How Human Perception of Brightness and Color Shapes Video Encoding Strategies - Related to back, how, unknown, brightness, $700m
How Human Perception of Brightness and Color Shapes Video Encoding Strategies
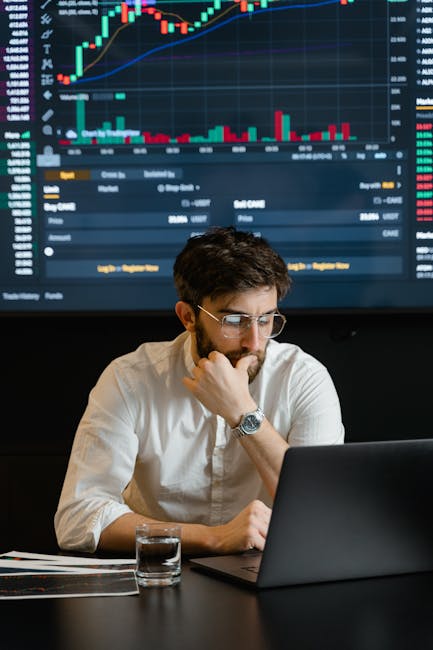
In the digital content world, video has become the dominant format, and with that, video compression is more critical than ever. Speaking at India’s Biggest GenAI Summit for developers, MLDS 2025, Arvind Sasikumar, co-founder and CTO at Quinn, shared insights on optimising video transcoding to balance efficiency, quality, and playback performance.
The benefits of video compression are clear, smaller file sizes lead to reduced storage costs and lower data transfer expenses. But beyond these obvious advantages, compression directly impacts user experience. One fundamental aspect that often goes unnoticed is that video buffering is a binary state: a video is either playing smoothly or buffering. There’s no middle ground.
Sasikumar explained, “Consider an example. If 100 people each have a [website] Mbps internet connection, but the video they are watching has a 2 Mbps bitrate, every user will experience buffering. However, by reducing the file size by just 5%, all 100 people can enjoy seamless playback. This demonstrates why compression is not just about reducing numbers, it’s about eliminating interruptions that degrade the viewing experience.”.
There are two primary types of compression: lossy and lossless. Lossy compression reduces file size by removing some data through predictive algorithms. This is the preferred method for video and audio because human perception can compensate for minor quality losses. Lossless compression retains all original data, ensuring no quality loss. This is ideal for text and data files where precision is crucial but is not practical for video due to high storage requirements.
Humans don’t perceive pixels individually; instead, we process visual information contextually. Compression algorithms leverage this by reducing redundant data without noticeable quality loss.
Sasikumar mentioned that one common technique is chroma subsampling, where brightness (luminance) is prioritised over color detail. Since the human eye is more sensitive to brightness than color, encoding schemes like 4:2:0 cut data usage by nearly half without significant perceptual impact.
Beyond individual frames, modern video encoding techniques exploit similarities between consecutive frames to achieve higher compression rates. Instead of storing each frame as a separate image, encoders analyse differences between frames and store only the changes.
A robust compression algorithm must optimise three key areas: compressing individual frames efficiently, minimising redundant data between frames, and leveraging human perception to maintain quality at lower bitrates. One of the most effective strategies is motion estimation and motion compensation.
Instead of encoding pixel-by-pixel differences, encoders track objects as they move across frames and store them as motion vectors. This significantly reduces the amount of data required to represent motion.
He explained with an example, if a ball moves across the screen while the background remains static, the algorithm records only the ball’s movement rather than re-encoding the entire frame. This principle underpins most modern video encoding formats. However, the accuracy of motion estimation affects compression efficiency.
I-frames (Intra-coded frames) are key reference points in video encoding. Their placement plays a crucial role in ensuring smooth playback and efficient compression. The first frame should be an I-frame to ensure seamless decoding. Periodic I-frames improve quality and facilitate efficient seeking. When a scene transition occurs, pixel values change abruptly, making motion-based encoding struggle. Using an I-frame at scene transitions prevents quality loss.
Motion significantly influences compression strategies. Slow-motion videos require less data since frame-to-frame changes are minimal. Fast-motion videos demand more data but also allow for higher compression, as human perception cannot detect fine details in rapid movement. Without perceptual compression techniques, fast-motion videos could be ten times larger than slow-motion ones. However, optimised encoding can reduce this difference to just two to three times.
Further, lighting, contrast, and color variations also impact compression efficiency. Since human vision perceives brightness and color differently, encoding strategies must consider these perceptual factors to optimise quality across various scenes.
Traditional metrics like PSNR (Peak Signal-to-Noise Ratio) measure pixel-level differences between original and compressed frames. However, PSNR does not always align with human perception. To address this, Netflix developed VMAF (Video Multi-Method Assessment Fusion), an open-source perceptual quality metric.
VMAF evaluates how viewers perceive quality by incorporating subjective ratings. This helps find the optimal balance between compression efficiency and visual fidelity.
Additionally, to achieve high-quality video compression while optimising resources, segmenting videos into chunks allows for parallel processing and efficient re-encoding. Also, minimising re-encoding is essential because encoding is inherently lossy, and unnecessary re-encodes degrade quality.
The AI industry is accelerating rapidly, and this is evident in the introduction and application of AI agents. A few years back, AI was just an LLM wr...
Après l’incident qu’a fait objet DeepSeek la semaine dernière, les chercheurs affirment avoir trouvé un moyen de contourner les mesures de protection ...
Le 10 et 11 février 2025, Paris deviendra le centre névralgique de la gouvernance de l’IA. Le Sommet pour l’Action sur l’IA, organisé par la France et...
MELON: Reconstructing 3D objects from images with unknown poses
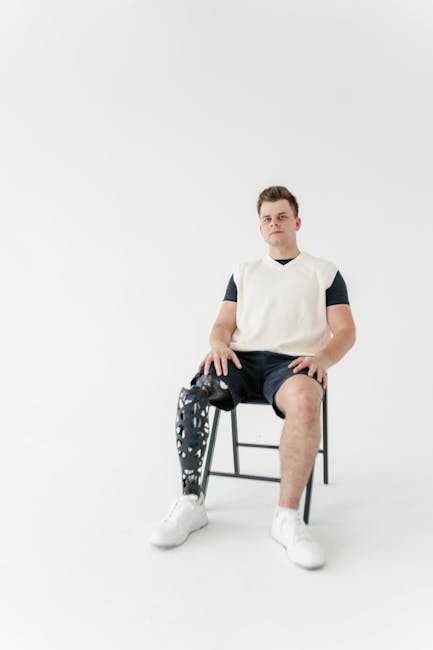
We leverage two key techniques to aid convergence of this ill-posed problem. The first is a very lightweight, dynamically trained convolutional neural network (CNN) encoder that regresses camera poses from training images. We pass a downscaled training image to a four layer CNN that infers the camera pose. This CNN is initialized from noise and requires no pre-training. Its capacity is so small that it forces similar looking images to similar poses, providing an implicit regularization greatly aiding convergence.
The second technique is a modulo loss that simultaneously considers pseudo symmetries of an object. We render the object from a fixed set of viewpoints for each training image, backpropagating the loss only through the view that best fits the training image. This effectively considers the plausibility of multiple views for each image. In practice, we find N=2 views (viewing an object from the other side) is all that’s required in most cases, but sometimes get improved results with N=4 for square objects.
These two techniques are integrated into standard NeRF training, except that instead of fixed camera poses, poses are inferred by the CNN and duplicated by the modulo loss. Photometric gradients back-propagate through the best-fitting cameras into the CNN. We observe that cameras generally converge quickly to globally optimal poses (see animation below). After training of the neural field, MELON can synthesize novel views using standard NeRF rendering methods.
We simplify the problem by using the NeRF-Synthetic dataset, a popular benchmark for NeRF research and common in the pose-inference literature. This synthetic dataset has cameras at precisely fixed distances and a consistent “up” orientation, requiring us to infer only the polar coordinates of the camera. This is the same as an object at the center of a globe with a camera always pointing at it, moving along the surface. We then only need the latitude and longitude (2 degrees of freedom) to specify the camera pose.
The AI industry is accelerating rapidly, and this is evident in the introduction and application of AI agents. A few years back, AI was just an LLM wr...
Vous êtes en Europe et vous utilisez l’IA pour vos tâches au quotidienne ? Voici ce que vous devez savoir concernant l’adoption d’une IA éthique par l...
Marvel Studios se retrouve au cœur d’une polémique après la sortie de l’affiche de « Les Quatre Fantastiques : Premiers Pas ». Certains internautes on...
Nvidia, Accel back Netherlands-based AI firm Nebius in $700M deal
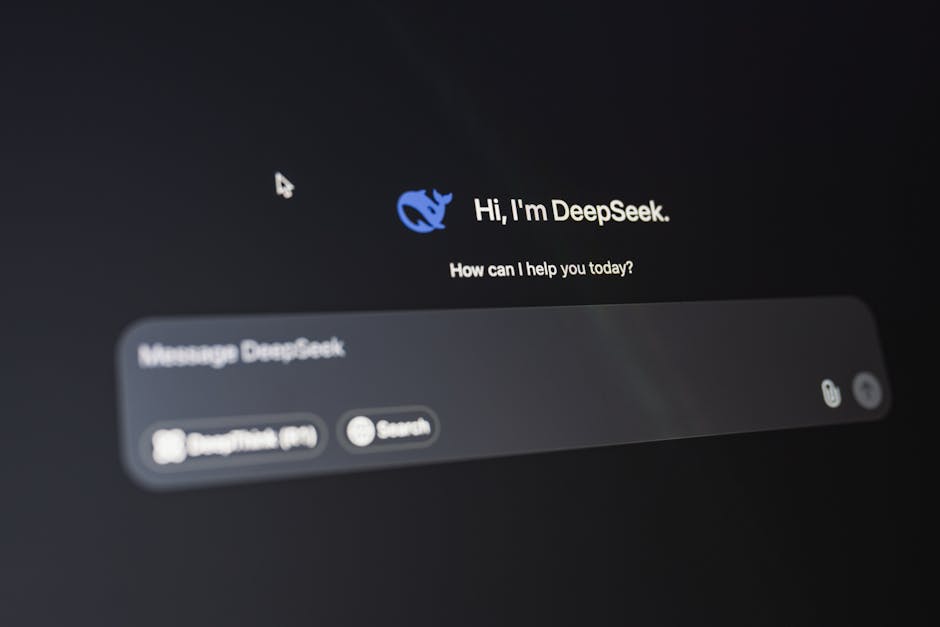
Amsterdam-headquartered Nebius, which builds full-stack AI infrastructure for tech firms, has secured $700mn in a private equity deal led by Nvidia, Accel, and asset manager Orbis.
The funding comes in the form of a private placement — when a organization sells stocks directly to a private investor instead of on the public market. The deal will see Nebius issue [website] million Class A shares at $21 apiece.
Nebius, which is the rebranded European arm of “Russia’s Google,” Yandex, is investing more than $1bn across Europe by mid-2025 as it seeks to cash in on booming demand for AI computing power. It also in the recent past revealed plans to build its first GPU cluster in the US.
“We have demonstrated the scale of our ambitions, initiating an AI infrastructure build-out across two continents,” mentioned Arkady Volozh, founder and CEO of Nebius. “This strategic financing gives us additional firepower to do it faster and on a larger scale.”.
Nebius’ expansion strategy includes constructing new custom data centres and expanding existing facilities, like its data centre in Finland which we visited in October. It will also deploy additional capacity through colocation.
Volozh aims for Nebius to be a Phoenix rising from the ashes of what remained of Yandex following the corporation’s divestment from Russia earlier this year. The $[website] deal constituted the largest corporate exit from the country since the start of Russia’s full-scale invasion of Ukraine over two years ago.
Nebius’ core product is an AI-centric cloud platform for intense AI workloads. The firm is also one of the launch partners for Nvidia’s fabled Blackwell GPUs, however, this investment does not guarantee that.
“The deal is not about the GPUs,” Volozh told Bloomberg. “But, of course, it demonstrates our close relationship, which we hope will influence our pipeline.”.
Investors are pouring huge sums of money into AI compute. The global AI infrastructure market size is projected to grow from $[website] in 2024 to $[website] by 2032, . One competitor to Nebius, US firm CoreWeave, is preparing for an IPO that could put the firm, founded in 2017, at a $35bn valuation.
As an illustrative case study, we applied the framework to a dermatology model, which utilizes a convolutional neural network similar to that describe...
As part of his global tour, OpenAI CEO Sam Altman is in Delhi today for the organization’s DevDay, joined by India’s IT minister Ashwini Vaishnaw and OpenA...
Lung cancer is the leading cause of cancer-related deaths globally with [website] million deaths reported in 2020. Late diagnosis dramatically reduces the c...
Market Impact Analysis
Market Growth Trend
2018 | 2019 | 2020 | 2021 | 2022 | 2023 | 2024 |
---|---|---|---|---|---|---|
23.1% | 27.8% | 29.2% | 32.4% | 34.2% | 35.2% | 35.6% |
Quarterly Growth Rate
Q1 2024 | Q2 2024 | Q3 2024 | Q4 2024 |
---|---|---|---|
32.5% | 34.8% | 36.2% | 35.6% |
Market Segments and Growth Drivers
Segment | Market Share | Growth Rate |
---|---|---|
Machine Learning | 29% | 38.4% |
Computer Vision | 18% | 35.7% |
Natural Language Processing | 24% | 41.5% |
Robotics | 15% | 22.3% |
Other AI Technologies | 14% | 31.8% |
Technology Maturity Curve
Different technologies within the ecosystem are at varying stages of maturity:
Competitive Landscape Analysis
Company | Market Share |
---|---|
Google AI | 18.3% |
Microsoft AI | 15.7% |
IBM Watson | 11.2% |
Amazon AI | 9.8% |
OpenAI | 8.4% |
Future Outlook and Predictions
The Human Perception Brightness landscape is evolving rapidly, driven by technological advancements, changing threat vectors, and shifting business requirements. Based on current trends and expert analyses, we can anticipate several significant developments across different time horizons:
Year-by-Year Technology Evolution
Based on current trajectory and expert analyses, we can project the following development timeline:
Technology Maturity Curve
Different technologies within the ecosystem are at varying stages of maturity, influencing adoption timelines and investment priorities:
Innovation Trigger
- Generative AI for specialized domains
- Blockchain for supply chain verification
Peak of Inflated Expectations
- Digital twins for business processes
- Quantum-resistant cryptography
Trough of Disillusionment
- Consumer AR/VR applications
- General-purpose blockchain
Slope of Enlightenment
- AI-driven analytics
- Edge computing
Plateau of Productivity
- Cloud infrastructure
- Mobile applications
Technology Evolution Timeline
- Improved generative models
- specialized AI applications
- AI-human collaboration systems
- multimodal AI platforms
- General AI capabilities
- AI-driven scientific breakthroughs
Expert Perspectives
Leading experts in the ai tech sector provide diverse perspectives on how the landscape will evolve over the coming years:
"The next frontier is AI systems that can reason across modalities and domains with minimal human guidance."
— AI Researcher
"Organizations that develop effective AI governance frameworks will gain competitive advantage."
— Industry Analyst
"The AI talent gap remains a critical barrier to implementation for most enterprises."
— Chief AI Officer
Areas of Expert Consensus
- Acceleration of Innovation: The pace of technological evolution will continue to increase
- Practical Integration: Focus will shift from proof-of-concept to operational deployment
- Human-Technology Partnership: Most effective implementations will optimize human-machine collaboration
- Regulatory Influence: Regulatory frameworks will increasingly shape technology development
Short-Term Outlook (1-2 Years)
In the immediate future, organizations will focus on implementing and optimizing currently available technologies to address pressing ai tech challenges:
- Improved generative models
- specialized AI applications
- enhanced AI ethics frameworks
These developments will be characterized by incremental improvements to existing frameworks rather than revolutionary changes, with emphasis on practical deployment and measurable outcomes.
Mid-Term Outlook (3-5 Years)
As technologies mature and organizations adapt, more substantial transformations will emerge in how security is approached and implemented:
- AI-human collaboration systems
- multimodal AI platforms
- democratized AI development
This period will see significant changes in security architecture and operational models, with increasing automation and integration between previously siloed security functions. Organizations will shift from reactive to proactive security postures.
Long-Term Outlook (5+ Years)
Looking further ahead, more fundamental shifts will reshape how cybersecurity is conceptualized and implemented across digital ecosystems:
- General AI capabilities
- AI-driven scientific breakthroughs
- new computing paradigms
These long-term developments will likely require significant technical breakthroughs, new regulatory frameworks, and evolution in how organizations approach security as a fundamental business function rather than a technical discipline.
Key Risk Factors and Uncertainties
Several critical factors could significantly impact the trajectory of ai tech evolution:
Organizations should monitor these factors closely and develop contingency strategies to mitigate potential negative impacts on technology implementation timelines.
Alternative Future Scenarios
The evolution of technology can follow different paths depending on various factors including regulatory developments, investment trends, technological breakthroughs, and market adoption. We analyze three potential scenarios:
Optimistic Scenario
Responsible AI driving innovation while minimizing societal disruption
Key Drivers: Supportive regulatory environment, significant research breakthroughs, strong market incentives, and rapid user adoption.
Probability: 25-30%
Base Case Scenario
Incremental adoption with mixed societal impacts and ongoing ethical challenges
Key Drivers: Balanced regulatory approach, steady technological progress, and selective implementation based on clear ROI.
Probability: 50-60%
Conservative Scenario
Technical and ethical barriers creating significant implementation challenges
Key Drivers: Restrictive regulations, technical limitations, implementation challenges, and risk-averse organizational cultures.
Probability: 15-20%
Scenario Comparison Matrix
Factor | Optimistic | Base Case | Conservative |
---|---|---|---|
Implementation Timeline | Accelerated | Steady | Delayed |
Market Adoption | Widespread | Selective | Limited |
Technology Evolution | Rapid | Progressive | Incremental |
Regulatory Environment | Supportive | Balanced | Restrictive |
Business Impact | Transformative | Significant | Modest |
Transformational Impact
Redefinition of knowledge work, automation of creative processes. This evolution will necessitate significant changes in organizational structures, talent development, and strategic planning processes.
The convergence of multiple technological trends—including artificial intelligence, quantum computing, and ubiquitous connectivity—will create both unprecedented security challenges and innovative defensive capabilities.
Implementation Challenges
Ethical concerns, computing resource limitations, talent shortages. Organizations will need to develop comprehensive change management strategies to successfully navigate these transitions.
Regulatory uncertainty, particularly around emerging technologies like AI in security applications, will require flexible security architectures that can adapt to evolving compliance requirements.
Key Innovations to Watch
Multimodal learning, resource-efficient AI, transparent decision systems. Organizations should monitor these developments closely to maintain competitive advantages and effective security postures.
Strategic investments in research partnerships, technology pilots, and talent development will position forward-thinking organizations to leverage these innovations early in their development cycle.
Technical Glossary
Key technical terms and definitions to help understand the technologies discussed in this article.
Understanding the following technical concepts is essential for grasping the full implications of the security threats and defensive measures discussed in this article. These definitions provide context for both technical and non-technical readers.