Honeywell Looks Accelerate: Latest Updates and Analysis
Honeywell Looks to Accelerate Kidney Diagnosis Using AI with Digital Holographic Microscopy
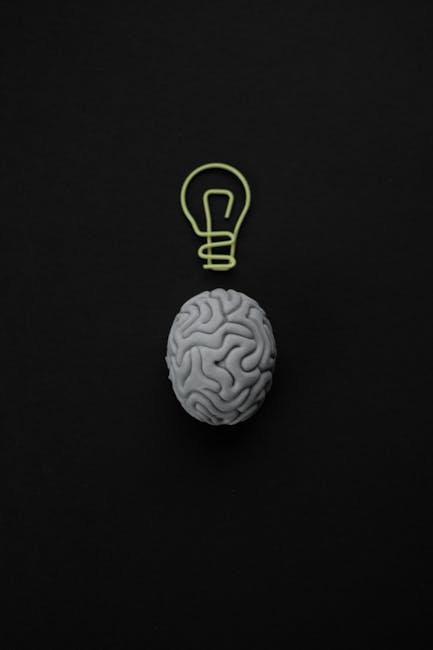
Global technology conglomerate Honeywell unveiled a new Digital Holographic Microscopy technology that uses AI to streamline medical diagnostics, enabling faster and more efficient patient care. It has potential applications across various industries, but its most immediate impact could be in healthcare testing, particularly for patients undergoing peritoneal dialysis.
Peritoneal dialysis patients face a heightened risk of abdominal lining infections, which currently take one to two days to diagnose due to the need for specialised laboratory equipment. Honeywell’s digital holographic microscopy aims to reduce this diagnostic time by capturing high-resolution images of a patient’s dialysis fluid at the point-of-care using a portable device.
AI algorithms analyse these images to determine white blood cell counts, allowing for rapid infection detection and enabling quicker treatment decisions.
As noted by Sarah Martin, president of Honeywell Sensing Solutions, the demand for rapid and precise diagnostics is increasing, requiring tools that enable quicker decision-making. Innovations such as digital holographic microscopy enhance accessibility to testing while improving efficiency in healthcare systems.
Unlike conventional microscopes, Digital Holographic Microscopy does not require expensive lenses or complex optical systems. Instead, it utilises light passing through a sample to generate holographic images, which are then processed using computational algorithms.
AI and machine learning models help differentiate between cell types, eliminating the need for chemical staining, making biological sample preparation easier and faster.
While its immediate focus is on healthcare, this can also be used in environmental monitoring. The technology can analyse air pollutants to assess indoor air quality and monitor microbial content in liquid samples, ensuring water safety in industries such as pharmaceuticals and food production.
Interestingly, a number of big tech companies and venture funds are investing in healthcare. Amazon, Microsoft, Google and Oracle are just a few of the tech titans in the space.
Honeywell has also been supporting rising startups. A few years ago Honeywell Hometown Solutions India Foundation (HHSIF), the philanthropic arm of Honeywell, partnered with IISc’s Society of Innovation and Development (SID) to support deep-tech startups. The initiative aimed to help startups transition from incubation to securing early seed investments.
After weeks of waiting, OpenAI has finally introduced [website], its latest and largest AI language model. It was internally referred to as Orion.
Un jeune Chinois, connu sous le nom de famille Liu, a été victime d’une fraude. Il pensait être en couple avec une femme nommée Mme Jiao. Liu a envoyé......
Welcome to part 2 of my LLM deep dive. If you’ve not read Part 1, I highly encourage you to check it out first.
Previously, we covered the first two ......
Soket AI Labs’ Introduces Project EKA to Develop Sovereign AI Models for India
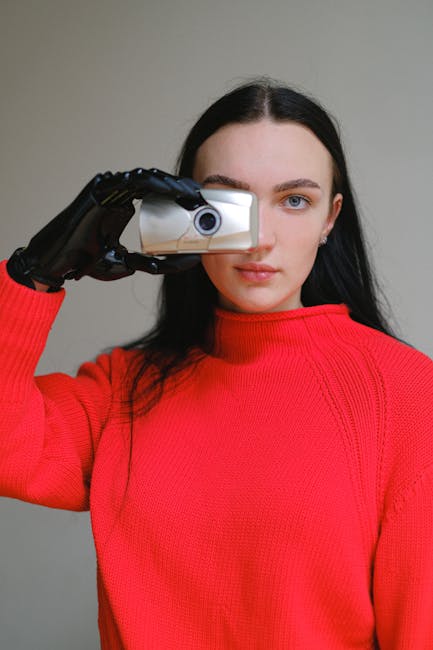
Project EKA, spearheaded by AI startup Soket Labs has emerged as India’s ambitious initiative to develop state-of-the-art foundation models that rival global AI systems while being optimised for India’s unique linguistic and socio-economic landscape.
Project EKA seeks to unite AI researchers, engineers, and institutions across the country to develop multilingual, high-efficiency AI models that cater to India’s needs while competing at a global scale.
, the initiative is focused on building an open, ethical, and high-impact AI ecosystem. Experts from premier institutions such as IITs, IISc, and other global research centers are collaborating to create a self-reliant AI infrastructure that spans multiple domains, from education and finance to national security and agriculture.
Beyond technological advancement, Project EKA aims to democratise AI access. AI-powered education tools could ensure that children across rural and urban areas learn in their native languages. In healthcare, AI-driven diagnostics could improve accessibility and efficiency. Meanwhile, in national security, real-time multilingual intelligence could enhance defense capabilities.
The project is rapidly gaining traction, with an expanding list of contributors from academia, industry, and AI research communities. While still in its early stages, EKA represents a growing movement toward India’s AI sovereignty, signaling a shift from AI dependency to AI leadership.
“I think we need at least $10 million to start working on frontier tech, and this money should be purely dedicated to R&D for building these models—no distractions like building applications or even thinking about GTM. This is where investors and founders need to align with patient capital,” said Abhishek Upperwal, founder and CEO of Soket AI Labs in an earlier interaction with AIM.
India’s push to develop its own AI foundation model gained momentum following DeepSeek’s launch. Upperwal had earlier noted that Pragna-1B, Soket’s [website] billion-parameter AI model, is a step toward building frontier models.
Trained on a $100K budget, the plan is to bootstrap larger models using smaller ones and open-source alternatives while keeping compute costs low. He emphasised that high-quality data and training optimisations make this feasible, citing DeepSeek as an example.
However, with only $2-3 million in funding, progress on such models would be slow or deprioritised in favor of revenue-generating products.
Global technology conglomerate Honeywell unveiled a new Digital Holographic Microscopy technology that uses AI to streamline medical diagnostics, enab......
Cloud-based data storage business Snowflake on Thursday unveiled its plans to open the Silicon Valley AI Hub, a dedicated space for developers, startu......
L’essor de l’intelligence artificielle est palpable dans le Doubs, où une nouvelle génération d’agences IA se développe pour répondre aux demandes cro......
Snowflake to Create a ‘Silicon Valley AI Hub’, Invest $200 Million to Back Startups
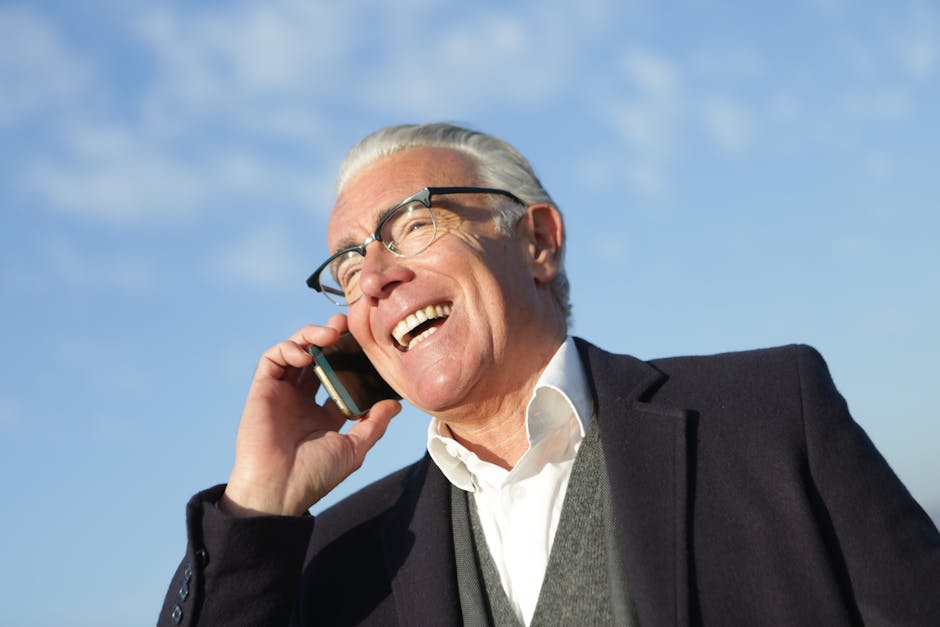
Cloud-based data storage firm Snowflake on Thursday presented its plans to open the Silicon Valley AI Hub, a dedicated space for developers, startups, and business leaders to collaborate and shape the future of AI.
Located in Menlo Park in California, the nearly 30,000 square foot hub will feature a range of spaces designed for the AI ecosystem, including a startup hub, event spaces, training rooms, a customer experience centre, and a video production studio. The space will offer enterprise executives, AI engineers, and early-stage startups a collaborative environment to define strategies, experiment with new technologies, and build their businesses.
“Snowflake is the most consequential data and AI corporation in the world today,” expressed Sridhar Ramaswamy, CEO of Snowflake.
“Whether you’re a developer looking for hands-on experience, a startup founder looking for a place to collaborate, or an executive looking to explore the art of the possible, the Silicon Valley AI Hub will serve as the epicentre of AI development and collaboration,” he added.
Through its Snowflake Startup Accelerator, the business has introduced a $200 million investment to support next-generation startups building AI-based products and industry solutions on the Snowflake platform.
The business aims to deliver technical assistance, give free credits, and unlock access to additional capital to help early-stage startups grow their businesses in the AI Data Cloud.
Moreover, Snowflake is also investing $20 million in AI upskilling to help train and certify more people on the platform.
Snowflake has made several announcements not long ago, including its plans to integrate OpenAI’s latest models into its Cortex AI platform and launching its new Cortex Agents.
With an AI Hub and additional investment to help AI startups, it could position itself as a helping force for AI-focused solutions in the cloud space.
Project EKA, spearheaded by AI startup Soket Labs has emerged as India’s ambitious initiative to develop state-of-the-art foundation models that rival......
As a general rule, I'm not a huge fan of talking to AI chatbots. Even though many of them sound pretty human, they're still.
After weeks of waiting, OpenAI has finally introduced [website], its latest and largest AI language model. It was internally referred to as Orion.
Market Impact Analysis
Market Growth Trend
2018 | 2019 | 2020 | 2021 | 2022 | 2023 | 2024 |
---|---|---|---|---|---|---|
23.1% | 27.8% | 29.2% | 32.4% | 34.2% | 35.2% | 35.6% |
Quarterly Growth Rate
Q1 2024 | Q2 2024 | Q3 2024 | Q4 2024 |
---|---|---|---|
32.5% | 34.8% | 36.2% | 35.6% |
Market Segments and Growth Drivers
Segment | Market Share | Growth Rate |
---|---|---|
Machine Learning | 29% | 38.4% |
Computer Vision | 18% | 35.7% |
Natural Language Processing | 24% | 41.5% |
Robotics | 15% | 22.3% |
Other AI Technologies | 14% | 31.8% |
Technology Maturity Curve
Different technologies within the ecosystem are at varying stages of maturity:
Competitive Landscape Analysis
Company | Market Share |
---|---|
Google AI | 18.3% |
Microsoft AI | 15.7% |
IBM Watson | 11.2% |
Amazon AI | 9.8% |
OpenAI | 8.4% |
Future Outlook and Predictions
The Honeywell Looks Accelerate landscape is evolving rapidly, driven by technological advancements, changing threat vectors, and shifting business requirements. Based on current trends and expert analyses, we can anticipate several significant developments across different time horizons:
Year-by-Year Technology Evolution
Based on current trajectory and expert analyses, we can project the following development timeline:
Technology Maturity Curve
Different technologies within the ecosystem are at varying stages of maturity, influencing adoption timelines and investment priorities:
Innovation Trigger
- Generative AI for specialized domains
- Blockchain for supply chain verification
Peak of Inflated Expectations
- Digital twins for business processes
- Quantum-resistant cryptography
Trough of Disillusionment
- Consumer AR/VR applications
- General-purpose blockchain
Slope of Enlightenment
- AI-driven analytics
- Edge computing
Plateau of Productivity
- Cloud infrastructure
- Mobile applications
Technology Evolution Timeline
- Improved generative models
- specialized AI applications
- AI-human collaboration systems
- multimodal AI platforms
- General AI capabilities
- AI-driven scientific breakthroughs
Expert Perspectives
Leading experts in the ai tech sector provide diverse perspectives on how the landscape will evolve over the coming years:
"The next frontier is AI systems that can reason across modalities and domains with minimal human guidance."
— AI Researcher
"Organizations that develop effective AI governance frameworks will gain competitive advantage."
— Industry Analyst
"The AI talent gap remains a critical barrier to implementation for most enterprises."
— Chief AI Officer
Areas of Expert Consensus
- Acceleration of Innovation: The pace of technological evolution will continue to increase
- Practical Integration: Focus will shift from proof-of-concept to operational deployment
- Human-Technology Partnership: Most effective implementations will optimize human-machine collaboration
- Regulatory Influence: Regulatory frameworks will increasingly shape technology development
Short-Term Outlook (1-2 Years)
In the immediate future, organizations will focus on implementing and optimizing currently available technologies to address pressing ai tech challenges:
- Improved generative models
- specialized AI applications
- enhanced AI ethics frameworks
These developments will be characterized by incremental improvements to existing frameworks rather than revolutionary changes, with emphasis on practical deployment and measurable outcomes.
Mid-Term Outlook (3-5 Years)
As technologies mature and organizations adapt, more substantial transformations will emerge in how security is approached and implemented:
- AI-human collaboration systems
- multimodal AI platforms
- democratized AI development
This period will see significant changes in security architecture and operational models, with increasing automation and integration between previously siloed security functions. Organizations will shift from reactive to proactive security postures.
Long-Term Outlook (5+ Years)
Looking further ahead, more fundamental shifts will reshape how cybersecurity is conceptualized and implemented across digital ecosystems:
- General AI capabilities
- AI-driven scientific breakthroughs
- new computing paradigms
These long-term developments will likely require significant technical breakthroughs, new regulatory frameworks, and evolution in how organizations approach security as a fundamental business function rather than a technical discipline.
Key Risk Factors and Uncertainties
Several critical factors could significantly impact the trajectory of ai tech evolution:
Organizations should monitor these factors closely and develop contingency strategies to mitigate potential negative impacts on technology implementation timelines.
Alternative Future Scenarios
The evolution of technology can follow different paths depending on various factors including regulatory developments, investment trends, technological breakthroughs, and market adoption. We analyze three potential scenarios:
Optimistic Scenario
Responsible AI driving innovation while minimizing societal disruption
Key Drivers: Supportive regulatory environment, significant research breakthroughs, strong market incentives, and rapid user adoption.
Probability: 25-30%
Base Case Scenario
Incremental adoption with mixed societal impacts and ongoing ethical challenges
Key Drivers: Balanced regulatory approach, steady technological progress, and selective implementation based on clear ROI.
Probability: 50-60%
Conservative Scenario
Technical and ethical barriers creating significant implementation challenges
Key Drivers: Restrictive regulations, technical limitations, implementation challenges, and risk-averse organizational cultures.
Probability: 15-20%
Scenario Comparison Matrix
Factor | Optimistic | Base Case | Conservative |
---|---|---|---|
Implementation Timeline | Accelerated | Steady | Delayed |
Market Adoption | Widespread | Selective | Limited |
Technology Evolution | Rapid | Progressive | Incremental |
Regulatory Environment | Supportive | Balanced | Restrictive |
Business Impact | Transformative | Significant | Modest |
Transformational Impact
Redefinition of knowledge work, automation of creative processes. This evolution will necessitate significant changes in organizational structures, talent development, and strategic planning processes.
The convergence of multiple technological trends—including artificial intelligence, quantum computing, and ubiquitous connectivity—will create both unprecedented security challenges and innovative defensive capabilities.
Implementation Challenges
Ethical concerns, computing resource limitations, talent shortages. Organizations will need to develop comprehensive change management strategies to successfully navigate these transitions.
Regulatory uncertainty, particularly around emerging technologies like AI in security applications, will require flexible security architectures that can adapt to evolving compliance requirements.
Key Innovations to Watch
Multimodal learning, resource-efficient AI, transparent decision systems. Organizations should monitor these developments closely to maintain competitive advantages and effective security postures.
Strategic investments in research partnerships, technology pilots, and talent development will position forward-thinking organizations to leverage these innovations early in their development cycle.
Technical Glossary
Key technical terms and definitions to help understand the technologies discussed in this article.
Understanding the following technical concepts is essential for grasping the full implications of the security threats and defensive measures discussed in this article. These definitions provide context for both technical and non-technical readers.