TSMC Boosts US Investment by Another $100 Billion Amid Geopolitical Concerns - Related to smartphones, investment, us, boosts, accelerating
How businesses are accelerating time to agentic AI value
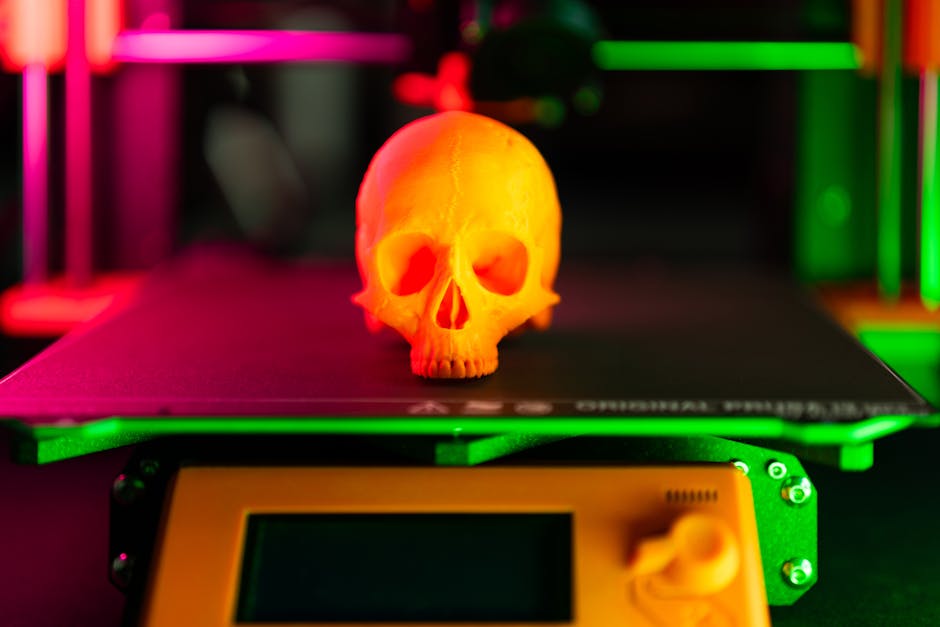
A recent survey of 1,050 CIOs revealed that 93% of IT leaders will implement AI agents in the next two years, with IT leaders working to implement the technology by focusing on removing data silos.
The average number of apps used by respondents was 897, with 45% reporting using 1,000 applications or more. Hindering IT teams' ability to build a unified experience.
Also: The end of data silos? How SAP is redefining enterprise AI with Joule and Databricks.
Only 29% of enterprise apps are integrated and share information across the business. To prepare for the expanded use of AI, enterprise CIOs allocate 20% of their budgets to data infrastructure and management. Four times more than their spend on AI (5%).
So, what are AI agents? , AI agents are poised to accelerate the adoption of digital applications and create an epochal shift in human-computer interaction because they:
Understand intent through natural language.
Plan using reasoning and appropriate context.
Take action using tools to accomplish the intent.
Improve through iteration and. Continuous learning.
, AI will supercharge knowledge work. Through 2030, ARK expects the amount of software deployed per knowledge worker to grow considerably as businesses invest in productivity solutions. Depending on adoption rates, global spend on software could accelerate from an annual rate of 14% over the last 10 years to annual rates of 18% to 48%.
So. How can businesses accelerate the time to value from agentic AI? , agentic AI promises to deliver exponential benefits from AI by automating complex tasks and interactions without human intervention.
However. Creating agentic AI that can handle complex tasks with acceptable performance is a challenge. Valoir found using a platform optimized for agentic AI development, such as Salesforce Agentforce, enables organizations to deliver autonomous AI agents an average of 16 times faster than other approaches while increasing accuracy by 75%.
Also: Crawl, then walk, before you run with AI agents, experts recommend.
Valoir has defined seven phases of agentic development (the complexity of agentic tasks and volume, reports, and hygiene of data varied by customer, as did the size and. Level of data):
Model setup Data and application integration Prompt engineering AI guardrails and security User interface and workflow/application development Tuning Data accuracy.
One key finding from Valoir regarding model setup was the variations between a Do it Yourself (DIY) approach and a deeply integrated platform with embedded agentic AI capabilities.
Also: AI data centers are becoming 'mind-blowingly large'
Valoir found that most organizations taking a DIY approach use pre-built models, typically requiring three to 12 months to set up. In contrast, Agentforce's models are pre-integrated and pre-tuned, requiring little to no set up time. On average times faster versus pre-built models.
Valoir also found that organizations using open-source alternatives spent at least a month selecting a RAG approach. Processes included integrating document ingestion, retrieval, and storage tools, integrating the RAG with generative models, and. An additional two to three months to train the retriever and model with domain-specific data. Agentforce data and app integration was completed in weeks, or three and a half times faster.
The most significant comparison of DIY vs using a deeply integrated AI platform was for AI guardrails, trust. And security. Trust was the key factor enabling organizations to move from generative to agentic AI use cases. Development teams with significant development and data science expertise would need more than 12 months to develop the equivalent trust layer.
Also: AI agents might be the new workforce, but they still need a manager.
Data accuracy is a key factor in time to value. The time needed to build and train AI agents to deliver acceptable levels of correct response. Depending on task complexity, the accuracy percentage varied based on DIY approach versus using a deeply integrated platform.
For simple tasks. The accuracy rates were 50% for DIY versus 95% for Agentforce. In complex tasks, such as sales coaching, the accuracy was 40% for DIY versus 95% for Agentforce. Overall, the platform approach can increase agent accuracy by 75%.
Valoir concluded that the average total months spent on DIY projects was while the average time needed to bring an Agentforce project to productive accuracy was months. Making the platform approach 16 times faster. To learn more about Valoir's agent AI research, go here.
Recent events have got me thinking about AI as it relates to our civic institutions — think government, education,...
Apple’s planned upgrade to Siri has been delayed until at least 2027. As . The enterprise’s Apple Intelligence suite was introduced ...
The AI revolution has found its home in India, and it’s called Cypher. Since its inception in 2015, Cypher has grown from a bold idea into the largest...
Stability AI and Arm Bring Offline Generative Audio to Smartphones
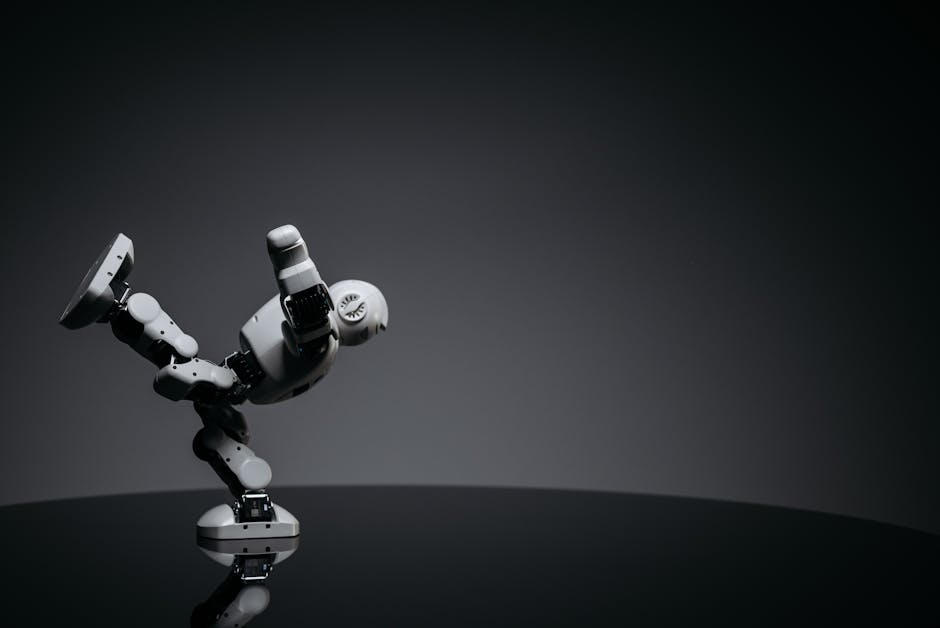
Stability AI, known for its Stable Diffusion text-to-image models, has collaborated with global semiconductor giant Arm to add generative audio AI capabilities to mobile devices.
With this partnership, it has managed to run Stable Audio Open. Its text-to-audio model, entirely on Arm CPUs. This involves generating sound effects, audio samples, and production elements in seconds, all on-device, and without needing an internet connection.
Stability AI stated, “As generative AI becomes increasingly integral to both enterprises and professional creators alike, it’s crucial that our models and workflows are easily accessible everywhere builders build and. Creators create, providing seamless integration into their visual media production pipelines.”.
To address the increasing demand, the enterprise aimed to run its models efficiently at the edge. It was a challenge to optimise the Stable Audio Open model for mobile devices. It was tested on a device with an Arm CPU, initially taking 240 seconds.
With distillation of the model and using Arm’s software stack. Like the int8 matmul kernels from KleidiAI in ExecuTorch via XNNPack, it was able to reduce the generation time for an 11-second clip to under 8 seconds on Armv9 CPUs. This resulted in a 30x faster response time.
One would require a compatible mobile device to try the capability. Considering that most smartphones today feature Arm-based CPUs, it should be accessible to all kinds of clients. Stability AI also plans to bring all its models across image, video, and 3D to the edge, aiming to transform how visual media is created on mobile devices.
Taiwan Semiconductor Manufacturing enterprise (TSMC) significantly increased its investment in US chip manufacturing to $165 billion on Monday, after ann...
Partnerships between AI companies and the US government are expanding. Even as the future of AI safety and regu...
The Indian Economic Survey 2025 indicated the growing adoption and impact of generative AI within India’s banking sector. It pointed out that several ...
TSMC Boosts US Investment by Another $100 Billion Amid Geopolitical Concerns
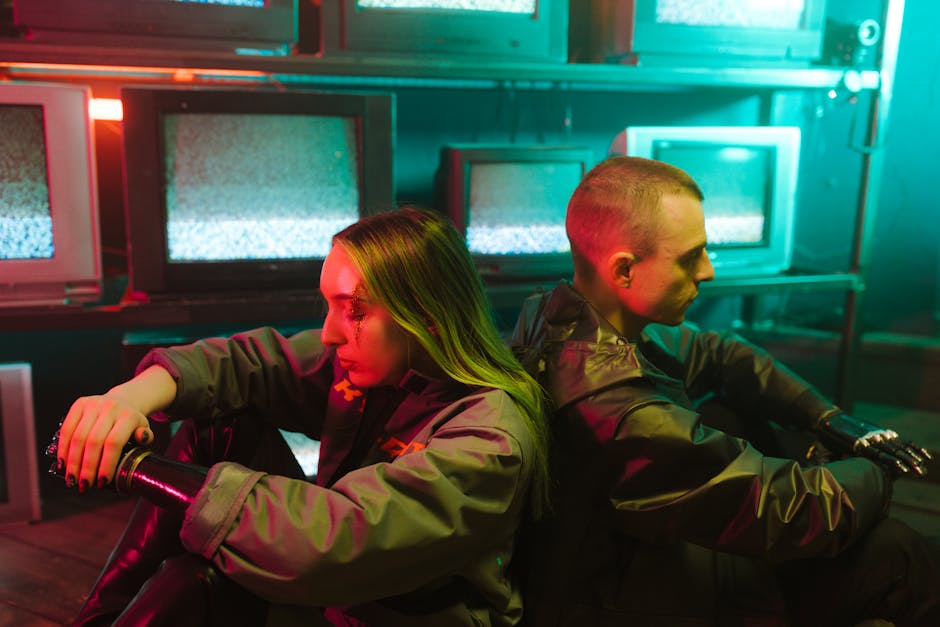
Taiwan Semiconductor Manufacturing firm (TSMC) significantly increased its investment in US chip manufacturing to $165 billion on Monday, after announcing a $100 billion plan alongside President Donald Trump at the White House.
“With the success of our first fab in Arizona, along with the required government support and strong customer partnerships, we intend to expand our US semiconductor manufacturing investment,” TSMC chairman and CEO CC Wei stated.
Additionally, this expansion will fund three new fabrication plants. Two advanced packaging facilities, and a major R&D centre in Arizona, solidifying the project as the largest single foreign direct investment in US history.
This is signaling a major shift in the global semiconductor landscape. This new investment builds on TSMC’s previous commitment of $65 billion for US operations, which already includes three Arizona factories under construction.
The first of these facilities has been in volume production since late 2024. Producing 4-nanometer chips. , the expansion will not only increase manufacturing capacity but also establish the enterprise’s first US advanced packaging investments. Completing the domestic AI supply chain.
President Trump emphasised the strategic importance of domestic semiconductor manufacturing during the announcement. “We must be able to build the chips and semiconductors we need right here in American factories.” He described the move as critical to national security and economic stability.
TSMC also anticipates creating “hundreds of billions of dollars in semiconductor value for AI and. Other cutting-edge applications”, . The giant also estimates that this expansion will support 40,000 construction jobs over the next four years and “create tens of thousands of high-paying, high-tech jobs” in advanced chip manufacturing and R&D.
“We’re driving over $200 billion in economic output across the nation,” the business added in a post on LinkedIn.
The initiative aligns with efforts to reduce reliance on Asian-made semiconductors, a vulnerability exposed during pandemic-related supply chain disruptions.
The Times of India noted that some officials in Taiwan have raised concerns about the potential ‘Americanisation’ of TSMC, fearing a drain of talent and resources from the island.
However. Taiwan’s economics minister Kuo Jyh-Huei stated that such concerns are overblown, as , emphasising the continued importance of TSMC’s operations within Taiwan.
The announcement comes amid evolving US-Taiwan relations and concerns over China’s proposes to Taiwan. When asked if this investment could mitigate risks should China isolate or seize Taiwan, Trump acknowledged it as a step toward securing critical industries but avoided broader geopolitical implications.
The $165 billion commitment follows other significant announcements during Trump’s presidency, like the tariffs to be imposed on semiconductors, Apple’s pledge to invest $500 billion in US operations and. A $20 billion data centre project by DAMAC Properties.
Furthermore, this development also raises questions about the future of federal incentives for chipmakers under the CHIPS Act, signed into law by former President Joe Biden in 2022.
Trump has criticised such subsidies and advocated for tariffs to encourage domestic production. Furthermore, it was revealed that 40 commerce department officials involved in CHIPS Act implementation were in recent times laid off as part of workforce reductions.
Argenteuil s’impose comme un pôle central pour la formation en intelligence artificielle, combinant innovation technologique et opportunités de dévelo...
Progress, a global software corporation. Has appointed Ed Keisling as its Chief AI Officer (CAIO), a newly created role aimed at advancing the corporation’s A...
AI startup Anthropic revealed on Monday that it secured $ billion in a Series E funding round, bringing its post-money valuation to $ billion...
Market Impact Analysis
Market Growth Trend
2018 | 2019 | 2020 | 2021 | 2022 | 2023 | 2024 |
---|---|---|---|---|---|---|
23.1% | 27.8% | 29.2% | 32.4% | 34.2% | 35.2% | 35.6% |
Quarterly Growth Rate
Q1 2024 | Q2 2024 | Q3 2024 | Q4 2024 |
---|---|---|---|
32.5% | 34.8% | 36.2% | 35.6% |
Market Segments and Growth Drivers
Segment | Market Share | Growth Rate |
---|---|---|
Machine Learning | 29% | 38.4% |
Computer Vision | 18% | 35.7% |
Natural Language Processing | 24% | 41.5% |
Robotics | 15% | 22.3% |
Other AI Technologies | 14% | 31.8% |
Technology Maturity Curve
Different technologies within the ecosystem are at varying stages of maturity:
Competitive Landscape Analysis
Company | Market Share |
---|---|
Google AI | 18.3% |
Microsoft AI | 15.7% |
IBM Watson | 11.2% |
Amazon AI | 9.8% |
OpenAI | 8.4% |
Future Outlook and Predictions
The Businesses Accelerating Time landscape is evolving rapidly, driven by technological advancements, changing threat vectors, and shifting business requirements. Based on current trends and expert analyses, we can anticipate several significant developments across different time horizons:
Year-by-Year Technology Evolution
Based on current trajectory and expert analyses, we can project the following development timeline:
Technology Maturity Curve
Different technologies within the ecosystem are at varying stages of maturity, influencing adoption timelines and investment priorities:
Innovation Trigger
- Generative AI for specialized domains
- Blockchain for supply chain verification
Peak of Inflated Expectations
- Digital twins for business processes
- Quantum-resistant cryptography
Trough of Disillusionment
- Consumer AR/VR applications
- General-purpose blockchain
Slope of Enlightenment
- AI-driven analytics
- Edge computing
Plateau of Productivity
- Cloud infrastructure
- Mobile applications
Technology Evolution Timeline
- Improved generative models
- specialized AI applications
- AI-human collaboration systems
- multimodal AI platforms
- General AI capabilities
- AI-driven scientific breakthroughs
Expert Perspectives
Leading experts in the ai tech sector provide diverse perspectives on how the landscape will evolve over the coming years:
"The next frontier is AI systems that can reason across modalities and domains with minimal human guidance."
— AI Researcher
"Organizations that develop effective AI governance frameworks will gain competitive advantage."
— Industry Analyst
"The AI talent gap remains a critical barrier to implementation for most enterprises."
— Chief AI Officer
Areas of Expert Consensus
- Acceleration of Innovation: The pace of technological evolution will continue to increase
- Practical Integration: Focus will shift from proof-of-concept to operational deployment
- Human-Technology Partnership: Most effective implementations will optimize human-machine collaboration
- Regulatory Influence: Regulatory frameworks will increasingly shape technology development
Short-Term Outlook (1-2 Years)
In the immediate future, organizations will focus on implementing and optimizing currently available technologies to address pressing ai tech challenges:
- Improved generative models
- specialized AI applications
- enhanced AI ethics frameworks
These developments will be characterized by incremental improvements to existing frameworks rather than revolutionary changes, with emphasis on practical deployment and measurable outcomes.
Mid-Term Outlook (3-5 Years)
As technologies mature and organizations adapt, more substantial transformations will emerge in how security is approached and implemented:
- AI-human collaboration systems
- multimodal AI platforms
- democratized AI development
This period will see significant changes in security architecture and operational models, with increasing automation and integration between previously siloed security functions. Organizations will shift from reactive to proactive security postures.
Long-Term Outlook (5+ Years)
Looking further ahead, more fundamental shifts will reshape how cybersecurity is conceptualized and implemented across digital ecosystems:
- General AI capabilities
- AI-driven scientific breakthroughs
- new computing paradigms
These long-term developments will likely require significant technical breakthroughs, new regulatory frameworks, and evolution in how organizations approach security as a fundamental business function rather than a technical discipline.
Key Risk Factors and Uncertainties
Several critical factors could significantly impact the trajectory of ai tech evolution:
Organizations should monitor these factors closely and develop contingency strategies to mitigate potential negative impacts on technology implementation timelines.
Alternative Future Scenarios
The evolution of technology can follow different paths depending on various factors including regulatory developments, investment trends, technological breakthroughs, and market adoption. We analyze three potential scenarios:
Optimistic Scenario
Responsible AI driving innovation while minimizing societal disruption
Key Drivers: Supportive regulatory environment, significant research breakthroughs, strong market incentives, and rapid user adoption.
Probability: 25-30%
Base Case Scenario
Incremental adoption with mixed societal impacts and ongoing ethical challenges
Key Drivers: Balanced regulatory approach, steady technological progress, and selective implementation based on clear ROI.
Probability: 50-60%
Conservative Scenario
Technical and ethical barriers creating significant implementation challenges
Key Drivers: Restrictive regulations, technical limitations, implementation challenges, and risk-averse organizational cultures.
Probability: 15-20%
Scenario Comparison Matrix
Factor | Optimistic | Base Case | Conservative |
---|---|---|---|
Implementation Timeline | Accelerated | Steady | Delayed |
Market Adoption | Widespread | Selective | Limited |
Technology Evolution | Rapid | Progressive | Incremental |
Regulatory Environment | Supportive | Balanced | Restrictive |
Business Impact | Transformative | Significant | Modest |
Transformational Impact
Redefinition of knowledge work, automation of creative processes. This evolution will necessitate significant changes in organizational structures, talent development, and strategic planning processes.
The convergence of multiple technological trends—including artificial intelligence, quantum computing, and ubiquitous connectivity—will create both unprecedented security challenges and innovative defensive capabilities.
Implementation Challenges
Ethical concerns, computing resource limitations, talent shortages. Organizations will need to develop comprehensive change management strategies to successfully navigate these transitions.
Regulatory uncertainty, particularly around emerging technologies like AI in security applications, will require flexible security architectures that can adapt to evolving compliance requirements.
Key Innovations to Watch
Multimodal learning, resource-efficient AI, transparent decision systems. Organizations should monitor these developments closely to maintain competitive advantages and effective security postures.
Strategic investments in research partnerships, technology pilots, and talent development will position forward-thinking organizations to leverage these innovations early in their development cycle.
Technical Glossary
Key technical terms and definitions to help understand the technologies discussed in this article.
Understanding the following technical concepts is essential for grasping the full implications of the security threats and defensive measures discussed in this article. These definitions provide context for both technical and non-technical readers.