How Johnson & Johnson India Built an In-House OCR Tool to Scan 4 Million Documents - Related to an, q3, scan, million, reports
How Johnson & Johnson India Built an In-House OCR Tool to Scan 4 Million Documents
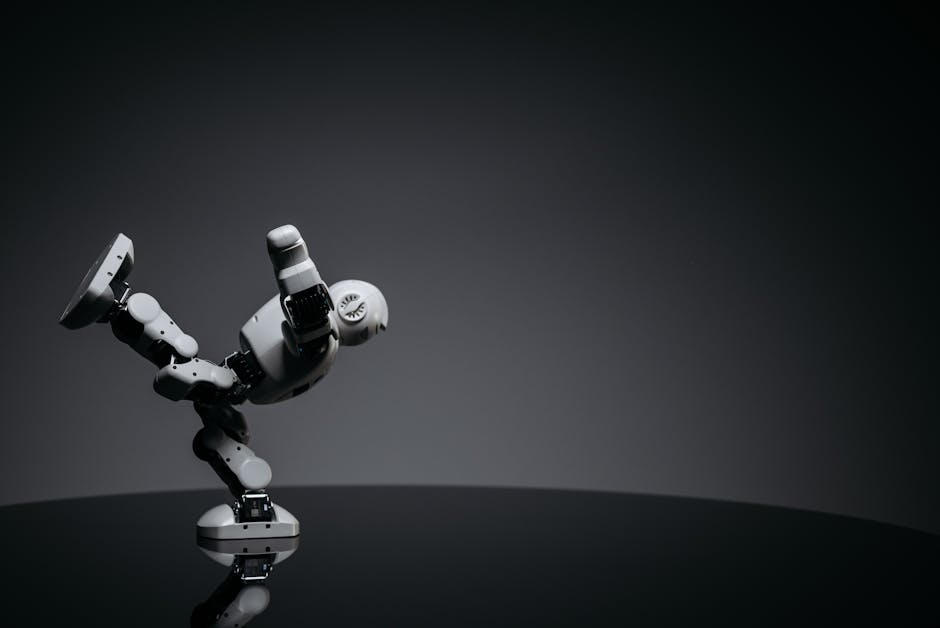
When the data science team at Johnson & Johnson in India faced the daunting challenge of extracting text from nearly 4 million documents, they had two choices. They could either rely on third-party Optical Character Recognition (OCR) services at a significant cost or develop an in-house solution tailored to their needs.
Venkata Karthik T, senior manager of data science at Johnson & Johnson, revealed his journey at MLDS 2025 and offered an insightful look into how necessity, innovation, and cost constraints led them to build a powerful, scalable OCR tool.
“At the end of the day, cost is a very critical factor for any project. So taking care of that is very significant,” Karthik explained. Additionally, using third-party solutions raised privacy concerns.
The project, spanning over a year with multiple iterations, demonstrated the power of in-house innovation. “It’s more like we picked up what was really essential and started implementing them. 95% of the problem gets solved with these things,” Karthik noted.
Handling sensitive internal documents required greater control over the data. Moreover, building an internal tool offered the advantage of customisation, enabling them to tailor the OCR engine to various use cases beyond document scanning.
The Thought Process Behind Building the Tool In-House.
Karthik mentioned that his team evaluated multiple OCR frameworks, prioritising activity metrics, capabilities, and usability. They created datasets for testing, categorising documents into digital, noisy, and handwritten types.
Digital documents were generated using SynthTIGER, noisy documents sourced from the FunSD dataset, and handwritten text taken from the IAM dataset. After rigorous testing, they narrowed their focus to four key OCR models: PaddleOCR, Tesseract, EasyOCR, and HDR Pipeline.
Each framework had its strengths and weaknesses. PaddleOCR excelled at table extraction but struggled with dense text. Tesseract worked well on dense text but had issues with tables. HDR Pipeline performed best for handwritten text.
“So instead of choosing one, we combined PaddleOCR and Tesseract. We took both outputs and saw which one had the highest confidence score. If one model identified text and another missed it, we merged results, improving overall accuracy,” Karthik noted.
The existing process was straightforward: send PDFs or images to a third-party service, extract text, and use it for downstream applications. However, the team sought to replicate and improve this with their own OCR pipeline.
The tool was evaluated using word error rate (WER), character error rate (CER), and accuracy. While third-party APIs achieved nearly 98-99% accuracy on digital and noisy documents, the hybrid model significantly improved internal performance. HDR Pipeline was particularly effective for handwritten text, achieving 85% accuracy.
How AI Helped with Cost – the Biggest Factor.
Cost efficiency was undoubtedly another key consideration. “We don’t want the API up and running all day. It’s an unnecessary cost,” Karthik noted. Instead of a costly front-end UI, the team deployed a backend API on Kubernetes. They optimised infrastructure using batch processing, streaming mode, and event-based triggering to ensure the system ran only when needed.
To further refine text extraction, the team introduced AI-powered error correction. Using ChatGPT for low-confidence words improved accuracy by 3%. They also experimented with fine-tuned BERT models to correct OCR mistakes without relying on expensive third-party APIs.
Additionally, they developed six pre-built extraction templates to streamline data retrieval. These templates allowed people to specify areas of interest, such as key-value pairs, structured tables, or spatial relationships within documents. This reduced the need for manual adjustments and sped up adoption within the organisation.
Since many documents contained tabular data, the team leveraged Microsoft’s Table Transformer. This model identified tables and their components, including rows, columns, and headers, before feeding them into PaddleOCR for text extraction.
For barcodes, they used a combination of YOLOv5 for detection and multiple open-source decoders. If these failed, they applied super-resolution techniques to enhance barcode clarity, boosting decoding accuracy to 84%.
Despite significant progress, Karthik expressed that challenges remain with handwritten text, where even humans struggle to decipher poor handwriting. However, the team is optimistic about integrating vision-language models (VLMs) like OCR-free RAG models to bypass traditional OCR altogether.
Presque toutes les applications de rencontres populaires, telles que Tinder, Badoo ou Bumble, sont conçues pour le public le plus large possible. Elle......
Tech Mahindra is in advanced discussions to establish a global capability centre (GCC) for Ohio-based Goodyear Tire & Rubber Co in Hyderabad, Mint rep......
WNS Reports $333 Mn Revenue in Q3 FY25, Highlighting AI Investments
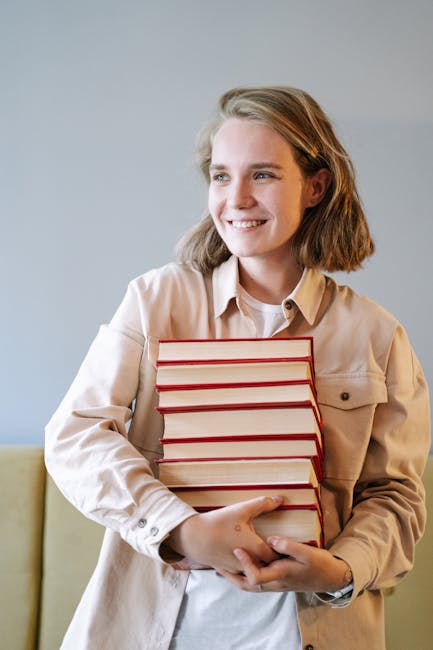
WNS, the business transformation and services firm, reported its fiscal third-quarter earnings for 2025 in late January, showcasing revenue growth and profitability while emphasising its commitment to AI and GenAI-driven transformation.
The enterprise recorded $333 million in revenue, marking a [website] year-over-year increase and a [website] sequential rise. However, full-year guidance was revised downward due to market headwinds.
Despite economic pressures, WNS continues to focus on AI and drive domain-led process automation and cost reduction. “We continue to make solid progress moving large transformational opportunities through the pipeline and are focused on closing these large deals to help accelerate revenue growth,” mentioned CEO Keshav Murugesh.
“WNS remains committed to our ongoing investments in domain expertise, data and analytics, and technology-enabled offerings leveraging AI and GenAI to ensure our ability to deliver long-term sustainable value to all of our stakeholders.”.
Speaking with AIM earlier, Gautam Singh, business unit head of WNS Analytics, mentioned that they are expecting revenue growth with generative AI in play. “About 5% of our revenue in the fiscal year 2025 is expected to be influenced by generative AI. I can cite examples where we’ve achieved 30-40% efficiency improvements through analytics, AI, and automation initiatives,” he had noted.
In Q3, the business added seven new clients and expanded 52 existing relationships, underscoring its growing AI-driven service portfolio. This aligns with WNS’s broader strategy of integrating automation and AI-powered analytics into its digital transformation services.
WNS posted a net profit of $[website] million, up from $[website] million in Q3 last year, supported by favourable currency movements and cost optimisation strategies. Adjusted net income (ANI) stood at $[website] million, down from $[website] million in the previous year, largely due to non-recurring tax benefits recorded in fiscal 2024.
The organization ended the quarter with $[website] million in cash and investments while reducing its debt to $[website] million.
“Our guidance for the full year reflects revenue less repair payments of -2% to -1% on a reported basis and -3% to -1% on a constant currency basis,” noted CFO Arijit Sen. “ANI guidance includes a one-time benefit in Q4 of $[website] million relating to a facility asset sale in India.”.
Google’s AI lab, DeepMind, has unveiled a new AI model, AlphaGeometry2, which they claim outperforms some of the top minds who have won a gold medal i......
I've turned to locally installed AI for research because I don't want third parties using my information to either build a profile or train the......
Accenture revealed on February 7 that it would acquire German management consulting firm Staufen AG to enhance its AI-driven solutions for manufactur......
Yotta Files for Nasdaq Listing, Eyes $463 Mn in IPO Proceeds
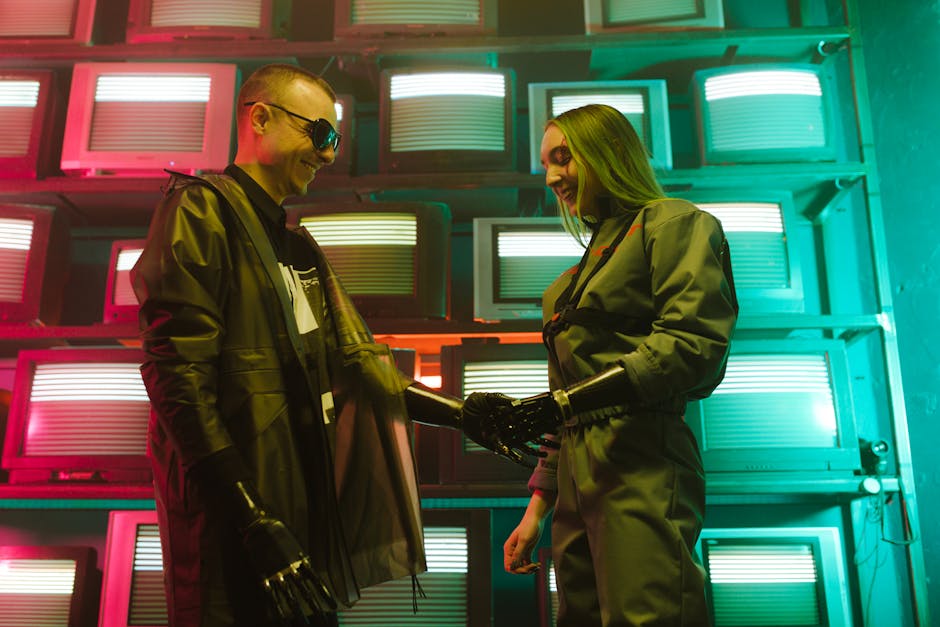
Yotta Data Services, a data centre and cloud computing firm backed by the Hiranandani Group, has submitted its final application to the US Securities and Exchange Commission (SEC) for a Nasdaq listing, CEO Sunil Gupta confirmed.
While Gupta did not disclose the exact size of the initial public offering (IPO), a business presentation to the SEC indicated that the listing is expected to generate approximately $463 million (₹4,064 crore) in cash. The final market registration form was submitted on December 30 through Yotta’s holding entity, Nidar Infrastructure.
“We are in the process of our public listing with the US SEC. While the final IPO size remains undisclosed, this marks the first time the promoters are diluting their equity stake in Yotta. The listing is expected soon, subject to regulatory clearance,” Gupta noted.
Yotta’s IPO proceeds are expected to fund these expansion projects and its growing GPU infrastructure. The firm has already installed 4,000 GPUs across its data centers and is in the process of activating another 2,000. It has also secured two major clients and is supplying more than half of the advanced chips acquired by the Indian government under its AI Mission.
Yotta’s SEC filing valued the enterprise at $[website] billion. Despite reporting $[website] million in revenue for FY24, the firm anticipates a significant jump to $[website] million in the current fiscal year.
However, high capital expenditures—driven by real estate, power, cooling infrastructure, and NVIDIA GPUs priced at around $40,000 each—have kept the corporation in the red. Yotta projects a net loss of $113 million for FY25, expected to narrow to $62 million the following year.
The firm’s net debt, currently at $[website] billion, could rise to $[website] billion by FY26 as it continues to scale its GPU capabilities and data centre expansion.
DeepSeek has grabbed the spotlight in the AI industry as the underdog that briefly became the world’s leading app, overtaking ChatGPT AI assistant. Wh......
Amazon has been focused on bringing generative AI to Alexa for the past few years, hitting multiple delays in launching new capabilities......
Market Impact Analysis
Market Growth Trend
2018 | 2019 | 2020 | 2021 | 2022 | 2023 | 2024 |
---|---|---|---|---|---|---|
23.1% | 27.8% | 29.2% | 32.4% | 34.2% | 35.2% | 35.6% |
Quarterly Growth Rate
Q1 2024 | Q2 2024 | Q3 2024 | Q4 2024 |
---|---|---|---|
32.5% | 34.8% | 36.2% | 35.6% |
Market Segments and Growth Drivers
Segment | Market Share | Growth Rate |
---|---|---|
Machine Learning | 29% | 38.4% |
Computer Vision | 18% | 35.7% |
Natural Language Processing | 24% | 41.5% |
Robotics | 15% | 22.3% |
Other AI Technologies | 14% | 31.8% |
Technology Maturity Curve
Different technologies within the ecosystem are at varying stages of maturity:
Competitive Landscape Analysis
Company | Market Share |
---|---|
Google AI | 18.3% |
Microsoft AI | 15.7% |
IBM Watson | 11.2% |
Amazon AI | 9.8% |
OpenAI | 8.4% |
Future Outlook and Predictions
The Johnson India Built landscape is evolving rapidly, driven by technological advancements, changing threat vectors, and shifting business requirements. Based on current trends and expert analyses, we can anticipate several significant developments across different time horizons:
Year-by-Year Technology Evolution
Based on current trajectory and expert analyses, we can project the following development timeline:
Technology Maturity Curve
Different technologies within the ecosystem are at varying stages of maturity, influencing adoption timelines and investment priorities:
Innovation Trigger
- Generative AI for specialized domains
- Blockchain for supply chain verification
Peak of Inflated Expectations
- Digital twins for business processes
- Quantum-resistant cryptography
Trough of Disillusionment
- Consumer AR/VR applications
- General-purpose blockchain
Slope of Enlightenment
- AI-driven analytics
- Edge computing
Plateau of Productivity
- Cloud infrastructure
- Mobile applications
Technology Evolution Timeline
- Improved generative models
- specialized AI applications
- AI-human collaboration systems
- multimodal AI platforms
- General AI capabilities
- AI-driven scientific breakthroughs
Expert Perspectives
Leading experts in the ai tech sector provide diverse perspectives on how the landscape will evolve over the coming years:
"The next frontier is AI systems that can reason across modalities and domains with minimal human guidance."
— AI Researcher
"Organizations that develop effective AI governance frameworks will gain competitive advantage."
— Industry Analyst
"The AI talent gap remains a critical barrier to implementation for most enterprises."
— Chief AI Officer
Areas of Expert Consensus
- Acceleration of Innovation: The pace of technological evolution will continue to increase
- Practical Integration: Focus will shift from proof-of-concept to operational deployment
- Human-Technology Partnership: Most effective implementations will optimize human-machine collaboration
- Regulatory Influence: Regulatory frameworks will increasingly shape technology development
Short-Term Outlook (1-2 Years)
In the immediate future, organizations will focus on implementing and optimizing currently available technologies to address pressing ai tech challenges:
- Improved generative models
- specialized AI applications
- enhanced AI ethics frameworks
These developments will be characterized by incremental improvements to existing frameworks rather than revolutionary changes, with emphasis on practical deployment and measurable outcomes.
Mid-Term Outlook (3-5 Years)
As technologies mature and organizations adapt, more substantial transformations will emerge in how security is approached and implemented:
- AI-human collaboration systems
- multimodal AI platforms
- democratized AI development
This period will see significant changes in security architecture and operational models, with increasing automation and integration between previously siloed security functions. Organizations will shift from reactive to proactive security postures.
Long-Term Outlook (5+ Years)
Looking further ahead, more fundamental shifts will reshape how cybersecurity is conceptualized and implemented across digital ecosystems:
- General AI capabilities
- AI-driven scientific breakthroughs
- new computing paradigms
These long-term developments will likely require significant technical breakthroughs, new regulatory frameworks, and evolution in how organizations approach security as a fundamental business function rather than a technical discipline.
Key Risk Factors and Uncertainties
Several critical factors could significantly impact the trajectory of ai tech evolution:
Organizations should monitor these factors closely and develop contingency strategies to mitigate potential negative impacts on technology implementation timelines.
Alternative Future Scenarios
The evolution of technology can follow different paths depending on various factors including regulatory developments, investment trends, technological breakthroughs, and market adoption. We analyze three potential scenarios:
Optimistic Scenario
Responsible AI driving innovation while minimizing societal disruption
Key Drivers: Supportive regulatory environment, significant research breakthroughs, strong market incentives, and rapid user adoption.
Probability: 25-30%
Base Case Scenario
Incremental adoption with mixed societal impacts and ongoing ethical challenges
Key Drivers: Balanced regulatory approach, steady technological progress, and selective implementation based on clear ROI.
Probability: 50-60%
Conservative Scenario
Technical and ethical barriers creating significant implementation challenges
Key Drivers: Restrictive regulations, technical limitations, implementation challenges, and risk-averse organizational cultures.
Probability: 15-20%
Scenario Comparison Matrix
Factor | Optimistic | Base Case | Conservative |
---|---|---|---|
Implementation Timeline | Accelerated | Steady | Delayed |
Market Adoption | Widespread | Selective | Limited |
Technology Evolution | Rapid | Progressive | Incremental |
Regulatory Environment | Supportive | Balanced | Restrictive |
Business Impact | Transformative | Significant | Modest |
Transformational Impact
Redefinition of knowledge work, automation of creative processes. This evolution will necessitate significant changes in organizational structures, talent development, and strategic planning processes.
The convergence of multiple technological trends—including artificial intelligence, quantum computing, and ubiquitous connectivity—will create both unprecedented security challenges and innovative defensive capabilities.
Implementation Challenges
Ethical concerns, computing resource limitations, talent shortages. Organizations will need to develop comprehensive change management strategies to successfully navigate these transitions.
Regulatory uncertainty, particularly around emerging technologies like AI in security applications, will require flexible security architectures that can adapt to evolving compliance requirements.
Key Innovations to Watch
Multimodal learning, resource-efficient AI, transparent decision systems. Organizations should monitor these developments closely to maintain competitive advantages and effective security postures.
Strategic investments in research partnerships, technology pilots, and talent development will position forward-thinking organizations to leverage these innovations early in their development cycle.
Technical Glossary
Key technical terms and definitions to help understand the technologies discussed in this article.
Understanding the following technical concepts is essential for grasping the full implications of the security threats and defensive measures discussed in this article. These definitions provide context for both technical and non-technical readers.