Cerebras CEO on DeepSeek: Every time computing gets cheaper, the market gets bigger - Related to cerebras, 10, market, -, taxes
5 ways AI can help with your taxes - and 10 major mistakes to avoid
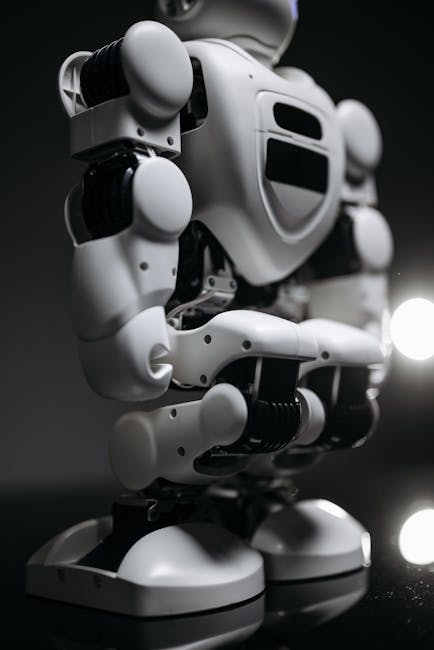
In a recent test of ChatGPT's Deep Research feature, the AI was asked to identify 20 jobs that OpenAI's new o3 model was likely to replace. As ZDNET's Sabrina Ortiz reported, "Right in time with tax return season, leading the table was the role of 'tax preparer' with a probability of 98% replacement, which ChatGPT deemed as 'near-certain automation.'"
There is no doubt that retail tax preparation services are using some level of AI to reduce their workload, but while tax preparers may well be replaced by a machine, I'm not convinced that will lead to accurate or reliable tax returns -- certainly not yet.
Also: From zero to millions? How regular people are cashing in on AI.
For example, there may come a time when you'll be able to tell an AI to find all your receipts and transactions, divide them into categories, identify which categories your deductions are in, and then enter them into the appropriate tax forms and bookkeeping systems. But that series of linked activities does not appear to be available now.
For those who already keep their records in a clear and organized manner, AI might help. But in my first year as a business owner, I kept all -- all -- my paperwork in a big duffle bag. When tax time came, I just dumped the duffle bag on the desk of my local tax preparer. Turning that disaster into a good tax return was a massive by-hand effort.
My business made very little that first year, but the fee I had to pay that preparer to excavate my documents and prepare my taxes was breathtaking. After learning my lesson, I got more rigorous about organizing my records. My point is that young entrepreneur me is not the only person with sloppy documentation. Some jobs will always require a human for at least some of the work.
Also: The best AI for coding in 2025 (and what not to use).
Even with good organization and rigorous bookkeeping (which I've done religiously for a few decades now), the various tools that we need to work together are usually from different vendors. The AIs are just not up to that level of organization across a wide range of documents and activities.
That mentioned, there are areas where an AI can help. Here are five of them.
1. Use AI functions available in tax prep software.
Tax programs like TurboTax and H&R Block tax software now offer varying degrees of AI assistance for your tax preparation. Don't expect your tax preparation software to do all the work for you, but it can help save time and provide some quick assistance.
Intuit has several AI-related offerings that are part of its TurboTax product. TurboTax can import data from 350 financial institutions and can auto-fill tax form fields using a tool called IntuitAssist. The AI is also used to check forms for accuracy and find errors, recommend deductions, and answer tax questions.
Also: This is the best money management app I've tested.
Intuit is also pitching a TurboTax Live Assisted program where an AI will match you with a tax expert who will work with you in a Zoom-like call to fill out your taxes. This is sort of a mix of artificial and real intelligence.
H&R Block Tax Assist is a new generative AI tool that can provide tax information, help with tax preparation, and answer free-form tax-related questions to help you understand the tax issues you're dealing with as you complete your returns.
H&R Block also says its Tax Assistant can answer questions about recent changes to tax code, but be careful because AI knowledge tends to lag a bit behind real-time regulation changes.
Now, all of this might sound good, but keep in mind that generative AI has the tendency to make mistakes, make stuff up, and mislead. That's not exactly what you want when preparing taxes. Geoffrey A. Fowler of the Washington Post provides a cautionary tale. He tried both TurboTax and H&R Block's AI features and found them to be "awful."
Also: Stop paying full price for PCs and Macs: 7 ways to save money.
To be fair, I've paid real-world human accountants for tax prep help, and have found some of them to be awful, too. Taxes aren't fun, and you have to double-check everything, whether it's your own work, the work of an AI, or the work of someone who implies to be an expert.
2. Use AI capabilities in expense tracking software.
Not all expense tracking services offer AI elements, but Fyle, SparkReceipt, and QuickBooks do.
I am a somewhat involuntary QuickBooks user. The price has gone up considerably over the years, but the switching costs are even higher. So I stick with QuickBooks. For imported expenses that don't have custom rules, QuickBooks attempts to assign categories using some AI capabilities. Don't count on this feature. Those assignments are almost always incorrect.
QuickBooks also constantly pushes its related services, some of which have AI capabilities. But I haven't found anything on offer that seems worth the upsell, so I haven't tapped into those additional AI capabilities.
Also: The US Copyright Office's new ruling on AI art is here - and it could change everything.
Fyle's big claim to fame is what it calls Conversational AI for Expense Tracking. Basically, all you do is snap a picture of your receipts with your phone and text it to Fyle. Fyle processes it in and categorizes it all automatically, saving a lot of time.
SparkReceipt also automates receipt scanning and categorization, along with invoices and bank statements. It will then enter your information, without the need for manual entry. The key feature here is the categorization of expenses, which can often take both time and effort to do by hand.
Microsoft's Copilot has powerful integration with Excel. No matter what data you're organizing for your tax filing or accounting process, some of it is likely to be run through Excel. Copilot will automate many of the Excel setup tasks that used to take a lot of time and sometimes hard-to-find Excel knowledge.
Also: The Microsoft 365 Copilot launch was a total disaster.
Rather than go into more details here, I recommend you watch this video from Singapore, where the instructor provides a detailed look at how Excel works with taxes. While tax policy in each country is different, the tasks the instructor performs are very similar throughout the world.
4. Chat with a chatbot for tax advice and guidance.
You can also use a chatbot like ChatGPT or Perplexity to get tax guidance and advice. Just keep in mind you want to ask questions about topics that have been written about in prior years, are stable with unchanging tax rules, and for which there is .
Also: How to make ChatGPT provide enhanced reports and citations.
Here are some examples I tried. They all resulted in good and accurate answers (at least as best as this non-accountant could tell).
Who needs to file a US federal tax return?
What are the IRS standard deduction amounts?
What are the tax brackets for past years?
What is the difference between a tax deduction and a tax credit?
What tax credits are available for education expenses?
How can I check the status of my federal tax refund?
Make sure you preface any questions you ask with your filing jurisdiction. If you're in the US, say so. If you're in Singapore, tell the AI that. Otherwise, the AI will probably not know which jurisdiction's tax rules are appropriate for you.
5. Upload and scan documents for analysis, summarization, and explanation.
You can feed your favorite chatbot PDFs for it to analyze and explain. For example, I uploaded a copy of the instructions for IRS Form 2553, which is the form used to elect S corporation status.
Also: US sets AI safety aside in favor of 'AI dominance'
I asked ChatGPT, "Explain this." I then asked it, "What are the most crucial things I should know?" It scanned the document and provided me with a list of crucial informational nuggets.
I asked ChatGPT to provide me with a list of 10 areas where you should not use AI to help with taxes. It's a good list, and I fully agree with all of its points.
Providing legally binding tax advice: AI does not replace professional tax advisors, CPAs, or attorneys. Ensuring complete tax compliance: AI may not account for the latest IRS rule changes, state-specific laws, or unique tax situations. Filing your tax return on your behalf: AI cannot submit tax forms directly to the IRS or state tax agencies. Determining eligibility for complex tax deductions and credits: Some deductions and credits (like the Qualified Business Income Deduction) require professional assessment. Guaranteeing IRS audit protection: AI cannot ensure you won't be audited or provide direct representation if you are audited. Handling late tax election relief requests: The IRS may require a written explanation of "reasonable cause," which is best handled by a tax professional. Interpreting ambiguous tax laws and regulations: AI cannot provide definitive answers on gray areas of tax law or IRS rulings. Preparing multi-state or international tax returns: AI may not accurately handle tax liabilities across multiple jurisdictions. Detecting tax fraud or avoiding penalties: AI cannot verify whether deductions, credits, or income reporting comply fully with IRS standards. Giving investment or retirement tax strategy recommendations: AI cannot advise on tax-efficient investment decisions, Roth IRA conversions, or estate planning strategies.
What do you think? Have you tried AI-powered tax tools like TurboTax Assist, H&R Block Tax Assist, or QuickBooks? Did they help or make things more complicated? Do you trust AI to handle tax prep, or do you still prefer human expertise? Where do you think AI tax tools need the most improvement? Let us know in the comments below.
Yotta Data Services, a data centre and cloud computing firm backed by the Hiranandani Group, has submitted its final application to the US Securities ......
Okta, the US based identity and access management firm, has named Shakeel Khan as its regional vice president and country manager for India, reinfo......
WNS, the business transformation and services firm, reported its fiscal third-quarter earnings for 2025 in late January, showcasing revenue growth ......
Inside Monday’s AI pivot: Building digital workforces through modular AI
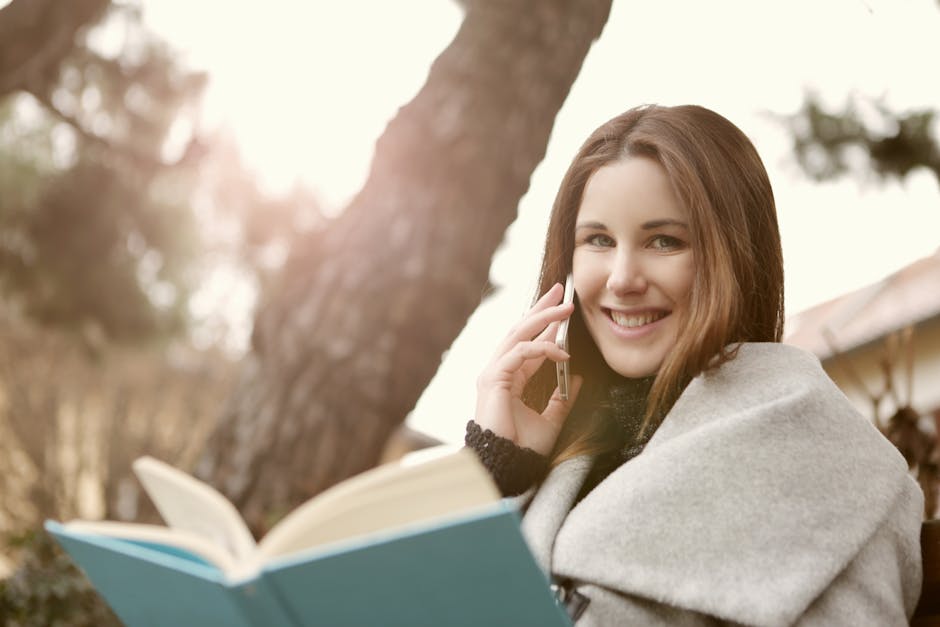
The [website] work platform has been steadily growing over the past decade, in a quest to achieve its goal of helping empower teams at organizations small and large to be more efficient and productive.
With the advent and popularity of generative AI in the last three years, particularly since the debut of ChatGPT, Monday — much like every other enterprise on the planet — began to consider and integrate the technology.
The initial deployment of gen AI at Monday didn’t quite generate the return on investment individuals wanted, however. That realization led to a bit of a rethink and pivot as the firm looked to give its individuals AI-powered tools that actually help to improve enterprise workflows. That pivot has now manifested itself with the firm’s “AI blocks” technology and the preview of its agentic AI technology that it calls “digital workforce.”.
Monday’s AI journey, for the most part, is all about realizing the business’s founding vision.
“We wanted to do two things, one is give people the power we had as developers,” Mann told VentureBeat in an . “So they can build whatever they want, and they feel the power that we feel, and the other end is to build something they really love.”.
Any type of vendor, particularly an enterprise software vendor, is always trying to improve and help its individuals. Monday’s AI adoption fits securely into that pattern.
The organization’s public AI strategy has evolved through several distinct phases:
AI assistant: Initial platform-wide integration; AI blocks: Modular AI capabilities for workflow customization; Digital workforce: Agentic AI.
Much like many other vendors, the first public foray into gen AI involved an assistant technology. The basic idea with any AI assistant is that it provides a natural language interface for queries. Mann explained that the Monday AI assistant was initially part of the corporation’s formula builder, giving non-technical people the confidence and ability to build things they couldn’t before. While the service is useful, there is still much more that organizations need and want to do.
Or Fridman, AI product group lead at Monday, explained that the main lesson learned from deploying the AI assistant is that consumers want AI to be integrated into their workflows. That’s what led the corporation to develop AI blocks.
Building the foundation for enterprise workflows with AI blocks.
Monday realized the limitations of the AI assistant approach and what customers really wanted.
Simply put, AI functionality needs to be in the right context for clients — directly in a column, component or service automation.
AI blocks are pre-built AI functions that Monday has made accessible and integrated directly into its workflow and automation tools. For example, in project management, the AI can provide risk mapping and predictability analysis, helping clients superior manage their projects. This allows them to focus on higher-level tasks and decision-making, while the AI handles the more repetitive or data-intensive work.
This approach has particular significance for the platform’s user base, 70% of which consists of non-technical companies. The modular nature allows businesses to implement AI capabilities without requiring deep technical expertise or major workflow disruptions.
Monday is taking a model agnostic approach to integrating AI.
An early approach taken by many vendors on their AI journeys was to use a single vendor large language model (LLM). From there, they could build a wrapper around it or fine tune for a specific use case.
Mann explained that Monday is taking a very agnostic approach. In his view, models are increasingly becoming a commodity. The enterprise builds products and solutions on top of available models, rather than creating its own proprietary models.
Looking a bit deeper, Assaf Elovic, Monday’s AI director, noted that the enterprise uses a variety of AI models. That includes OpenAI models such as GPT-4o via Azure, and others through Amazon Bedrock, ensuring flexibility and strong performance. Elovic noted that the enterprise’s usage follows the same data residency standards as all Monday elements. That includes multi-region support and encryption, to ensure the privacy and security of customer data.
Agentic AI and the path to the digital workforce.
The latest step in Monday’s AI journey is in the same direction as the rest of the industry — the adoption of agentic AI.
The promise of agentic AI is more autonomous operations that can enable an entire workflow. Some organizations build agentic AI on top of frameworks such as LangChain or Crew AI. But that’s not the specific direction that Monday is taking with its digital workforce platform.
Elovic explained that Monday’s agentic flow is deeply connected to its own AI blocks infrastructure. The same tools that power its agents are built on AI blocks like sentiment analysis, information extraction and summarization.
Mann noted that digital workforce isn’t so much about using a specific agentic AI tool or framework, but about creating superior automation and flow across the integrated components on the Monday platform. Digital workforce agents are tightly integrated into the platform and workflows. This allows the agents to have contextual awareness of the user’s data, processes and existing setups within Monday.
The first digital workforce agent is set to become available in March. Mann noted it will be called the monday “expert” designed to build solutions for specific customers. customers describe their problems and needs to the agent, and the AI will provide them relevant workflows, boards and automations to address those challenges.
AI specialization and integration provides differentiation in a commoditized market.
There is no shortage of competition across the markets that Monday serves.
As a workflow platform, it crosses multiple industry verticals including customer relationship management (CRM) and project management. There are big players across these industries including Salesforce and Atlassian, which have both deeply invested in AI.
Mann expressed the deep integration with AI blocks across various Monday tools differentiate the firm from its rivals. At a more basic level, he expressed, it’s really all about meeting people where they are and embedding useful AI capabilities in the context of workflow.
Monday’s evolution hints at a model for enterprise software development where AI capabilities are deeply integrated yet highly customizable. This approach addresses a crucial challenge in enterprise AI adoption: The need for solutions that are both powerful and accessible to non-technical consumers.
The enterprise’s strategy also points to a future where AI implementation focuses on empowerment rather than replacement.
“If a technology makes large companies more efficient, what does it do for SMBs?” mentioned Mann, highlighting how AI democratization could level the playing field between large and small enterprises.
India’s first Drone Centres of Excellence (CoEs) have been launched in Odisha’s Kalahandi district under the Sansad Adarsh Gram Yojana (SAGY).
Presque toutes les applications de rencontres populaires, telles que Tinder, Badoo ou Bumble, sont conçues pour le public le plus large possible. Elle......
À l’occasion de la Saint-Valentin, Tinder teste de nouvelles fonctionnalités IA basées sur la mise en relation, alors que les utilisateurs continuent ......
Cerebras CEO on DeepSeek: Every time computing gets cheaper, the market gets bigger
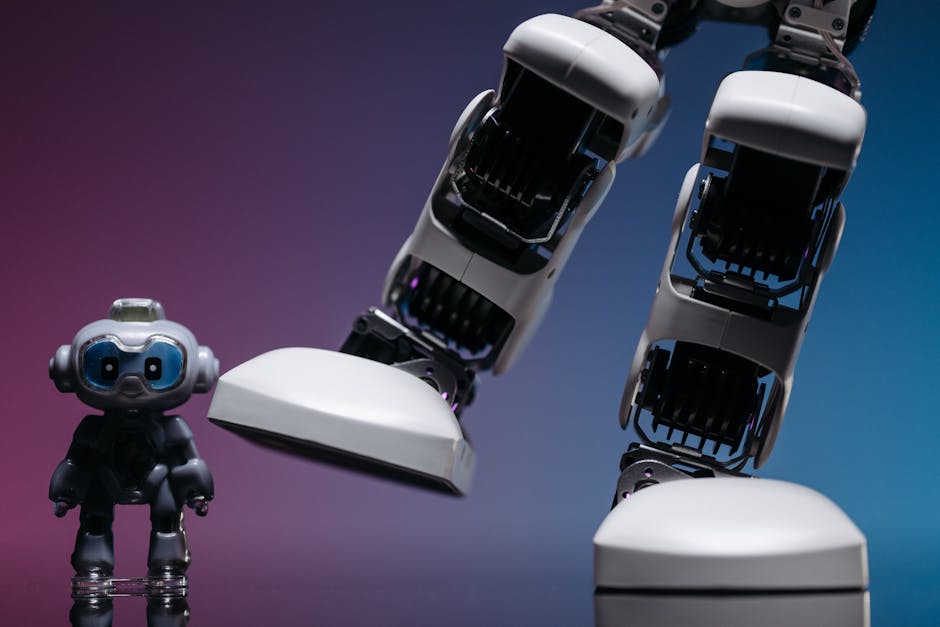
AI computer pioneer Cerebras Systems has been "crushed" with demand to run DeepSeek's R1 large language model, says enterprise co-founder and CEO Andrew Feldman.
"We are thinking about how to meet the demand; it's big," Feldman told me in an interview via Zoom last week.
DeepSeek R1 is heralded by some as a watershed moment for artificial intelligence because the cost of pre-training the model can be as little as one-tenth that of dominant models such as OpenAI's GPTo1 while having results as good or enhanced.
The impact of DeepSeek on the economics of AI is significant, Feldman indicated. But the more profound result is that it will spur even larger AI systems.
Also: Perplexity lets you try DeepSeek R1 without the security risk.
"As we bring down the cost of compute, the market gets bigger and bigger and bigger," expressed Feldman.
Numerous AI cloud services rushed to offer DeepSeek inference after the AI model became a sensation, including Cerebras but also much larger firms such as Amazon's AWS. (You can try Cerebras's inference service here.).
Cerebras's edge is speed. , running inference on the enterprise's CS-3 computers achieves output 57 times faster than other DeepSeek service providers.
Cerebras also highlights its speed relative to other large language models. In a demo of a reasoning problem done by DeepSeek running on Cerebras versus OpenAI's o1 mini, the Cerebras machine finishes in a second and a half, while o1 takes a full 22 seconds to complete the task.
"This speed can't be achieved with any number of GPUs," expressed Feldman, referring to the chips sold for AI by Nvidia, Advanced Micro Devices, and Intel.
The challenge for anyone hosting DeepSeek is that DeepSeek, like other so-called reasoning models, such as OpenAI's GPTo1, uses much more computing power when it produces output at inference time, making it harder to deliver results at the user prompt in a timely fashion.
"A basic GPT model does one inference pass through all the parameters for every word" of input at the prompt, Feldman explained.
"These reasoning models, or, chain-of-thought models, do that many times" for each word, "and so they use a great deal more compute at inference time."
Cerebras followed one standard procedure for companies wanting to run DeepSeek inference: download the R1 neural parameters -- or weights -- on Hugging Face, then use the parameters to train a smaller open-source model, in this case, Meta Platforms's Llama 70B, to create a "distillation" of R1.
"We were able to do that extremely quickly, and we were able to produce results that are just plain faster than everybody else -- not by a little bit, by a lot," showcased Feldman.
Also: I tested DeepSeek's R1 and V3 coding skills - and we're not all doomed (yet).
Cerebras's results with the DeepSeek R1 distilled Llama 70B are comparable to . Cerebras is not disclosing DeepSeek R1 distilled Llama 70B pricing for inference, but mentioned that it is "Competitively priced, especially for delivering top industry performance."
DeepSeek's breakthrough has several implications.
One, it's a big victory for open-source AI, Feldman indicated, by which he means AI models that post their neural parameters for download. Many of a new AI model's advances can be replicated by researchers when they have access to the weights, even without having access to the source code. Private models such as GPT-4 do not disclose their weights.
"Open source is having its minute for sure," mentioned Feldman. "This was the first top-flight open-source reasoning model."
At the same time that the economics of DeepSeek have stunned the AI world, the advance will lead to a continued investment in cutting-edge chip and networking technology for AI, mentioned Feldman.
Also: Is DeepSeek's new image model another win for cheaper AI?
"The public markets have been wrong every single time in the past 50 years," introduced Feldman, alluding to the massive sell-off in shares of Nvidia and other AI technology providers. "Every time compute has been made less expensive, they [public market investors] have systematically assumed that made the market smaller. And in every single instance, over 50 years, it's made the market bigger."
Feldman cited the example of driving down the price of x86 PCs, which led to more PCs being sold and used. Nowadays, he noted, "You have 25 computers in your house. You have one in your pocket, you've got one you're working on, your dishwasher has one, your washing machine has one, your TVs each have one."
Not only more of the same, but larger and larger AI systems will be built to get results beyond the reach of commodity AI -- a point that Feldman has been making since Cerebras's founding almost a decade ago.
"When you are 50 or 70 times faster than the competition, you can do things they can't do at all," he mentioned, alluding to Cerebras's CS-3 and its chip, the world's largest semiconductor, the WSE-3. "At some point, differences in degree become differences in kind."
Also: Apple researchers reveal the secret sauce behind DeepSeek AI.
Cerebras started its public inference service last August, demonstrating speeds much faster than most other providers for running generative AI. It states to be "the world's fastest AI inference provider."
Aside from the distilled Llama model, Cerebras is not currently offering the full R1 in inference because doing so is cost-prohibitive for most clients.
"A 671-billion-parameter model is an expensive model to run," says Feldman, referring to the full R1. "What we saw with Llama 405B was a huge amount of interest at the 70B node and much less at the 405B node because it was way more expensive. That's where the market is right now."
Cerebras does have some clients who pay for the full Llama 405B because "they find the added accuracy worth the added cost," he noted.
Cerebras is also betting that privacy and security are aspects it can use to its advantage. The initial enthusiasm for DeepSeek was followed by numerous reports of concerns with the model's handling of data.
"If you use their app, your data goes to China," noted Feldman of the Android and iOS native apps from DeepSeek AI. "If you use us, the data is hosted in the US, we don't store your weights or any of your information, all that stays in the US"
Asked about numerous security vulnerabilities that researchers have publicized about DeepSeek R1, Feldman was philosophical. Some issues will be worked out as the technology matures, he indicated.
Also: Security firm discovers DeepSeek has 'direct links' to Chinese government servers.
"This industry is moving so fast. Nobody's seen anything like it," noted Feldman. "It's getting advanced week over week, month over month. But is it perfect? No. Should you use an LLM [large language model] to replace your common sense? You should not."
Following the R1 announcement, Cerebras last Thursday revealed it has added support for running Le Chat, the inference prompt run by French AI startup Mistral. When running Le Chat's "Flash Answers" feature, at 1,100 tokens per second, the model is "10 times faster than popular models such as ChatGPT 4o, Sonnet [website], and DeepSeek R1," claimed Cerebras, "making it the world's fastest AI assistant."
L’innovation technologique transforme la restauration. SELF, le premier restaurant entièrement automatisé, ouvre à l’aéroport de Barcelone. Grâce à un......
Google’s AI lab, DeepMind, has unveiled a new AI model, AlphaGeometry2, which they claim outperforms some of the top minds who have won a gold medal i......
Daimler Truck is one of the world’s largest manufacturers of commercial vehicles, including trucks and buses. The organization operates globally across fou......
Market Impact Analysis
Market Growth Trend
2018 | 2019 | 2020 | 2021 | 2022 | 2023 | 2024 |
---|---|---|---|---|---|---|
23.1% | 27.8% | 29.2% | 32.4% | 34.2% | 35.2% | 35.6% |
Quarterly Growth Rate
Q1 2024 | Q2 2024 | Q3 2024 | Q4 2024 |
---|---|---|---|
32.5% | 34.8% | 36.2% | 35.6% |
Market Segments and Growth Drivers
Segment | Market Share | Growth Rate |
---|---|---|
Machine Learning | 29% | 38.4% |
Computer Vision | 18% | 35.7% |
Natural Language Processing | 24% | 41.5% |
Robotics | 15% | 22.3% |
Other AI Technologies | 14% | 31.8% |
Technology Maturity Curve
Different technologies within the ecosystem are at varying stages of maturity:
Competitive Landscape Analysis
Company | Market Share |
---|---|
Google AI | 18.3% |
Microsoft AI | 15.7% |
IBM Watson | 11.2% |
Amazon AI | 9.8% |
OpenAI | 8.4% |
Future Outlook and Predictions
The Gets Ways Help landscape is evolving rapidly, driven by technological advancements, changing threat vectors, and shifting business requirements. Based on current trends and expert analyses, we can anticipate several significant developments across different time horizons:
Year-by-Year Technology Evolution
Based on current trajectory and expert analyses, we can project the following development timeline:
Technology Maturity Curve
Different technologies within the ecosystem are at varying stages of maturity, influencing adoption timelines and investment priorities:
Innovation Trigger
- Generative AI for specialized domains
- Blockchain for supply chain verification
Peak of Inflated Expectations
- Digital twins for business processes
- Quantum-resistant cryptography
Trough of Disillusionment
- Consumer AR/VR applications
- General-purpose blockchain
Slope of Enlightenment
- AI-driven analytics
- Edge computing
Plateau of Productivity
- Cloud infrastructure
- Mobile applications
Technology Evolution Timeline
- Improved generative models
- specialized AI applications
- AI-human collaboration systems
- multimodal AI platforms
- General AI capabilities
- AI-driven scientific breakthroughs
Expert Perspectives
Leading experts in the ai tech sector provide diverse perspectives on how the landscape will evolve over the coming years:
"The next frontier is AI systems that can reason across modalities and domains with minimal human guidance."
— AI Researcher
"Organizations that develop effective AI governance frameworks will gain competitive advantage."
— Industry Analyst
"The AI talent gap remains a critical barrier to implementation for most enterprises."
— Chief AI Officer
Areas of Expert Consensus
- Acceleration of Innovation: The pace of technological evolution will continue to increase
- Practical Integration: Focus will shift from proof-of-concept to operational deployment
- Human-Technology Partnership: Most effective implementations will optimize human-machine collaboration
- Regulatory Influence: Regulatory frameworks will increasingly shape technology development
Short-Term Outlook (1-2 Years)
In the immediate future, organizations will focus on implementing and optimizing currently available technologies to address pressing ai tech challenges:
- Improved generative models
- specialized AI applications
- enhanced AI ethics frameworks
These developments will be characterized by incremental improvements to existing frameworks rather than revolutionary changes, with emphasis on practical deployment and measurable outcomes.
Mid-Term Outlook (3-5 Years)
As technologies mature and organizations adapt, more substantial transformations will emerge in how security is approached and implemented:
- AI-human collaboration systems
- multimodal AI platforms
- democratized AI development
This period will see significant changes in security architecture and operational models, with increasing automation and integration between previously siloed security functions. Organizations will shift from reactive to proactive security postures.
Long-Term Outlook (5+ Years)
Looking further ahead, more fundamental shifts will reshape how cybersecurity is conceptualized and implemented across digital ecosystems:
- General AI capabilities
- AI-driven scientific breakthroughs
- new computing paradigms
These long-term developments will likely require significant technical breakthroughs, new regulatory frameworks, and evolution in how organizations approach security as a fundamental business function rather than a technical discipline.
Key Risk Factors and Uncertainties
Several critical factors could significantly impact the trajectory of ai tech evolution:
Organizations should monitor these factors closely and develop contingency strategies to mitigate potential negative impacts on technology implementation timelines.
Alternative Future Scenarios
The evolution of technology can follow different paths depending on various factors including regulatory developments, investment trends, technological breakthroughs, and market adoption. We analyze three potential scenarios:
Optimistic Scenario
Responsible AI driving innovation while minimizing societal disruption
Key Drivers: Supportive regulatory environment, significant research breakthroughs, strong market incentives, and rapid user adoption.
Probability: 25-30%
Base Case Scenario
Incremental adoption with mixed societal impacts and ongoing ethical challenges
Key Drivers: Balanced regulatory approach, steady technological progress, and selective implementation based on clear ROI.
Probability: 50-60%
Conservative Scenario
Technical and ethical barriers creating significant implementation challenges
Key Drivers: Restrictive regulations, technical limitations, implementation challenges, and risk-averse organizational cultures.
Probability: 15-20%
Scenario Comparison Matrix
Factor | Optimistic | Base Case | Conservative |
---|---|---|---|
Implementation Timeline | Accelerated | Steady | Delayed |
Market Adoption | Widespread | Selective | Limited |
Technology Evolution | Rapid | Progressive | Incremental |
Regulatory Environment | Supportive | Balanced | Restrictive |
Business Impact | Transformative | Significant | Modest |
Transformational Impact
Redefinition of knowledge work, automation of creative processes. This evolution will necessitate significant changes in organizational structures, talent development, and strategic planning processes.
The convergence of multiple technological trends—including artificial intelligence, quantum computing, and ubiquitous connectivity—will create both unprecedented security challenges and innovative defensive capabilities.
Implementation Challenges
Ethical concerns, computing resource limitations, talent shortages. Organizations will need to develop comprehensive change management strategies to successfully navigate these transitions.
Regulatory uncertainty, particularly around emerging technologies like AI in security applications, will require flexible security architectures that can adapt to evolving compliance requirements.
Key Innovations to Watch
Multimodal learning, resource-efficient AI, transparent decision systems. Organizations should monitor these developments closely to maintain competitive advantages and effective security postures.
Strategic investments in research partnerships, technology pilots, and talent development will position forward-thinking organizations to leverage these innovations early in their development cycle.
Technical Glossary
Key technical terms and definitions to help understand the technologies discussed in this article.
Understanding the following technical concepts is essential for grasping the full implications of the security threats and defensive measures discussed in this article. These definitions provide context for both technical and non-technical readers.