While We Grapple With Geometry, Google DeepMind’s AI Model Beats Math Olympiad Gold Medalists - Related to we, geometry,, adopt, grapple, but
97% of CEOs Plan to Adopt AI, But Only 2% Ready: Cisco Study
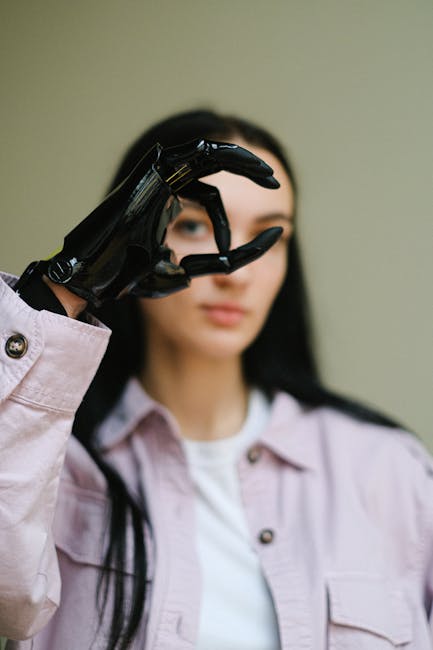
Cisco, one of the world’s leading networking and security companies, released a study on Monday detailing the fears, ambitions, and actions of CEOs regarding AI.
The investigation revealed that “97% of CEOs plan to adapt and incorporate AI into their business, but only 2% feel ready for AI”.
The findings surveyed more than 2,500 CEOs over 25 years of age, representing over 250 companies in Europe, North America, South America, Africa, and Asia-Pacific (APAC).
While most CEOs understand that AI integration is necessary, many feel that their gaps in understanding the technology are hindering growth opportunities.
The study revealed that over 70% of CEOs believe that their understanding of AI hinders their ability to ask “critical questions” in the boardroom. Moreover, 58% of them “believe their understanding of AI will have a negative impact on the growth of their business over the next five years”.
These CEOs also highlighted multiple other factors that hold them back from integrating AI into their businesses, including concerns about infrastructure and budget limitations, security concerns, skill gaps, and so on. About 28% of the respondents feel the potential benefits of AI have been exaggerated.
While 10% of respondents revealed having no concerns about AI, only 2% stated they “feel everything is in place” and are ready to integrate it.
Underscoring the urgency to act, Cisco’s executive vice president and chief product officer, Jeetu Patel, stated in a statement, “Eventually, there will be only two kinds of companies: those that are AI companies and those that are irrelevant.”.
Similarly, a majority of CEOs in the findings revealed their concerns about losing their competitive edge due to infrastructure gaps and lack of investment in new technologies.
Last year, a analysis from Menlo Ventures revealed that AI spending in enterprises saw a sixfold increase from 2023, from $[website] billion to $[website] billion. The analysis also revealed that organisations had outlined 10 potential AI use cases, and 24% of them are prioritised for implementation in the near term. Menlo surveyed 600 US enterprise IT decision-makers for the analysis.
Organisations use generative AI in their stack to generate code, support chatbots, perform enterprise searches and more. The investigation also revealed that 47% of solutions in organisations are built in-house, while 53% are sourced from vendors.
That expressed, it was also revealed that organisations are not just giving into the hype but also focusing on return on investments.
“Buyers are playing the long game. They are far more focused on tools that can deliver measurable value (30%) and that understand the unique context of their work (26%) over those offering the lowest price tag (1%),” the findings read.
Google’s AI lab, DeepMind, has unveiled a new AI model, AlphaGeometry2, which they claim outperforms some of the top minds who have won a gold medal i......
I've turned to locally installed AI for research because I don't want third parties using my information to either build a profile or train the......
La France et les Émirats arabes unis unissent leurs forces pour créer un centre de données IA d’une capacité de 1 gigawatt. Ce projet est estimé entre......
Is Mistral’s Le Chat Truly the ‘World’s Fastest AI Assistant’?
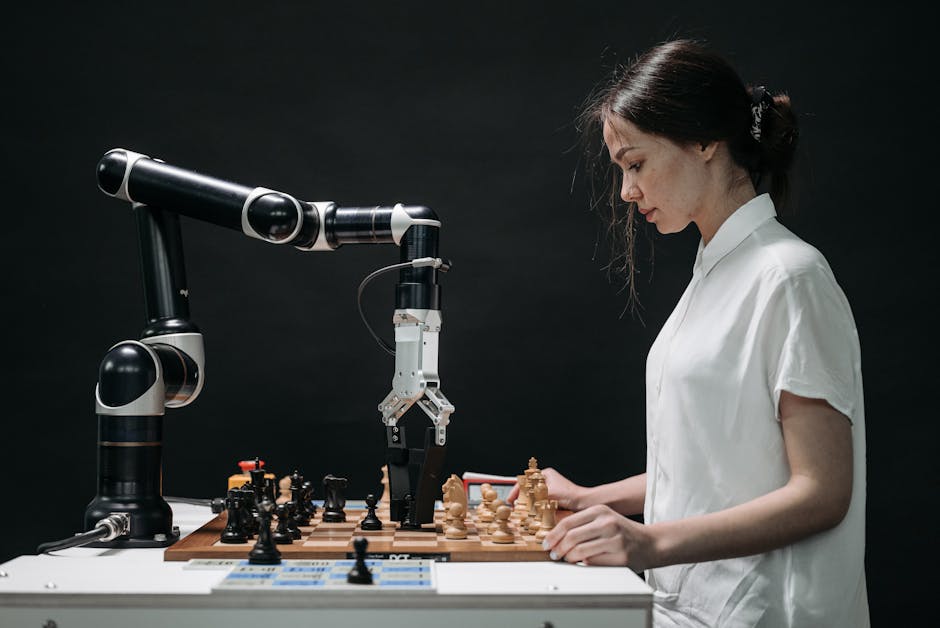
French AI startup Mistral unveiled the Le Chat app for iOS and Android a few days ago. The app functions as an AI chatbot or assistant, rivalling ChatGPT, Claude, and Gemini, among others. The app offers most of its attributes for free, with upgraded limits in the Pro tier, which costs $[website] monthly.
Le Chat offers web search, image and document understanding capabilities alongside code interpretation and image generation.
Given the sheer number of AI assistant applications in the market, a new entrant must offer a formidable differentiation. Mistral proposes its low-latency models are powered by the ‘fastest inference engines on the planet’. Furthermore, Mistral also says that it responds faster than any other chat assistant, up to 1,100 words per second, via their Flash Answers feature.
Cerebras Inference, a service that delivers high-speed processing to AI applications, is the secret sauce to its speed.
, Cerebras Inference is the ‘world’s fastest AI inference provider’ and makes Le Chat 10 times faster than GPT-4o, Claude Sonnet [website], and DeepSeek R1. Cerbras also revealed that the 123 billion-parameter Mistral Large model is behind Le Chat.
Mistral and Cerebras compared Le Chat with Claude [website] Sonnet and ChatGPT-4o using a prompt to generate a snake game using Python.
The results from Mistral’s YouTube video revealed that ChatGPT outputs 85 tokens per second, Claude 120 tokens per second, and Le Chat outperformed the two with 1,100 tokens per second.
In a video by Cerebras, it was revealed that Le Chat took [website] seconds to complete the task, Claude took [website] Sonnet took 19 seconds, and GPT-4o took 46 seconds.
“This performance is made possible by the Wafer Scale Engine 3’s SRAM-based inference architecture in combination with speculative decoding techniques developed in collaboration with researchers at Mistral,” stated Cerebras in a blogpost.
Several consumers also resonate with these asserts. A user named Marc on X expressed that the model is “mind-blowingly fast” and added that it built a simple React application in less than 5 seconds.
Ok Le Chat by @MistralAI is 10x faster than ChatGPT. Maybe 100x. — Pol Maire (@polmaire) February 6, 2025.
Here’s What We Found in Our Real-World Tests.
Besides, we at AIM also conducted a real-time test of some of the leading models, albeit with a different prompt, which expects AI models to solve a Chemistry numerical problem from an IIT-JEE question paper – which is often considered one of the world’s most difficult examinations.
We considered OpenAI’s GPT-4o, o3 Mini, o3 Mini High, Anthropic’s Claude [website] Sonnet, DeepSeek R1, Google’s Gemini [website] Flash, and of course, Mistral’s Le Chat.
The following question was used as input: “Ice at –10°C is to be converted into steam at 110°C. The mass of ice is 10-3 kg. What amount of heat is required?”.
While we timed the results, Mistral’s Le Chat was the fastest model, but it comes with a caveat.
Le Chat returned the output in less than 4 seconds in three out of six times we tested the model. On the other hand, Google’s Gemini [website] Flash returned the output under 6 seconds all the times we tested it.
It begs the question of whether Flash Answers came into action each time despite being enabled by default.
Note that we were using the free version of the Le Chat assistant, and the pro version provides an upgraded limit to the Flash Answers feature.
Moreover, the speeds at which these models perform also depend upon the nature of the queries. Reasoning models, with their lengthy chain of thoughts, prioritise the accuracy of the answer and are bound to take more time.
For instance, when we tested the prompt with DeepSeek R1, it took over a minute to complete the problem, with a chain of thoughts that involved verification steps, where the model mentioned, “But let me check if all the values are correct. Did I use the right specific heat for steam?” and so on.
Furthermore, it also took a great deal of time to ensure the answer was provided with the right number of decimal figures.
A test from Artificial Analysis revealed that OpenAI’s o3-mini was the fastest model among the competition, which outputs 214 tokens per second, ahead of the o1-mini, at 167 tokens per second.
, o3-mini also achieved a high score of 89 on its Quality Index, which is on par with o1 (90 points) and DeepSeek R1 (89 points). This quality index quantifies the overall capabilities of the AI model.
OpenAI has prioritised inference time scaling to deliver outputs at higher speeds. With Cerbreas’ inference capabilities, Mistral seems to have joined the race. Moreover, there is an ongoing battle of token speeds between inference providers like Cerebras, Groq, and SambaNova.
These ambitions to deliver high-speed responses align with what Jensen Huang, CEO of NVIDIA, presented last year. He envisioned a future where AI systems perform various tasks, such as tree search, chain of thought, and mental simulations, reflecting on their own answers and responding in real-time—within a single second.
Le modèle d’intelligence artificielle DeepSeek fait trembler l’industrie technologique et les marchés financiers. Avec un coût de développement bien i......
WNS, the business transformation and services firm, reported its fiscal third-quarter earnings for 2025 in late January, showcasing revenue growth ......
While We Grapple With Geometry, Google DeepMind’s AI Model Beats Math Olympiad Gold Medalists
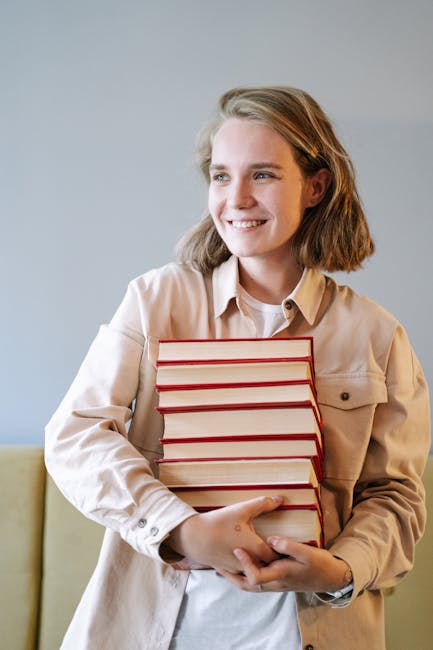
Google’s AI lab, DeepMind, has unveiled a new AI model, AlphaGeometry2, which they claim outperforms some of the top minds who have won a gold medal in the International Mathematical Olympiad.
Last year, it hit the silver medal mark, and this year, we have a gold.
The research paper asserts to have an overall solving rate of 84% for all geometry problems over the last 25 years.
DeepMind initially % solve rate. Looking back, it sounds like good progress with a year of new development. With AlphaGeometry2, the model now tackles locus-type theorems, linear equations, and non-constructive problem statements.
The AI model is built as a neuro-symbolic system that combines a language model with a symbolic engine to tackle challenging geometry problems. Under the hood, it leverages the Gemini architecture with an increased model size and a diverse dataset.
DeepMind’s model was trained on algorithmically generated synthetic data. The method starts by sampling a random diagram and using the symbolic engine to deduce all possible facts from it. It avoids using human-crafted problems and instead starts from random diagrams.
As per the research paper, AlphaGeometry2 translates geometry problems in natural language. The paper mentions, “To do this, we utilise Gemini Team Gemini (2024) to translate problems from natural language into the AlphaGeometry language and implement a new automated diagram generation algorithm.”.
The paper mentions the approach of setting the stage for test results, “There are a total of 45 geometry problems in the 2000-2024 International Math Olympiad (IMO), which we translate into 50 AlphaGeometry problems (we call this set IMO-AG-50). Some problems are split into two due to specifics of our formalisation.”.
In addition, it also sheds light on how effective it was, where the model solved 42 out of 50 of all 2000-2024 International Math Olympiad (IMO) geometry problems, thus surpassing an average gold medallist for the first time.
The model was also pitched against other models like OpenAI’s o1, but as you can see in the table above, AlphaGeometry2 solved most of the questions.
To arrive at a conclusion, the paper highlights, “Our geometry experts and International Math Olympiad (IMO) medallists consider many AlphaGeometry solutions to exhibit superhuman creativity.” They also add, “Despite good initial results, we think the auto-formalisation can be further improved with more formalisation examples and supervised fine-tuning.”.
With models like AlphaGeometry2, AI is also getting into the high school math competition, which is an intriguing development.
French artificial intelligence startup Mistral has presented plans to invest “several billion euros” in building its first data centre in France. The ......
Accenture showcased on February 7 that it would acquire German management consulting firm Staufen AG to enhance its AI-driven solutions for manufactur......
Market Impact Analysis
Market Growth Trend
2018 | 2019 | 2020 | 2021 | 2022 | 2023 | 2024 |
---|---|---|---|---|---|---|
23.1% | 27.8% | 29.2% | 32.4% | 34.2% | 35.2% | 35.6% |
Quarterly Growth Rate
Q1 2024 | Q2 2024 | Q3 2024 | Q4 2024 |
---|---|---|---|
32.5% | 34.8% | 36.2% | 35.6% |
Market Segments and Growth Drivers
Segment | Market Share | Growth Rate |
---|---|---|
Machine Learning | 29% | 38.4% |
Computer Vision | 18% | 35.7% |
Natural Language Processing | 24% | 41.5% |
Robotics | 15% | 22.3% |
Other AI Technologies | 14% | 31.8% |
Technology Maturity Curve
Different technologies within the ecosystem are at varying stages of maturity:
Competitive Landscape Analysis
Company | Market Share |
---|---|
Google AI | 18.3% |
Microsoft AI | 15.7% |
IBM Watson | 11.2% |
Amazon AI | 9.8% |
OpenAI | 8.4% |
Future Outlook and Predictions
The Ceos Plan Adopt landscape is evolving rapidly, driven by technological advancements, changing threat vectors, and shifting business requirements. Based on current trends and expert analyses, we can anticipate several significant developments across different time horizons:
Year-by-Year Technology Evolution
Based on current trajectory and expert analyses, we can project the following development timeline:
Technology Maturity Curve
Different technologies within the ecosystem are at varying stages of maturity, influencing adoption timelines and investment priorities:
Innovation Trigger
- Generative AI for specialized domains
- Blockchain for supply chain verification
Peak of Inflated Expectations
- Digital twins for business processes
- Quantum-resistant cryptography
Trough of Disillusionment
- Consumer AR/VR applications
- General-purpose blockchain
Slope of Enlightenment
- AI-driven analytics
- Edge computing
Plateau of Productivity
- Cloud infrastructure
- Mobile applications
Technology Evolution Timeline
- Improved generative models
- specialized AI applications
- AI-human collaboration systems
- multimodal AI platforms
- General AI capabilities
- AI-driven scientific breakthroughs
Expert Perspectives
Leading experts in the ai tech sector provide diverse perspectives on how the landscape will evolve over the coming years:
"The next frontier is AI systems that can reason across modalities and domains with minimal human guidance."
— AI Researcher
"Organizations that develop effective AI governance frameworks will gain competitive advantage."
— Industry Analyst
"The AI talent gap remains a critical barrier to implementation for most enterprises."
— Chief AI Officer
Areas of Expert Consensus
- Acceleration of Innovation: The pace of technological evolution will continue to increase
- Practical Integration: Focus will shift from proof-of-concept to operational deployment
- Human-Technology Partnership: Most effective implementations will optimize human-machine collaboration
- Regulatory Influence: Regulatory frameworks will increasingly shape technology development
Short-Term Outlook (1-2 Years)
In the immediate future, organizations will focus on implementing and optimizing currently available technologies to address pressing ai tech challenges:
- Improved generative models
- specialized AI applications
- enhanced AI ethics frameworks
These developments will be characterized by incremental improvements to existing frameworks rather than revolutionary changes, with emphasis on practical deployment and measurable outcomes.
Mid-Term Outlook (3-5 Years)
As technologies mature and organizations adapt, more substantial transformations will emerge in how security is approached and implemented:
- AI-human collaboration systems
- multimodal AI platforms
- democratized AI development
This period will see significant changes in security architecture and operational models, with increasing automation and integration between previously siloed security functions. Organizations will shift from reactive to proactive security postures.
Long-Term Outlook (5+ Years)
Looking further ahead, more fundamental shifts will reshape how cybersecurity is conceptualized and implemented across digital ecosystems:
- General AI capabilities
- AI-driven scientific breakthroughs
- new computing paradigms
These long-term developments will likely require significant technical breakthroughs, new regulatory frameworks, and evolution in how organizations approach security as a fundamental business function rather than a technical discipline.
Key Risk Factors and Uncertainties
Several critical factors could significantly impact the trajectory of ai tech evolution:
Organizations should monitor these factors closely and develop contingency strategies to mitigate potential negative impacts on technology implementation timelines.
Alternative Future Scenarios
The evolution of technology can follow different paths depending on various factors including regulatory developments, investment trends, technological breakthroughs, and market adoption. We analyze three potential scenarios:
Optimistic Scenario
Responsible AI driving innovation while minimizing societal disruption
Key Drivers: Supportive regulatory environment, significant research breakthroughs, strong market incentives, and rapid user adoption.
Probability: 25-30%
Base Case Scenario
Incremental adoption with mixed societal impacts and ongoing ethical challenges
Key Drivers: Balanced regulatory approach, steady technological progress, and selective implementation based on clear ROI.
Probability: 50-60%
Conservative Scenario
Technical and ethical barriers creating significant implementation challenges
Key Drivers: Restrictive regulations, technical limitations, implementation challenges, and risk-averse organizational cultures.
Probability: 15-20%
Scenario Comparison Matrix
Factor | Optimistic | Base Case | Conservative |
---|---|---|---|
Implementation Timeline | Accelerated | Steady | Delayed |
Market Adoption | Widespread | Selective | Limited |
Technology Evolution | Rapid | Progressive | Incremental |
Regulatory Environment | Supportive | Balanced | Restrictive |
Business Impact | Transformative | Significant | Modest |
Transformational Impact
Redefinition of knowledge work, automation of creative processes. This evolution will necessitate significant changes in organizational structures, talent development, and strategic planning processes.
The convergence of multiple technological trends—including artificial intelligence, quantum computing, and ubiquitous connectivity—will create both unprecedented security challenges and innovative defensive capabilities.
Implementation Challenges
Ethical concerns, computing resource limitations, talent shortages. Organizations will need to develop comprehensive change management strategies to successfully navigate these transitions.
Regulatory uncertainty, particularly around emerging technologies like AI in security applications, will require flexible security architectures that can adapt to evolving compliance requirements.
Key Innovations to Watch
Multimodal learning, resource-efficient AI, transparent decision systems. Organizations should monitor these developments closely to maintain competitive advantages and effective security postures.
Strategic investments in research partnerships, technology pilots, and talent development will position forward-thinking organizations to leverage these innovations early in their development cycle.
Technical Glossary
Key technical terms and definitions to help understand the technologies discussed in this article.
Understanding the following technical concepts is essential for grasping the full implications of the security threats and defensive measures discussed in this article. These definitions provide context for both technical and non-technical readers.