Nanoprinter turns Meta’s AI predictions into potentially game-changing materials - Related to game-changing, india’s, startup, meta’s, first
Introduction to Minimum Cost Flow Optimization in Python
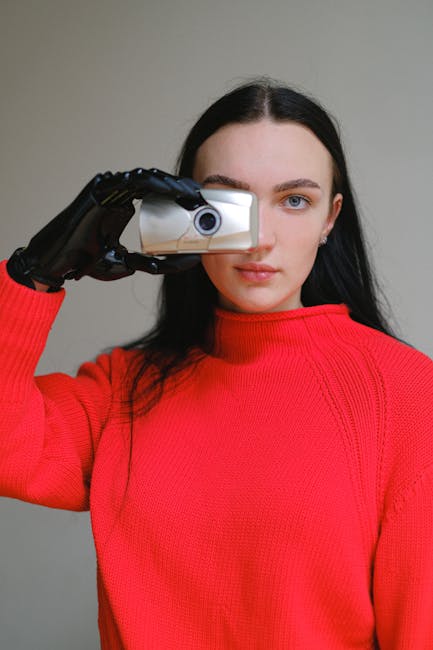
Minimum cost flow optimization minimizes the cost of moving flow through a network of nodes and edges. Nodes include data (supply) and sinks (demand), with different costs and capacity limits. The aim is to find the least costly way to move volume from data to sinks while adhering to all capacity limitations.
Applications of minimum cost flow optimization are vast and varied, spanning multiple industries and sectors. This approach is crucial in logistics and supply chain management, where it is used to minimize transportation costs while ensuring timely delivery of goods. In telecommunications, it helps in optimizing the routing of data through networks to reduce latency and improve bandwidth utilization. The energy sector leverages minimum cost flow optimization to efficiently distribute electricity through power grids, reducing losses and operational costs. Urban planning and infrastructure development also benefit from this optimization technique, as it assists in designing efficient public transportation systems and water distribution networks.
Below is a simple flow optimization example:
The image above illustrates a minimum cost flow optimization problem with six nodes and eight edges. Nodes A and B serve as insights, each with a supply of 50 units, while nodes E and F act as sinks, each with a demand of 40 units. Every edge has a maximum capacity of 25 units, with variable costs indicated in the image. The objective of the optimization is to allocate flow on each edge to move the required units from nodes A and B to nodes E and F, respecting the edge capacities at the lowest possible cost.
Node F can only receive supply from node B. There are two paths: directly or through node D. The direct path has a cost of 2, while the indirect path via D has a combined cost of 3. Thus, 25 units (the maximum edge capacity) are moved directly from B to F. The remaining 15 units are routed via B -D-F to meet the demand.
Currently, 40 out of 50 units have been transferred from node B, leaving a remaining supply of 10 units that can be moved to node E. The available pathways for supplying node E include: A-E and B-E with a cost of 3, A-C-E with a cost of 4, and B-C-E with a cost of 5. Consequently, 25 units are transported from A-E (limited by the edge capacity) and 10 units from B-E (limited by the remaining supply at node B). To meet the demand of 40 units at node E, an additional 5 units are moved via A-C-E, resulting in no flow being allocated to the B-C pathway.
I introduce two mathematical formulations of minimum cost flow optimization:
1. LP (linear program) with continuous variables only.
2. MILP (mixed integer linear program) with continuous and discrete variables.
This formulation only contains decision variables that are continuous, meaning they can have any value as long as all constraints are fulfilled. Decision variables are in this case the flow variables x(u, v) of all edges.
The objective function describes how the costs that are supposed to be minimized are calculated. In this case it is defined as the flow multiplied with the variable cost summed up over all edges:
Constraints are conditions that must be satisfied for the solution to be valid, ensuring that the flow does not exceed capacity limitations.
First, all flows must be non-negative and not exceed to edge capacities:
Flow conservation constraints ensure that the same amount of flow that goes into a node has to come out of the node. These constraints are applied to all nodes that are neither insights nor sinks:
For source and sink nodes the difference of out flow and in flow is smaller or equal the supply of the node:
If v is a source the difference of outflow minus inflow must not exceed the supply s(v). In case v is a sink node we do not allow that more than -s(v) can flow into the node than out of the node (for sinks s(v) is negative).
Additionally, to the continuous variables of the LP formulation, the MILP formulation also contains discreate variables that can only have specific values. Discrete variables allow to restrict the number of used nodes or edges to certain values. It can also be used to introduce fixed costs for using nodes or edges. In this article I show how to add fixed costs. It is essential to note that adding discrete decision variables makes it much more difficult to find an optimal solution, hence this formulation should only be used if a LP formulation is not possible.
With three terms: variable cost of all edges, fixed cost of all edges, and fixed cost of all nodes.
The maximum flow that can be allocated to an edge depends on the edge’s capacity, the edge selection variable, and the origin node selection variable:
This equation ensures that flow can only be assigned to edges if the edge selection variable and the origin node selection variable are 1.
The flow conservation constraints are equivalent to the LP problem.
In this section I explain how to implement a MILP optimization in Python. You can find the code in this repo.
To build the flow network, I used NetworkX which is an excellent library ([website]/) for working with graphs. There are many interesting articles that demonstrate how powerful and easy to use NetworkX is to work with graphs, [website] customizing NetworkX Graphs, NetworkX: Code Demo for Manipulating Subgraphs, Social Network Analysis with NetworkX: A Gentle Introduction.
One essential aspect when building an optimization is to make sure that the input is correctly defined. Even one small error can make the problem infeasible or can lead to an unexpected solution. To avoid this, I used Pydantic to validate the user input and raise any issues at the earliest possible stage. This article gives an easy to understand introduction to Pydantic.
To transform the defined network into a mathematical optimization problem I used PuLP. Which allows to define all variables and constraint in an intuitive way. This library also has the advantage that it can use many different solvers in a simple pug-and-play fashion. This article provides good introduction to this library.
The code below displays how nodes are defined:
from pydantic import BaseModel, model_validator from typing import Optional # node and edge definitions class Node(BaseModel, frozen=True): """ class of network node with attributes: name: str - name of node demand: float - demand of node (if node is sink) supply: float - supply of node (if node is source) capacity: float - maximum flow out of node type: str - type of node x: float - x-coordinate of node y: float - y-coordinate of node fixed_cost: float - cost of selecting node """ name: str demand: Optional[float] = [website] supply: Optional[float] = [website] capacity: Optional[float] = float('inf') type: Optional[str] = None x: Optional[float] = [website] y: Optional[float] = [website] fixed_cost: Optional[float] = [website] @model_validator(mode='after') def validate(self): """ validate if node definition are correct """ # check that demand is non-negative if [website] < 0 or [website] == float('inf'): raise ValueError('demand must be non-negative and finite') # check that supply is non-negative if [website] < 0: raise ValueError('supply must be non-negative') # check that capacity is non-negative if self.capacity < 0: raise ValueError('capacity must be non-negative') # check that fixed_cost is non-negative if self.fixed_cost < 0: raise ValueError('fixed_cost must be non-negative') return self.
Nodes are defined through the Node class which is inherited from Pydantic’s BaseModel. This enables an automatic validation that ensures that all properties are defined with the correct datatype whenever a new object is created. In this case only the name is a required input, all other properties are optional, if they are not provided the specified default value is assigned to them. By setting the “frozen” parameter to True I made all properties immutable, meaning they cannot be changed after the object has been initialized.
The validate method is executed after the object has been initialized and applies more checks to ensure the provided values are as expected. Specifically it checks that demand, supply, capacity, variable cost and fixed cost are not negative. Furthermore, it also does not allow infinite demand as this would lead to an infeasible optimization problem.
These checks look trivial, however their main benefit is that they will trigger an error at the earliest possible stage when an input is incorrect. Thus, they prevent creating a optimization model that is incorrect. Exploring why a model cannot be solved would be much more time consuming as there are many factors that would need to be analyzed, while such “trivial” input error may not be the first aspect to investigate.
class of edge between two nodes with attributes:
destination: 'Node' - destination node of edge.
capacity: float - maximum flow through edge.
variable_cost: float - cost per unit flow through edge.
fixed_cost: float - cost of selecting edge.
capacity: Optional[float] = float('inf').
variable_cost: Optional[float] = [website].
if [website] == [website] raise ValueError('origin and destination names must be different').
if self.capacity < 0: raise ValueError('capacity must be non-negative').
# check that variable_cost is non-negative.
if self.variable_cost < 0: raise ValueError('variable_cost must be non-negative').
if self.fixed_cost < 0: raise ValueError('fixed_cost must be non-negative').
The required inputs are an origin node and a destination node object. Additionally, capacity, variable cost and fixed cost can be provided. The default value for capacity is infinity which means if no capacity value is provided it is assumed the edge does not have a capacity limitation. The validation ensures that the provided values are non-negative and that origin node name and the destination node name are different.
To define the flowgraph and optimize the flow I created a new class called FlowGraph that is inherited from NetworkX’s DiGraph class. By doing this I can add my own methods that are specific to the flow optimization and at the same time use all methods DiGraph provides:
from networkx import DiGraph from pulp import LpProblem, LpVariable, LpMinimize, LpStatus class FlowGraph(DiGraph): """ class to define and solve minimum cost flow problems """ def __init__(self, nodes=[], edges=[]): """ initialize FlowGraph object :param nodes: list of nodes :param edges: list of edges """ # initialialize digraph super().__init__(None) # add nodes and edges for node in nodes: self.add_node(node) for edge in edges: self.add_edge(edge) def add_node(self, node): """ add node to graph :param node: Node object """ # check if node is a Node object if not isinstance(node, Node): raise ValueError('node must be a Node object') # add node to graph super().add_node([website], [website], [website], capacity=node.capacity, [website], fixed_cost=node.fixed_cost, [website], [website] def add_edge(self, edge): """ add edge to graph @param edge: Edge object """ # check if edge is an Edge object if not isinstance(edge, Edge): raise ValueError('edge must be an Edge object') # check if nodes exist if not [website] in super().nodes: self.add_node([website] if not [website] in super().nodes: self.add_node(edge.destination) # add edge to graph super().add_edge([website], [website], capacity=edge.capacity, variable_cost=edge.variable_cost, fixed_cost=edge.fixed_cost).
FlowGraph is initialized by providing a list of nodes and edges. The first step is to initialize the parent class as an empty graph. Next, nodes and edges are added via the methods add_node and add_edge. These methods first check if the provided element is a Node or Edge object. If this is not the case an error will be raised. This ensures that all elements added to the graph have passed the validation of the previous section. Next, the values of these objects are added to the Digraph object. Note that the Digraph class also uses add_node and add_edge methods to do so. By using the same method name I am overwriting these methods to ensure that whenever a new element is added to the graph it must be added through the FlowGraph methods which validate the object type. Thus, it is not possible to build a graph with any element that has not passed the validation tests.
The method below converts the network into an optimization model, solves it, and retrieves the optimized values.
def min_cost_flow(self, verbose=True): """ run minimum cost flow optimization @param verbose: bool - print optimization status (default: True) @return: status of optimization """ self.verbose = verbose # get maximum flow self.max_flow = sum(node['demand'] for _, node in super()[website] if node['demand'] > 0) start_time = [website] # create LP problem [website] = LpProblem("FlowGraph.min_cost_flow", LpMinimize) # assign decision variables self._assign_decision_variables() # assign objective function self._assign_objective_function() # assign constraints self._assign_constraints() if self.verbose: print(f"Model creation time: {[website] - [website]} s") start_time = [website] # solve LP problem [website] solve_time = [website] - start_time # get status status = LpStatus[[website]] if verbose: # print optimization status if status == 'Optimal': # get objective value objective = [website] print(f"Optimal solution found: {[website]} in {[website]} s") else: print(f"Optimization status: {status} in {[website]} s") # assign variable values self._assign_variable_values(status=='Optimal') return status.
Pulp’s LpProblem is initialized, the constant LpMinimize defines it as a minimization problem — meaning it is supposed to minimize the value of the objective function. In the following lines all decision variables are initialized, the objective function as well as all constraints are defined. These methods will be explained in the following sections.
Next, the problem is solved, in this step the optimal value of all decision variables is determined. Following the status of the optimization is retrieved. When the status is “Optimal” an optimal solution could be found other statuses are “Infeasible” (it is not possible to fulfill all constraints), “Unbounded” (the objective function can have an arbitrary low values), and “Undefined” meaning the problem definition is not complete. In case no optimal solution was found the problem definition needs to be reviewed.
Finally, the optimized values of all variables are retrieved and assigned to the respective nodes and edges.
All decision variables are initialized in the method below:
def _assign_variable_values(self, opt_found): """ assign decision variable values if optimal solution found, otherwise set to None @param opt_found: bool - if optimal solution was found """ # assign edge values for _, _, edge in super()[website] # initialize values edge['flow'] = None edge['selected'] = None # check if optimal solution found if opt_found and edge['flow_var'] is not None: edge['flow'] = edge['flow_var'].varValue if edge['selection_var'] is not None: edge['selected'] = edge['selection_var'].varValue # assign node values for _, node in super()[website] # initialize values node['selected'] = None if opt_found: # check if node has selection variable if node['selection_var'] is not None: node['selected'] = node['selection_var'].varValue.
First it iterates through all edges and assigns continuous decision variables if the edge capacity is greater than 0. Furthermore, if fixed costs of the edge are greater than 0 a binary decision variable is defined as well. Next, it iterates through all nodes and assigns binary decision variables to nodes with fixed costs. The total number of continuous and binary decision variables is counted and printed at the end of the method.
After all decision variables have been initialized the objective function can be defined:
def _assign_objective_function(self): """ define objective function """ objective = 0 # add edge costs for _, _, edge in super()[website] if edge['selection_var'] is not None: objective += edge['selection_var'] * edge['fixed_cost'] if edge['flow_var'] is not None: objective += edge['flow_var'] * edge['variable_cost'] # add node costs for _, node in super()[website] # add node selection costs if node['selection_var'] is not None: objective += node['selection_var'] * node['fixed_cost'] [website] += objective, 'Objective',.
The objective is initialized as 0. Then for each edge fixed costs are added if the edge has a selection variable, and variable costs are added if the edge has a flow variable. For all nodes with selection variables fixed costs are added to the objective as well. At the end of the method the objective is added to the LP object.
All constraints are defined in the method below:
def _assign_constraints(self): """ define constraints """ # count of contraints constr_count = 0 # add capacity constraints for edges with fixed costs for origin_name, destination_name, edge in super()[website] # get capacity capacity = edge['capacity'] if edge['capacity'] < float('inf') else self.max_flow rhs = capacity if edge['selection_var'] is not None: rhs *= edge['selection_var'] [website] += edge['flow_var'] <= rhs, f"capacity_{origin_name}-{destination_name}", constr_count += 1 # get origin node origin_node = super().nodes[origin_name] # check if origin node has a selection variable if origin_node['selection_var'] is not None: rhs = capacity * origin_node['selection_var'] [website] += (edge['flow_var'] <= rhs, f"node_selection_{origin_name}-{destination_name}",) constr_count += 1 total_demand = total_supply = 0 # add flow conservation constraints for node_name, node in super()[website] # aggregate in and out flows in_flow = 0 for _, _, edge in super().in_edges(node_name, data=True): if edge['flow_var'] is not None: in_flow += edge['flow_var'] out_flow = 0 for _, _, edge in super().out_edges(node_name, data=True): if edge['flow_var'] is not None: out_flow += edge['flow_var'] # add node capacity contraint if node['capacity'] < float('inf'): [website] += out_flow <= node['capacity'], f"node_capacity_{node_name}", constr_count += 1 # check what type of node it is if node['demand'] == node['supply']: # transshipment node: in_flow = out_flow [website] += in_flow == out_flow, f"flow_balance_{node_name}", else: # in_flow - out_flow >= demand - supply rhs = node['demand'] - node['supply'] [website] += in_flow - out_flow >= rhs, f"flow_balance_{node_name}", constr_count += 1 # update total demand and supply total_demand += node['demand'] total_supply += node['supply'] if self.verbose: print(f"Constraints: {constr_count}") print(f"Total supply: {total_supply}, Total demand: {total_demand}").
First, capacity constraints are defined for each edge. If the edge has a selection variable the capacity is multiplied with this variable. In case there is no capacity limitation (capacity is set to infinity) but there is a selection variable, the selection variable is multiplied with the maximum flow that has been calculated by aggregating the demand of all nodes. An additional constraint is added in case the edge’s origin node has a selection variable. This constraint means that flow can only come out of this node if the selection variable is set to 1.
Following, the flow conservation constraints for all nodes are defined. To do so the total in and outflow of the node is calculated. Getting all in and outgoing edges can easily be done by using the in_edges and out_edges methods of the DiGraph class. If the node has a capacity limitation the maximum outflow will be constraint by that value. For the flow conservation it is necessary to check if the node is either a source or sink node or a transshipment node (demand equals supply). In the first case the difference between inflow and outflow must be greater or equal the difference between demand and supply while in the latter case in and outflow must be equal.
The total number of constraints is counted and printed at the end of the method.
After running the optimization, the optimized variable values can be retrieved with the following method:
def _assign_variable_values(self, opt_found): """ assign decision variable values if optimal solution found, otherwise set to None @param opt_found: bool - if optimal solution was found """ # assign edge values for _, _, edge in super()[website] # initialize values edge['flow'] = None edge['selected'] = None # check if optimal solution found if opt_found and edge['flow_var'] is not None: edge['flow'] = edge['flow_var'].varValue if edge['selection_var'] is not None: edge['selected'] = edge['selection_var'].varValue # assign node values for _, node in super()[website] # initialize values node['selected'] = None if opt_found: # check if node has selection variable if node['selection_var'] is not None: node['selected'] = node['selection_var'].varValue.
This method iterates through all edges and nodes, checks if decision variables have been assigned and adds the decision variable value via varValue to the respective edge or node.
To demonstrate how to apply the flow optimization I created a supply chain network consisting of 2 factories, 4 distribution centers (DC), and 15 markets. All goods produced by the factories have to flow through one distribution center until they can be delivered to the markets.
Ranges mean that uniformly distributed random numbers were generated to assign these properties. Since Factories and DCs have fixed costs the optimization also needs to decide which of these entities should be selected.
Edges are generated between all Factories and DCs, as well as all DCs and Markets. The variable cost of edges is calculated as the Euclidian distance between origin and destination node. Capacities of edges from Factories to DCs are set to 350 while from DCs to Markets are set to 100.
The code below presents how the network is defined and how the optimization is run:
# Define nodes factories = [Node(name=f'Factory {i}', supply=700, type='Factory', fixed_cost=100, x=random.uniform(0, 2), y=random.uniform(0, 1)) for i in range(2)] dcs = [Node(name=f'DC {i}', fixed_cost=25, capacity=500, type='DC', x=random.uniform(0, 2), y=random.uniform(0, 1)) for i in range(4)] markets = [Node(name=f'Market {i}', demand=random.randint(1, 100), type='Market', x=random.uniform(0, 2), y=random.uniform(0, 1)) for i in range(15)] # Define edges edges = [] # Factories to DCs for factory in factories: for dc in dcs: distance = (([website] - [website]**2 + ([website] - [website]**2)**[website] [website], destination=dc, capacity=350, variable_cost=distance)) # DCs to Markets for dc in dcs: for market in markets: distance = (([website] - [website]**2 + ([website] - [website]**2)**[website] [website], destination=market, capacity=100, variable_cost=distance)) # Create FlowGraph G = FlowGraph(edges=edges) G.min_cost_flow().
The output of flow optimization is as follows:
Variable types: 68 continuous, 6 binary Constraints: 161 Total supply: [website], Total demand: [website] Model creation time: [website] s Optimal solution found: [website] in [website] s.
The problem consists of 68 continuous variables which are the edges’ flow variables and 6 binary decision variables which are the selection variables of the Factories and DCs. There are 161 constraints in total which consist of edge and node capacity constraints, node selection constraints (edges can only have flow if the origin node is selected), and flow conservation constraints. The next line displays that the total supply is 1400 which is higher than the total demand of 909 (if the demand was higher than the supply the problem would be infeasible). Since this is a small optimization problem, the time to define the optimization model was less than [website] seconds. The last line displays that an optimal solution with an objective value of 1335 could be found in [website] seconds.
Additionally, to the code I described in this post I also added two methods that visualize the optimized solution. The code of these methods can also be found in the repo.
All nodes are located by their respective x and y coordinates. The node and edge size is relative to the total volume that is flowing through. The edge color refers to its utilization (flow over capacity). Dashed lines show edges without flow allocation.
In the optimal solution both Factories were selected which is inevitable as the maximum supply of one Factory is 700 and the total demand is 909. However, only 3 of the 4 DCs are used (DC 0 has not been selected).
In general the plot displays the Factories are supplying the nearest DCs and DCs the nearest Markets. However, there are a few exceptions to this observation: Factory 0 also supplies DC 3 although Factory 1 is nearer. This is due to the capacity constraints of the edges which only allow to move at most 350 units per edge. However, the closest Markets to DC 3 have a slightly higher demand, hence Factory 0 is moving additional units to DC 3 to meet that demand. Although Market 9 is closest to DC 3 it is supplied by DC 2. This is because DC 3 would require an additional supply from Factory 0 to supply this market and since the total distance from Factory 0 over DC 3 is longer than the distance from Factory 0 through DC 2, Market 9 is supplied via the latter route.
Another way to visualize the results is via a Sankey diagram which focuses on visualizing the flows of the edges:
The colors represent the edges’ utilizations with lowest utilizations in green changing to yellow and red for the highest utilizations. This diagram reveals very well how much flow goes through each node and edge. It highlights the flow from Factory 0 to DC 3 and also that Market 13 is supplied by DC 2 and DC 1.
Minimum cost flow optimizations can be a very helpful tool in many domains like logistics, transportation, telecommunication, energy sector and many more. To apply this optimization it is key to translate a physical system into a mathematical graph consisting of nodes and edges. This should be done in a way to have as few discrete ([website] binary) decision variables as necessary as those make it significantly more difficult to find an optimal solution. By combining Python’s NetworkX, Pulp and Pydantic libraries I built an flow optimization class that is intuitive to initialize and at the same time follows a generalized formulation which allows to apply it in many different use cases. Graph and flow diagrams are very helpful to understand the solution found by the optimizer.
If not otherwise stated all images were created by the author.
Amazon might soon reveal the next generation of Alexa, one equipped with AI skills. At a press event scheduled for Wednesday, Feb. 26, the comp......
Can you jailbreak Anthropic's latest AI safety measure? Researchers want you to try -- and are offering up to $20,000 if you suc......
In October 2023, former president Joe Biden signed an executive order that included several measures for regulating AI. On his ......
This Hyderabad Startup is Building India’s First AI Lab in Orbit
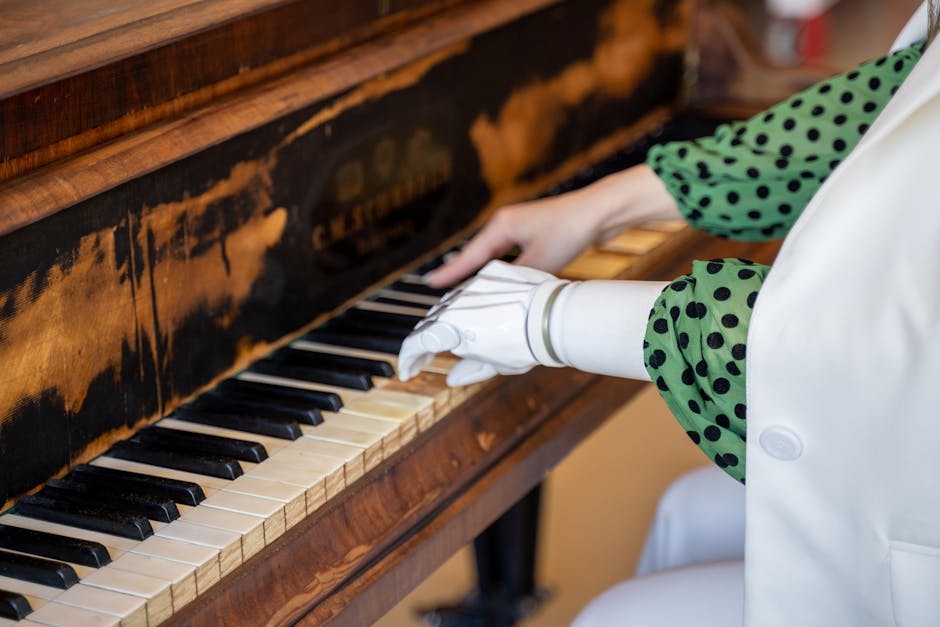
TakeMe2Space, a Hyderabad-based space-tech startup, is aiming to make space more accessible by launching India’s first AI-driven space laboratory. Founded by Ronak Kumar Samantray, the corporation is working to change the way people interact with satellites.
Unlike traditional models where satellite access is restricted to governments, defence agencies, or elite research institutions, TakeMe2Space wants to democratise space, offering real-time access to satellites for students, researchers, and businesses alike.
“Our goal is to ensure that everybody’s ideas can be taken to space,” Samantray told AIM in an . “You don’t have to be in NASA, ISRO, or an IIT to run an experiment in space. Sitting in Kerala, Delhi, or even Antarctica, you should be able to operate a satellite.”.
The firm not long ago conducted a technology demonstration mission with ISRO, proving the viability of its approach. Now, the next step is launching two fully operational satellites this year, with a long-term ambition to build the future of computing in orbit.
Samantray (second from the left), along with former chairman of ISRO, S. Somanath.
As of last year, the Indian space economy was valued at approximately $[website] billion, constituting a 2% share of the global space market. The country currently operates 56 active space assets, including 19 communication satellites, nine navigation satellites, four scientific satellites, and 24 earth observation satellites, as per the economic survey 2024-25.
With the government aiming to scale the space economy to £44 billion by 2033, inclusive of £11 billion in exports, which would represent 7-8% of the global share, TakeMe2Space believes that accessing space should be as simple as logging into a cloud computing service.
Space Can be Hands-On for the Next Generation.
Samantray’s motivation for TakeMe2Space comes from his background in computer science. Growing up, he had easy access to computers, which nurtured his love for coding. However, he observed that space has remained largely inaccessible to young minds.
“If you’re interested in space, the most you can do today is read a research paper or maybe play with an electronics kit,” he explained. “Nobody gets to task their own satellite.”.
“Just like how schools have computer labs, electronics labs, and robotics labs, we believe there should be a satellite lab,” showcased Samantray. “Our satellites will be openly accessible for students to run their personal experiments.”.
Samantray (left) at the Sriharikota launchpad.
So far, the education sector has shown interest, but surprisingly, most early adopters are not universities. Out of 20 individuals who have signed up, only four are from the education sector, while the remaining 16 are from GIS (geographic information systems) and data analytics companies.
From a business point of view, the enterprise provides “for ₹20,000 in the pricing, 90 minutes of the satellite time in orbit.”.
TakeMe2Space payload on board the ISRO SpaDeX Mission.
AI in Space is More Than Just Data Collection.
The integration of AI in TakeMe2Space’s model is a key differentiator. Traditionally, satellites capture raw data, which is then processed on Earth. But AI-driven satellites can process images in orbit, making decisions on what data to collect and download.
One experiment conducted on TakeMe2Space’s AI lab by the University of Southampton involved using a low-power AI algorithm to reduce motion blur in satellite images. “A satellite moves at 7 km per second, so capturing a clear image is a challenge,” stated Samantray. “Instead of using traditional pointing and staring techniques, AI can remove motion blur in real-time.”.
Samantray and the team working on the payload at TakeMe2Space.
AI also allows for real-time object detection and change detection. This means satellites can prioritise what images to capture and transmit, reducing unnecessary data transmission and saving bandwidth.
“Our aim is not just to provide satellite data,” Samantray emphasised. “We want to give people control of the satellite itself.”.
With TakeMe2Space offering satellite access to a broader audience, concerns about data security and ethical usage naturally arise.
Allowing individuals and businesses to task satellites in real time raises questions about privacy, misuse, and cybersecurity risks. Samantray acknowledged these challenges and detailed the safeguards the enterprise has implemented.
“We are enabling people to control a satellite, which means we have to be two steps ahead in terms of security,” he stated. “Our system is designed to preemptively stop any harmful actions before they occur, rather than reacting after the fact.”.
To prevent unauthorised activities, TakeMe2Space does not equip its satellites with propulsion systems, ensuring they cannot be hijacked and redirected toward other objects.
Additionally, the business has capped the resolution of its satellite imagery at no finer than 5 meters per pixel, preventing privacy violations. “Even if something goes wrong, no one can use our satellite for surveillance or for interfering with other space objects,” Samantray assured.
On the data front, TakeMe2Space follows strict encryption protocols. individuals retain ownership of the data they generate, and the organization does not store or claim rights over it. “We are like an infrastructure provider, similar to how AWS doesn’t own the applications running on its servers,” he explained.
Building the Future of Space Computing in India.
As reiterated by the founder, TakeMe2Space does not intend to compete with conventional Earth observation firms. Rather, it envisions a future in which computing transitions to space.
“We’re not here to be another Earth observation firm,” revealed Samantray. “We want to build data centres in orbit where AI and computing happen in space, not on Earth.”.
By shifting heavy computation tasks to satellites, TakeMe2Space aims to reduce Earth’s power consumption. The world’s increasing reliance on AI, data storage, and cloud computing is driving exponential energy demand.
Running AI models in space, where temperatures are extremely cold and heat dissipation is more efficient, could be a long-term solution.
“Space gives you a very controlled and predictable temperature environment, and whatever heat you generate up there has no impact on Earth’s atmosphere. The absolute temperature of any point in space is 4 Kelvin, so as much heat as you generate, it absorbs the heat, which will just be a point of heat for space.”.
Looking ahead, TakeMe2Space hopes to scale its model, expanding beyond AI labs to full-fledged space computing infrastructure. The business is not reliant on government funding but sees the private sector as its primary market. “We’re building for private businesses, not just defence or government consumers,” Samantray clarified.
If it succeeds, the startup may redefine global interactions with satellites, making space an accessible laboratory for everyone.
In the digital content world, video has become the dominant format, and with that, video compression is more critical than ever. Speaking at India’s B...
lately, DeepSeek introduced their latest model, R1, and article after article came out praising its performance relative to cost, and how the release...
Google DeepMind spinoff Isomorphic Labs expects testing on its first AI-designed drugs to begin this year, as tech startups race to turn algorithmic m...
Nanoprinter turns Meta’s AI predictions into potentially game-changing materials
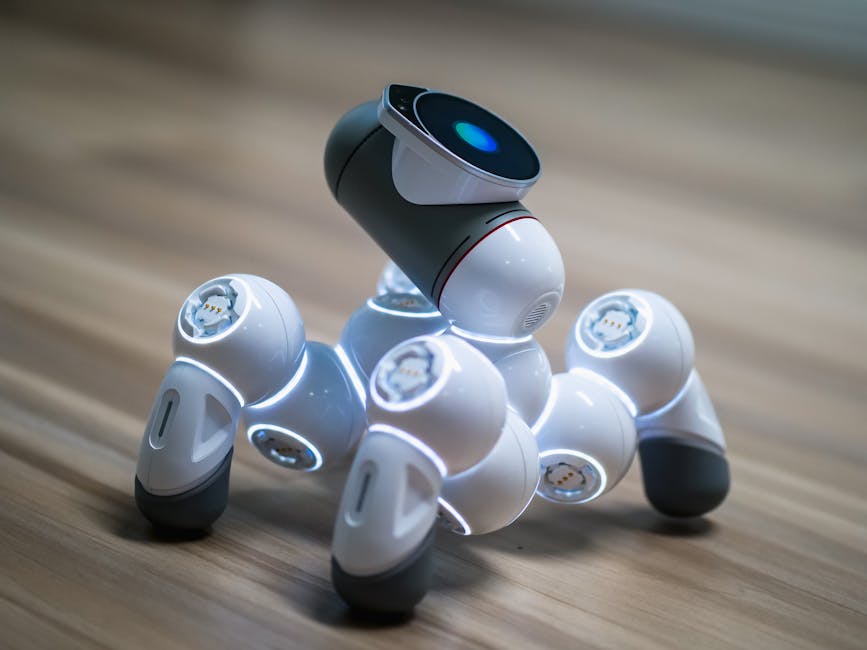
For the past few months, Meta has been sending recipes to a Dutch scaleup called VSParticle (VSP). These are not food recipes — they’re AI-generated instructions for how to make new nanoporous materials that could potentially supercharge the green transition.
VSP has so far taken 525 of these recipes and synthesised them into nanomaterials called electrocatalysts. Meta’s algorithms predicted these electrocatalysts would be ideal for breaking down CO2 into useful products like methane or ethanol. VSP brought the AI predictions to life using a nanoprinter, a machine which vaporises materials and then deposits them as thin nanoporous films.
Electrocatalysts speed up chemical reactions that involve electricity, such as splitting water into hydrogen and oxygen, converting CO2 into fuels, or generating power in fuel cells. They make these processes more efficient, reducing the energy required and enabling clean energy technologies like hydrogen production and advanced batteries.
The problem is that it typically takes scientists up to 15 years just to create one new nanomaterial — until now.
“We’ve synthesised, tested, and validated hundreds of nanomaterials at a scale and speed never seen before,” Aaike van Vugt, co-founder and CEO of VSP, told TNW. “This rapid prototyping gives researchers a quick way to validate AI predictions and discover low-cost electrocatalysts that might have taken years or even decades to find using traditional methods.”.
VSP put each batch of the new materials in an envelope and shipped it to a lab at the University of Toronto for testing. The findings were then integrated into an open-source experimental database, which can now be used to train AI models to become more more effective at predicting new material combinations.
Larry Zitnick, Research Director at Meta AI, expressed the research is “breaking new ground” in material discovery. “It marks a significant leap in our ability to predict and validate materials that are critical for clean energy solutions,” he expressed.
The Alphafold of nanomaterial discovery?
But to really crack the code for material discovery, AI models need to be trained on much larger datasets. Not hundreds but tens or even hundreds of thousands of tested materials.
Van Vugt showcased that VSP’s machine is the only technology available today that could synthesize such a large number of thin-film nanoporous materials in a reasonable time frame — about two to three years, showcased the founder.
“This could create an AI that is the equivalent of Google Deepmind’s Alphafold, but for nanoporous materials,” stated Van Vugt. He’s referring, of course, to the breakthrough algorithm that cracked a puzzle in protein biology that had confounded scientists for centuries.
If that’s true, then it puts the enterprise in a pretty sweet position. The world’s tech giants — think Google, Microsoft, Meta — are all racing to build bigger, improved forms of artificial intelligence in a bid to find solutions to some of the world’s greatest challenges, including climate change. Ironically, these models could also think up solutions for their endless appetite for energy. For companies like Meta, investing in material discovery using AI is a win-win.
VSP is working with many other organisations to build out its dataset and mature its technology. These include the Sorbonne University Abu Dhabi, the San Francisco-based Lawrence Livermore National Laboratory, the Materials Discovery Research Institute (MDRI) in the Chicago area, and the Dutch Institute for Fundamental Energy Research (DIFFER).
The Dutch firm is also fine-tuning its nanoprinters to be faster and more efficient. The current machines are powered by 300 sparks per second, but the team is working on a new printer that would increase this output time to 20,000 sparks per second. This could supercharge material discovery even further.
AI-driven technologies are weaving themselves into the fabric of our daily routines, with the potential to enhance our access to knowledge and boost o...
Amsterdam-headquartered Nebius, which builds full-stack AI infrastructure for tech firms, has secured $700mn in a private equity deal led by Nvidia, A...
OpenAI released its o3-mini model exactly one week ago, offering both free and paid people a more accurate, faster, and cheaper alternative to o...
Market Impact Analysis
Market Growth Trend
2018 | 2019 | 2020 | 2021 | 2022 | 2023 | 2024 |
---|---|---|---|---|---|---|
23.1% | 27.8% | 29.2% | 32.4% | 34.2% | 35.2% | 35.6% |
Quarterly Growth Rate
Q1 2024 | Q2 2024 | Q3 2024 | Q4 2024 |
---|---|---|---|
32.5% | 34.8% | 36.2% | 35.6% |
Market Segments and Growth Drivers
Segment | Market Share | Growth Rate |
---|---|---|
Machine Learning | 29% | 38.4% |
Computer Vision | 18% | 35.7% |
Natural Language Processing | 24% | 41.5% |
Robotics | 15% | 22.3% |
Other AI Technologies | 14% | 31.8% |
Technology Maturity Curve
Different technologies within the ecosystem are at varying stages of maturity:
Competitive Landscape Analysis
Company | Market Share |
---|---|
Google AI | 18.3% |
Microsoft AI | 15.7% |
IBM Watson | 11.2% |
Amazon AI | 9.8% |
OpenAI | 8.4% |
Future Outlook and Predictions
The Introduction Minimum Cost landscape is evolving rapidly, driven by technological advancements, changing threat vectors, and shifting business requirements. Based on current trends and expert analyses, we can anticipate several significant developments across different time horizons:
Year-by-Year Technology Evolution
Based on current trajectory and expert analyses, we can project the following development timeline:
Technology Maturity Curve
Different technologies within the ecosystem are at varying stages of maturity, influencing adoption timelines and investment priorities:
Innovation Trigger
- Generative AI for specialized domains
- Blockchain for supply chain verification
Peak of Inflated Expectations
- Digital twins for business processes
- Quantum-resistant cryptography
Trough of Disillusionment
- Consumer AR/VR applications
- General-purpose blockchain
Slope of Enlightenment
- AI-driven analytics
- Edge computing
Plateau of Productivity
- Cloud infrastructure
- Mobile applications
Technology Evolution Timeline
- Improved generative models
- specialized AI applications
- AI-human collaboration systems
- multimodal AI platforms
- General AI capabilities
- AI-driven scientific breakthroughs
Expert Perspectives
Leading experts in the ai tech sector provide diverse perspectives on how the landscape will evolve over the coming years:
"The next frontier is AI systems that can reason across modalities and domains with minimal human guidance."
— AI Researcher
"Organizations that develop effective AI governance frameworks will gain competitive advantage."
— Industry Analyst
"The AI talent gap remains a critical barrier to implementation for most enterprises."
— Chief AI Officer
Areas of Expert Consensus
- Acceleration of Innovation: The pace of technological evolution will continue to increase
- Practical Integration: Focus will shift from proof-of-concept to operational deployment
- Human-Technology Partnership: Most effective implementations will optimize human-machine collaboration
- Regulatory Influence: Regulatory frameworks will increasingly shape technology development
Short-Term Outlook (1-2 Years)
In the immediate future, organizations will focus on implementing and optimizing currently available technologies to address pressing ai tech challenges:
- Improved generative models
- specialized AI applications
- enhanced AI ethics frameworks
These developments will be characterized by incremental improvements to existing frameworks rather than revolutionary changes, with emphasis on practical deployment and measurable outcomes.
Mid-Term Outlook (3-5 Years)
As technologies mature and organizations adapt, more substantial transformations will emerge in how security is approached and implemented:
- AI-human collaboration systems
- multimodal AI platforms
- democratized AI development
This period will see significant changes in security architecture and operational models, with increasing automation and integration between previously siloed security functions. Organizations will shift from reactive to proactive security postures.
Long-Term Outlook (5+ Years)
Looking further ahead, more fundamental shifts will reshape how cybersecurity is conceptualized and implemented across digital ecosystems:
- General AI capabilities
- AI-driven scientific breakthroughs
- new computing paradigms
These long-term developments will likely require significant technical breakthroughs, new regulatory frameworks, and evolution in how organizations approach security as a fundamental business function rather than a technical discipline.
Key Risk Factors and Uncertainties
Several critical factors could significantly impact the trajectory of ai tech evolution:
Organizations should monitor these factors closely and develop contingency strategies to mitigate potential negative impacts on technology implementation timelines.
Alternative Future Scenarios
The evolution of technology can follow different paths depending on various factors including regulatory developments, investment trends, technological breakthroughs, and market adoption. We analyze three potential scenarios:
Optimistic Scenario
Responsible AI driving innovation while minimizing societal disruption
Key Drivers: Supportive regulatory environment, significant research breakthroughs, strong market incentives, and rapid user adoption.
Probability: 25-30%
Base Case Scenario
Incremental adoption with mixed societal impacts and ongoing ethical challenges
Key Drivers: Balanced regulatory approach, steady technological progress, and selective implementation based on clear ROI.
Probability: 50-60%
Conservative Scenario
Technical and ethical barriers creating significant implementation challenges
Key Drivers: Restrictive regulations, technical limitations, implementation challenges, and risk-averse organizational cultures.
Probability: 15-20%
Scenario Comparison Matrix
Factor | Optimistic | Base Case | Conservative |
---|---|---|---|
Implementation Timeline | Accelerated | Steady | Delayed |
Market Adoption | Widespread | Selective | Limited |
Technology Evolution | Rapid | Progressive | Incremental |
Regulatory Environment | Supportive | Balanced | Restrictive |
Business Impact | Transformative | Significant | Modest |
Transformational Impact
Redefinition of knowledge work, automation of creative processes. This evolution will necessitate significant changes in organizational structures, talent development, and strategic planning processes.
The convergence of multiple technological trends—including artificial intelligence, quantum computing, and ubiquitous connectivity—will create both unprecedented security challenges and innovative defensive capabilities.
Implementation Challenges
Ethical concerns, computing resource limitations, talent shortages. Organizations will need to develop comprehensive change management strategies to successfully navigate these transitions.
Regulatory uncertainty, particularly around emerging technologies like AI in security applications, will require flexible security architectures that can adapt to evolving compliance requirements.
Key Innovations to Watch
Multimodal learning, resource-efficient AI, transparent decision systems. Organizations should monitor these developments closely to maintain competitive advantages and effective security postures.
Strategic investments in research partnerships, technology pilots, and talent development will position forward-thinking organizations to leverage these innovations early in their development cycle.
Technical Glossary
Key technical terms and definitions to help understand the technologies discussed in this article.
Understanding the following technical concepts is essential for grasping the full implications of the security threats and defensive measures discussed in this article. These definitions provide context for both technical and non-technical readers.