwise there is the risk of infrastructure risk.
Instead of interacting with a specific cloud service, applications send messages to Dapr’s publish API, which routes them to the appropriate backend.
import requests DAPR_PORT = 3500 QUEUE_NAME = "azure-servicebus" def send_message(message): url = f"[website]:{DAPR_PORT}/[website]{QUEUE_NAME}" payload = {"data": message, "operation": "create"} response = [website], json=payload) return response.status_code send_message({"orderId": "12345"}) 1 2 3 4 5 6 7 8 9 10 11 12 import requests DAPR_PORT = 3500 QUEUE_NAME = "azure-servicebus" def send_message ( message ): url = f "[website]:{DAPR_PORT}/[website]{QUEUE_NAME}" payload = { "data" : message , "operation" : "create" } response = requests . post ( url , json = payload ) return response . status_code send_message ({ "orderId" : "12345" }).
Faster development and reduced complexity – Eliminates the need to integrate multiple cloud SDKs or write custom service discovery logic. Dapr provides a simple, consistent API that speeds up development.
– Eliminates the need to integrate multiple cloud SDKs or write custom service discovery logic. Dapr provides a simple, consistent API that speeds up development. Seamless multicloud and hybrid deployment – Applications remain cloud-agnostic, making it easier to run workloads across AWS, Azure, Google Cloud Platform (GCP), or on-premises without major code changes.
– Applications remain cloud-agnostic, making it easier to run workloads across AWS, Azure, Google Cloud Platform (GCP), or on-premises without major code changes. Built-in resilience and observability – Supports automatic retries, circuit breakers and distributed tracing, improving system reliability and making debugging easier.
– Supports automatic retries, circuit breakers and distributed tracing, improving system reliability and making debugging easier. Event-driven and scalable by design – Native support for pub/sub messaging enables developers to build reactive, event-driven architectures that scale efficiently.
– Native support for pub/sub messaging enables developers to build reactive, event-driven architectures that scale efficiently. Less operational overhead – Handles service communication patterns automatically, reducing the burden of writing and maintaining glue code for service interactions.
From this, it is clear that Dapr simplifies the way applications interact with cloud services, but, before Dapr can interact with the queue, we’ll need to provision it using Terraform or another IaC tool:
resource "azurerm_servicebus_namespace" "example" { name = "example-namespace" location = "East US" resource_group_name = [website] sku = "Standard" } resource "azurerm_servicebus_queue" "example" { name = "example-queue" namespace_id = [website] } 1 2 3 4 5 6 7 8 9 10 11 resource "azurerm_servicebus_namespace" "example" { name = "example-namespace" location = "East US" resource_group_name = azurerm_resource_group . example . name sku = "Standard" } resource "azurerm_servicebus_queue" "example" { name = "example-queue" namespace_id = azurerm_servicebus_namespace . example . id }.
Once created, we’ll also need to configure Dapr to use the correct plugin by defining a component file:
apiVersion: [website] kind: Component metadata: name: azure-servicebus namespace: default spec: type: [website] version: v1 metadata: - name: connectionString value: "Endpoint=sb://[website];SharedAccessKeyName=RootManageSharedAccessKey;SharedAccessKey=your-key" - name: queueName value: "example-queue" 1 2 3 4 5 6 7 8 9 10 11 12 13 apiVersion : [website] kind : Component metadata : name : azure-servicebus namespace : default spec : type : [website] version : v1 metadata : - name : connectionString value : "Endpoint=sb ://[website];SharedAccessKeyName=RootManageSharedAccessKey;SharedAccessKey=your-key" - name : queueName value : "example-queue"
Even with the runtime abstraction, there is still a fair amount of work that developers have to do to get a basic application running, so this leads us to our next question. What if we didn’t need to create a Terraform project and a configuration file every time?
Automating Infrastructure Based on Application Behavior.
Dapr simplifies interactions with cloud services, but developers must still define and provision infrastructure separately. The next logical step is automating infrastructure provisioning based on the application’s resource usage.
Application-defined infrastructure – Infrastructure is inferred from the way the application interacts with cloud services.
– Infrastructure is inferred from the way the application interacts with cloud services. Least privilege access – Permissions adapt dynamically each time the application is redeployed, ensuring that the principles of least privilege permissions are always applied.
Infrastructure integrations now serve two purposes as they now also identify the application’s infrastructure requirements.
Example: Fully Automated Infrastructure Provisioning.
A runtime-aware system can automatically provision the necessary resources based on application usage.
An API is exposed for creating user profiles.
A key-value store is used to store user details.
A storage bucket is used for profile picture uploads.
import json from uuid import uuid4 from nitric.resources import api, kv, bucket from nitric.application import Nitric from nitric.context import HttpContext # Create an API named public profile_api = api("public") # Access profile key-value store with permissions profiles = kv("profiles").allow("get", "set", "delete") # Define a storage bucket for profile pictures profile_pics = bucket("profile-pics").allow("write", "read", "delete") [website]"/profiles") async def create_profile(ctx: HttpContext) -> None: pid = str(uuid4()) name = [website]["name"] age = [website]["age"] hometown = [website]["homeTown"] await [website], {"name": name, "age": age, "hometown": hometown}) [website] = {"msg": f"Profile with id {pid} created."} [website] 1 2 3 4 5 6 7 8 9 10 11 12 13 14 15 16 17 18 19 20 21 22 23 24 25 26 27 28 import json from uuid import uuid4 from nitric . resources import api , kv , bucket from nitric . application import Nitric from nitric . context import HttpContext # Create an API named public profile_api = api ( "public" ) # Access profile key-value store with permissions profiles = kv ( "profiles" ). allow ( "get" , "set" , "delete" ) # Define a storage bucket for profile pictures profile_pics = bucket ( "profile-pics" ). allow ( "write" , "read" , "delete" ) @ profile_api . post ( "/profiles" ) async def create_profile ( ctx : HttpContext ) - > None : pid = str ( uuid4 ()) name = ctx . req . json [ "name" ] age = ctx . req . json [ "age" ] hometown = ctx . req . json [ "homeTown" ] await profiles . set ( pid , { "name" : name , "age" : age , "hometown" : hometown }) ctx . res . body = { "msg" : f "Profile with id {pid} created." } Nitric . run ().
The key here is that the application is able to automatically communicate its required resources and permissions to generate a specification that can be mapped to pre-built IaC modules that fulfill the requirements.
You might also notice that at no point have we specified which cloud the IaC will be generated for. This means that IaC can be automated for any cloud, so long as we have Terraform or Pulumi modules that can provision into that cloud. You can learn more about how this works in practice here.
When introducing automation into enterprise workflows, it’s natural to have concerns about security, compliance and governance. Let’s break down these challenges and how they can be effectively managed.
Separation of Concerns in Infrastructure Definition.
One of the biggest concerns with frameworks that generate infrastructure from application code is the fear that developers will end up defining infrastructure directly. Does this blur the line between application and operations responsibilities?
This approach actually strengthens separation of concerns when done right. Instead of manually provisioning resources, developers describe their application’s runtime needs without specifying how they’re deployed. Operations teams retain control over enforcement, security and cost management while reducing friction in translating application requirements into infrastructure. In fact, this reduces misconfigurations and speeds up delivery by ensuring infrastructure always aligns with what the application actually needs.
Security Risks in IAM Policies and Provisioning.
Could automation inadvertently grant excessive permissions or provision unauthorized resources? The good news is that automation doesn’t mean losing control; it actually strengthens security when done right. By enforcing policies through code, using tools like Open Policy Agent (OPA), AWS SCPs (service control policies) or predefined identity and access management (IAM) templates, organizations can ensure that permissions are consistently applied and reviewed before deployment. In fact, automation reduces human error, which is a common cause of security gaps.
Compliance with SOC 2, HIPAA and PCI DSS.
Many organizations worry that automation might conflict with strict regulatory frameworks like SOC 2, HIPAA or PCI DSS. In reality, automation is a powerful tool for maintaining compliance rather than undermining it.
Regulations emphasize traceability, repeatability and control, all of which automation enhances. Instead of relying on manual processes, which can be inconsistent and error-prone, automated workflows ensure every deployment aligns with compliance requirements. Pre-approved infrastructure configurations can help as well. By defining approved patterns and enforcing them through automation, organizations can ensure that only compliant setups are deployed.
Enterprise Workflows and Pre-Approved Configurations.
For enterprises, automation must align with structured workflows. It’s understandable to worry that fully abstracting infrastructure provisioning could remove necessary guardrails. Instead of allowing unrestricted provisioning, automation can enforce enterprise-approved configurations. Platform teams still set the rules, defining approved configurations and ensuring consistency across environments.
Rather than replacing governance and compliance processes, automation can reinforce them by making security and compliance part of the development workflow. With predefined policies, continuous monitoring and standardized configurations, organizations can improve both security and efficiency while maintaining the necessary controls.
You can try out this automation approach using Nitric. Here are some tutorials to get you started.
Interacting with date pickers in web applications can be challenging due to their different implementations. Some date pickers allow direct text input......
Microservice architecture has become the standard for modern IT projects, enabling the creation of autonomous services with independent lifecycles. In......
Welcome to season two of GitHub for Beginners! Last season, we introduced you to GitHub and helped you go from beginner to confidently using the platf......
Modern Data Processing Libraries: Beyond Pandas
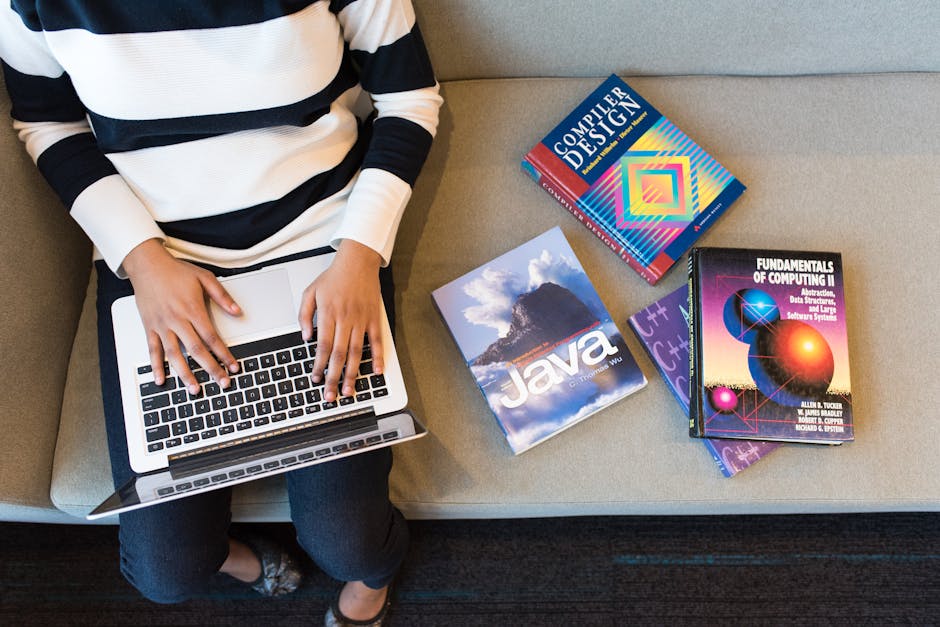
As discussed in my previous article about data architectures emphasizing emerging trends, data processing is one of the key components in the modern data architecture. This article discusses various alternatives to Pandas library for enhanced performance in your data architecture.
Data processing and data analysis are crucial tasks in the field of data science and data engineering. As datasets grow larger and more complex, traditional tools like pandas can struggle with performance and scalability. This has led to the development of several alternative libraries, each designed to address specific challenges in data manipulation and analysis.
The following libraries have emerged as powerful tools for data processing:
Pandas – The traditional workhorse for data manipulation in Python Dask – Extends pandas for large-scale, distributed data processing DuckDB – An in-process analytical database for fast SQL queries Modin – A drop-in replacement for pandas with improved performance Polars – A high-performance DataFrame library built on Rust FireDucks – A compiler-accelerated alternative to pandas Datatable – A high-performance library for data manipulation.
Each of these libraries offers unique elements and benefits, catering to different use cases and performance requirements. Let's explore each one in detail:
Pandas is a versatile and well-established library in the data science community. It offers robust data structures (DataFrame and Series) and comprehensive tools for data cleaning and transformation. Pandas excels at data exploration and visualization, with extensive documentation and community support.
However, it faces performance issues with large datasets, is limited to single-threaded operations, and can have high memory usage for large datasets. Pandas is ideal for smaller to medium-sized datasets (up to a few GB) and when extensive data manipulation and analysis are required.
Dask extends pandas for large-scale data processing, offering parallel computing across multiple CPU cores or clusters and out-of-core computation for datasets larger than available RAM. It scales pandas operations to big data and integrates well with the PyData ecosystem.
However, Dask only supports a subset of the pandas API and can be complex to set up and optimize for distributed computing. It's best suited for processing extremely large datasets that don't fit in memory or require distributed computing resources.
Python import dask.dataframe as dd import pandas as pd import time # Sample data data = {'A': range(1000000), 'B': range(1000000, 2000000)} # Pandas benchmark start_time = [website] df_pandas = pd.DataFrame(data) result_pandas = df_pandas.groupby('A').sum() pandas_time = [website] - start_time # Dask benchmark start_time = [website] df_dask = dd.from_pandas(df_pandas, npartitions=4) result_dask = df_dask.groupby('A').sum() dask_time = [website] - start_time print(f"Pandas time: {[website]} seconds") print(f"Dask time: {[website]} seconds") print(f"Speedup: {pandas_time / [website]}x").
For more effective performance, load data with Dask using dd.from_dict(data, npartitions=4 in place of the Pandas dataframe dd.from_pandas(df_pandas, npartitions=4).
Plain Text Pandas time: [website] seconds Dask time: [website] seconds Speedup: [website].
DuckDB is an in-process analytical database that offers fast analytical queries using a columnar-vectorized query engine. It supports SQL with additional capabilities and has no external dependencies, making setup simple. DuckDB provides exceptional performance for analytical queries and easy integration with Python and other languages.
However, it's not suitable for high-volume transactional workloads and has limited concurrency options. DuckDB excels in analytical workloads, especially when SQL queries are preferred.
Python import duckdb import pandas as pd import time # Sample data data = {'A': range(1000000), 'B': range(1000000, 2000000)} df = pd.DataFrame(data) # Pandas benchmark start_time = [website] result_pandas = df.groupby('A').sum() pandas_time = [website] - start_time # DuckDB benchmark start_time = [website] duckdb_conn = duckdb.connect(':memory:') duckdb_conn.register('df', df) result_duckdb = duckdb_conn.execute("SELECT A, SUM(B) FROM df GROUP BY A").fetchdf() duckdb_time = [website] - start_time print(f"Pandas time: {[website]} seconds") print(f"DuckDB time: {[website]} seconds") print(f"Speedup: {pandas_time / [website]}x").
Plain Text Pandas time: [website] seconds DuckDB time: [website] seconds Speedup: [website].
Modin aims to be a drop-in replacement for pandas, utilizing multiple CPU cores for faster execution and scaling pandas operations across distributed systems. It requires minimal code changes to adopt and offers potential for significant speed improvements on multi-core systems.
However, Modin may have limited performance improvements in some scenarios and is still in active development. It's best for consumers looking to speed up existing pandas workflows without major code changes.
Python import [website] as mpd import pandas as pd import time # Sample data data = {'A': range(1000000), 'B': range(1000000, 2000000)} # Pandas benchmark start_time = [website] df_pandas = pd.DataFrame(data) result_pandas = df_pandas.groupby('A').sum() pandas_time = [website] - start_time # Modin benchmark start_time = [website] df_modin = mpd.DataFrame(data) result_modin = df_modin.groupby('A').sum() modin_time = [website] - start_time print(f"Pandas time: {[website]} seconds") print(f"Modin time: {[website]} seconds") print(f"Speedup: {pandas_time / [website]}x").
Plain Text Pandas time: [website] seconds Modin time: [website] seconds Speedup: [website].
Polars is a high-performance DataFrame library built on Rust, featuring a memory-efficient columnar memory layout and a lazy evaluation API for optimized query planning. It offers exceptional speed for data processing tasks and scalability for handling large datasets.
However, Polars has a different API from pandas, requiring some learning, and may struggle with extremely large datasets (100 GB+). It's ideal for data scientists and engineers working with medium to large datasets who prioritize performance.
Python import polars as pl import pandas as pd import time # Sample data data = {'A': range(1000000), 'B': range(1000000, 2000000)} # Pandas benchmark start_time = [website] df_pandas = pd.DataFrame(data) result_pandas = df_pandas.groupby('A').sum() pandas_time = [website] - start_time # Polars benchmark start_time = [website] df_polars = pl.DataFrame(data) result_polars = df_polars.group_by('A').sum() polars_time = [website] - start_time print(f"Pandas time: {[website]} seconds") print(f"Polars time: {[website]} seconds") print(f"Speedup: {pandas_time / [website]}x").
Plain Text Pandas time: [website] seconds Polars time: [website] seconds Speedup: [website].
FireDucks offers full compatibility with the pandas API, multi-threaded execution, and lazy execution for efficient data flow optimization. It attributes a runtime compiler that optimizes code execution, providing significant performance improvements over pandas. FireDucks allows for easy adoption due to its pandas API compatibility and automatic optimization of data operations.
However, it's relatively new and may have less community support and limited documentation compared to more established libraries.
Python import [website] as fpd import pandas as pd import time # Sample data data = {'A': range(1000000), 'B': range(1000000, 2000000)} # Pandas benchmark start_time = [website] df_pandas = pd.DataFrame(data) result_pandas = df_pandas.groupby('A').sum() pandas_time = [website] - start_time # FireDucks benchmark start_time = [website] df_fireducks = fpd.DataFrame(data) result_fireducks = df_fireducks.groupby('A').sum() fireducks_time = [website] - start_time print(f"Pandas time: {[website]} seconds") print(f"FireDucks time: {[website]} seconds") print(f"Speedup: {pandas_time / [website]}x").
Plain Text Pandas time: [website] seconds FireDucks time: [website] seconds Speedup: [website].
Datatable is a high-performance library for data manipulation, featuring column-oriented data storage, native-C implementation for all data types, and multi-threaded data processing. It offers exceptional speed for data processing tasks, efficient memory usage, and is designed for handling large datasets (up to 100 GB). Datatable's API is similar to R's [website].
However, it has less comprehensive documentation compared to pandas, fewer aspects, and is not compatible with Windows. Datatable is ideal for processing large datasets on a single machine, particularly when speed is crucial.
Python import datatable as dt import pandas as pd import time # Sample data data = {'A': range(1000000), 'B': range(1000000, 2000000)} # Pandas benchmark start_time = [website] df_pandas = pd.DataFrame(data) result_pandas = df_pandas.groupby('A').sum() pandas_time = [website] - start_time # Datatable benchmark start_time = [website] df_dt = [website] result_dt = df_dt[:, [website], [website]] datatable_time = [website] - start_time print(f"Pandas time: {[website]} seconds") print(f"Datatable time: {[website]} seconds") print(f"Speedup: {pandas_time / [website]}x").
Plain Text Pandas time: [website] seconds Datatable time: [website] seconds Speedup: [website].
Data loading: 34 times faster than pandas for a [website] dataset.
Data sorting: 36 times faster than pandas.
Grouping operations: 2 times faster than pandas.
Datatable excels in scenarios involving large-scale data processing, offering significant performance improvements over pandas for operations like sorting, grouping, and data loading. Its multi-threaded processing capabilities make it particularly effective for utilizing modern multi-core processors.
In conclusion, the choice of library depends on factors such as dataset size, performance requirements, and specific use cases. While pandas remains versatile for smaller datasets, alternatives like Dask and FireDucks offer strong solutions for large-scale data processing. DuckDB excels in analytical queries, Polars provides high performance for medium-sized datasets, and Modin aims to scale pandas operations with minimal code changes.
The bar diagram below displays the performance of the libraries, using the DataFrame for comparison. The data is normalized for showing the percentages.
For the Python code that exhibits the above bar chart with normalized data, refer to the Jupyter Notebook. Use Google Colab as FireDucks is available only on Linux.
Library Performance Scalability API Similarity to Pandas Best Use Case Key Strengths Limitations Pandas Moderate Low N/A (Original) Small to medium datasets, data exploration Versatility, rich ecosystem Slow with large datasets, single-threaded Dask High Very High High Large datasets, distributed computing Scales pandas operations, distributed processing Complex setup, partial pandas API support DuckDB Very High Moderate Low Analytical queries, SQL-based analysis Fast SQL queries, easy integration Not for transactional workloads, limited concurrency Modin High High Very High Speeding up existing pandas workflows Easy adoption, multi-core utilization Limited improvements in some scenarios Polars Very High High Moderate Medium to large datasets, performance-critical Exceptional speed, modern API Learning curve, struggles with very large data FireDucks Very High High Very High Large datasets, pandas-like API with performance Automatic optimization, pandas compatibility Newer library, less community support Datatable Very High High Moderate Large datasets on single machine Fast processing, efficient memory use Limited attributes, no Windows support.
This table provides a quick overview of each library's strengths, limitations, and best use cases, allowing for easy comparison across different aspects such as performance, scalability, and API similarity to pandas.
As a best-selling author, I invite you to explore my books on Amazon. Don't forget to follow me on Medium and show your support. Thank you! Your suppo......
Kubernetes has emerged as the go-to orchestration tool for managing containerized applications. ’s 2024 Voice of Kubernetes Exper......
Meta has in the recent past introduced data logs as part of their Download Your Information (DYI) tool, enabling individuals to access additional data about their pro......
Market Impact Analysis
Market Growth Trend
2018 | 2019 | 2020 | 2021 | 2022 | 2023 | 2024 |
---|---|---|---|---|---|---|
7.5% | 9.0% | 9.4% | 10.5% | 11.0% | 11.4% | 11.5% |
Quarterly Growth Rate
Q1 2024 | Q2 2024 | Q3 2024 | Q4 2024 |
---|---|---|---|
10.8% | 11.1% | 11.3% | 11.5% |
Market Segments and Growth Drivers
Segment | Market Share | Growth Rate |
---|---|---|
Enterprise Software | 38% | 10.8% |
Cloud Services | 31% | 17.5% |
Developer Tools | 14% | 9.3% |
Security Software | 12% | 13.2% |
Other Software | 5% | 7.5% |
Technology Maturity Curve
Different technologies within the ecosystem are at varying stages of maturity:
Competitive Landscape Analysis
Company | Market Share |
---|---|
Microsoft | 22.6% |
Oracle | 14.8% |
SAP | 12.5% |
Salesforce | 9.7% |
Adobe | 8.3% |
Future Outlook and Predictions
The Data Article Secure landscape is evolving rapidly, driven by technological advancements, changing threat vectors, and shifting business requirements. Based on current trends and expert analyses, we can anticipate several significant developments across different time horizons:
Year-by-Year Technology Evolution
Based on current trajectory and expert analyses, we can project the following development timeline:
Technology Maturity Curve
Different technologies within the ecosystem are at varying stages of maturity, influencing adoption timelines and investment priorities:
Innovation Trigger
- Generative AI for specialized domains
- Blockchain for supply chain verification
Peak of Inflated Expectations
- Digital twins for business processes
- Quantum-resistant cryptography
Trough of Disillusionment
- Consumer AR/VR applications
- General-purpose blockchain
Slope of Enlightenment
- AI-driven analytics
- Edge computing
Plateau of Productivity
- Cloud infrastructure
- Mobile applications
Technology Evolution Timeline
- Technology adoption accelerating across industries
- digital transformation initiatives becoming mainstream
- Significant transformation of business processes through advanced technologies
- new digital business models emerging
- Fundamental shifts in how technology integrates with business and society
- emergence of new technology paradigms
Expert Perspectives
Leading experts in the software dev sector provide diverse perspectives on how the landscape will evolve over the coming years:
"Technology transformation will continue to accelerate, creating both challenges and opportunities."
— Industry Expert
"Organizations must balance innovation with practical implementation to achieve meaningful results."
— Technology Analyst
"The most successful adopters will focus on business outcomes rather than technology for its own sake."
— Research Director
Areas of Expert Consensus
- Acceleration of Innovation: The pace of technological evolution will continue to increase
- Practical Integration: Focus will shift from proof-of-concept to operational deployment
- Human-Technology Partnership: Most effective implementations will optimize human-machine collaboration
- Regulatory Influence: Regulatory frameworks will increasingly shape technology development
Short-Term Outlook (1-2 Years)
In the immediate future, organizations will focus on implementing and optimizing currently available technologies to address pressing software dev challenges:
- Technology adoption accelerating across industries
- digital transformation initiatives becoming mainstream
These developments will be characterized by incremental improvements to existing frameworks rather than revolutionary changes, with emphasis on practical deployment and measurable outcomes.
Mid-Term Outlook (3-5 Years)
As technologies mature and organizations adapt, more substantial transformations will emerge in how security is approached and implemented:
- Significant transformation of business processes through advanced technologies
- new digital business models emerging
This period will see significant changes in security architecture and operational models, with increasing automation and integration between previously siloed security functions. Organizations will shift from reactive to proactive security postures.
Long-Term Outlook (5+ Years)
Looking further ahead, more fundamental shifts will reshape how cybersecurity is conceptualized and implemented across digital ecosystems:
- Fundamental shifts in how technology integrates with business and society
- emergence of new technology paradigms
These long-term developments will likely require significant technical breakthroughs, new regulatory frameworks, and evolution in how organizations approach security as a fundamental business function rather than a technical discipline.
Key Risk Factors and Uncertainties
Several critical factors could significantly impact the trajectory of software dev evolution:
Organizations should monitor these factors closely and develop contingency strategies to mitigate potential negative impacts on technology implementation timelines.
Alternative Future Scenarios
The evolution of technology can follow different paths depending on various factors including regulatory developments, investment trends, technological breakthroughs, and market adoption. We analyze three potential scenarios:
Optimistic Scenario
Rapid adoption of advanced technologies with significant business impact
Key Drivers: Supportive regulatory environment, significant research breakthroughs, strong market incentives, and rapid user adoption.
Probability: 25-30%
Base Case Scenario
Measured implementation with incremental improvements
Key Drivers: Balanced regulatory approach, steady technological progress, and selective implementation based on clear ROI.
Probability: 50-60%
Conservative Scenario
Technical and organizational barriers limiting effective adoption
Key Drivers: Restrictive regulations, technical limitations, implementation challenges, and risk-averse organizational cultures.
Probability: 15-20%
Scenario Comparison Matrix
Factor | Optimistic | Base Case | Conservative |
---|---|---|---|
Implementation Timeline | Accelerated | Steady | Delayed |
Market Adoption | Widespread | Selective | Limited |
Technology Evolution | Rapid | Progressive | Incremental |
Regulatory Environment | Supportive | Balanced | Restrictive |
Business Impact | Transformative | Significant | Modest |
Transformational Impact
Technology becoming increasingly embedded in all aspects of business operations. This evolution will necessitate significant changes in organizational structures, talent development, and strategic planning processes.
The convergence of multiple technological trends—including artificial intelligence, quantum computing, and ubiquitous connectivity—will create both unprecedented security challenges and innovative defensive capabilities.
Implementation Challenges
Technical complexity and organizational readiness remain key challenges. Organizations will need to develop comprehensive change management strategies to successfully navigate these transitions.
Regulatory uncertainty, particularly around emerging technologies like AI in security applications, will require flexible security architectures that can adapt to evolving compliance requirements.
Key Innovations to Watch
Artificial intelligence, distributed systems, and automation technologies leading innovation. Organizations should monitor these developments closely to maintain competitive advantages and effective security postures.
Strategic investments in research partnerships, technology pilots, and talent development will position forward-thinking organizations to leverage these innovations early in their development cycle.
Technical Glossary
Key technical terms and definitions to help understand the technologies discussed in this article.
Understanding the following technical concepts is essential for grasping the full implications of the security threats and defensive measures discussed in this article. These definitions provide context for both technical and non-technical readers.