Triangle Forecasting: Why Traditional Impact Estimates Are Inflated (And How to Fix Them) - Related to nouveau, impact, how, :, combinator
OpenAI change d’identité visuelle : nouveau logo et nouvelle police
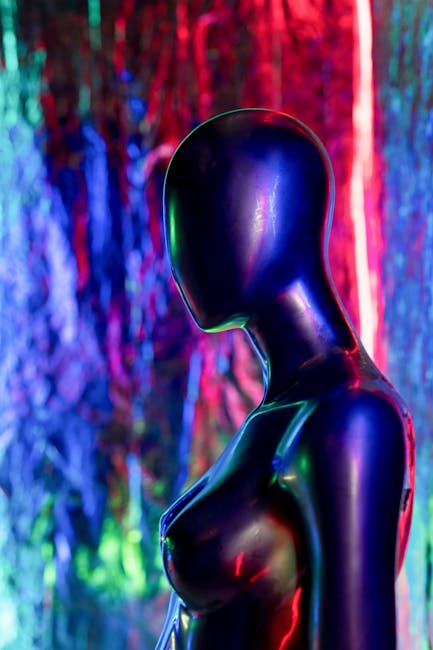
Ça fait environ 10 ans qu’OpenAI a été créé et depuis ce temps, l’entreprise a rarement effectué de mises à jour interne. Sauf il y a deux jours où OpenAI a fait une refonte totale en proposant un nouveau logo et une police personnalisée. Mais je n’ai pas constaté aucun changement drastique mis à part ces éléments.
On peut même dire qu’il ne s’agit que de quelques changements subtils. Autrement dit, une typographie renouvelée, un logotype modernisé, un emblème repensé et des couleurs actualisées.
Malgré ces changements et cette refonte, OpenAI a tout de même souhaité préserver sa familiarité, tout en optant pour la modernité.
Elle a même mis à disposition cette documentation détaillée en ligne. Ces ressources permettent à l’ensemble de son écosystème de s’approprier la nouvelle identité visuelle.
Un rebranding pour une identité visuelle plus humaine.
Dans un entretien avec le magazine Wallpaper , les responsables du design d’OpenAI, Veit Moeller et Shannon Jager, ont dévoilé les motivations derrière ce changement d’identité visuelle d’OpenAI.
Cette refonte, initiée à la demande du PDG Sam Altman qui souhaitait insuffler plus d’humanité à la marque, était devenue nécessaire pour harmoniser une communication jusqu’à des niveaux disparates en termes de typographie, logos et couleurs.
Ce projet d’envergure, développé sur plus d’une année, s’est notamment concrétisé par la création d’une police propriétaire, OpenAI Sans.
L’entreprise a également enrichi sa bibliothèque visuelle en intégrant des œuvres de photographes renommés et des créations abstraites générées par Sora, son propre modèle d’IA.
Cette évolution de la marque se reflète avant tout par la croissance spectaculaire de ChatGPT.
What are your thoughts on OpenAI’s new logo and font?
The organization has just rolled out a more modern sans-serif look. Images credit: OpenAI [website] — InsideTelecom (@insidetelecom_) February 5, 2025.
Conçu à l’origine comme un projet de recherche, l’assistant conversationnel a rapidement conquis le public.
Pour preuve, le chatbot a atteint le million d’utilisateurs en seulement cinq jours après son lancement.
Aujourd’hui encore, son succès ne s’est pas démenti. Seulement en 2024, ChatGPT compte plus de 300 millions d’utilisateurs actifs hebdomadaires.
Le nouveau logo d’OpenAI, un motif floral à trois triangles.
À titre d’information, la refonte d’OpenAI a été effectuée sans l’intervention d’une agence externe.
Big news: OpenAI just unveiled their new logo! Check out the fresh design I had the honor of creating. What do you think? 😉 [website] — Vadim Carazan (@VadimCarazan) April 1, 2024.
Cela prouve l’engagement d’OpenAI à garder et à maintenir son engagement envers la philosophie qu’elle a adoptée.
C’est-à-dire l’enrichissement de la créativité humaine, et non sa substitution. Et à travers des visuels qui font echo et une typographie évocatrice qu’OpenAI espère atteindre cet objectif.
Cela dit, cette nouvelle identité visuelle d’OpenAI introduit un logo « blossom » qui fait référence à un motif floral constitué de trois triangles entrelacés.
Quant à ChatGPT, il a juste servi au calcul de l’épaisseur des caractères. La refonte d’OpenAI a été réalisée de façon traditionnelle.
We leverage two key techniques to aid convergence of this ill-posed problem. The first is a very lightweight, dynamically trained convolutional neural...
It’s been a great year for the Dutch startup ecosystem.
Venture capitalists have, so far, invested $[website] into Netherlands-based early-stage companie...
Amsterdam-headquartered Nebius, which builds full-stack AI infrastructure for tech firms, has secured $700mn in a private equity deal led by Nvidia, A...
Y Combinator Announces its First AI Startup School
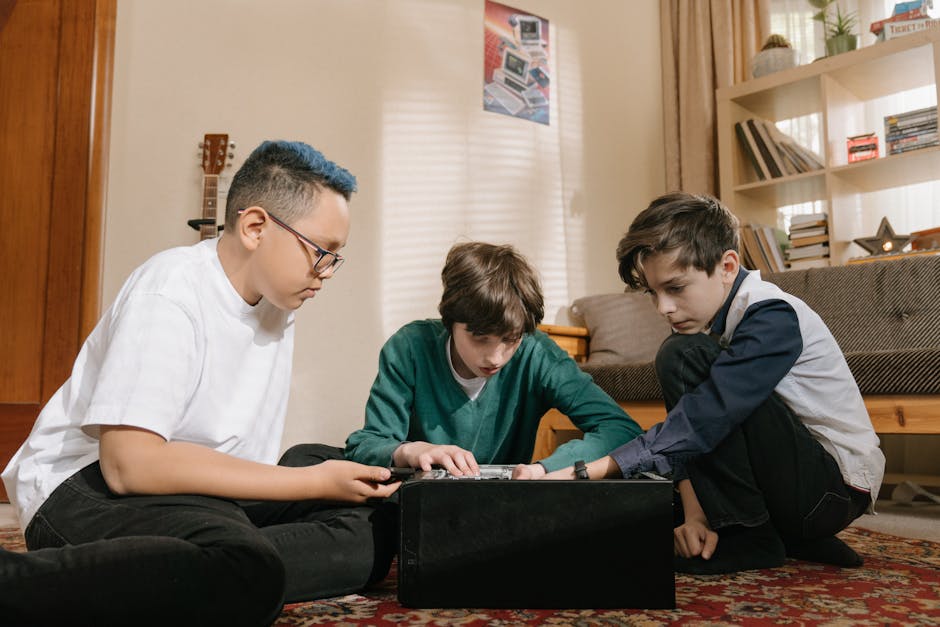
SF-based startup accelerator Y Combinator is launching its inaugural AI Startup School on 16-17 June in San Francisco. The event will bring together 2,000 top AI students and researchers.
“I’m proud to announce YC’s first AI Startup School,” wrote Garry Tan, CEO and president of YC, on Linkedin. “It’s a totally free conference bringing together the next top CS and AI minds in the world.”.
The event will feature leaders including Tesla CEO Elon Musk, OpenAI CEO Sam Altman, OpenAI co-founder Andrej Karpathy, and World Labs CEO Fei-Fei Li, among others, who will share insights on building the future of AI.
The event is open to final-year undergraduates, master’s and PhD candidates in AI, as well as recent graduates in fields such as computer science, applied math, robotics, and AI research.
, YC will also cover up to $500 for airfare and assist attendees in finding accommodation with SF-based founders.
Last month, YC also revealed applications for its first-ever Spring 2025 batch. The deadline to apply is Feb 11, 2025, at 8 pm PT. Applicants will receive a response by March 12.
In September last year, Tan introduced that the startup accelerator was expanding to four batches a year. Traditionally, batches ran between June and September (Summer Batch) and January and April (Winter Batch), but from 2024 onwards, it has added the Spring and Fall sessions.
Founded in 2005, YC is one of the most sought-after places for entrepreneurs worldwide. Since then, YC has invested in over 4,500 companies, including Airbnb, Meesho, Zepto, Dropbox, Stripe, and Reddit. Since 2022, the startup accelerator’s standard deal has been to invest $500,000 in each selected startup.
UK startup PhysicsX, founded by former Formula 1 engineering whizz Robin “Dr. Rob” Tuluie, has unveiled an AI tool that could fast-track the time it t...
Google DeepMind has made available Gemini [website] Flash and introduced new models, including Gemini [website] Pro Experimental and Gemini [website] Flash-Lite. These ...
Are you ready for a world where AI agents work alongside humans, not just as tools but as decision-makers? Imagine walking into your favourite coffee ...
Triangle Forecasting: Why Traditional Impact Estimates Are Inflated (And How to Fix Them)
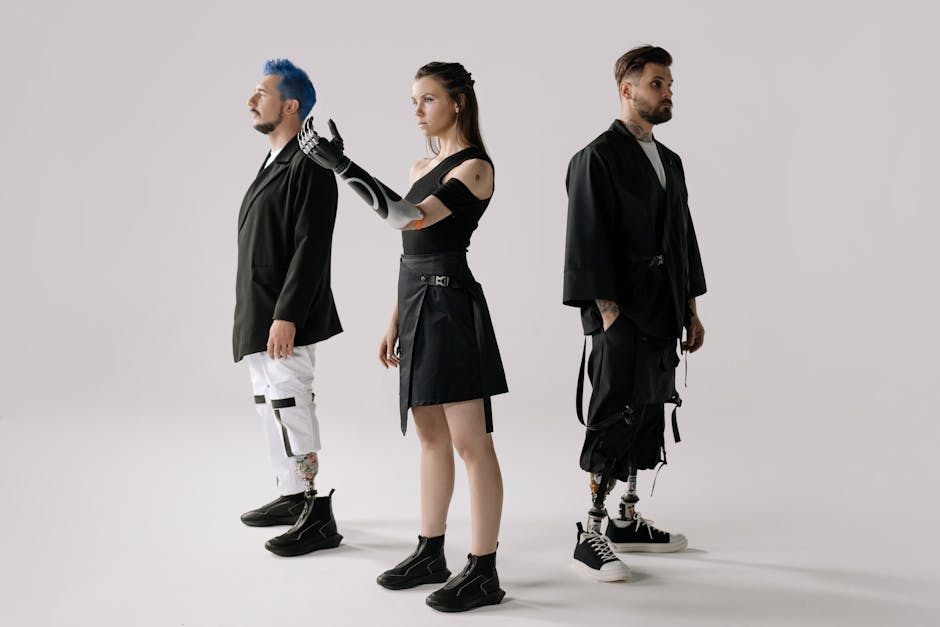
Accurate impact estimations can make or break your business case.
Yet, despite its importance, most teams use oversimplified calculations that can lead to inflated projections. These shot-in-the-dark numbers not only destroy credibility with stakeholders but can also result in misallocation of resources and failed initiatives. But there’s a improved way to forecast effects of gradual customer acquisition, without requiring messy Excel spreadsheets and formulas that error out.
By the end of this article, you will be able to calculate accurate yearly forecasts and implement a scalable Python solution for Triangle Forecasting.
When asked for annual impact estimations, product teams routinely overestimate impact by applying a one-size-fits-all approach to customer cohorts. Teams frequently opt for a simplistic approach:
Multiply monthly revenue (or any other relevant metric) by twelve to estimate annual impact.
While the calculation is easy, this formula ignores a fundamental premise that applies to most businesses:
Customer acquisition happens gradually throughout the year.
The contribution from all end-clients to yearly estimates is not equal since later cohorts contribute fewer months of revenue.
Triangle Forecasting can cut projection errors by accounting for effects of customer acquisition timelines.
Let us explore this concept with a basic example. Let’s say you’re launching a new subscription service:
Monthly subscription fee: $100 per customer.
Monthly customer acquisition target: 100 new end-people.
Goal: Calculate total revenue for the year.
An oversimplified multiplication indicates a revenue of $1,440,000 in the first year (= 100 new clients/month * 12 months * $100 spent / month * 12 months).
This 46% overestimation is why impact estimations frequently do not pass stakeholders’ sniff test.
Accurate forecasting is not just about mathematics — It is a tool that helps you build trust and gets your initiatives approved faster without the risk of over-promising and under-delivering.
Moreover, data professionals spend hours building manual forecasts in Excel, which are volatile, can result in formula errors, and are challenging to iterate upon.
Having a standardized, explainable methodology can help simplify this process.
Triangle Forecasting is a systematic, mathematical approach to estimate the yearly impact when end-people are acquired gradually. It accounts for the fact that incoming end-people will contribute differently to the annual impact, depending on when they onboard on to your product.
New Product Launches: When customer acquisition happens over time.
When customer acquisition happens over time Subscription Revenue Forecasts: For accurate revenue projections for subscription-based products.
For accurate revenue projections for subscription-based products Phased Rollouts: For estimating the cumulative impact of gradual rollouts.
For estimating the cumulative impact of gradual rollouts Acquisition Planning: For setting realistic monthly acquisition targets to hit annual goals.
The “triangle” in Triangle Forecasting refers to the way individual cohort contributions are visualized. A cohort refers to the month in which the clients were acquired. Each bar in the triangle represents a cohort’s contribution to the annual impact. Earlier cohorts have longer bars because they contributed for an extended period.
To calculate the impact of a new initiative, model or feature in the first year :
Calculate number of clients acquired (Am).
Calculate average monthly spend/impact per customer (S).
Calculate remaining months in year (Rm = 13-m).
2. Total yearly impact = Sum of all monthly cohort impacts.
Let’s calculate the actual revenue for our subscription service:
January: 100 clients × $100 × 12 months = $120,000.
February: 100 clients × $100 × 11 months = $110,000.
March: 100 clients × $100 × 10 months = $100,000.
The total annual revenue equals $780,000— 46% lower than the oversimplified estimate!
💡 : Save the spreadsheet calculations as a template to reuse for different scenarios.
Need to build estimates without perfect data? Read my guide on “Building Defendable Impact Estimates When Data is Imperfect”.
Putting Theory into Practice: An Implementation Guide.
While we can implement Triangle Forecasting in Excel using the above method, these spreadsheets become impossible to maintain or modify quickly. Product owners also struggle to improvement forecasts quickly when assumptions or timelines change.
Here’s how we can perform build the same forecast in Python in minutes:
import pandas as pd import numpy as np import [website] as plt def triangle_forecast(monthly_acquisition_rate, monthly_spend_per_customer): """ Calculate yearly impact using triangle forecasting method. """ # Create a DataFrame for calculations months = range(1, 13) df = pd.DataFrame(index=months, columns=['month', 'new_customers', 'months_contributing', 'total_impact']) # Convert to list if single number, else use provided list acquisitions = [monthly_acquisitions] * 12 if type(monthly_acquisitions) in [int, float] else monthly_acquisitions # Calculate impact for each cohort for month in months: [website][month, 'month'] = f'Month {month}' [website][month, 'new_customers'] = acquisitions[month-1] [website][month, 'months_contributing'] = 13 - month [website][month, 'total_impact'] = ( acquisitions[month-1] * monthly_spend_per_customer * (13 - month) ) total_yearly_impact = df['total_impact'].sum() return df, total_yearly_impact.
Continuing with our previous example of subscription service, the revenue from each monthly cohort can be visualized as follows:
# Example monthly_acquisitions = 100 # 100 new clients each month monthly_spend = 100 # $100 per customer per month # Calculate forecast df, total_impact = triangle_forecast(monthly_acquisitions, monthly_spend) # Print results print("Monthly Breakdown:") print(df) print(f"
Total Yearly Impact: ${total_impact:,.2f}").
We can also leverage Python to visualize the cohort contributions as a bar chart. Note how the impact decreases linearly as we move through the months.
Using this Python code, you can now generate and iterate on annual impact estimations quickly and efficiently, without having to manually perform version control on crashing spreadsheets.
While the above example is straightforward, assuming monthly acquisitions and spending are constant across all months, that need not necessarily be true. Triangle forecasting can be easily adapted and scaled to account for :
For varying monthly spend based on spend tiers, create a distinct triangle forecast for each cohort and then aggregate individual cohort’s impacts to calculate the total annual impact.
Typically, businesses don’t acquire consumers at a constant rate throughout the year. Acquisition might start at a slow pace and ramp up as marketing kicks in, or we might have a burst of early adopters followed by slower growth. To handle varying rates, pass a list of monthly targets instead of a single rate:
# Example: Gradual ramp-up in acquisitions varying_acquisitions = [50, 75, 100, 150, 200, 250, 300, 300, 300, 250, 200, 150] df, total_impact = triangle_forecast(varying_acquisitions, monthly_spend).
To account for seasonality, multiply each month’s impact by its corresponding seasonal factor ([website], [website] for high-season months like December, [website] for low-season months like February, etc.) before calculating the total impact.
Here is how you can modify the Python code to account for seasonal variations:
import pandas as pd import numpy as np import [website] as plt def triangle_forecast(monthly_acquisitions, monthly_spend_per_customer, seasonal_factors = None): """ Calculate yearly impact using triangle forecasting method. """ # Create a DataFrame for calculations months = range(1, 13) df = pd.DataFrame(index=months, columns=['month', 'new_customers', 'months_contributing', 'total_impact']) # Convert to list if single number, else use provided list acquisitions = [monthly_acquisitions] * 12 if type(monthly_acquisitions) in [int, float] else monthly_acquisitions if seasonal_factors is None: seasonality = [1] * 12 else: seasonality = [seasonal_factors] * 12 if type(seasonal_factors) in [int, float] else seasonal_factors # Calculate impact for each cohort for month in months: [website][month, 'month'] = f'Month {month}' [website][month, 'new_customers'] = acquisitions[month-1] [website][month, 'months_contributing'] = 13 - month [website][month, 'total_impact'] = ( acquisitions[month-1] * monthly_spend_per_customer * (13 - month)* seasonality[month-1] ) total_yearly_impact = df['total_impact'].sum() return df, total_yearly_impact # Seasonality-adjusted example monthly_acquisitions = 100 # 100 new individuals each month monthly_spend = 100 # $100 per customer per month seasonal_factors = [[website], # January (New Year) [website], # February (Post-holiday) [website], # March [website], # April [website], # May [website], # June (Summer) [website], # July (Summer) [website], # August [website], # September [website], # October (Halloween) [website], # November (Pre-holiday) [website] # December (Holiday) ] # Calculate forecast df, total_impact = triangle_forecast(monthly_acquisitions, monthly_spend, seasonal_factors).
These customizations can help you model different growth scenarios including:
Gradual ramp-ups in early stages of launch.
Step-function growth based on promotional campaigns.
Seasonal variations in customer acquisition.
Having dependable and intuitive forecasts can make or break the case for your initiatives.
But that’s not all — triangle forecasting also finds applications beyond revenue forecasting, including calculating:
Ready to dive in? Download the Python template shared above and build your first Triangle forecast in 15 minutes!
Input your monthly acquisition targets Set your expected monthly customer impact Visualize your annual trajectory with automated visualizations.
Real-world estimations often require dealing with imperfect or incomplete data. Check out my article “Building Defendable Impact Estimates When Data is Imperfect” for a framework to build defendable estimates in such scenarios.
Thank you to my wonderful mentor, Kathryne Maurer, for developing the core concept and first iteration of the Triangle Forecasting method and allowing me to build on it through equations and code.
I’m always open to feedback and suggestions on how to make these guides more valuable for you. Happy reading!
Machine learning (ML) practitioners looking to reuse existing datasets to train an ML model often spend a lot of time understanding the data, making s...
As part of his global tour, OpenAI CEO Sam Altman is in Delhi today for the firm’s DevDay, joined by India’s IT minister Ashwini Vaishnaw and OpenA...
Text-to-image diffusion models have shown exceptional capabilities in generating high-quality images from text prompts. However, leading models featur...
Market Impact Analysis
Market Growth Trend
2018 | 2019 | 2020 | 2021 | 2022 | 2023 | 2024 |
---|---|---|---|---|---|---|
23.1% | 27.8% | 29.2% | 32.4% | 34.2% | 35.2% | 35.6% |
Quarterly Growth Rate
Q1 2024 | Q2 2024 | Q3 2024 | Q4 2024 |
---|---|---|---|
32.5% | 34.8% | 36.2% | 35.6% |
Market Segments and Growth Drivers
Segment | Market Share | Growth Rate |
---|---|---|
Machine Learning | 29% | 38.4% |
Computer Vision | 18% | 35.7% |
Natural Language Processing | 24% | 41.5% |
Robotics | 15% | 22.3% |
Other AI Technologies | 14% | 31.8% |
Technology Maturity Curve
Different technologies within the ecosystem are at varying stages of maturity:
Competitive Landscape Analysis
Company | Market Share |
---|---|
Google AI | 18.3% |
Microsoft AI | 15.7% |
IBM Watson | 11.2% |
Amazon AI | 9.8% |
OpenAI | 8.4% |
Future Outlook and Predictions
The Openai Change Identité landscape is evolving rapidly, driven by technological advancements, changing threat vectors, and shifting business requirements. Based on current trends and expert analyses, we can anticipate several significant developments across different time horizons:
Year-by-Year Technology Evolution
Based on current trajectory and expert analyses, we can project the following development timeline:
Technology Maturity Curve
Different technologies within the ecosystem are at varying stages of maturity, influencing adoption timelines and investment priorities:
Innovation Trigger
- Generative AI for specialized domains
- Blockchain for supply chain verification
Peak of Inflated Expectations
- Digital twins for business processes
- Quantum-resistant cryptography
Trough of Disillusionment
- Consumer AR/VR applications
- General-purpose blockchain
Slope of Enlightenment
- AI-driven analytics
- Edge computing
Plateau of Productivity
- Cloud infrastructure
- Mobile applications
Technology Evolution Timeline
- Improved generative models
- specialized AI applications
- AI-human collaboration systems
- multimodal AI platforms
- General AI capabilities
- AI-driven scientific breakthroughs
Expert Perspectives
Leading experts in the ai tech sector provide diverse perspectives on how the landscape will evolve over the coming years:
"The next frontier is AI systems that can reason across modalities and domains with minimal human guidance."
— AI Researcher
"Organizations that develop effective AI governance frameworks will gain competitive advantage."
— Industry Analyst
"The AI talent gap remains a critical barrier to implementation for most enterprises."
— Chief AI Officer
Areas of Expert Consensus
- Acceleration of Innovation: The pace of technological evolution will continue to increase
- Practical Integration: Focus will shift from proof-of-concept to operational deployment
- Human-Technology Partnership: Most effective implementations will optimize human-machine collaboration
- Regulatory Influence: Regulatory frameworks will increasingly shape technology development
Short-Term Outlook (1-2 Years)
In the immediate future, organizations will focus on implementing and optimizing currently available technologies to address pressing ai tech challenges:
- Improved generative models
- specialized AI applications
- enhanced AI ethics frameworks
These developments will be characterized by incremental improvements to existing frameworks rather than revolutionary changes, with emphasis on practical deployment and measurable outcomes.
Mid-Term Outlook (3-5 Years)
As technologies mature and organizations adapt, more substantial transformations will emerge in how security is approached and implemented:
- AI-human collaboration systems
- multimodal AI platforms
- democratized AI development
This period will see significant changes in security architecture and operational models, with increasing automation and integration between previously siloed security functions. Organizations will shift from reactive to proactive security postures.
Long-Term Outlook (5+ Years)
Looking further ahead, more fundamental shifts will reshape how cybersecurity is conceptualized and implemented across digital ecosystems:
- General AI capabilities
- AI-driven scientific breakthroughs
- new computing paradigms
These long-term developments will likely require significant technical breakthroughs, new regulatory frameworks, and evolution in how organizations approach security as a fundamental business function rather than a technical discipline.
Key Risk Factors and Uncertainties
Several critical factors could significantly impact the trajectory of ai tech evolution:
Organizations should monitor these factors closely and develop contingency strategies to mitigate potential negative impacts on technology implementation timelines.
Alternative Future Scenarios
The evolution of technology can follow different paths depending on various factors including regulatory developments, investment trends, technological breakthroughs, and market adoption. We analyze three potential scenarios:
Optimistic Scenario
Responsible AI driving innovation while minimizing societal disruption
Key Drivers: Supportive regulatory environment, significant research breakthroughs, strong market incentives, and rapid user adoption.
Probability: 25-30%
Base Case Scenario
Incremental adoption with mixed societal impacts and ongoing ethical challenges
Key Drivers: Balanced regulatory approach, steady technological progress, and selective implementation based on clear ROI.
Probability: 50-60%
Conservative Scenario
Technical and ethical barriers creating significant implementation challenges
Key Drivers: Restrictive regulations, technical limitations, implementation challenges, and risk-averse organizational cultures.
Probability: 15-20%
Scenario Comparison Matrix
Factor | Optimistic | Base Case | Conservative |
---|---|---|---|
Implementation Timeline | Accelerated | Steady | Delayed |
Market Adoption | Widespread | Selective | Limited |
Technology Evolution | Rapid | Progressive | Incremental |
Regulatory Environment | Supportive | Balanced | Restrictive |
Business Impact | Transformative | Significant | Modest |
Transformational Impact
Redefinition of knowledge work, automation of creative processes. This evolution will necessitate significant changes in organizational structures, talent development, and strategic planning processes.
The convergence of multiple technological trends—including artificial intelligence, quantum computing, and ubiquitous connectivity—will create both unprecedented security challenges and innovative defensive capabilities.
Implementation Challenges
Ethical concerns, computing resource limitations, talent shortages. Organizations will need to develop comprehensive change management strategies to successfully navigate these transitions.
Regulatory uncertainty, particularly around emerging technologies like AI in security applications, will require flexible security architectures that can adapt to evolving compliance requirements.
Key Innovations to Watch
Multimodal learning, resource-efficient AI, transparent decision systems. Organizations should monitor these developments closely to maintain competitive advantages and effective security postures.
Strategic investments in research partnerships, technology pilots, and talent development will position forward-thinking organizations to leverage these innovations early in their development cycle.
Technical Glossary
Key technical terms and definitions to help understand the technologies discussed in this article.
Understanding the following technical concepts is essential for grasping the full implications of the security threats and defensive measures discussed in this article. These definitions provide context for both technical and non-technical readers.