Akool combines GenAI models with 2D avatars to create lifelike characters - Related to avatars, combines, embedding, representative, akool
Akool combines GenAI models with 2D avatars to create lifelike characters
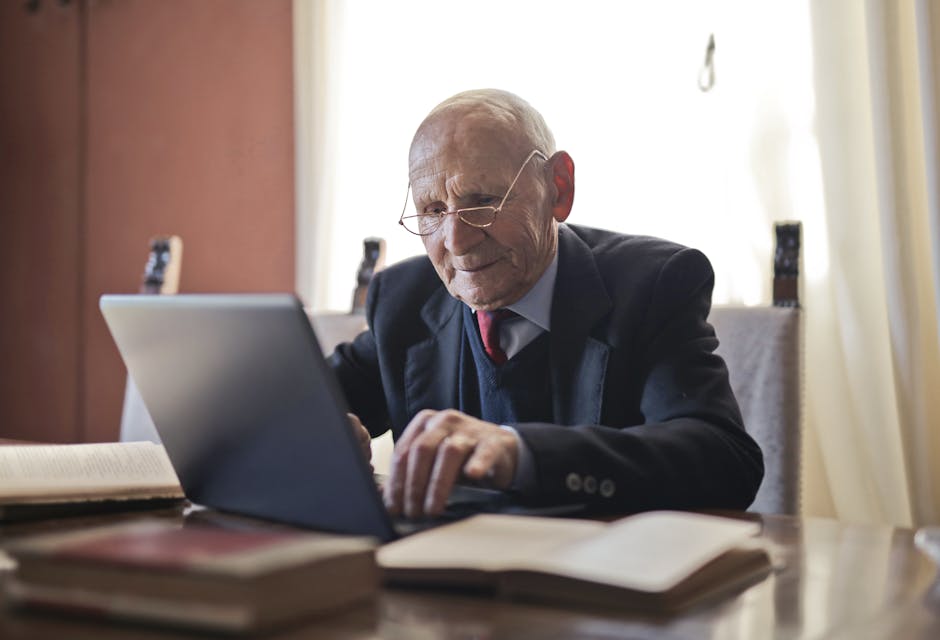
Akool, a startup doing AI-driven avatar content creation, presented enhancements to Akool Streaming Avatars that connect avatars with AI models.
Akool has added advanced video generation technology that now seamlessly integrates with large language models (LLMs) to help model builders create dynamic, 2D lifelike avatars.
With the ability to upload a photo and provide a voice recording, clients can create a personalized avatar that becomes a natural extension of the LLM. Making interactions with the avatar feel more human with a face and voice familiar to the end user.
Human interaction is key to consumers — 82% of and 74% of consumers say they want more of it. Available now, Akool Streaming Avatars significantly close the gap between AI models and end-clients, making AI more accessible, engaging, and human-like, enabling businesses to differentiate their offerings and drive user adoption, the business noted.
Ultimately, Akool Streaming Avatars make traditionally text-based LLMs human through emotive real-time engagement.
“Consumers find static avatars boring, bland and, frankly, ineffective. But Akool Streaming Avatars are dynamic and expressive, creating new levels of engagement and interactivity,” mentioned Jiajun Lu, CEO of Akool, in a statement. “From individual content producers to large enterprises, our Streaming Avatars significantly reduce the cost of video creation and are an ideal option for anyone looking for new and efficient ways to use AI to reach global audiences and end-customers.”.
In a message to GamesBeat, Lu noted his inspiration came from a blend of interests and market needs, but. He was primarily excited to create digital humans.
Moving to another aspect, this advanced innovation empowers businesses to build and deliver advanced, context-aware avatars that provide real-time responses, transforming industries such as:
E-Commerce: Virtual sales assistants guide consumers through product inquiries and purchasing decisions. Akool’s avatars have reduced customer service response times by 40% while increasing user satisfaction by 30%.
Virtual sales assistants guide customers through product inquiries and. Purchasing decisions. Akool’s avatars have reduced customer service response times by 40% while increasing user satisfaction by 30%. Education: Develop interactive, engaging lessons with lifelike virtual instructors that provide real-time feedback.
Develop interactive, engaging lessons with lifelike virtual instructors that provide real-time feedback. Healthcare: Avatars provide personalized medical advice from a familiar face by accessing patient data and delivering empathetic responses.
Avatars provide personalized medical advice from a familiar face by accessing patient data and. Delivering empathetic responses. Customer service: LLM-driven avatars handle inquiries with emotional intelligence, reducing response times and improving satisfaction.
By leveraging Akool’s studio-grade video generation technology. end-customers also gain access to easier integration. Ready-to-use application programming interfaces (APIs) and software development kits (SDKs) allow for quick deployment and the integration of avatars to existing mobile and. Web applications.
Akool also says the avatars have emotional intelligence. Akool Streaming Avatars convey the speaker’s emotions naturally, enhancing the authenticity of live interactions and fostering deeper audience engagement. With the lowest latency on the market, end consumers can have natural and interactive experiences, the firm stated.
And it enables gesture-capable avatars. By delivering intricate details such as facial expressions, body language, and gestures, Akool Streaming Avatars enable the creation of highly realistic and expressive characters. The corporation mentioned.
The corporation offers two variations of AI Avatars, including streaming avatars. These are designed for real-time interaction, this AI avatar can respond dynamically to inputs. Making it suitable for interactive sessions such as live customer support.
And it also has talking avatars. They’re designed to deliver pre-recorded or scripted messages in a dynamic and engaging way. This tool generates a video of an avatar speaking based on text input or pre-recorded audio. The avatar mimics human-like lip-syncing and facial expressions, making it suitable for marketing videos, e-learning content, personalized messages and social media content.
Founded in 2022 and already achieving nearly $40 million in invoiced ARR. The business is a global leader in generative AI-driven technology, transforming the digital content creation landscape. Akool mentioned its solutions have already saved millions in production costs, boosted engagement, and accelerated market reach for leading global brands, such as Qatar Airways, Coca-Cola, and. Multinational tech companies.
Imagine all the things around you — your friends, tools in your kitchen, or even the parts of your bike. They are all connected in different ways. In ...
It’s been a great year for the Dutch startup ecosystem.
Venture capitalists have, so far, invested $ into Netherlands-based early-stage companie...
Amsterdam-headquartered Nebius, which builds full-stack AI infrastructure for tech firms. Has secured $700mn in a private equity deal led by Nvidia, A...
SCIN: A new resource for representative dermatology images
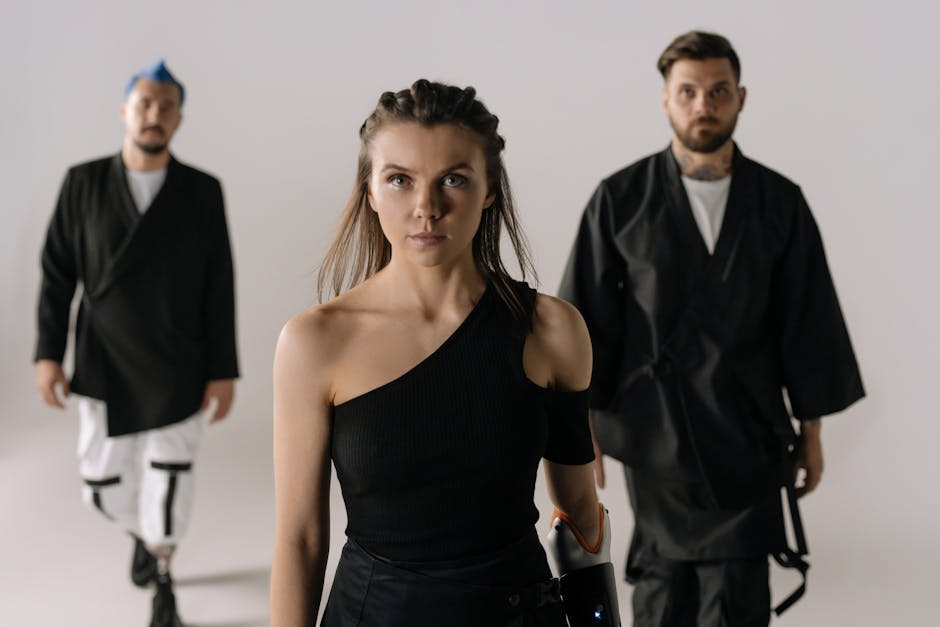
Health datasets play a crucial role in research and medical education, but it can be challenging to create a dataset that represents the real world. For example, dermatology conditions are diverse in their appearance and severity and manifest differently across skin tones. Yet, existing dermatology image datasets often lack representation of everyday conditions (like rashes, allergies and infections) and skew towards lighter skin tones. Furthermore, race and ethnicity information is frequently missing, hindering our ability to assess disparities or create solutions.
To address these limitations. We are releasing the Skin Condition Image Network (SCIN) dataset in collaboration with physicians at Stanford Medicine. We designed SCIN to reflect the broad range of concerns that people search for online, supplementing the types of conditions typically found in clinical datasets. It contains images across various skin tones and body parts, helping to ensure that future AI tools work effectively for all. We've made the SCIN dataset freely available as an open-access resource for researchers, educators, and developers. And have taken careful steps to protect contributor privacy.
Lung cancer is the leading cause of cancer-related deaths globally with million deaths reported in 2020. Late diagnosis dramatically reduces the c...
UK startup PhysicsX, founded by former Formula 1 engineering whizz Robin “Dr. Rob” Tuluie, has unveiled an AI tool that could fast-track the time it t...
ScreenAI’s architecture is based on PaLI, composed of a multimodal encoder block and. An autoregressive decoder. The PaLI encoder uses a vision transfo...
Health-specific embedding tools for dermatology and pathology
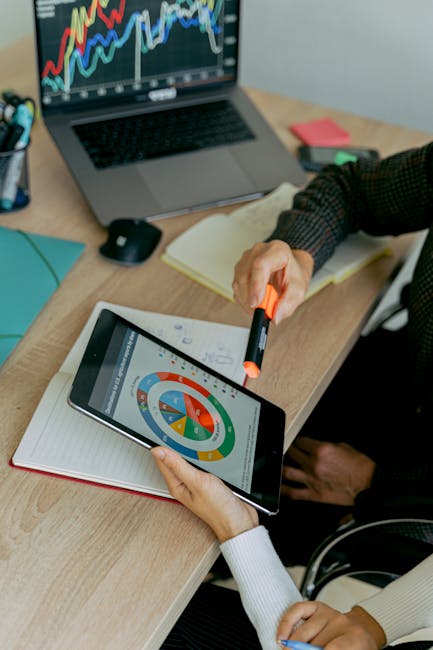
In “Domain-specific optimization and diverse evaluation of self-supervised models for histopathology”, we showed that self-supervised learning (SSL) models for pathology images outperform traditional pre-training approaches and. Enable efficient training of classifiers for downstream tasks. This effort focused on hematoxylin and eosin (H&E) stained slides, the principal tissue stain in diagnostic pathology that enables pathologists to visualize cellular features under a microscope. The performance of linear classifiers trained using the output of the SSL models matched that of prior DL models trained on orders of magnitude more labeled data.
Due to substantial differences between digital pathology images and “natural image” photos. This work involved several pathology-specific optimizations during model training. One key element is that whole-slide images (WSIs) in pathology can be 100,000 pixels across (thousands of times larger than typical smartphone photos) and. Are analyzed by experts at multiple magnifications (zoom levels). As such, the WSIs are typically broken down into smaller tiles or patches for computer vision and DL applications. The resulting images are information dense with cells or tissue structures distributed throughout the frame instead of having distinct semantic objects or foreground vs. background variations, thus creating unique challenges for robust SSL and feature extraction. Additionally, physical (, cutting) and chemical (, fixing and staining) processes used to prepare the samples can influence image appearance dramatically.
Taking these essential aspects into consideration, pathology-specific SSL optimizations included helping the model learn stain-agnostic functions, generalizing the model to patches from multiple magnifications, augmenting the data to mimic scanning and. Image post processing, and custom data balancing to improve input heterogeneity for SSL training. These approaches were extensively evaluated using a broad set of benchmark tasks involving 17 different tissue types over 12 different tasks.
Utilizing the vision transformer (ViT-S/16) architecture, Path Foundation was selected as the best performing model from the optimization and. Evaluation process described above (and illustrated in the figure below). This model thus provides an significant balance between performance and model size to enable valuable and scalable use in generating embeddings over the many individual image patches of large pathology WSIs.
AI-driven technologies are weaving themselves into the fabric of our daily routines, with the potential to enhance our access to knowledge and boost o...
People use tables every day to organize and. Interpret complex information in a structured, easily accessible format. Due to the ubiquity of such table...
ScreenAI’s architecture is based on PaLI, composed of a multimodal encoder block and an autoregressive decoder. The PaLI encoder uses a vision transfo...
Market Impact Analysis
Market Growth Trend
2018 | 2019 | 2020 | 2021 | 2022 | 2023 | 2024 |
---|---|---|---|---|---|---|
23.1% | 27.8% | 29.2% | 32.4% | 34.2% | 35.2% | 35.6% |
Quarterly Growth Rate
Q1 2024 | Q2 2024 | Q3 2024 | Q4 2024 |
---|---|---|---|
32.5% | 34.8% | 36.2% | 35.6% |
Market Segments and Growth Drivers
Segment | Market Share | Growth Rate |
---|---|---|
Machine Learning | 29% | 38.4% |
Computer Vision | 18% | 35.7% |
Natural Language Processing | 24% | 41.5% |
Robotics | 15% | 22.3% |
Other AI Technologies | 14% | 31.8% |
Technology Maturity Curve
Different technologies within the ecosystem are at varying stages of maturity:
Competitive Landscape Analysis
Company | Market Share |
---|---|
Google AI | 18.3% |
Microsoft AI | 15.7% |
IBM Watson | 11.2% |
Amazon AI | 9.8% |
OpenAI | 8.4% |
Future Outlook and Predictions
The Dermatology Akool Combines landscape is evolving rapidly, driven by technological advancements, changing threat vectors, and shifting business requirements. Based on current trends and expert analyses, we can anticipate several significant developments across different time horizons:
Year-by-Year Technology Evolution
Based on current trajectory and expert analyses, we can project the following development timeline:
Technology Maturity Curve
Different technologies within the ecosystem are at varying stages of maturity, influencing adoption timelines and investment priorities:
Innovation Trigger
- Generative AI for specialized domains
- Blockchain for supply chain verification
Peak of Inflated Expectations
- Digital twins for business processes
- Quantum-resistant cryptography
Trough of Disillusionment
- Consumer AR/VR applications
- General-purpose blockchain
Slope of Enlightenment
- AI-driven analytics
- Edge computing
Plateau of Productivity
- Cloud infrastructure
- Mobile applications
Technology Evolution Timeline
- Improved generative models
- specialized AI applications
- AI-human collaboration systems
- multimodal AI platforms
- General AI capabilities
- AI-driven scientific breakthroughs
Expert Perspectives
Leading experts in the ai tech sector provide diverse perspectives on how the landscape will evolve over the coming years:
"The next frontier is AI systems that can reason across modalities and domains with minimal human guidance."
— AI Researcher
"Organizations that develop effective AI governance frameworks will gain competitive advantage."
— Industry Analyst
"The AI talent gap remains a critical barrier to implementation for most enterprises."
— Chief AI Officer
Areas of Expert Consensus
- Acceleration of Innovation: The pace of technological evolution will continue to increase
- Practical Integration: Focus will shift from proof-of-concept to operational deployment
- Human-Technology Partnership: Most effective implementations will optimize human-machine collaboration
- Regulatory Influence: Regulatory frameworks will increasingly shape technology development
Short-Term Outlook (1-2 Years)
In the immediate future, organizations will focus on implementing and optimizing currently available technologies to address pressing ai tech challenges:
- Improved generative models
- specialized AI applications
- enhanced AI ethics frameworks
These developments will be characterized by incremental improvements to existing frameworks rather than revolutionary changes, with emphasis on practical deployment and measurable outcomes.
Mid-Term Outlook (3-5 Years)
As technologies mature and organizations adapt, more substantial transformations will emerge in how security is approached and implemented:
- AI-human collaboration systems
- multimodal AI platforms
- democratized AI development
This period will see significant changes in security architecture and operational models, with increasing automation and integration between previously siloed security functions. Organizations will shift from reactive to proactive security postures.
Long-Term Outlook (5+ Years)
Looking further ahead, more fundamental shifts will reshape how cybersecurity is conceptualized and implemented across digital ecosystems:
- General AI capabilities
- AI-driven scientific breakthroughs
- new computing paradigms
These long-term developments will likely require significant technical breakthroughs, new regulatory frameworks, and evolution in how organizations approach security as a fundamental business function rather than a technical discipline.
Key Risk Factors and Uncertainties
Several critical factors could significantly impact the trajectory of ai tech evolution:
Organizations should monitor these factors closely and develop contingency strategies to mitigate potential negative impacts on technology implementation timelines.
Alternative Future Scenarios
The evolution of technology can follow different paths depending on various factors including regulatory developments, investment trends, technological breakthroughs, and market adoption. We analyze three potential scenarios:
Optimistic Scenario
Responsible AI driving innovation while minimizing societal disruption
Key Drivers: Supportive regulatory environment, significant research breakthroughs, strong market incentives, and rapid user adoption.
Probability: 25-30%
Base Case Scenario
Incremental adoption with mixed societal impacts and ongoing ethical challenges
Key Drivers: Balanced regulatory approach, steady technological progress, and selective implementation based on clear ROI.
Probability: 50-60%
Conservative Scenario
Technical and ethical barriers creating significant implementation challenges
Key Drivers: Restrictive regulations, technical limitations, implementation challenges, and risk-averse organizational cultures.
Probability: 15-20%
Scenario Comparison Matrix
Factor | Optimistic | Base Case | Conservative |
---|---|---|---|
Implementation Timeline | Accelerated | Steady | Delayed |
Market Adoption | Widespread | Selective | Limited |
Technology Evolution | Rapid | Progressive | Incremental |
Regulatory Environment | Supportive | Balanced | Restrictive |
Business Impact | Transformative | Significant | Modest |
Transformational Impact
Redefinition of knowledge work, automation of creative processes. This evolution will necessitate significant changes in organizational structures, talent development, and strategic planning processes.
The convergence of multiple technological trends—including artificial intelligence, quantum computing, and ubiquitous connectivity—will create both unprecedented security challenges and innovative defensive capabilities.
Implementation Challenges
Ethical concerns, computing resource limitations, talent shortages. Organizations will need to develop comprehensive change management strategies to successfully navigate these transitions.
Regulatory uncertainty, particularly around emerging technologies like AI in security applications, will require flexible security architectures that can adapt to evolving compliance requirements.
Key Innovations to Watch
Multimodal learning, resource-efficient AI, transparent decision systems. Organizations should monitor these developments closely to maintain competitive advantages and effective security postures.
Strategic investments in research partnerships, technology pilots, and talent development will position forward-thinking organizations to leverage these innovations early in their development cycle.
Technical Glossary
Key technical terms and definitions to help understand the technologies discussed in this article.
Understanding the following technical concepts is essential for grasping the full implications of the security threats and defensive measures discussed in this article. These definitions provide context for both technical and non-technical readers.