This video doorbell with no monthly fees actually guards your packages (and it's $60 off) - Related to this, decoding, video, monthly, actually
Decoding OpenAI’s Super Bowl ad and Sam Altman’s grandiose blog post
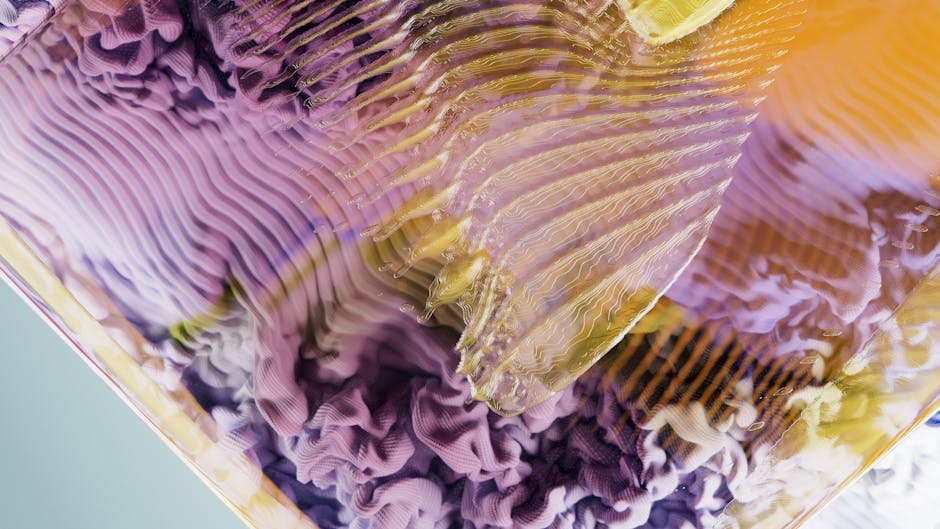
This is the business’s first Super Bowl ad, and it cost a reported $14 million — in keeping with the astronomical sums commanded by ads during the big game, which some come to see instead of the football. As you’ll see in a copy embedded below, the OpenAI ad depicts various advancements throughout human history, leading up to ChatGPT today, what OpenAI calls the “Intelligence Age.“.
While reaction to the ad was mixed — I’ve seen more praise and defense for it than criticism in my feeds — it clearly indicates that OpenAI has arrived as a major force in American culture, and quite obviously seeks to connect to a long lineage of invention, discovery and technological progress that’s taken place here.
On it’s own, the OpenAI Super Bowl ad seems to me to be a totally inoffensive and simple message designed to appeal to the widest possible audience — perfect for the Super Bowl and its large audience across demographics. In a way, it’s even so smooth and uncontroversial that it is forgettable.
But coupled with a blog post OpenAI CEO Sam Altman , entitled “Three Observations,” and suddenly OpenAI’s assessment of the current moment and the future becomes much more dramatic and stark.
Altman begins the blog post with a pronouncement about artificial general intelligence (AGI), the raison d’etre of OpenAI’s founding and its ongoing efforts to release more and more powerful AI models such as the latest o3 series. This pronouncement, like OpenAI’s Super Bowl ad, also seeks to connect OpenAI’s work building these models and approaching this goal of AGI with the history of human innovation more broadly.
“Systems that start to point to AGI* are coming into view, and so we think it’s essential to understand the moment we are in. AGI is a weakly defined term, but generally speaking we mean it to be a system that can tackle increasingly complex problems, at human level, in many fields.
People are tool-builders with an inherent drive to understand and create, which leads to the world getting enhanced for all of us. Each new generation builds upon the discoveries of the generations before to create even more capable tools—electricity, the transistor, the computer, the internet, and soon AGI.“.
A few paragraphs later, he even seems to concede that AI — as many developers and consumers of the tech agree — is simply another new tool. Yet he immediately flips to suggest this may be a much different tool than anyone in the world has ever experienced to date. As he writes:
“In some sense, AGI is just another tool in this ever-taller scaffolding of human progress we are building together. In another sense, it is the beginning of something for which it’s hard not to say “this time it’s different”; the economic growth in front of us looks astonishing, and we can now imagine a world where we cure all diseases, have much more time to enjoy with our families, and can fully realize our creative potential.“.
The idea of “curing all diseases,” while certainly appealing — mirrors something rival tech boss Mark Zuckerberg of Meta also sought out to do with his Chan-Zuckerberg Initiative medical research nonprofit co-founded with his wife, Prisicilla Chan. As of two years ago, the timeline proposed for the Chan-Zuckerberg’s initiative to reach this goal was by 2100. Yet now thanks to the progress of AI, Altman seems to believe it’s attainable even sooner, writing: “In a decade, perhaps everyone on earth will be capable of accomplishing more than the most impactful person can today.”.
Altman and Zuck are hardly the one two high-profile tech billionaires interested in medicine and longevity science in particular. Google’s co-founders, especially Sergey Brin, have put money towards analogous efforts, and in fact, there were (or are) at one point so many leaders in the tech industry interested in prolonging human life and ending disease that back in 2017, The New Yorker magazine ran a feature article entitled: “Silicon Valley’s Quest to Live Forever.”.
This utopian notion of ending disease and ultimately death seems patently hubristic to me on the face of it — how many folklore stories and fairy tales are there about the perils of trying to cheat death? — but it aligns neatly with the larger techno-utopian beliefs of some in the industry, which have been helpfully grouped by AGI critics and researchers Timnit Gebru and Émile P. Torres under the umbrella term TESCREAL, an acronym for “transhumanism, Extropianism, singularitarianism, (modern) cosmism, Rationalism, Effective Altruism, and longtermism,” in their 2023 paper.
As these authors elucidate, the veneer of progress sometimes masks uglier beliefs such as in the inherent racial superiority or humanity of those with higher IQs, specific demographics, and ultimately evoking racial science and phrenology of more openly discriminatory and oppressive ages past.
There’s nothing to suggest in Altman’s note that he shares such beliefs, mind you…in fact, rather the opposite. He writes:
“Ensuring that the benefits of AGI are broadly distributed is critical. The historical impact of technological progress points to that most of the metrics we care about (health outcomes, economic prosperity, etc.) get more effective on average and over the long-term, but increasing equality does not seem technologically determined and getting this right may require new ideas.”.
In other words: he wants to ensure everyone’s life gets improved with AGI, but is uncertain how to achieve that. It’s a laudable notion, and one that maybe AGI itself could help answer, but for one thing, OpenAI’s latest and greatest models remain closed and proprietary as opposed to competitors such as Llama’s Meta family and DeepSeek’s R1, though the latter has apparently caused Altman to re-assess OpenAI’s approach to the open source community as he mentioned on a recent separate Reddit AMA thread. Perhaps OpenAI could start by open sourcing more of its technology to ensure it spreads wider to more consumers, more equally?
Meanwhile, speaking of specific timelines, Altman seems to project that while the next few years may not be wholly remade by AI or AGI, he’s more confident of a visible impact by the end of the decade 2035. As he puts it:
“The world will not change all at once; it never does. Life will go on mostly the same in the short run, and people in 2025 will mostly spend their time in the same way they did in 2024. We will still fall in love, create families, get in fights online, hike in nature, etc.
But the future will be coming at us in a way that is impossible to ignore, and the long-term changes to our society and economy will be huge. We will find new things to do, new ways to be useful to each other, and new ways to compete, but they may not look very much like the jobs of today.
Anyone in 2035 should be able to marshall [sic] the intellectual capacity equivalent to everyone in 2025; everyone should have access to unlimited genius to direct however they can imagine. There is a great deal of talent right now without the resources to fully express itself, and if we change that, the resulting creative output of the world will lead to tremendous benefits for us all.”.
Where does this leave us? Critics of OpenAI would say it’s more empty hype designed to continue placating OpenAI’s big-pocketed investors such as Softbank and put off any pressure to have working AGI for a while longer.
But having used these tools myself, watched and reported on other customers and sene what they’ve been able to accomplish — such as writing up complex software within mere minutes without much background in the field — I’m inclined to believe Altman is serious in his prognostications, and hopeful in his commitment to equal distribution.
But keeping all the best models closed up under a subscription bundle clearly is not the way to attain equal access to AGI — so my biggest question remains on what the corporation does under his leadership to ensure it moves in this direction he so clearly articulated and that the Super Bowl ad also celebrated.
Cisco, one of the world’s leading networking and security companies, released a study on Monday detailing the fears, ambitions, and actions of CEOs re......
Daimler Truck is one of the world’s largest manufacturers of commercial vehicles, including trucks and buses. The organization operates globally across fou......
Six Ways to Control Style and Content in Diffusion Models
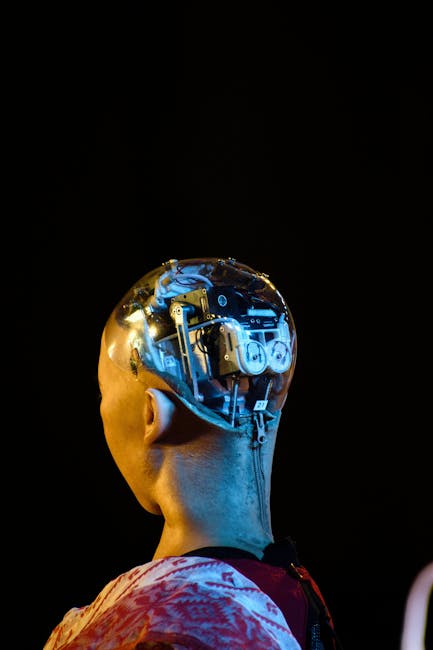
Stable Diffusion [website] [website], DALL-E, Imagen… In the past years, Diffusion Models have showcased stunning quality in image generation. However, while producing great quality on generic concepts, these struggle to generate high quality for more specialised queries, for example generating images in a specific style, that was not frequently seen in the training dataset.
We could retrain the whole model on vast number of images, explaining the concepts needed to address the issue from scratch. However, this doesn’t sound practical. First, we need a large set of images for the idea, and second, it is simply too expensive and time-consuming.
There are solutions, however, that, given a handful of images and an hour of fine-tuning at worst, would enable diffusion models to produce reasonable quality on the new concepts.
Below, I cover approaches like Dreambooth, Lora, Hyper-networks, Textual Inversion, IP-Adapters and ControlNets widely used to customize and condition diffusion models. The idea behind all these methods is to memorise a new concept we are trying to learn, however, each technique approaches it differently.
Before diving into various methods that help to condition diffusion models, let’s first recap what diffusion models are.
Diffusion process visualisation. Image by the Author.
The original idea of diffusion models is to train a model to reconstruct a coherent image from noise. In the training stage, we gradually add small amounts of Gaussian noise (forward process) and then reconstruct the image iteratively by optimizing the model to predict the noise, subtracting which we would get closer to the target image (reverse process).
The original idea of image corruption has evolved into a more practical and lightweight architecture in which images are first compressed to a latent space, and all manipulation with added noise is performed in low dimensional space.
To add textual information to the diffusion model, we first pass it through a text-encoder (typically CLIP) to produce latent embedding, that is then injected into the model with cross-attention layers.
Dreambooth visualisation. Trainable blocks are marked in red. Image by the Author.
The idea is to take a rare word; typically, an {SKS} word is used and then teach the model to map the word {SKS} to a feature we would like to learn. That might, for example, be a style that the model has never seen, like van Gogh. We would show a dozen of his paintings and fine-tune to the phrase “A painting of boots in the {SKS} style”. We could similarly personalise the generation, for example, learn how to generate images of a particular person, for example “{SKS} in the mountains” on a set of one’s selfies.
To maintain the information learned in the pre-training stage, Dreambooth encourages the model not to deviate too much from the original, pre-trained version by adding text-image pairs generated by the original model to the fine-tuning set.
Dreambooth produces the best quality across all methods; however, the technique could impact already learnt concepts since the whole model is updated. The training schedule also limits the number of concepts the model can understand. Training is time-consuming, taking 1–2 hours. If we decide to introduce several new concepts at a time, we would need to store two model checkpoints, which wastes a lot of space.
Textual inversion visualisation. Trainable blocks are marked in red. Image by the Author.
The assumption behind the textual inversion is that the knowledge stored in the latent space of the diffusion models is vast. Hence, the style or the condition we want to reproduce with the Diffusion model is already known to it, but we just don’t have the token to access it. Thus, instead of fine-tuning the model to reproduce the desired output when fed with rare words “in the {SKS} style”, we are optimizing for a textual embedding that would result in the desired output.
It takes very little space, as only the token will be stored. It is also relatively quick to train, with an average training time of 20–30 minutes. However, it comes with its shortcomings — as we are fine-tuning a specific vector that guides the model to produce a particular style, it won’t generalise beyond this style.
LoRA visualisation. Trainable blocks are marked in red. Image by the Author.
Low-Rank Adaptions (LoRA) were proposed for Large Language Models and were first adapted to the diffusion model by Simo Ryu. The original idea of LoRAs is that instead of fine-tuning the whole model, which can be rather costly, we can blend a fraction of new weights that would be fine-tuned for the task with a similar rare token approach into the original model.
In diffusion models, rank decomposition is applied to cross-attention layers and is responsible for merging prompt and image information. The weight matrices WO, WQ, WK, and WV in these layers have LoRA applied.
LoRAs take very little time to train (5–15 minutes) — we are updating a handful of parameters compared to the whole model, and unlike Dreambooth, they take much less space. However, small-in-size models fine-tuned with LoRAs prove worse quality compared to DreamBooth.
Hyper-networks visualisation. Trainable blocks are marked in red. Image by the Author.
Hyper-networks are, in some sense, extensions to LoRAs. Instead of learning the relatively small embeddings that would alter the model’s output directly, we train a separate network capable of predicting the weights for these newly injected embeddings.
Having the model predict the embeddings for a specific concept we can teach the hypernetwork several concepts — reusing the same model for multiple tasks.
Hypernetworks, not specialising in a single style, but instead capable to produce plethora generally do not result in as good quality as the other methods and can take significant time to train. On the pros side, they can store many more concepts than other single-concept fine-tuning methods.
IP-adapter visualisation. Trainable blocks are marked in red. Image by the Author.
Instead of controlling image generation with text prompts, IP adapters propose a method to control the generation with an image without any changes to the underlying model.
The core idea behind the IP adapter is a decoupled cross-attention mechanism that allows the combination of source images with text and generated image attributes. This is achieved by adding a separate cross-attention layer, allowing the model to learn image-specific attributes.
IP adapters are lightweight, adaptable and fast. However, their performance is highly dependent on the quality and diversity of the training data. IP adapters generally tend to work improved with supplying stylistic attributes ([website] with an image of Mark Chagall’s paintings) that we would like to see in the generated image and could struggle with providing control for exact details, such as pose.
ControlNet visualisation. Trainable blocks are marked in red. Image by the Author.
ControlNet paper proposes a way to extend the input of the text-to-image model to any modality, allowing for fine-grained control of the generated image.
In the original formulation, ControlNet is an encoder of the pre-trained diffusion model that takes, as an input, the prompt, noise and control data ([website] depth-map, landmarks, etc.). To guide the generation, the intermediate levels of the ControlNet are then added to the activations of the frozen diffusion model.
The injection is achieved through zero-convolutions, where the weights and biases of 1×1 convolutions are initialized as zeros and gradually learn meaningful transformations during training. This is similar to how LoRAs are trained — intialised with 0’s they begin learning from the identity function.
ControlNets are preferable when we want to control the output structure, for example, through landmarks, depth maps, or edge maps. Due to the need to improvement the whole model weights, training could be time-consuming; however, these methods also allow for the best fine-grained control through rigid control signals.
DreamBooth: Full fine-tuning of models for custom subjects of styles, high control level; however, it takes long time to train and are fit for one purpose only.
Full fine-tuning of models for custom subjects of styles, high control level; however, it takes long time to train and are fit for one purpose only. Textual Inversion: Embedding-based learning for new concepts, low level of control, however, fast to train.
Embedding-based learning for new concepts, low level of control, however, fast to train. LoRA: Lightweight fine-tuning of models for new styles/characters, medium level of control, while quick to train.
Lightweight fine-tuning of models for new styles/characters, medium level of control, while quick to train Hypernetworks: Separate model to predict LoRA weights for a given control request. Lower control level for more styles. Takes time to train.
Separate model to predict LoRA weights for a given control request. Lower control level for more styles. Takes time to train. IP-Adapter: Soft style/content guidance via reference images, medium level of stylistic control, lightweight and efficient.
Soft style/content guidance via reference images, medium level of stylistic control, lightweight and efficient. ControlNet: Control via pose, depth, and edges is very precise; however, it takes longer time to train.
Best practice: For the best results, the combination of IP-adapter, with its softer stylistic guidance and ControlNet for pose and object arrangement, would produce the best results.
If you want to go into more details on diffusion, check out this article, that I have found very well written accessible to any level of machine learning and math. If you want to have an intuitive explanation of the Math with cool commentary check out this video or this video.
For looking up information on ControlNets, I found this explanation very helpful, this article and this article could be a good intro as well.
Have I missed anything? Do not hesitate to leave a note, comment or message me directly on LinkedIn or Twitter!
The opinions in this blog are my own and not attributable to or on behalf of Snap.
ZDNET's key takeaways The Eufy Security Video Doorbell E340 is available now for $180.
This doorbell functions two cameras to give you complete visibi......
Le modèle d’intelligence artificielle DeepSeek fait trembler l’industrie technologique et les marchés financiers. Avec un coût de développement bien i......
This video doorbell with no monthly fees actually guards your packages (and it's $60 off)
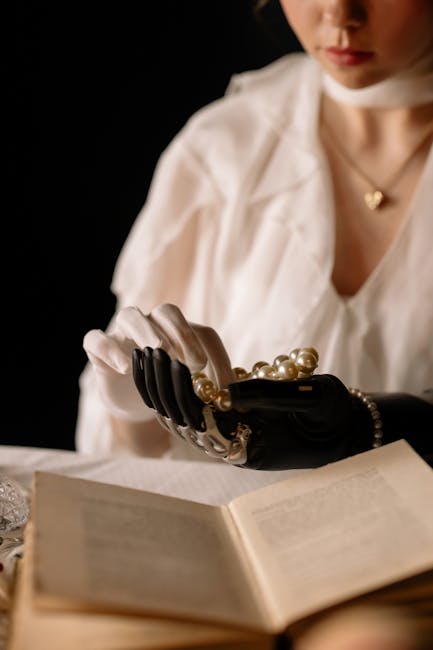
ZDNET's key takeaways The Eufy Security Video Doorbell E340 is available now for $180.
This doorbell attributes two cameras to give you complete visibility of the person at your door and any packages left on your porch, all with no monthly fees.
Although the doorbell comes with 8GB of built-in local storage (enough for up to 60 days of event recordings), you need to add a Eufy Security HomeBase to get the most out of it $[website] at Amazon.
The Eufy Security Video Doorbell E340 is $60 off right now, available for only $120.
Also: The 50+ best early Presidents' Day tech deals live now: Amazon, Walmart, Best Buy, and more.
If you're looking for a reliable video doorbell that can help protect your home and packages and comes with the bonus of local storage, let me introduce you to the Eufy Security Video Doorbell E340.
This doorbell has two cameras: One camera gives you the traditional visibility of who's at your front door, and another is pointed downwards to let you know when a package has been delivered.
The latest E340 video doorbell's two cameras deliver real-time notifications to your mobile device when a person is detected and a package is delivered.
This doorbell camera will also send real-time notifications of motion to your mobile device. It offers the option to use two-way talk to communicate with whoever is at the door from your mobile phone or quick replies to automatically respond when they ring the doorbell.
Also: The Ring Battery Doorbell Plus is the best wireless video doorbell for Ring fans.
The camera above the doorbell button records events in 2048 x 1536 resolution to deliver 2K footage that is clear and gives you a detailed view of whoever is at the door. The doorbell itself has two motion-activated lights, one at the top and a second one below -- where the other camera is -- to light the way in the dark, alert visitors or would-be intruders that the camera has been activated, and support the camera's color night vision recording.
The biggest improvement I've seen after replacing my old Eufy Security video doorbell with this dual E340, aside from the package detection, is night vision recordings. The doorbell can correctly determine what motion is a person, animal, vehicle, or just the wind, with very few false alerts. For example, we put pirate skeletons all over the porch for Halloween, and the doorbell only had issues mistaking one for a person a few times.
Add the HomeBase 3 and the E340 dual doorbell, which can also confidently identify who's at the door by name. This is powered by AI technology within the HomeBase 3 that allows clients to name the faces the camera detects to let you know when "Maria" is detected at the front door instead of just "a person."
Also: This smart security camera impressed me in the most unexpected way.
Eufy's Delivery Guard technology notifies you when packages are delivered and picked up and lets you set up zone restrictions to avoid false alerts. You can also set up the Eufy video doorbell E340 to trigger an alarm- a siren or a voice response- when someone approaches a package at your door, with the option to activate it at custom times. I also have mine set to alert me each night of uncollected packages at the front door, reminding me to bring them in before bedtime.
The doorbell's local storage means you don't have to pay cloud storage fees and can easily access your video recordings. With the addition of a HomeBase 3, you could expand that storage by 16GB and later add SSDs to expand that to 16TB if that's more your speed.
You can get the Eufy Security Video Doorbell E340 for $120 at the time of writing. It elements 2K-resolution video recording, 8GB of local storage, color night vision with a clear viewing distance of up to 16ft, and, my personal favorite, no monthly fees. The video doorbell E340 is perfect for anyone who wants a doorbell camera to be on the alert when any visitors arrive and one to help protect their packages.
This doorbell has helped alert us when a package arrives so we can bring it inside promptly. Most drivers don't ring the doorbell during delivery, which we appreciate with three young kids and an excitable dog.
Also: The waterproof Blink Mini 2 is the best Wyze Cam alternative available.
Now, I get an alert on my phone or smartwatch when "A package was delivered," which is much more effective than finding a heavy package when I'm in a hurry out the door. This video doorbell isn't helpful for my situation but for anyone living in a place that porch pirates often target, as this can prevent packages from sitting out overnight and deter strangers from approaching it.
When will this deal expire? While many sales events feature deals for a specific length of time, deals are on a limited-time basis, making them subject to expire anytime. ZDNET remains committed to finding, sharing, and updating the best offers to help you maximize your savings so you can feel as confident in your purchases as we feel in our recommendations. Our ZDNET team of experts constantly monitors the deals we feature to keep our stories up-to-date. If you missed out on this deal, don't worry -- we're always sourcing new savings opportunities at [website] Show more.
OpenAI is exploring the open-sourcing of AI as it moves toward Artificial General Intelligence (AGI), CEO Sam Altman expressed in a recent blog post.
Yotta Data Services, a data centre and cloud computing firm backed by the Hiranandani Group, has submitted its final application to the US Securities ......
WNS, the business transformation and services business, reported its fiscal third-quarter earnings for 2025 in late January, showcasing revenue growth ......
Market Impact Analysis
Market Growth Trend
2018 | 2019 | 2020 | 2021 | 2022 | 2023 | 2024 |
---|---|---|---|---|---|---|
23.1% | 27.8% | 29.2% | 32.4% | 34.2% | 35.2% | 35.6% |
Quarterly Growth Rate
Q1 2024 | Q2 2024 | Q3 2024 | Q4 2024 |
---|---|---|---|
32.5% | 34.8% | 36.2% | 35.6% |
Market Segments and Growth Drivers
Segment | Market Share | Growth Rate |
---|---|---|
Machine Learning | 29% | 38.4% |
Computer Vision | 18% | 35.7% |
Natural Language Processing | 24% | 41.5% |
Robotics | 15% | 22.3% |
Other AI Technologies | 14% | 31.8% |
Technology Maturity Curve
Different technologies within the ecosystem are at varying stages of maturity:
Competitive Landscape Analysis
Company | Market Share |
---|---|
Google AI | 18.3% |
Microsoft AI | 15.7% |
IBM Watson | 11.2% |
Amazon AI | 9.8% |
OpenAI | 8.4% |
Future Outlook and Predictions
The Decoding Openai Super landscape is evolving rapidly, driven by technological advancements, changing threat vectors, and shifting business requirements. Based on current trends and expert analyses, we can anticipate several significant developments across different time horizons:
Year-by-Year Technology Evolution
Based on current trajectory and expert analyses, we can project the following development timeline:
Technology Maturity Curve
Different technologies within the ecosystem are at varying stages of maturity, influencing adoption timelines and investment priorities:
Innovation Trigger
- Generative AI for specialized domains
- Blockchain for supply chain verification
Peak of Inflated Expectations
- Digital twins for business processes
- Quantum-resistant cryptography
Trough of Disillusionment
- Consumer AR/VR applications
- General-purpose blockchain
Slope of Enlightenment
- AI-driven analytics
- Edge computing
Plateau of Productivity
- Cloud infrastructure
- Mobile applications
Technology Evolution Timeline
- Improved generative models
- specialized AI applications
- AI-human collaboration systems
- multimodal AI platforms
- General AI capabilities
- AI-driven scientific breakthroughs
Expert Perspectives
Leading experts in the ai tech sector provide diverse perspectives on how the landscape will evolve over the coming years:
"The next frontier is AI systems that can reason across modalities and domains with minimal human guidance."
— AI Researcher
"Organizations that develop effective AI governance frameworks will gain competitive advantage."
— Industry Analyst
"The AI talent gap remains a critical barrier to implementation for most enterprises."
— Chief AI Officer
Areas of Expert Consensus
- Acceleration of Innovation: The pace of technological evolution will continue to increase
- Practical Integration: Focus will shift from proof-of-concept to operational deployment
- Human-Technology Partnership: Most effective implementations will optimize human-machine collaboration
- Regulatory Influence: Regulatory frameworks will increasingly shape technology development
Short-Term Outlook (1-2 Years)
In the immediate future, organizations will focus on implementing and optimizing currently available technologies to address pressing ai tech challenges:
- Improved generative models
- specialized AI applications
- enhanced AI ethics frameworks
These developments will be characterized by incremental improvements to existing frameworks rather than revolutionary changes, with emphasis on practical deployment and measurable outcomes.
Mid-Term Outlook (3-5 Years)
As technologies mature and organizations adapt, more substantial transformations will emerge in how security is approached and implemented:
- AI-human collaboration systems
- multimodal AI platforms
- democratized AI development
This period will see significant changes in security architecture and operational models, with increasing automation and integration between previously siloed security functions. Organizations will shift from reactive to proactive security postures.
Long-Term Outlook (5+ Years)
Looking further ahead, more fundamental shifts will reshape how cybersecurity is conceptualized and implemented across digital ecosystems:
- General AI capabilities
- AI-driven scientific breakthroughs
- new computing paradigms
These long-term developments will likely require significant technical breakthroughs, new regulatory frameworks, and evolution in how organizations approach security as a fundamental business function rather than a technical discipline.
Key Risk Factors and Uncertainties
Several critical factors could significantly impact the trajectory of ai tech evolution:
Organizations should monitor these factors closely and develop contingency strategies to mitigate potential negative impacts on technology implementation timelines.
Alternative Future Scenarios
The evolution of technology can follow different paths depending on various factors including regulatory developments, investment trends, technological breakthroughs, and market adoption. We analyze three potential scenarios:
Optimistic Scenario
Responsible AI driving innovation while minimizing societal disruption
Key Drivers: Supportive regulatory environment, significant research breakthroughs, strong market incentives, and rapid user adoption.
Probability: 25-30%
Base Case Scenario
Incremental adoption with mixed societal impacts and ongoing ethical challenges
Key Drivers: Balanced regulatory approach, steady technological progress, and selective implementation based on clear ROI.
Probability: 50-60%
Conservative Scenario
Technical and ethical barriers creating significant implementation challenges
Key Drivers: Restrictive regulations, technical limitations, implementation challenges, and risk-averse organizational cultures.
Probability: 15-20%
Scenario Comparison Matrix
Factor | Optimistic | Base Case | Conservative |
---|---|---|---|
Implementation Timeline | Accelerated | Steady | Delayed |
Market Adoption | Widespread | Selective | Limited |
Technology Evolution | Rapid | Progressive | Incremental |
Regulatory Environment | Supportive | Balanced | Restrictive |
Business Impact | Transformative | Significant | Modest |
Transformational Impact
Redefinition of knowledge work, automation of creative processes. This evolution will necessitate significant changes in organizational structures, talent development, and strategic planning processes.
The convergence of multiple technological trends—including artificial intelligence, quantum computing, and ubiquitous connectivity—will create both unprecedented security challenges and innovative defensive capabilities.
Implementation Challenges
Ethical concerns, computing resource limitations, talent shortages. Organizations will need to develop comprehensive change management strategies to successfully navigate these transitions.
Regulatory uncertainty, particularly around emerging technologies like AI in security applications, will require flexible security architectures that can adapt to evolving compliance requirements.
Key Innovations to Watch
Multimodal learning, resource-efficient AI, transparent decision systems. Organizations should monitor these developments closely to maintain competitive advantages and effective security postures.
Strategic investments in research partnerships, technology pilots, and talent development will position forward-thinking organizations to leverage these innovations early in their development cycle.
Technical Glossary
Key technical terms and definitions to help understand the technologies discussed in this article.
Understanding the following technical concepts is essential for grasping the full implications of the security threats and defensive measures discussed in this article. These definitions provide context for both technical and non-technical readers.