2025 has already brought us the most performant AI ever: What can we do with these supercharged capabilities (and what’s next)? - Related to assist, 2025, we, valuation, what’s
2025 has already brought us the most performant AI ever: What can we do with these supercharged capabilities (and what’s next)?
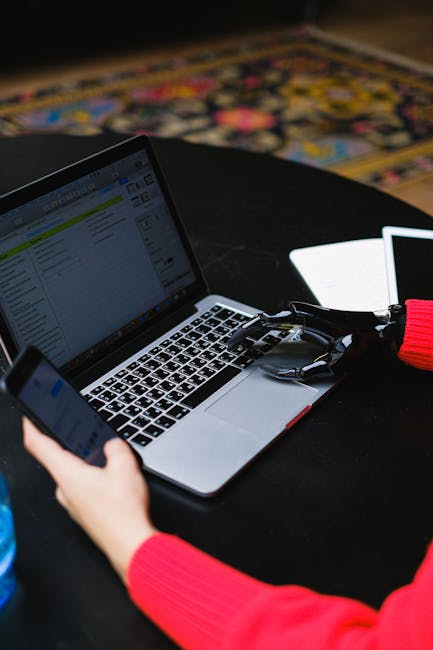
The latest AI large language model (LLM) releases, such as Claude from Anthropic and Grok 3 from xAI. Are often performing at PhD levels — at least . This accomplishment marks the next step toward what former Google CEO Eric Schmidt envisions: A world where everyone has access to “a great polymath,” an AI capable of drawing on vast bodies of knowledge to solve complex problems across disciplines.
Wharton Business School Professor Ethan Mollick noted on his One Useful Thing blog that these latest models were trained using significantly more computing power than GPT-4 at its launch two years ago. With Grok 3 trained on up to 10 times as much compute. He added that this would make Grok 3 the first “gen 3” AI model, emphasizing that “this new generation of AIs is smarter, and the jump in capabilities is striking.”.
For example. Claude exhibits emergent capabilities, such as anticipating user needs and the ability to consider novel angles in problem-solving. , it is the first hybrid reasoning model, combining a traditional LLM for fast responses with advanced reasoning capabilities for solving complex problems.
Mollick attributed these advances to two converging trends: The rapid expansion of compute power for training LLMs, and. AI’s increasing ability to tackle complex problem-solving (often described as reasoning or thinking). He concluded that these two trends are “supercharging AI abilities.”.
What can we do with this supercharged AI?
In a significant step. OpenAI launched its “deep research” AI agent at the beginning of February. In his review on Platformer, Casey Newton commented that deep research appeared “impressively competent.” Newton noted that deep research and similar tools could significantly accelerate research, analysis and other forms of knowledge work. Though their reliability in complex domains is still an open question.
Based on a variant of the still unreleased o3 reasoning model, deep research can engage in extended reasoning over long durations. It does this using chain-of-thought (COT) reasoning, breaking down complex tasks into multiple logical steps, just as a human researcher might refine their approach. It can also search the web, enabling it to access more up-to-date information than what is in the model’s training data.
Timothy Lee wrote in Understanding AI about several tests experts did of deep research. Noting that “its performance demonstrates the impressive capabilities of the underlying o3 model.” One test asked for directions on how to build a hydrogen electrolysis plant. Commenting on the quality of the output, a mechanical engineer “estimated that it would take an experienced professional a week to create something as good as the 4,000-word analysis OpenAI generated in four minutes.”.
Google DeepMind also lately released “AI co-scientist,” a multi-agent AI system built on its Gemini LLM. It is designed to help scientists create novel hypotheses and research plans. Already, Imperial College London has proved the value of this tool. . Penadés, his team spent years unraveling why certain superbugs resist antibiotics. AI replicated their findings in just 48 hours. While the AI dramatically accelerated hypothesis generation, human scientists were still needed to confirm the findings. Nevertheless, Penadés stated the new AI application “has the potential to supercharge science.”.
What would it mean to supercharge science?
Last October, Anthropic CEO Dario Amodei wrote in his “Machines of Loving Grace” blog that he expected “powerful AI” — his term for what most call artificial general intelligence (AGI) — would lead to “the next 50 to 100 years of biological [research] progress in 5 to 10 years.” Four months ago. The idea of compressing up to a century of scientific progress into a single decade seemed extremely optimistic. With the recent advances in AI models now including Anthropic Claude , OpenAI deep research and Google AI co-scientist, what Amodei referred to as a near-term “radical transformation” is starting to look much more plausible.
However, while AI may fast-track scientific discovery, biology, at least. Is still bound by real-world constraints — experimental validation, regulatory approval and clinical trials. The question is no longer whether AI will transform science (as it certainly will), but rather how quickly its full impact will be realized.
In a February 9 blog post, OpenAI CEO Sam Altman claimed that “systems that start to point to AGI are coming into view.” He described AGI as “a system that can tackle increasingly complex problems, at human level, in many fields.”.
Altman believes achieving this milestone could unlock a near-utopian future in which the “economic growth in front of us looks astonishing, and. We can now imagine a world where we cure all diseases, have much more time to enjoy with our families and can fully realize our creative potential.”.
These advances of AI are hugely significant and portend a much different future in a brief period of time. Yet, AI’s meteoric rise has not been without stumbles. Consider the recent downfall of the Humane AI Pin — a device hyped as a smartphone replacement after a buzzworthy TED Talk. Barely a year later, the corporation collapsed, and its remnants were sold off for a fraction of their once-lofty valuation.
Real-world AI applications often face significant obstacles for many reasons. From lack of relevant expertise to infrastructure limitations. This has certainly been the experience of Sensei Ag, a startup backed by one of the world’s wealthiest investors. The organization set out to apply AI to agriculture by breeding improved crop varieties and using robots for harvesting but has met major hurdles. , the startup has faced many setbacks, from technical challenges to unexpected logistical difficulties, highlighting the gap between AI’s potential and its practical implementation.
As we look to the near future. Science is on the cusp of a new golden age of discovery, with AI becoming an increasingly capable partner in research. Deep-learning algorithms working in tandem with human curiosity could unravel complex problems at record speed as AI systems sift vast troves of data, spot patterns invisible to humans and suggest cross-disciplinary hypotheses.
Already, scientists are using AI to compress research timelines — predicting protein structures, scanning literature and reducing years of work to months or even days — unlocking opportunities across fields from climate science to medicine.
Yet. As the potential for radical transformation becomes clearer, so too do the looming risks of disruption and instability. Altman himself acknowledged in his blog that “the balance of power between capital and labor could easily get messed up,” a subtle but significant warning that AI’s economic impact could be destabilizing.
This concern is already materializing. As demonstrated in Hong Kong, as the city in recent times cut 10,000 civil service jobs while simultaneously ramping up AI investments. If such trends continue and become more expansive, we could see widespread workforce upheaval, heightening social unrest and placing intense pressure on institutions and governments worldwide.
AI’s growing capabilities in scientific discovery. Reasoning and decision-making mark a profound shift that presents both extraordinary promise and formidable challenges. While the path forward may be marked by economic disruptions and institutional strains, history has shown that societies can adapt to technological revolutions, albeit not always easily or without consequence.
To navigate this transformation successfully, societies must invest in governance. Education and workforce adaptation to ensure that AI’s benefits are equitably distributed. Even as AI regulation faces political resistance, scientists, policymakers and business leaders must collaborate to build ethical frameworks, enforce transparency standards and. Craft policies that mitigate risks while amplifying AI’s transformative impact. If we rise to this challenge with foresight and responsibility, people and AI can tackle the world’s greatest challenges, ushering in a new age with breakthroughs that once seemed impossible.
The team behind the Opera web browser has unveiled a new AI agent called Browser Operator. Capable of performing browsing tasks for consumers. This new a...
SpaceTech startup TakeMe2Space has secured ₹ crore in a pre-seed funding round led by Seafund, with participation from Artha Venture Fund, Blume Ve...
Hyderabad is rapidly establishing itself as a major life sciences hub. Rivaling Bengaluru, thanks to a robust state-supported business ecosystem attra...
Anthropic raises $3.5 billion, reaching $61.5 billion valuation as AI investment frenzy continues
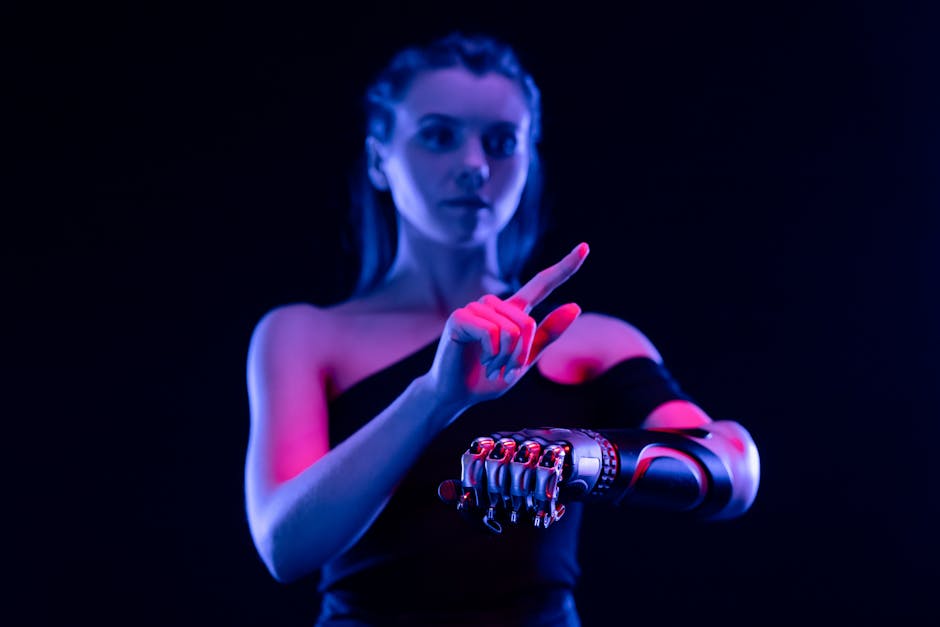
Anthropic closed a $ billion series E funding round, valuing the AI firm at $ billion post-money, the firm introduced today. Lightspeed Venture Partners led the round with a $1 billion contribution, cementing Anthropic’s status as one of the world’s most valuable private companies and demonstrating investors’ unwavering appetite for leading AI developers despite already astronomical valuations.
The financing attracted participation from an impressive roster of investors including Salesforce Ventures, Cisco Investments, Fidelity Management & Research Company, General Catalyst, D1 Capital Partners, Jane Street, Menlo Ventures and. Bessemer Venture Partners.
“With this investment, Anthropic will advance its development of next-generation AI systems, expand its compute capacity, deepen its research in mechanistic interpretability and alignment, and accelerate its international expansion,” the firm noted in its announcement.
Revenue skyrockets 1,000% year-over-year as enterprise clients flock to Claude.
Anthropic’s dramatic valuation reflects its exceptional commercial momentum. The organization’s annualized revenue reached $1 billion by December 2024, representing a tenfold increase year-over-year, ’s finances. That growth has accelerated further, with revenue reportedly increasing by 30% in just the first two months of 2025, .
Founded in 2021 by former OpenAI researchers including siblings Dario and Daniela Amodei. Anthropic has positioned itself as a more research-focused and safety-oriented alternative to its chief rival. The enterprise’s Claude chatbot has gained significant market share since its public launch in March 2023, particularly in enterprise applications.
Krishna Rao, Anthropic’s CFO, mentioned in a statement that the investment “fuels our development of more intelligent and capable AI systems that expand what humans can achieve,” adding that “continued advances in scaling across all aspects of model training are powering breakthroughs in intelligence and. Expertise.”.
AI valuation metrics evolve: 58x revenue multiple displays market maturation.
In relation to this, the funding round comes at a pivotal moment in AI startup valuations. While Anthropic’s latest round values the organization at roughly 58 times its annualized revenue, down from approximately 150 times a year ago, this still represents an extraordinary premium compared to traditional software companies, which typically trade at 10 to 20 times revenue.
What we’re witnessing with AI valuations isn’t merely another tech bubble. But rather a fundamental recalibration of how growth is valued in the marketplace. Traditional valuation models simply weren’t designed for companies experiencing growth curves this steep. When a firm like Anthropic can increase revenue tenfold in a single year — something that would take a typical software firm a decade to achieve — investors are essentially buying future market dominance rather than current financials.
This phenomenon creates a fascinating paradox: As AI companies grow larger, their revenue multiples are contracting. Yet they remain astronomically high compared to any other sector. This hints at investors aren’t simply drunk on AI hype but are making calculated bets that these firms will eventually grow into their valuations by capturing the enormous productivity gains that advanced AI promises to unleash across every sector of the economy.
Anthropic’s valuation surge contrasts with conventional tech wisdom that multiples should decrease as companies mature. The continued investor enthusiasm underscores beliefs that AI represents a fundamental technological shift rather than just another software category.
Amazon and. Google back Anthropic’s B2B strategy with $11 billion combined investment.
Moving to another aspect, the funding comes after Anthropic secured major strategic investments from tech giants. Amazon has invested a total of $8 billion in the startup, making AWS Anthropic’s “primary cloud and training partner” for deploying its largest AI models. Google has committed more than $3 billion to the corporation.
Unlike OpenAI, which has increasingly focused on developing consumer applications. Anthropic has positioned itself primarily as a B2B technology provider enabling other companies to build with its models. This approach has attracted clients ranging from startups like Cursor and Replit to global corporations including Zoom, Snowflake and. Pfizer.
“Replit integrated Claude into ‘Agent’ to turn natural language into code, driving 10X revenue growth,” Anthropic noted in its announcement. Other notable implementations include Thomson Reuters’ tax platform CoCounsel, which uses Claude to assist tax professionals, and Novo Nordisk, which has used Claude to reduce clinical study study writing “from 12 weeks to 10 minutes.”.
Anthropic also highlighted that Claude now helps power Amazon’s Alexa+, “bringing advanced AI capabilities to millions of households and Prime members.”.
SoftBank, OpenAI and DeepSeek intensify global AI competition with billion-dollar moves.
SoftBank is finalizing a massive $40 billion investment in OpenAI at a $260 billion pre-money valuation. Highlighting the escalating stakes in the AI race.
Meanwhile, Chinese AI firm DeepSeek has disrupted the market with its R1 model, which reportedly achieved similar capabilities to competitors’ systems but at a fraction of the cost. This challenge has prompted established players to accelerate their development timelines.
Anthropic lately responded with the launch of Claude Sonnet and Claude Code. With Sonnet specifically optimized for programming tasks. The firm proposes these products have “set a new high-water mark in coding abilities” and plans “to make further progress in the coming months.”.
The trillion-dollar AI market: Investors bet big despite profitability questions.
The massive funding rounds flowing into leading AI companies signal that investors believe the generative AI market could indeed reach the $1 trillion valuation that analysts predict within the next decade.
However. Profitability remains a distant goal. Like its competitors, Anthropic continues to operate at a significant loss as it invests heavily in research, model development, and compute infrastructure. The long path to profitability hasn’t deterred investors, who view these companies as platforms that could fundamentally transform how humans interact with technology.
As the AI arms race intensifies. The key question remains whether these multi-billion dollar valuations will eventually be justified by sustainable business models or if the current investment environment represents an AI bubble. For now, Anthropic’s successful fundraise indicates investors are firmly betting on the former.
AI startup Anthropic introduced on Monday that it secured $ billion in a Series E funding round, bringing its post-money valuation to $ billion...
Stability AI. Known for its Stable Diffusion text-to-image models, has collaborated with global semiconductor giant Arm to add generative audio AI cap...
Google makes Gemini Code Assist free with 180,000 code completions per month as AI-powered dev race heats up
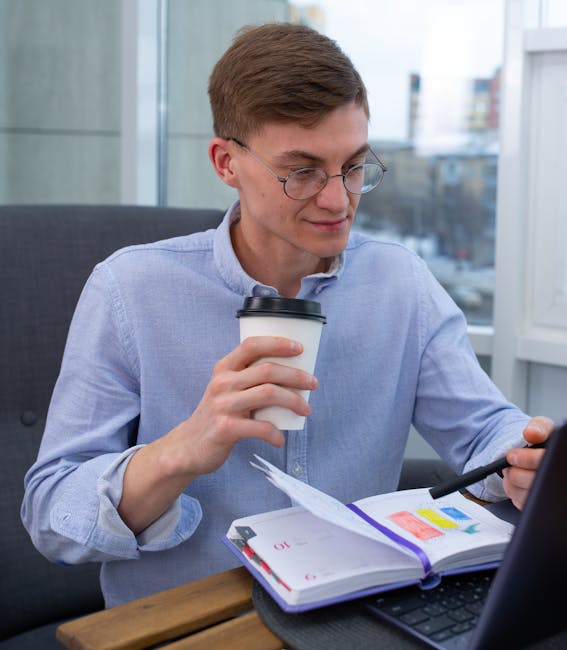
Google has released a free version of its AI-powered coding assistant, Gemini Code Assist, expanding access to advanced coding tools for developers worldwide.
In relation to this, this launch follows the October 2024 debut of Gemini Code Assist Enterprise ($45 per month per user or $19 per month with an annual subscription) and arrives just a day after Anthropic introduced Claude Code, highlighting the growing competition among AI-powered developer tools.
Gemini Code Assist is powered by the Gemini model. Fine-tuned to handle real-world coding scenarios and supporting all programming languages in the public domain.
people can generate up to 180,000 code completions per month — significantly more than other free coding assistants, including popular tool Cursor AI which offers only 2,000 code completions per month on its free tier — while leveraging a 128,000-token context window for working with larger codebases. The assistant integrates with Visual Studio Code, JetBrains IDEs, Firebase, Android Studio and GitHub.
In GitHub, Gemini Code Assist reviews code in both public and private repositories, detecting bugs. Suggesting stylistic improvements and summarizing pull requests.
In the official corporation blog post from Google, Ryan J. Salva, senior director of product management at Google Cloud, emphasized that AI coding tools are becoming essential for developers and should be accessible to everyone. Regardless of their financial resources. He noted that AI not only accelerates coding but also enhances code quality through faster and more efficient reviews.
Building on these developments, this free version builds upon the capabilities of Gemini Code Assist Enterprise, launched in October 2024, replacing Google’s prior AI coding assistant, Duet.
As previously , VentureBeat senior AI reporter Emilia David. The enterprise version offers deeper integrations with Google Cloud services like Firebase, BigQuery and Colab Enterprise.
It provides advanced customization options, including code suggestions based on internal libraries. It also ensures customer data is not used to train Google’s models and allows customers to control and purge their data at any time. Google further offers indemnification for any AI-generated code via the Enterprise Code Assist plan.
The free version of Gemini Code Assist stands out for its higher usage limits compared to other free AI coding tools:
• GitHub Copilot Free offers 2,000 code completions per month — approximately 80 completions per working day — along with 50 chat requests per month. It provides access to both GPT-4o and Claude Sonnet models for powering the backend.
• Amazon Q Developer Free Tier includes code suggestions in IDEs and CLIs, 50 monthly interactions for tasks like debugging and. Adding tests, and 10 uses of AI-driven software development agents per month. The Amazon Q Developer Agent for code transformation allows up to 1,000 lines of submitted code monthly.
• Claude Code (Beta, by Anthropic) integrates directly with developers’ terminals, helping with file edits, bug fixes. Codebase analysis, test execution and Git operations, powered by Claude’s new Sonnet model. While currently in beta as a research preview, Claude Code charges based on token usage, with typical costs ranging from $5 to $10 per developer per day, though intensive use can exceed $100 per hour.
Compared to these offerings. Gemini Code Assist’s 180,000 monthly code completions — equivalent to 6,000 daily requests — far exceeds the limits of both GitHub Copilot Free and Amazon Q Developer. Its availability at no cost, with no credit card required for sign-up, makes it especially attractive to students, hobbyists and. Startups.
Early reactions on Reddit’s r/singularity subreddit highlight both excitement and skepticism. User axseem commented, “I can’t keep up with all these releases anymore?” while Comedian_Then observed, “You see why competition is good? Miraculously they start pushing the technology so hard we can’t keep up with all the models and prices constantly dropping.”.
User bilalazhar72 emphasized the appeal of a free, widely accessible tool, stating, “At the end of the day what matters to most people is that the AI code assist is free and it should be free… In the long run the most cheap and most easy accessible option wins.” However, Bitter-Good-2540 speculated about Google’s strategic motives, suggesting, “It serves Google also, they can train new models with your code lol.” Meanwhile, imDaGoatnocap highlighted its practical benefits, saying, “I guess it serves as a decent free tier option for people who can’t afford Cursor or Windsurf.”.
With global availability and a straightforward sign-up process requiring only a personal Gmail account, Google DeepMind aims to democratize access to AI-powered coding assistance.
As competition in the AI coding space intensifies — with offerings from GitHub, Amazon and now Anthropic, not to mention startups such as Cursor AI, Qodo and. Codeium’s Windsurf — Google’s decision to provide a free version with significantly higher usage limits positions Gemini Code Assist as a compelling choice for developers seeking accessible and powerful coding support.
Wipro GE Healthcare has introduced Versana Premier R3, an advanced AI-powered ultrasound system designed to enhance clinical efficiency, streamline wo...
At the Mobile World Congress (MWC) 2025 held in Barcelona, Jio Platforms Limited (JPL), along with AMD, Cisco, and Nokia, on Monday unveiled that it ...
Market Impact Analysis
Market Growth Trend
2018 | 2019 | 2020 | 2021 | 2022 | 2023 | 2024 |
---|---|---|---|---|---|---|
23.1% | 27.8% | 29.2% | 32.4% | 34.2% | 35.2% | 35.6% |
Quarterly Growth Rate
Q1 2024 | Q2 2024 | Q3 2024 | Q4 2024 |
---|---|---|---|
32.5% | 34.8% | 36.2% | 35.6% |
Market Segments and Growth Drivers
Segment | Market Share | Growth Rate |
---|---|---|
Machine Learning | 29% | 38.4% |
Computer Vision | 18% | 35.7% |
Natural Language Processing | 24% | 41.5% |
Robotics | 15% | 22.3% |
Other AI Technologies | 14% | 31.8% |
Technology Maturity Curve
Different technologies within the ecosystem are at varying stages of maturity:
Competitive Landscape Analysis
Company | Market Share |
---|---|
Google AI | 18.3% |
Microsoft AI | 15.7% |
IBM Watson | 11.2% |
Amazon AI | 9.8% |
OpenAI | 8.4% |
Future Outlook and Predictions
The What Billion Code landscape is evolving rapidly, driven by technological advancements, changing threat vectors, and shifting business requirements. Based on current trends and expert analyses, we can anticipate several significant developments across different time horizons:
Year-by-Year Technology Evolution
Based on current trajectory and expert analyses, we can project the following development timeline:
Technology Maturity Curve
Different technologies within the ecosystem are at varying stages of maturity, influencing adoption timelines and investment priorities:
Innovation Trigger
- Generative AI for specialized domains
- Blockchain for supply chain verification
Peak of Inflated Expectations
- Digital twins for business processes
- Quantum-resistant cryptography
Trough of Disillusionment
- Consumer AR/VR applications
- General-purpose blockchain
Slope of Enlightenment
- AI-driven analytics
- Edge computing
Plateau of Productivity
- Cloud infrastructure
- Mobile applications
Technology Evolution Timeline
- Improved generative models
- specialized AI applications
- AI-human collaboration systems
- multimodal AI platforms
- General AI capabilities
- AI-driven scientific breakthroughs
Expert Perspectives
Leading experts in the ai tech sector provide diverse perspectives on how the landscape will evolve over the coming years:
"The next frontier is AI systems that can reason across modalities and domains with minimal human guidance."
— AI Researcher
"Organizations that develop effective AI governance frameworks will gain competitive advantage."
— Industry Analyst
"The AI talent gap remains a critical barrier to implementation for most enterprises."
— Chief AI Officer
Areas of Expert Consensus
- Acceleration of Innovation: The pace of technological evolution will continue to increase
- Practical Integration: Focus will shift from proof-of-concept to operational deployment
- Human-Technology Partnership: Most effective implementations will optimize human-machine collaboration
- Regulatory Influence: Regulatory frameworks will increasingly shape technology development
Short-Term Outlook (1-2 Years)
In the immediate future, organizations will focus on implementing and optimizing currently available technologies to address pressing ai tech challenges:
- Improved generative models
- specialized AI applications
- enhanced AI ethics frameworks
These developments will be characterized by incremental improvements to existing frameworks rather than revolutionary changes, with emphasis on practical deployment and measurable outcomes.
Mid-Term Outlook (3-5 Years)
As technologies mature and organizations adapt, more substantial transformations will emerge in how security is approached and implemented:
- AI-human collaboration systems
- multimodal AI platforms
- democratized AI development
This period will see significant changes in security architecture and operational models, with increasing automation and integration between previously siloed security functions. Organizations will shift from reactive to proactive security postures.
Long-Term Outlook (5+ Years)
Looking further ahead, more fundamental shifts will reshape how cybersecurity is conceptualized and implemented across digital ecosystems:
- General AI capabilities
- AI-driven scientific breakthroughs
- new computing paradigms
These long-term developments will likely require significant technical breakthroughs, new regulatory frameworks, and evolution in how organizations approach security as a fundamental business function rather than a technical discipline.
Key Risk Factors and Uncertainties
Several critical factors could significantly impact the trajectory of ai tech evolution:
Organizations should monitor these factors closely and develop contingency strategies to mitigate potential negative impacts on technology implementation timelines.
Alternative Future Scenarios
The evolution of technology can follow different paths depending on various factors including regulatory developments, investment trends, technological breakthroughs, and market adoption. We analyze three potential scenarios:
Optimistic Scenario
Responsible AI driving innovation while minimizing societal disruption
Key Drivers: Supportive regulatory environment, significant research breakthroughs, strong market incentives, and rapid user adoption.
Probability: 25-30%
Base Case Scenario
Incremental adoption with mixed societal impacts and ongoing ethical challenges
Key Drivers: Balanced regulatory approach, steady technological progress, and selective implementation based on clear ROI.
Probability: 50-60%
Conservative Scenario
Technical and ethical barriers creating significant implementation challenges
Key Drivers: Restrictive regulations, technical limitations, implementation challenges, and risk-averse organizational cultures.
Probability: 15-20%
Scenario Comparison Matrix
Factor | Optimistic | Base Case | Conservative |
---|---|---|---|
Implementation Timeline | Accelerated | Steady | Delayed |
Market Adoption | Widespread | Selective | Limited |
Technology Evolution | Rapid | Progressive | Incremental |
Regulatory Environment | Supportive | Balanced | Restrictive |
Business Impact | Transformative | Significant | Modest |
Transformational Impact
Redefinition of knowledge work, automation of creative processes. This evolution will necessitate significant changes in organizational structures, talent development, and strategic planning processes.
The convergence of multiple technological trends—including artificial intelligence, quantum computing, and ubiquitous connectivity—will create both unprecedented security challenges and innovative defensive capabilities.
Implementation Challenges
Ethical concerns, computing resource limitations, talent shortages. Organizations will need to develop comprehensive change management strategies to successfully navigate these transitions.
Regulatory uncertainty, particularly around emerging technologies like AI in security applications, will require flexible security architectures that can adapt to evolving compliance requirements.
Key Innovations to Watch
Multimodal learning, resource-efficient AI, transparent decision systems. Organizations should monitor these developments closely to maintain competitive advantages and effective security postures.
Strategic investments in research partnerships, technology pilots, and talent development will position forward-thinking organizations to leverage these innovations early in their development cycle.
Technical Glossary
Key technical terms and definitions to help understand the technologies discussed in this article.
Understanding the following technical concepts is essential for grasping the full implications of the security threats and defensive measures discussed in this article. These definitions provide context for both technical and non-technical readers.