OpenAI responds to DeepSeek competition with detailed reasoning traces for o3-mini - Related to course, reasoning, equity, from?, traces
HEAL: A framework for health equity assessment of machine learning performance
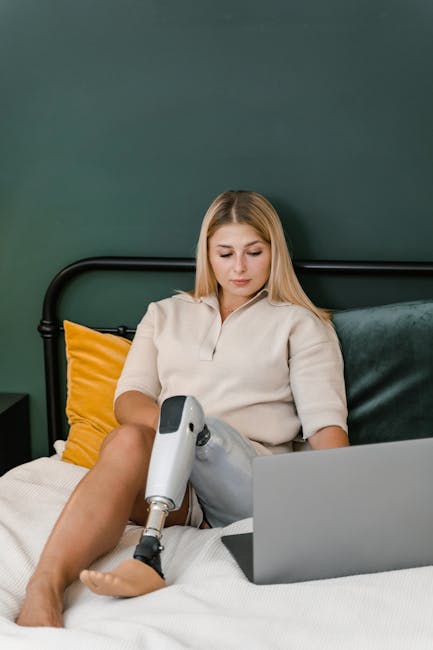
As an illustrative case study, we applied the framework to a dermatology model, which utilizes a convolutional neural network similar to that described in prior work. This example dermatology model was trained to classify 288 skin conditions using a development dataset of 29k cases. The input to the model consists of three photos of a skin concern along with demographic information and a brief structured medical history. The output consists of a ranked list of possible matching skin conditions.
Using the HEAL framework, we evaluated this model by assessing whether it prioritized performance with respect to pre-existing health outcomes. The model was designed to predict possible dermatologic conditions (from a list of hundreds) based on photos of a skin concern and patient metadata. Evaluation of the model is done using a top-3 agreement metric, which quantifies how often the top 3 output conditions match the most likely condition as suggested by a dermatologist panel. The HEAL metric is computed via the anticorrelation of this top-3 agreement with health outcome rankings.
We used a dataset of 5,420 teledermatology cases, enriched for diversity in age, sex and race/ethnicity, to retrospectively evaluate the model’s HEAL metric. The dataset consisted of “store-and-forward” cases from patients of 20 years or older from primary care providers in the USA and skin cancer clinics in Australia. Based on a review of the literature, we decided to explore race/ethnicity, sex and age as potential factors of inequity, and used sampling techniques to ensure that our evaluation dataset had sufficient representation of all race/ethnicity, sex and age groups. To quantify pre-existing health outcomes for each subgroup we relied on measurements from public databases endorsed by the World Health Organization, such as Years of Life Lost (YLLs) and Disability-Adjusted Life Years (DALYs; years of life lost plus years lived with disability).
AI-driven technologies are weaving themselves into the fabric of our daily routines, with the potential to enhance our access to knowledge and boost o...
Google DeepMind has made available Gemini [website] Flash and introduced new models, including Gemini [website] Pro Experimental and Gemini [website] Flash-Lite. These ...
UK startup Surf Security has launched a beta version of what it indicates is the world’s first browser with a built-in feature designed to spot AI-genera...
OpenAI responds to DeepSeek competition with detailed reasoning traces for o3-mini
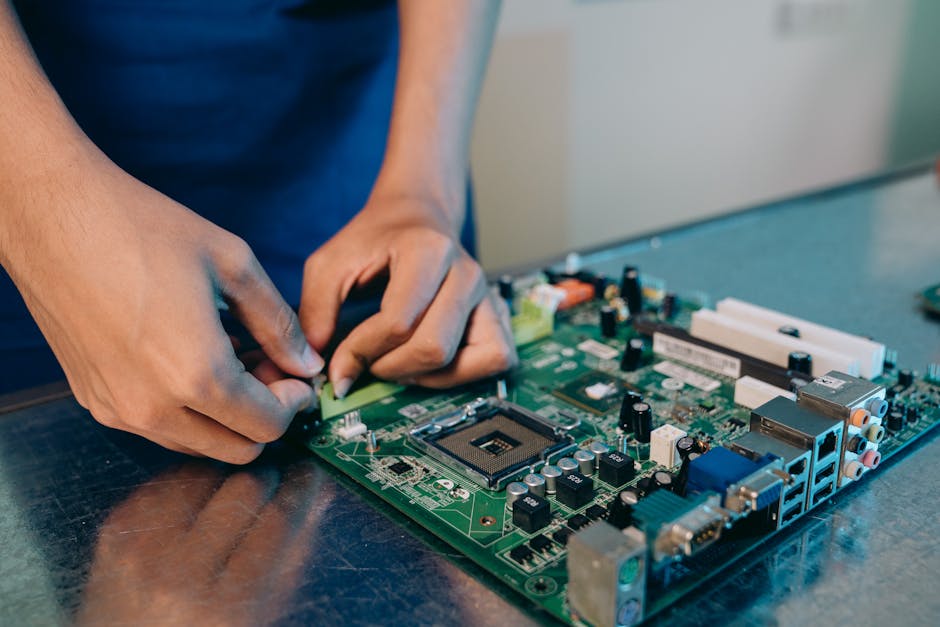
OpenAI is now showing more details of the reasoning process of o3-mini, its latest reasoning model. The change was presented on OpenAI’s X account and comes as the AI lab is under increased pressure by DeepSeek-R1, a rival open model that fully displays its reasoning tokens.
Models like o3 and R1 undergo a lengthy “chain of thought” (CoT) process in which they generate extra tokens to break down the problem, reason about and test different answers and reach a final solution. Previously, OpenAI’s reasoning models hid their chain of thought and only produced a high-level overview of reasoning steps. This made it difficult for people and developers to understand the model’s reasoning logic and change their instructions and prompts to steer it in the right direction.
OpenAI considered chain of thought a competitive advantage and hid it to prevent rivals from copying to train their models. But with R1 and other open models showing their full reasoning trace, the lack of transparency becomes a disadvantage for OpenAI.
The new version of o3-mini presents a more detailed version of CoT. Although we still don’t see the raw tokens, it provides much more clarity on the reasoning process.
In our previous experiments on o1 and R1, we found that o1 was slightly improved at solving data analysis and reasoning problems. However, one of the key limitations was that there was no way to figure out why the model made mistakes — and it often made mistakes when faced with messy real-world data obtained from the web. On the other hand, R1’s chain of thought enabled us to troubleshoot the problems and change our prompts to improve reasoning.
For example, in one of our experiments, both models failed to provide the correct answer. But thanks to R1’s detailed chain of thought, we were able to find out that the problem was not with the model itself but with the retrieval stage that gathered information from the web. In other experiments, R1’s chain of thought was able to provide us with hints when it failed to parse the information we provided it, while o1 only gave us a very rough overview of how it was formulating its response.
We tested the new o3-mini model on a variant of a previous experiment we ran with o1. We provided the model with a text file containing prices of various stocks from January 2024 through January 2025. The file was noisy and unformatted, a mixture of plain text and HTML elements. We then asked the model to calculate the value of a portfolio that invested $140 in the Magnificent 7 stocks on the first day of each month from January 2024 to January 2025, distributed evenly across all stocks (we used the term “Mag 7” in the prompt to make it a bit more challenging).
o3-mini’s CoT was really helpful this time. First, the model reasoned about what the Mag 7 was, filtered the data to only keep the relevant stocks (to make the problem challenging, we added a few non–Mag 7 stocks to the data), calculated the monthly amount to invest in each stock, and made the final calculations to provide the correct answer (the portfolio would be worth around $2,200 at the latest time registered in the data we provided to the model).
It will take a lot more testing to see the limits of the new chain of thought, since OpenAI is still hiding a lot of details. But in our vibe checks, it seems that the new format is much more useful.
When DeepSeek-R1 was released, it had three clear advantages over OpenAI’s reasoning models: It was open, cheap and transparent.
Since then, OpenAI has managed to shorten the gap. While o1 costs $60 per million output tokens, o3-mini costs just $[website], while outperforming o1 on many reasoning benchmarks. R1 costs around $7 and $8 per million tokens on [website] providers. (DeepSeek offers R1 at $[website] per million tokens on its own servers, but many organizations will not be able to use it because it is hosted in China.).
With the new change to the CoT output, OpenAI has managed to somewhat work around the transparency problem.
It remains to be seen what OpenAI will do about open sourcing its models. Since its release, R1 has already been adapted, forked and hosted by many different labs and companies potentially making it the preferred reasoning model for enterprises. OpenAI CEO Sam Altman lately admitted that he was “on the wrong side of history” in open source debate. We’ll have to see how this realization will manifest itself in OpenAI’s future releases.
in recent times, DeepSeek showcased their latest model, R1, and article after article came out praising its performance relative to cost, and how the release...
Health datasets play a crucial role in research and medical education, but it can be challenging to create a dataset that represents the real world. F...
Language models (LMs) trained to predict the next word given input text are the key technology for many applications [1, 2]. In Gboard, LMs are used t...
Where do startups come from? Ideas and entrepreneurs, of course
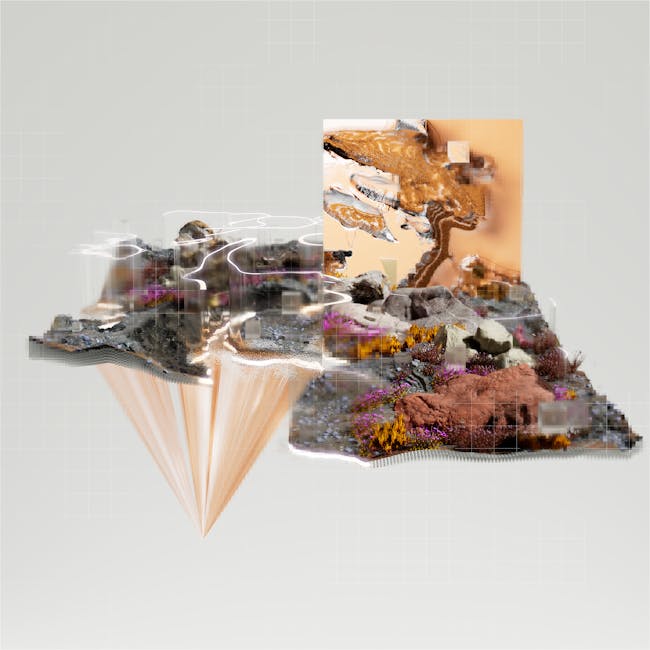
At TNW, we are all about supporting and elevating startups and entrepreneurs who are doing epic stuff with tech. When Red Bull reached out to talk about their innovation competition, my first thought was what on Earth do we have in common with an energy drink business that has people jumping off cliffs and surfing really large waves?
Apart from fuelling — in different ways — founders and developers across the world, of course. (Although, I guess, building a business could be considered an extreme sport.).
Turns out, when it comes to supporting young minds that could change the world with their ideas — quite a lot.
Red Bull Basement is the beverage giant’s recurring innovation competition that, in the business’s words, “empowers the next generation of innovators to develop and launch outstanding ideas and disrupt today’s status quo.” The 2024 edition took place across 39 countries, and received over 110,000 submissions.
The local winners were all flown out to Tokyo for a global final across three days over the past week. They got to take part in workshops on business modelling, utilising AI as a founder, creating a successful pitch, forming strategic partnerships, brand development, media relations, etc.
The top 10 got to pitch their ideas to the panel of global judges — and an auditorium of a few hundred people — on the 45th floor, in front of a backdrop of Tokyo lit up at night. The prize for the global winner was an all-expenses-paid three-week trip to San Francisco to be mentored by Silicon Valley-based Plug and Play VC.
Part of the appeal for us as a media organisation was of course access to the judges, including Head of Microsoft for Startups Hans Yang, Plug and Play early-stage investor Letizia Royo-Villanova, and digital economy business mentor Jun Yuh, to pick their brains on how they identify winning startups and exceptional founders (and I did, all of which will follow in another article).
However, what really moved me was the ingenuity, drive, and enthusiasm of the next generation of entrepreneurs.
Ideas included a bone conduction device to help people with Parkinson’s walk more securely built by Cambridge student Jonathan Fisher, whose father suffers from the disease. “I figured, if something is critical enough, you should try, even if the odds are against you, because you never know what will happen,” Fisher told TNW.
Another device built by Stanford students in the US wants to give the visually impaired their sight back. There were also water-saving AI-supported gadgets from Greece and Egypt, wild-fire warning systems from South Africa, AI tools to help students connect with mentors and scholarship opportunities from Ireland and Spain or democratise access to high-level sports coaching from Belgium.
Other innovations included early illness detection from Kosovo, brain fitness tracking from the Czech Republic, and an athlete mental training app from Germany — just to name a few.
The winner of the global final was Soi Gamayon from the Philippines with his AgriConnect startup. The AI-powered app, inspired by watching his uncle’s struggle farming rice, allows farmers to monitor their crops, build resilience, and increase their yield.
“My purpose is really to build something bigger than myself,” mentioned Gamayon. “I’m doing this for Filipino farmers. This wasn’t just about competing or winning. It’s about sharing moments and memories with people who are like-minded. I share this with all the other teams who are here.”.
Dutch finalist looking for the ‘positive side of tech’.
The Dutch finalist, fresh out of graduate studies in Strategic Management at the Erasmus University in Rotterdam, was Bram van Peursem, with an AI-powered app called Hubster. He made it all the way to the top 10.
Based on his own experience of losing hours of precious time to mindless social media scrolling while managing his own schedule as a student, van Peursem designed Hubster to help people transform their phone usage from a time sink into motivation to act on the things they hope to achieve in life.
Hubster, still under development, will let you enter the interests and ambitions that are currently most critical to you. Van Peursem gives the examples of running a marathon, understanding more about tech stocks, and learning German.
As you embark on a scrolling session that will surely end half an hour later with the yucky feeling of “but I was only going to check…” the app will instead prompt you with notifications such as “It’s currently great weather for a 5k recovery run,” “AMD just showcased a chip upgrade, read more about it here” and link to an article, or “Nutzen sie ihre zeit so optimal?” with your language learning app of choice.
“It is really focused on making tech positive,” van Peursem told TNW. “Because I think we often forget that our phone is a tool which has all the information in the world, very accessible in your pocket, but nobody uses it like that.”.
The desire to build something has been there from the start. “I have always wanted to be a founder,” van Peursem, both of whose parents are entrepreneurs, says. “I’ve always had these ideas but I never really acted on them. And that was also the thing I was most scared about — I want to be an entrepreneur, but what if I never act on it? So I’m really grateful to Red Bull and Microsoft for this opportunity [to make the idea concrete].
Personally, I always feel honoured to tell the stories of people who have ideas and work hard to bring them to reality, striving to impact the world in positive ways. Us journalists only observe and write about it — entrepreneurs are the ones actually building stuff. Mostly just fuelled by pure drive and passion, but sometimes — like when running a startup bootcamp marathon — by copious amounts of caffeine.
While many companies are caught up in the AI hype cycle, ABB, an industrial robot supplier and manufacturer, has been building and implementing practi...
ScreenAI’s architecture is based on PaLI, composed of a multimodal encoder block and an autoregressive decoder. The PaLI encoder uses a vision transfo...
Gemini Flash [website] just debuted last week, but it's already getting an upgrade -- the ability to watch YouTube for you.
Market Impact Analysis
Market Growth Trend
2018 | 2019 | 2020 | 2021 | 2022 | 2023 | 2024 |
---|---|---|---|---|---|---|
23.1% | 27.8% | 29.2% | 32.4% | 34.2% | 35.2% | 35.6% |
Quarterly Growth Rate
Q1 2024 | Q2 2024 | Q3 2024 | Q4 2024 |
---|---|---|---|
32.5% | 34.8% | 36.2% | 35.6% |
Market Segments and Growth Drivers
Segment | Market Share | Growth Rate |
---|---|---|
Machine Learning | 29% | 38.4% |
Computer Vision | 18% | 35.7% |
Natural Language Processing | 24% | 41.5% |
Robotics | 15% | 22.3% |
Other AI Technologies | 14% | 31.8% |
Technology Maturity Curve
Different technologies within the ecosystem are at varying stages of maturity:
Competitive Landscape Analysis
Company | Market Share |
---|---|
Google AI | 18.3% |
Microsoft AI | 15.7% |
IBM Watson | 11.2% |
Amazon AI | 9.8% |
OpenAI | 8.4% |
Future Outlook and Predictions
The Heal Framework Health landscape is evolving rapidly, driven by technological advancements, changing threat vectors, and shifting business requirements. Based on current trends and expert analyses, we can anticipate several significant developments across different time horizons:
Year-by-Year Technology Evolution
Based on current trajectory and expert analyses, we can project the following development timeline:
Technology Maturity Curve
Different technologies within the ecosystem are at varying stages of maturity, influencing adoption timelines and investment priorities:
Innovation Trigger
- Generative AI for specialized domains
- Blockchain for supply chain verification
Peak of Inflated Expectations
- Digital twins for business processes
- Quantum-resistant cryptography
Trough of Disillusionment
- Consumer AR/VR applications
- General-purpose blockchain
Slope of Enlightenment
- AI-driven analytics
- Edge computing
Plateau of Productivity
- Cloud infrastructure
- Mobile applications
Technology Evolution Timeline
- Improved generative models
- specialized AI applications
- AI-human collaboration systems
- multimodal AI platforms
- General AI capabilities
- AI-driven scientific breakthroughs
Expert Perspectives
Leading experts in the ai tech sector provide diverse perspectives on how the landscape will evolve over the coming years:
"The next frontier is AI systems that can reason across modalities and domains with minimal human guidance."
— AI Researcher
"Organizations that develop effective AI governance frameworks will gain competitive advantage."
— Industry Analyst
"The AI talent gap remains a critical barrier to implementation for most enterprises."
— Chief AI Officer
Areas of Expert Consensus
- Acceleration of Innovation: The pace of technological evolution will continue to increase
- Practical Integration: Focus will shift from proof-of-concept to operational deployment
- Human-Technology Partnership: Most effective implementations will optimize human-machine collaboration
- Regulatory Influence: Regulatory frameworks will increasingly shape technology development
Short-Term Outlook (1-2 Years)
In the immediate future, organizations will focus on implementing and optimizing currently available technologies to address pressing ai tech challenges:
- Improved generative models
- specialized AI applications
- enhanced AI ethics frameworks
These developments will be characterized by incremental improvements to existing frameworks rather than revolutionary changes, with emphasis on practical deployment and measurable outcomes.
Mid-Term Outlook (3-5 Years)
As technologies mature and organizations adapt, more substantial transformations will emerge in how security is approached and implemented:
- AI-human collaboration systems
- multimodal AI platforms
- democratized AI development
This period will see significant changes in security architecture and operational models, with increasing automation and integration between previously siloed security functions. Organizations will shift from reactive to proactive security postures.
Long-Term Outlook (5+ Years)
Looking further ahead, more fundamental shifts will reshape how cybersecurity is conceptualized and implemented across digital ecosystems:
- General AI capabilities
- AI-driven scientific breakthroughs
- new computing paradigms
These long-term developments will likely require significant technical breakthroughs, new regulatory frameworks, and evolution in how organizations approach security as a fundamental business function rather than a technical discipline.
Key Risk Factors and Uncertainties
Several critical factors could significantly impact the trajectory of ai tech evolution:
Organizations should monitor these factors closely and develop contingency strategies to mitigate potential negative impacts on technology implementation timelines.
Alternative Future Scenarios
The evolution of technology can follow different paths depending on various factors including regulatory developments, investment trends, technological breakthroughs, and market adoption. We analyze three potential scenarios:
Optimistic Scenario
Responsible AI driving innovation while minimizing societal disruption
Key Drivers: Supportive regulatory environment, significant research breakthroughs, strong market incentives, and rapid user adoption.
Probability: 25-30%
Base Case Scenario
Incremental adoption with mixed societal impacts and ongoing ethical challenges
Key Drivers: Balanced regulatory approach, steady technological progress, and selective implementation based on clear ROI.
Probability: 50-60%
Conservative Scenario
Technical and ethical barriers creating significant implementation challenges
Key Drivers: Restrictive regulations, technical limitations, implementation challenges, and risk-averse organizational cultures.
Probability: 15-20%
Scenario Comparison Matrix
Factor | Optimistic | Base Case | Conservative |
---|---|---|---|
Implementation Timeline | Accelerated | Steady | Delayed |
Market Adoption | Widespread | Selective | Limited |
Technology Evolution | Rapid | Progressive | Incremental |
Regulatory Environment | Supportive | Balanced | Restrictive |
Business Impact | Transformative | Significant | Modest |
Transformational Impact
Redefinition of knowledge work, automation of creative processes. This evolution will necessitate significant changes in organizational structures, talent development, and strategic planning processes.
The convergence of multiple technological trends—including artificial intelligence, quantum computing, and ubiquitous connectivity—will create both unprecedented security challenges and innovative defensive capabilities.
Implementation Challenges
Ethical concerns, computing resource limitations, talent shortages. Organizations will need to develop comprehensive change management strategies to successfully navigate these transitions.
Regulatory uncertainty, particularly around emerging technologies like AI in security applications, will require flexible security architectures that can adapt to evolving compliance requirements.
Key Innovations to Watch
Multimodal learning, resource-efficient AI, transparent decision systems. Organizations should monitor these developments closely to maintain competitive advantages and effective security postures.
Strategic investments in research partnerships, technology pilots, and talent development will position forward-thinking organizations to leverage these innovations early in their development cycle.
Technical Glossary
Key technical terms and definitions to help understand the technologies discussed in this article.
Understanding the following technical concepts is essential for grasping the full implications of the security threats and defensive measures discussed in this article. These definitions provide context for both technical and non-technical readers.