LatentView Wins Ten GenAI Projects, Secures Key $3.2M Deal with 37.5% YoY Revenue Growth - Related to revenue, growth, yoy, key, how
From 220M data points to revenue: How AI is transforming sports entertainment ROI
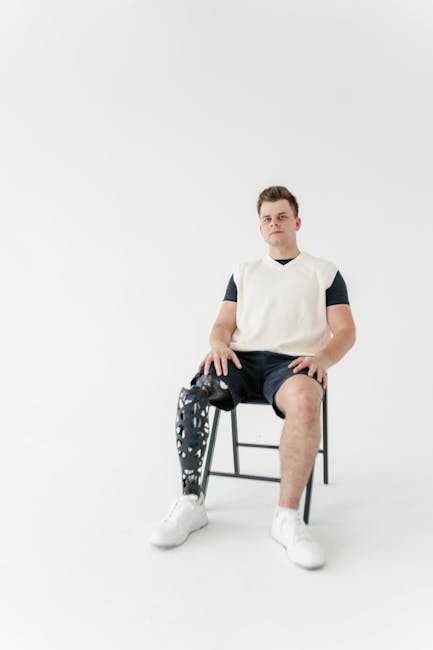
The Super Bowl is one of the largest sports entertainment events on the planet, bringing in more than a hundred million viewers and a billion in revenue.
But for NFL teams and sports entertainment in general, there is a long road to championship as franchises aim to build brand, grow fandom and maximize revenues.
One of the ways to make that happen is AI.
The technology is no stranger to the world of sports entertainment. Predating the modern era of generative AI — as far back as 2017 — big vendors like IBM were already discussing how AI would disrupt sport entertainment networks. The NFL itself is using AI to help improve player safety with a Digital Athlete system developed in partnership with AWS. The NFL is also using AWS to build gen AI-powered apps using the Amazon MemoryDB database.
For individual teams, both in the NFL and across the sports entertainment landscape, there are other options for implementing gen AI. One such option, launching today, comes from Elevate, a technology vendor led by Al Guido, who is also the president of the San Francisco 49ers NFL football team.
The corporation’s new Elevate performance and insights cloud (EPIC) data and AI platform combines consumer insights, ticketing management and property analytics to help sports and entertainment organizations engage advanced with fans. The platform helps organizations with targeted engagement efforts to advanced understand potential customer personas. That information helps determine stadium seating options, ticket pricing and fan retention. The platform has already been used by more than 25 organizations, including the Tennessee Titans.
Elevate has been in operation since 2018, but now with the advent of gen AI, the organization is able to do much more with data.
“Building EPIC has reinforced a fundamental truth that we’ve seen and validated with our clients since we’ve been in operation — data is only as powerful as the decisions it enables,” Guido, Elevat’s chairman and CEO, told VentureBeat. “In sports, the challenge isn’t just capturing that data but harnessing it to drive real, actionable intelligence that improves fan engagement, revenue strategies and operational efficiency.”.
The data challenges of building an AI-first engagement system.
Elevate already has data for approximately 220 million people in its system. The corporation collects first-party data through its client work and relationships. This includes data on fan behavior, ticket sales, sponsorships and other property-related information. Elevate also licenses and purchases third-party data sets to further enrich user profiles.
Guido noted that many organizations collect what seems like infinite amounts of data, but they struggle to unify and leverage it. EPIC was designed to bridge that gap.
To fully benefit from modern gen AI, data should be in a vector database format, Elevate contends. CIO Jim Caruso explained to VentureBeat that his organization has undergone an intensive process to not only vectorize data, but to make sure it’s the right data to help inform business decisions.
There is no shortage of database vendors and technologies that claim to make vectorizing data simple. In reality, Caruso stressed that the vectorization process isn’t as simple as turning on a switch. As part of building EPIC, they reevaluated all data and how it could work together to provide the best insights. The actual vectorization process involved testing different approaches and processing pipelines to find the right balance of accuracy and performance.
Currently, Elevate uses Amazon Sagemaker to make its vectorization work.
How Anthopic Claude, XGBoost and Amazon Bedrock help to power AI insights for EPIC.
Caruso explained that the EPIC system provides a wide range of AI-powered applications, from pricing tickets to developing consumer insights personas. Elevate is using a combination of different technologies to build those tools.
At the core is the Anthropic Claude Haiku [website] large language model (LLM), which has been fine-tuned on Elevate’s data. Claude provides the interface to ask questions and get insights based on different personas.
For example, one persona could be a venue operator that wants to determine the best way to configure premium seating in a venue. That operator will need to understand who would be interested in those seats and how they should be marketed to different groups.
Elevate went beyond just identifying broad demographic segments, like suburban millennials. Instead, they created a series of distinct personas with a range of attributes including finances, buying preferences, entertainment choices and social networking engagement. The key goal is to provide very concrete, detailed personas that enable organizations to make specific business decisions.
The system also uses the XGBoost (eXtreme Gradient Boosting) open-source machine learning (ML) library via Amazon Sagemaker to specifically help with numerical data for ticket pricing. XGBoost is a supervised ML algorithm that uses decision trees to make predictions. Caruso explained that his team converted historical data, as well as real-time data, into 55 different elements. These include event details, inventory details and recent sales information. All were then then fed into the XGBoost algorithm.
The competitive landscape for AI across sports entertainment.
Guido noted that across the NFL and beyond, the initial response to EPIC has been positive.
Many properties face similar challenges: fragmented data insights, evolving fan expectations and the need for smarter, more efficient revenue generation. Guido also clearly recognizes that the competitive landscape for this kind of technology is expanding. There are traditional customer relationship management (CRM) and analytics providers, like Salesforce, but in his view, they often lack the industry-specific intelligence that EPIC brings to sports and live entertainment.
“What sets EPIC apart is its deep integration with the realities of sports,” noted Guido.
How AI-powered insights are driving real-world impact for the Tennessee Titans.
Among the early customers of EPIC is the NFL’s Tennessee Titans. The team is working with Elevate as it develops a new $[website] billion stadium set to open in 2027.
As part of the engagement, Elevate has helped lead sponsorship sales for the new stadium. The corporation developed a strategic partnership revenue roadmap, a category-specific go-to-market strategy and set annual sales goals through the stadium’s launch.
With EPIC, the Titans have been able to build out detailed personas for fans to inform targeted marketing strategies, from messaging to premium seating and hospitality offerings. Although the new stadium is still several years away from opening, the Titans have been able to exceed sales targets for premium seating already, with data and AI-powered insights as the foundation.
It’s not just for the NFL; college athletics are also benefiting from AI-powered insights.
While there is big money in the NFL, there is also a lot of opportunity (as well as many challenges) at other levels of sports entertainment, including colleges.
“University athletic departments are undergoing a profound digital transformation, and data is at the center of it,” Tom Moreland, chief commercial officer at the University of Illinois Athletics, told VentureBeat. “One of the biggest lessons we’ve learned is that technology alone isn’t the solution — strategy comes first.”.
Moreland explained that his school has been prioritizing how it collects, interprets and applies data to enhance the experiences of its coaches, student-athletes, and fans.
So far, the EPIC platform has provided University of Illinois Athletics with the crucial data-driven insights required to improve football and men’s basketball ticketing, as well as an annual giving model. Moreland noted that the EPIC analysis provided intelligence that enabled the school to move beyond assumptions and make strategic, informed decisions. Ultimately, he noted, EPIC empowered his department to create a more engaging and sustainable model for loyal fans and donors.
“Athletic departments that take the time to invest in data quality, structure and application will be the ones that truly benefit from any new technology,” introduced Moreland.
Après l’incident qu’a fait objet DeepSeek la semaine dernière, les chercheurs affirment avoir trouvé un moyen de contourner les mesures de protection ...
John Schulman, cofondateur d’OpenAI et ancien chercheur d’Anthropic, quitte la startup d’intelligence artificielle. Son départ marque un tournant impo...
Vous êtes en Europe et vous utilisez l’IA pour vos tâches au quotidienne ? Voici ce que vous devez savoir concernant l’adoption d’une IA éthique par l...
LatentView Wins Ten GenAI Projects, Secures Key $3.2M Deal with 37.5% YoY Revenue Growth
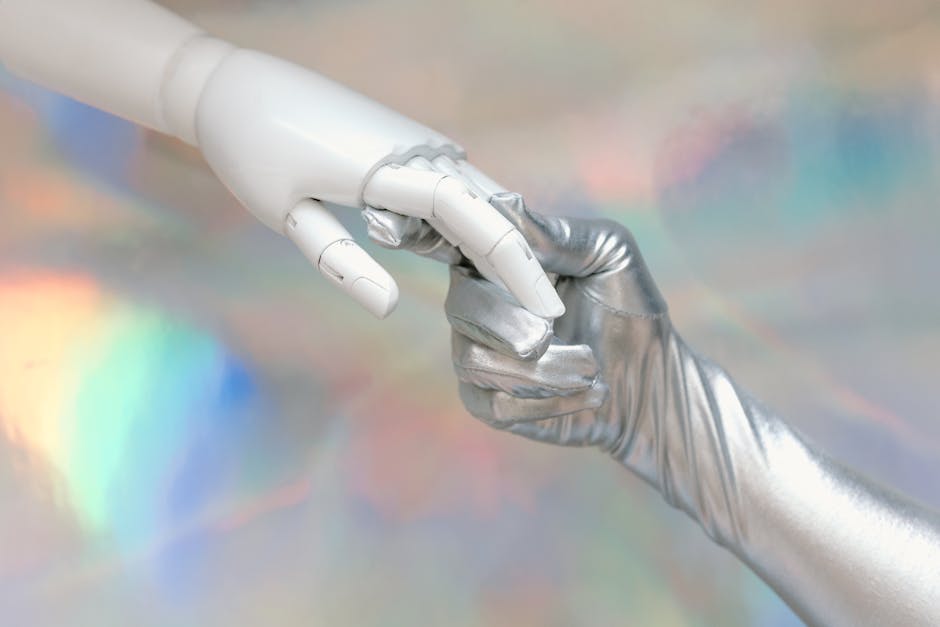
Chennai-based data analytics organization LatentView Analytics unveiled its financial results for the third quarter of FY25, reporting a total operating revenue of ₹2,278 million. This marks a [website] quarter-over-quarter (QoQ) and [website] year-over-year (YoY) increase in revenue, attributing the growth to momentum on consulting, GenAI projects, and new client wins.
LatentView has secured a $[website] million partnership with a global technology leader, collaborating with its Analytics and Decision Support team. The initiative will deliver an integrated, scalable business intelligence and data engineering solution to streamline workflows and enhance data-driven decision-making.
Moreover, LatentView onboarded nine new clients, including three former clients and 10 GenAI projects, during the quarter.
“This strong performance was driven by momentum in consulting, GenAI projects, and new client wins,” revealed Rajan Sethuraman, chief executive officer of LatentView Analytics. The business revealed it will continue investing in building GenAI capabilities.
During the earnings call, Sethuraman noted that the organization had a strong quarter in its Data Engineering horizontal and that its partnership with Databricks remains robust, as LatentView has become an elite, top-tier partner of Databricks. He stated, “Our Databricks Center of Excellence, along with the partnership we are building with Databricks, continues to grow.”.
He further noted that the corporation is seeing some interest from the Indian market in employing and deploying high-end generative AI and other solutions that could help them manage their operations and improve their decision-making effectiveness.
LatentView is experiencing good traction with GCCs in India and has won two major clients. “On the GCC front, there has been expansion with some of the accounts. We have also seen good interest in new conversations, and we expect to close a few more in the current quarter.”.
Sethuraman expressed that they have doubled down in the consumer goods space in terms of investments that we have made, both organic and inorganic, through the acquisition of Decision Point.
However, the corporation is experiencing some headwinds in terms of general market conditions for CPG companies. These have resulted in subdued performance and impacted their market capitalisation and appetite for spending on initiatives.
“We are looking at this and figuring out ways to reallocate effort and resources in the right direction so that we continue to do well in this space. This will continue to be an area of focus, and I will keep you updated in subsequent interactions on how this space is playing out,” mentioned Sethuraman.
Other key highlights from the quarter include a 62% YoY growth in the financial services vertical and the appointment of Remadevi Thottathil as Chief Human Resources Officer. The business also hosted several major events, including the LatentView Roundtable and its first Investor Day.
As the firm prepares for FY26, it remains focused on deepening client relationships and leveraging emerging technologies to drive growth.
For the past few months, Meta has been sending recipes to a Dutch scaleup called VSParticle (VSP). These are not food recipes — they’re AI-generated i...
People use tables every day to organize and interpret complex information in a structured, easily accessible format. Due to the ubiquity of such table...
OpenAI CEO Sam Altman is set to visit India for the second time this Wednesday. His Asia tour has already seen several product launches so far and his...
What Pushes Indian Engineers to Leave for the US?
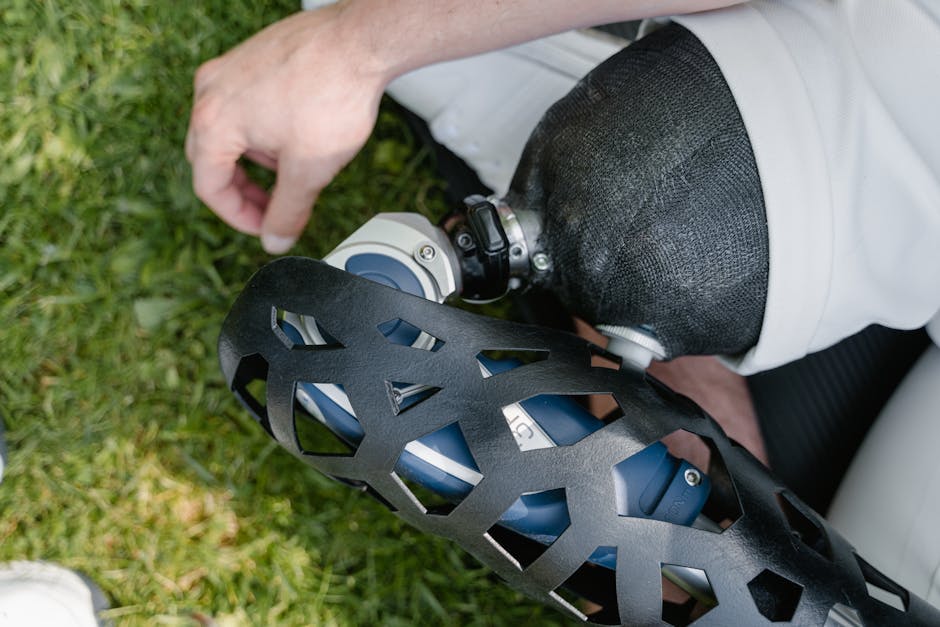
During conversations with engineers or IIT graduates, a common aspiration emerges – their plans to move abroad for higher education or career opportunities.
India has long faced the challenge of brain drain, and the issue continues to escalate. The country is now poised to become the world’s leading exporter of students.
To understand the broader implications, AIM spoke with professor V Ramgopal Rao, group vice chancellor of BITS Pilani and former director of IIT Delhi. “Our 23 IITs admit about 16,000 undergraduates annually, and our 20 AIIMS enroll around 2,000 MBBS students. In contrast, a single large US university might admit as many undergraduates as all our IITs combined,” Rao pointed out.
He suggested that government policies should foster the creation of significant endowment funds similar to those at top US universities. Additionally, introducing ‘study-now-pay-later’ schemes, like Australia’s Higher Education Loan Program (HELP), could make higher education more accessible.
These programs allow students to defer tuition payments until their income surpasses a set threshold, balancing immediate educational needs with long-term financial sustainability.
In the past, studying abroad was often seen as a straightforward path. Students and job aspirants who could afford expenses between ₹50 lakh to ₹1 crore could invest in an average or top 150 university in the US or Canada, secure a job, and consider their future set. However, that is no longer the case.
While sharing his experience of studying abroad, Harnoor Singh, a software engineer at Microsoft, mentioned, “Today, I see students at institutions like Georgia Tech leaving behind lucrative job offers in India, packages ranging from ₹50 lakh to ₹1 crore, to pursue education in the US. This trend contributes to a significant brain drain for India.”.
He added that even those attending top universities like the University of California, Berkeley face uncertainty. For instance, a professor at UC Berkeley, a leading feeder school for Google, has pointed out that a degree alone is no longer enough to secure employment.
Sharing his story on his YouTube channel, Singh mentioned, “I made the decision to study in the US eight years ago with one goal in mind: pursuing computer science (CS). In India, getting into a top-tier institution for CS meant securing an exceptional JEE rank, which I knew might not be feasible. Even if I had gained admission, I might have ended up in a decent university without my preferred major. That realisation led me to explore international options, targeting universities that offered scholarships and aligned with my academic and financial goals.”.
So, failure is not an option when it comes to the decision to move abroad. Many successful entrepreneurs and professionals in the US, including nearly 45% of Fortune 500 founders and 50% of emerging startups, come from immigrant backgrounds. They thrive because they are forced into an environment where they must excel or risk going back home.
In contrast, in India, an income of ₹1-2 lakh per month can provide a comfortable lifestyle with good food, housing, and transportation. This often leads people to stay within their comfort zones, maintaining a mediocrity mindset. There’s a safety net, family support, and stability that discourages taking big risks.
However, the stakes are different in the US. Having no fallback compels one to push themselves harder, innovate, and create opportunities. This is why many aim for O-1 visas to become entrepreneurs, go the extra mile in their careers, and do whatever it takes to stay and succeed.
The National Education Policy 2020 opened new avenues, allowing India’s publicly funded higher education institutions to set up campuses abroad. IIT Delhi was the first to seek government approval for this. Now, both IIT Delhi and IIT Madras have been greenlit to establish campuses in Abu Dhabi and Tanzania, respectively. Other IITs might follow soon. This is indeed a historic leap for India, as the country’s premier institutions extend their reach overseas.
Rao mentioned that setting up international campuses isn’t without its challenges. Navigating regulatory landscapes, securing resources, maintaining quality standards, and upholding institutional values across borders are no small feat. It’s crucial not to rush this expansion.
“We need to see how these two campuses fare before jumping into further ventures. With thoughtful planning and execution, the potential benefits, boosting global rankings, enhancing reputation, and fostering growth, could well outweigh the hurdles,” he mentioned.
As India works towards its vision of becoming a ‘Viksit Bharat’ by 2047, the Union Budget for 2025-26 addressed the brain drain issue head-on with measures aimed at strengthening India’s IITs.
The Budget raised the funding for IITs to ₹11,349 crore, a 10% increase from the previous year. It also laid the groundwork for expanding infrastructure to accommodate a growing student population, with a focus on new hostels and academic buildings.
This expansion will particularly benefit the IITs established after 2014, such as those in Tirupati, Palakkad, Bhilai, Jammu, and Goa. Moreover, the Budget proposes 10,000 fellowships for technological research, providing enhanced financial support to researchers at IITs and the Indian Institute of Science (IISc).
With these initiatives, IITs are set for greater growth, supporting India’s ambition to retain and nurture its brightest minds and paving the way for a self-sufficient future.
We leverage two key techniques to aid convergence of this ill-posed problem. The first is a very lightweight, dynamically trained convolutional neural...
Machine learning (ML) practitioners looking to reuse existing datasets to train an ML model often spend a lot of time understanding the data, making s...
Market Impact Analysis
Market Growth Trend
2018 | 2019 | 2020 | 2021 | 2022 | 2023 | 2024 |
---|---|---|---|---|---|---|
23.1% | 27.8% | 29.2% | 32.4% | 34.2% | 35.2% | 35.6% |
Quarterly Growth Rate
Q1 2024 | Q2 2024 | Q3 2024 | Q4 2024 |
---|---|---|---|
32.5% | 34.8% | 36.2% | 35.6% |
Market Segments and Growth Drivers
Segment | Market Share | Growth Rate |
---|---|---|
Machine Learning | 29% | 38.4% |
Computer Vision | 18% | 35.7% |
Natural Language Processing | 24% | 41.5% |
Robotics | 15% | 22.3% |
Other AI Technologies | 14% | 31.8% |
Technology Maturity Curve
Different technologies within the ecosystem are at varying stages of maturity:
Competitive Landscape Analysis
Company | Market Share |
---|---|
Google AI | 18.3% |
Microsoft AI | 15.7% |
IBM Watson | 11.2% |
Amazon AI | 9.8% |
OpenAI | 8.4% |
Future Outlook and Predictions
The Revenue From 220M landscape is evolving rapidly, driven by technological advancements, changing threat vectors, and shifting business requirements. Based on current trends and expert analyses, we can anticipate several significant developments across different time horizons:
Year-by-Year Technology Evolution
Based on current trajectory and expert analyses, we can project the following development timeline:
Technology Maturity Curve
Different technologies within the ecosystem are at varying stages of maturity, influencing adoption timelines and investment priorities:
Innovation Trigger
- Generative AI for specialized domains
- Blockchain for supply chain verification
Peak of Inflated Expectations
- Digital twins for business processes
- Quantum-resistant cryptography
Trough of Disillusionment
- Consumer AR/VR applications
- General-purpose blockchain
Slope of Enlightenment
- AI-driven analytics
- Edge computing
Plateau of Productivity
- Cloud infrastructure
- Mobile applications
Technology Evolution Timeline
- Improved generative models
- specialized AI applications
- AI-human collaboration systems
- multimodal AI platforms
- General AI capabilities
- AI-driven scientific breakthroughs
Expert Perspectives
Leading experts in the ai tech sector provide diverse perspectives on how the landscape will evolve over the coming years:
"The next frontier is AI systems that can reason across modalities and domains with minimal human guidance."
— AI Researcher
"Organizations that develop effective AI governance frameworks will gain competitive advantage."
— Industry Analyst
"The AI talent gap remains a critical barrier to implementation for most enterprises."
— Chief AI Officer
Areas of Expert Consensus
- Acceleration of Innovation: The pace of technological evolution will continue to increase
- Practical Integration: Focus will shift from proof-of-concept to operational deployment
- Human-Technology Partnership: Most effective implementations will optimize human-machine collaboration
- Regulatory Influence: Regulatory frameworks will increasingly shape technology development
Short-Term Outlook (1-2 Years)
In the immediate future, organizations will focus on implementing and optimizing currently available technologies to address pressing ai tech challenges:
- Improved generative models
- specialized AI applications
- enhanced AI ethics frameworks
These developments will be characterized by incremental improvements to existing frameworks rather than revolutionary changes, with emphasis on practical deployment and measurable outcomes.
Mid-Term Outlook (3-5 Years)
As technologies mature and organizations adapt, more substantial transformations will emerge in how security is approached and implemented:
- AI-human collaboration systems
- multimodal AI platforms
- democratized AI development
This period will see significant changes in security architecture and operational models, with increasing automation and integration between previously siloed security functions. Organizations will shift from reactive to proactive security postures.
Long-Term Outlook (5+ Years)
Looking further ahead, more fundamental shifts will reshape how cybersecurity is conceptualized and implemented across digital ecosystems:
- General AI capabilities
- AI-driven scientific breakthroughs
- new computing paradigms
These long-term developments will likely require significant technical breakthroughs, new regulatory frameworks, and evolution in how organizations approach security as a fundamental business function rather than a technical discipline.
Key Risk Factors and Uncertainties
Several critical factors could significantly impact the trajectory of ai tech evolution:
Organizations should monitor these factors closely and develop contingency strategies to mitigate potential negative impacts on technology implementation timelines.
Alternative Future Scenarios
The evolution of technology can follow different paths depending on various factors including regulatory developments, investment trends, technological breakthroughs, and market adoption. We analyze three potential scenarios:
Optimistic Scenario
Responsible AI driving innovation while minimizing societal disruption
Key Drivers: Supportive regulatory environment, significant research breakthroughs, strong market incentives, and rapid user adoption.
Probability: 25-30%
Base Case Scenario
Incremental adoption with mixed societal impacts and ongoing ethical challenges
Key Drivers: Balanced regulatory approach, steady technological progress, and selective implementation based on clear ROI.
Probability: 50-60%
Conservative Scenario
Technical and ethical barriers creating significant implementation challenges
Key Drivers: Restrictive regulations, technical limitations, implementation challenges, and risk-averse organizational cultures.
Probability: 15-20%
Scenario Comparison Matrix
Factor | Optimistic | Base Case | Conservative |
---|---|---|---|
Implementation Timeline | Accelerated | Steady | Delayed |
Market Adoption | Widespread | Selective | Limited |
Technology Evolution | Rapid | Progressive | Incremental |
Regulatory Environment | Supportive | Balanced | Restrictive |
Business Impact | Transformative | Significant | Modest |
Transformational Impact
Redefinition of knowledge work, automation of creative processes. This evolution will necessitate significant changes in organizational structures, talent development, and strategic planning processes.
The convergence of multiple technological trends—including artificial intelligence, quantum computing, and ubiquitous connectivity—will create both unprecedented security challenges and innovative defensive capabilities.
Implementation Challenges
Ethical concerns, computing resource limitations, talent shortages. Organizations will need to develop comprehensive change management strategies to successfully navigate these transitions.
Regulatory uncertainty, particularly around emerging technologies like AI in security applications, will require flexible security architectures that can adapt to evolving compliance requirements.
Key Innovations to Watch
Multimodal learning, resource-efficient AI, transparent decision systems. Organizations should monitor these developments closely to maintain competitive advantages and effective security postures.
Strategic investments in research partnerships, technology pilots, and talent development will position forward-thinking organizations to leverage these innovations early in their development cycle.
Technical Glossary
Key technical terms and definitions to help understand the technologies discussed in this article.
Understanding the following technical concepts is essential for grasping the full implications of the security threats and defensive measures discussed in this article. These definitions provide context for both technical and non-technical readers.