IBM Granite 3.2 uses conditional reasoning, time series forecasting and document vision to tackle challenging enterprise use cases - Related to uses, developers, time, civic, ibm
Generative AI and Civic Institutions
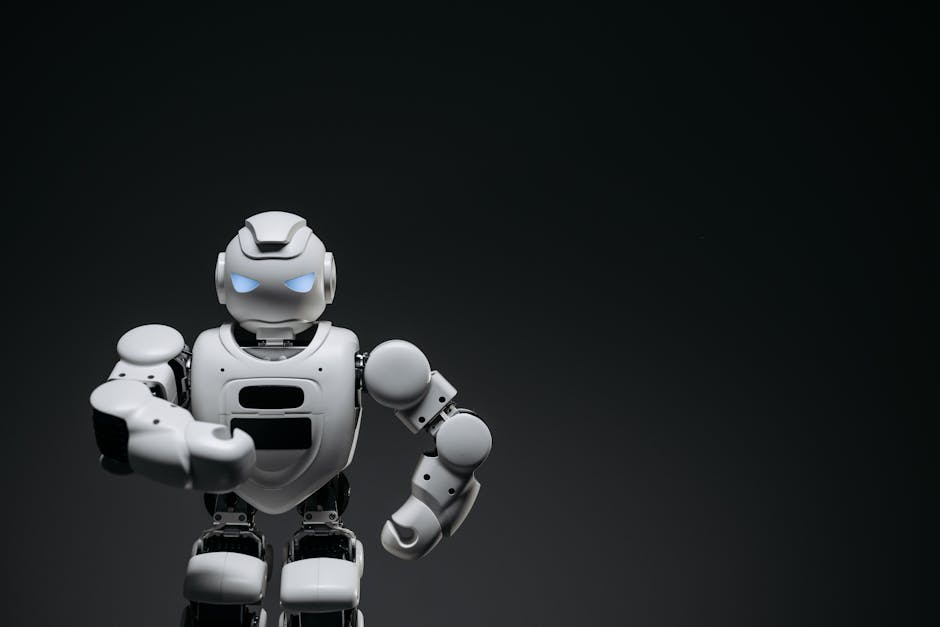
Recent events have got me thinking about AI as it relates to our civic institutions — think government, education, public libraries, and so on. We often forget that civic and governmental organizations are inherently deeply different from private companies and profit-making enterprises. They exist to enable people to live their best lives, protect people’s rights, and. Make opportunities accessible, even if (especially if) this work doesn’t have immediate monetary returns. The public library is an example I often think about, as I come from a library-loving and defending family — their goal is to provide books, cultural materials, social supports, community engagement, and. A love of reading to the entire community, regardless of ability to pay.
In the private sector, efficiency is an optimization goal because any dollar spent on providing a product or service to clients is a dollar taken away from the profits. The (simplified) goal is to spend the bare minimum possible to run your business. With the maximum amount returned to you or the shareholders in profit form. In the civic space, on the other hand, efficiency is only a meaningful goal insomuch as it enables higher effectiveness — more of the service the institution provides getting to more constituents.
In the civic space, efficiency is only a meaningful goal insomuch as it enables higher effectiveness — more of the service the institution provides getting to more constituents.
So, if you’re at the library, and you could use an Ai Chatbot to answer patron questions online instead of assigning a librarian to do that. That librarian could be helping in-person patrons, developing educational curricula, supporting community services, or many other things. That’s a general efficiency that could make for higher effectiveness of the library as an institution. Moving from card catalogs to digital catalogs is a prime example of this kind of efficiency to effectiveness pipeline, because you can find out from your couch whether the book you want is in stock using search keywords instead of flipping through hundreds of notecards in a cabinet drawer like we did when I was a kid.
However, we can pivot too hard in the direction of efficiency and. Lose sight of the end goal of effectiveness. If, for example, your online librarian chat is often used by schoolchildren at home to get homework help, replacing them with an AI chatbot could be a disaster — after getting incorrect information from such a bot and getting a bad grade at school. A child might be turned off from patronizing the library or seeking help there for a long time, or forever. So, it’s significant to deploy Generative Ai solutions only when it is well thought out and purposeful, not just because the media is telling us that “AI is neat.” (Eagle-eyed readers will know that this is basically similar advice to what I’ve stated in the past about deploying AI in businesses as well.).
As a result, what we thought was a gain in efficiency leading to net higher effectiveness actually could diminish the number of lifelong patrons and. Library visitors, which would mean a loss of effectiveness for the library. Sometimes unintended effects from attempts to improve efficiency can diminish our ability to provide a universal service. That is, there may be a tradeoff between making every single dollar stretch as far as it can possibly go and providing reliable, comprehensive services to all the constituents of your institution.
Sometimes unintended effects from attempts to improve efficiency can diminish our ability to provide a universal service.
It’s worth it to take a closer look at this concept — AI as a driver of efficiency. Broadly speaking, the theory we hear often is that incorporating generative AI more into our workplaces and organizations can increase productivity. Framing it at the most Econ 101 level: using AI, more work can be completed by fewer people in the same amount of time. Right?
Let’s challenge some aspects of this idea. AI is useful to complete certain tasks but is sadly inadequate for others. (As our imaginary schoolchild library patron learned, an LLM is not a reliable source of facts, and should not be treated like one.) So, AI’s ability to increase the volume of work being done with fewer people (efficiency) is limited by what kind of work we need to complete.
If our chat interface is only used for simple questions like “What are the library’s hours on Memorial Day?” we can hook up a RAG (Retrieval Augmented Generation) system with an LLM and. Make that quite useful. But outside of the limited bounds of what information we can provide to the LLM, we should probably set guard rails and make the model refuse to try and answer. To avoid giving out false information to patrons.
So, let’s play that out. We have a chatbot that does a very limited job, but does it well. The librarian who was on chatbot duty now may have some reduction in the work required of them, but. There are still going to be a subset of questions that still require their help. We have some choices: put the librarian on chatbot duty for a reduced number of hours a week. Hoping the questions come in when they’re on? Tell people to just call the reference desk or send an email if the chatbot refuses to answer them? Hope that people come in to the library in person to ask their questions?
I suspect the likeliest option is actually “the patron will seek their answer elsewhere, perhaps from another LLM like ChatGPT, Claude. Or Gemini.” Once again, we’ve ended up in a situation where the library loses patronage because their offering wasn’t meeting the needs of the patron. And to boot, the patron may have gotten another wrong answer somewhere else, for all we know.
I am spinning out this long example just to illustrate that efficiency and effectiveness in the civic environment can have a lot more push and. Pull than we would initially assume. It’s not to say that AI isn’t useful to help civic organizations stretch their capabilities to serve the public, of course! But just like with any application of generative AI, we need to be very careful to think about what we’re doing, what our goals are, and whether those two are compatible.
Now, this has been a very simplistic example, and. Eventually we could hook up the whole encyclopedia to that chatbot RAG or something, of course, and try to make it work. In fact, I think we can and should continue developing more ways to chain together AI models to expand the scope of valuable work they can do. Including making different specific models for different responsibilities. However, this development is itself work. It’s not really just a matter of “people do work” or “models do work”, but. Instead it’s “people do work building AI” or “people do work providing services to people”. There’s a calculation to be made to determine when it would be more efficient to do the targeted work itself, and when AI is the right way to go.
Working on the AI has an advantage in that it will hopefully render the task reproducible, so it will lead to efficiency. But let’s remember that AI engineering is vastly different from the work of the reference librarian. We’re not interchanging the same workers, tasks, or skill sets here, and. In our contemporary economy, the AI engineer’s time costs a heck of a lot more. So if we did want to measure this efficiency all in dollars and cents, the same amount of time spent working at the reference desk and. Doing the chat service will be much cheaper than paying an AI engineer to develop a superior agentic AI for the use case. Given a bit of time, we could calculate out how many hours, days, years of work as a reference librarian we’d need to save with this chatbot to make it worth building, but often that calculation isn’t done before we move towards AI solutions.
We need to interrogate the assumption that incorporating generative AI in any given scenario is a guaranteed net gain in efficiency.
While we’re on this topic of weighing whether the AI solution is worth doing in a particular situation, we should remember that developing and. Using AI for tasks does not happen in a vacuum. It has some cost environmentally and economically when we choose to use a generative AI tool, even when it’s a single prompt and. A single response. Consider that the newly released has increased prices 30x for input tokens ($ per million to $75 per million) and. 15x for output tokens ($10 per million to $150 per million) just since GPT-4o. And that isn’t even taking into account the water consumption for cooling data centers (3 bottles per 100 word output for GPT-4), electricity use. And rare earth minerals used in GPUs. Many civic institutions have as a macro level goal to improve the world around them and the lives of the citizens of their communities. And concern for the environment has to have a place in that. Should organizations whose purpose is to have a positive impact weigh the possibility of incorporating AI more carefully? I think so.
Plus, I don’t often get too much into this, but. I think we should take a moment to consider some folks’ end game for incorporating AI — reducing staffing altogether. Instead of making our existing dollars in an institution go farther, some people’s idea is just reducing the number of dollars and. Redistributing those dollars somewhere else. This brings up many questions, naturally, about where those dollars will go instead and whether they will be used to advance the interests of the community residents some other way. But let’s set that aside for now. My concern is for the people who might lose their jobs under this administrative model.
For-profit companies hire and fire employees all the time, and. Their priorities and objectives are focused on profit, so this is not particularly hypocritical or inconsistent. But as I noted above, civic organizations have objectives around improving the community or communities in which they exist. In a very real way, they are advancing that goal when part of what they provide is economic opportunity to their workers. We live in a Society where working is the overwhelmingly predominant way people provide for themselves and their families, and giving jobs to people in the community and supporting the economic well-being of the community is a role that civic institutions do play.
[R]educing staffing is not an unqualified good for civic organizations and government, but instead must be balanced critically against whatever other use the money that was paying their salaries will go to.
At the bare minimum. This means that reducing staffing is not an unqualified good for civic organizations and government, but instead must be balanced critically against whatever other use the money that was paying their salaries will go to. It’s not impossible for reducing staff to be the right decision, but. We have to bluntly acknowledge that when members of communities experience joblessness, that effect cascades. They are now no longer able to patronize the shops and services they would have been supporting with their money, the tax base may be reduced, and this negatively affects the whole collective.
Workers aren’t just workers; they’re also patrons. individuals, and participants in all aspects of the community. When we think of civic workers as simply money pits to be replaced with AI or whose cost for labor we need to minimize, we lose sight of the reasons for the work to be done in the first place.
I hope this discussion has brought some clarity about how really difficult it is to decide if, when. And how to apply generative AI to the civic space. It’s not nearly as simple a thought process as it might be in the for-profit sphere because the purpose and. Core meaning of civic institutions are completely different. Those of us who do machine learning and build AI solutions in the private sector might think, “Oh, I can see a way to use this in government,” but we have to recognize and appreciate the complex contextual implications that might have.
Next month, I’ll be bringing you a discussion of how social science research is incorporating generative AI. Which has some very intriguing aspects.
“It’s a lemon”-OpenAI’s largest AI model ever arrives to mixed reviews: offers marginal gains in capability and poor coding performance despite 30x the cost. .
Using GPT-4 to generate 100 words consumes up to 3 bottles of water: New research demonstrates generative AI consumes a lot of water – up to 1,408ml to generate 100 words of text. .
Environmental Implications of the AI Boom: The digital world can’t exist without the natural resources to run it. What are the costs of the tech we’re using… .
Economics of Generative AI: What’s the business model for generative AI, given what we know today about the technology and. The market? .
Stability AI, known for its Stable Diffusion text-to-image models, has collaborated with global semiconductor giant Arm to add generative audio AI cap...
Co-founder and CEO of Perplexity AI, Aravind Srinivas. Has joined Astra as an angel investor, lending his expertise and resources to the AI startup. F...
Building on these developments, there are various platforms to help people create videos, art, posters, and all kinds of creative assets. You will also find AI artists selling prompt...
IBM Granite 3.2 uses conditional reasoning, time series forecasting and document vision to tackle challenging enterprise use cases
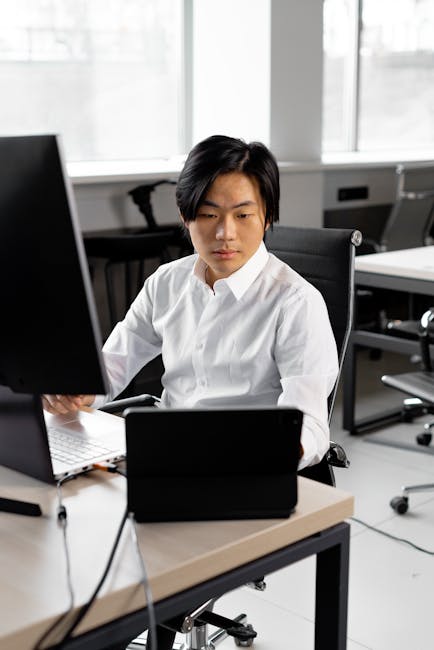
In the wake of the disruptive debut of DeepSeek-R1, reasoning models have been all the rage so far in 2025.
IBM is now joining the party. With the debut today of its Granite large language model (LLM) family. Unlike other reasoning approaches such as DeepSeek-R1 or OpenAI’s o3, IBM is deeply embedding reasoning into its core open-source Granite models. It’s an approach that IBM refers to as conditional reasoning, where the step-by-step chain of thought (CoT) reasoning is an option within the models (as opposed to being a separate model).
It’s a flexible approach where reasoning can be conditionally activated with a flag. Allowing consumers to control when to use more intensive processing. The new reasoning capability builds on the performance gains IBM introduced with the release of the Granite LLMs in Dec. 2024.
IBM is also releasing a new vision model in the Granite family specifically optimized for document processing. The model is particularly useful for digitizing legacy documents, a challenge many large organizations struggle with.
Another enterprise AI challenge IBM aims to solve with Granite is predictive modelling. Machine learning (ML) has been used for predictions for decades, but. It hasn’t had the natural language interface and ease of use of modern gen AI. That’s where IBM’s Granite time series forecasting models fit in; they apply transformer technology to predict future values from time-based data.
“Reasoning is not something a model is, it’s something a model does,” David Cox, VP for AI models at IBM Research, told VentureBeat.
What IBM’s reasoning actually brings to enterprise AI.
While there has been no shortage of excitement and. Hype around reasoning models in 2025, reasoning for its own sake doesn’t necessarily provide value to enterprise people.
The ability to reason in many respects has long been part of gen AI. Simply prompting an LLM to answer in a step-by-step approach triggers a basic CoT reasoning output. Modern reasoning in models like DeepSeek-R1 and now Granite goes a bit deeper by using reinforcement learning to train and enable reasoning capabilities.
While CoT prompts may be effective for certain tasks like mathematics. The reasoning capabilities in Granite can benefit a wider range of enterprise applications. Cox noted that by encouraging the model to spend more time thinking, enterprises can improve complex decision-making processes. Reasoning can benefit software engineering tasks, IT issue resolution and other agentic workflows where the model can break down problems, make more effective judgments and recommend more informed solutions.
IBM also hints at that, with reasoning turned on. Granite is able to outperform rivals including DeepSeek-R1 on instruction-following tasks.
Not every query needs more reasoning; why conditional thinking matters.
Although Granite has advanced reasoning capabilities, Cox stressed that not every query actually needs more reasoning. In fact, many types of common queries can actually be negatively impacted with more reasoning.
For example, for a knowledge-based query, a standalone reasoning model like DeepSeek-R1 might spend up to 50 seconds on an internal monologue to answer a basic question like “Where is Rome?”.
One of the key innovations in Granite is the introduction of a conditional thinking feature. Which allows developers to dynamically activate or deactivate the model’s reasoning capabilities. This flexibility enables consumers to strike a balance between speed and depth of analysis, depending on the specific task at hand.
Going a step further, the Granite models benefit from a method developed by IBM’s Red Hat business unit that uses something called a “particle filter” to enable more flexible reasoning capabilities.
Additionally, this approach allows the model to dynamically control and manage multiple threads of reasoning. Evaluating which ones are the most promising to arrive at the final result. This provides a more dynamic and adaptive reasoning process, rather than a linear CoT. Cox explained that this particle filter technique gives enterprises even more flexibility in how they can use the model’s reasoning capabilities.
In the particle filter approach. There are many threads of reasoning occurring simultaneously. The particle filter is pruning the less effective approaches, focusing on the ones that provide advanced outcomes. So, instead of just doing CoT reasoning, there are multiple approaches to solving a problem. The model can intelligently navigate complex problems, selectively focusing on the most promising lines of reasoning.
How IBM is solving real enterprise uses cases for documents.
Large organizations tend to have equally large volumes of documents, many of which were scanned years ago and. Now sitting in archives. All that data has been difficult to use with modern systems.
Moving to another aspect, the new Granite vision model is designed to help solve that enterprise challenge. While many multimodal models focus on general image understanding, Granite ’s vision capabilities are engineered specifically for document processing — reflecting IBM’s focus on solving tangible enterprise problems rather than chasing benchmark scores.
The system targets what Cox described as “irrational amounts of old scanned documents” sitting in enterprise archives. Particularly in financial institutions. These represent opaque data stores that have remained largely untapped despite their potential business value.
For organizations with decades of paper records, the ability to intelligently process documents containing charts, figures and tables represents a substantial operational advantage over general-purpose multimodal models that excel at describing vacation photos but struggle with structured business documents.
On enterprise benchmarks such as DocVQA and ChartQA, IBM Granite vision presents strong results against rivals.
Time series forecasting addresses critical business prediction needs.
Perhaps the most technically distinctive component of the release is IBM’s “tiny time mixers” (TTM)– specialized transformer-based models designed specifically for time series forecasting.
However. Time series forecasting, which enables predictive analytics and modelling, is not new. Cox noted that for various reasons, time series models have remained stuck in the older era of machine learning (ML) and have not benefited from the same attention of the newer. Flashier gen AI models.
The Granite TTM models apply the architectural innovations that powered LLM advances to an entirely different problem domain: Predicting future values based on historical patterns. This capability addresses critical business needs across financial forecasting, equipment maintenance scheduling and anomaly detection.
Taking a practical enterprise-focused approach to gen AI.
There is no shortage of hype and vendors are all claiming to outdo each other on an endless array of industry benchmarks.
For enterprise decision-makers, taking note of benchmarks can be interesting. But that’s not what solves pain points. Cox emphasized that IBM is taking the ‘suit and tie’ approach to enterprise AI, looking to solve real problems.
“I think there’s a lot of magical thinking happening that we can have one super intelligent model that’s going to somehow do everything we need it to do and, at least for the time being. We’re not even close to that,” presented Cox. “Our strategy is ‘Let’s build real, practical tools using this very exciting technology, and let’s build in as many of the functions as possible that make it easy to do real work.'”.
Partnerships between AI companies and the US government are expanding, even as the future of AI safety and. Regu...
Sorbonne Université dévoile « Delacroix numérique », un ambitieux projet qui allie intelligence artificielle et humanités numériques pour redécouvrir ...
Les attaques par deepfakes se multiplient de façon inquiétante. Selon une enquête de Regula, près de 49 % des entreprises ont déjà été confrontées à d...
Why Developers are Quitting LangChain
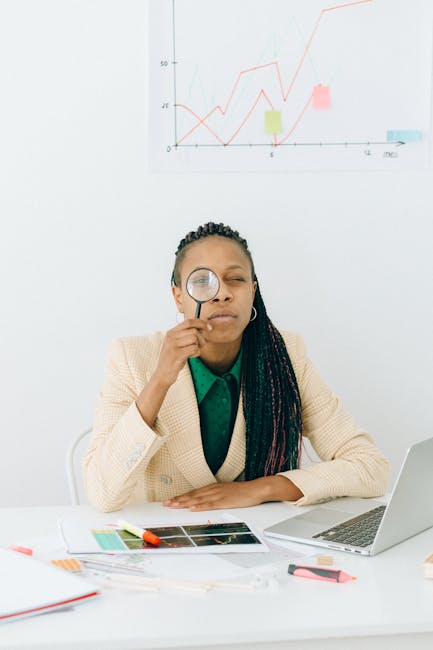
LangChain once held promise as a go-to framework for many developers to build applications powered by LLMs. Even then, it was not perfect and people had a lot of issues. However, a growing number of developers are now moving away from it, citing issues ranging from unnecessary complexity to unstable updates.
While some still find value in LangChain’s attributes. The overall sentiment indicates that many seek alternatives such as Pydantic or LlamaIndex. One of the most common complaints among developers is LangChain’s instability. Frequent changes to the API structure, coupled with inconsistent documentation, have frustrated people.
In a Reddit discussion, a developer mentioned, “It’s unstable, the interface constantly changes. The documentation is regularly out of date, and the abstractions are overly complicated.” Similar sentiments are echoed throughout the community. Many developers find themselves reading the source code instead of relying on the documentation.
‘LangChain is Overcomplicating Things for No Reason’.
A few months back, the engineering team at Octomind. A software enterprise, wrote a detailed blog on why they dropped out of LangChain. The framework’s inflexibility made it difficult to improve lower-level behaviour, and its intentional abstraction of details hindered writing lower-level code.
“When we wanted to move from an architecture with a single sequential agent to something more complex. LangChain was the limiting factor,” read the blog.
LangChain’s complexity has led many to question its design choices. Developers have criticised its layers of abstraction, which make it harder to understand and modify. Experienced developers like Praveer Kochhar, co-founder of Kogo Tech Labs, have questioned the framework and declared that it is not meant for production.
Meanwhile, Angelina Y, the co-founder of OSCR AI. stated that as time passes, more people realise that frameworks like LangChain and LlamaIndex are not good for production. “Practically becoming a versatile tool of no use! Of course, I must say that they are very good for making prototypes, especially LlamaIndex,” she added.
Many feel that the framework prioritises “enterprise-level” aesthetics over practical usability.
Last year. AIM also noted that there are a lot of problems with LangChain that continue to remain unresolved. It also uses the same amount of code as the original libraries of OpenAI and others, which makes it feel like bloatware on top of the original APIs, making it inefficient for production use.
For a framework that aims to help developers build reliable AI applications. Many find LangChain unsuitable for production. A developer stated that their team did a POC project with LangChain, and. There were so many changes that they couldn’t improvement without major code edits. “We are going to get rid of LangChain in our code instead of upgrading it.”.
While some developers acknowledge that LangChain is still in rapid development. Many feel it lacks the stability required for serious projects. While LangGraph, a related project, is stable, LangChain itself has become bloated.
Kieran Klaassen, co-founder of Every Inc, showcased, “LangChain is where good AI projects go to die.” He added that experienced developers call it “the worst library they’ve ever worked with” due to its bloated abstractions and. Black-box design.
He advised developers to build their own stack instead. “You’ll spend less time fighting someone else’s broken framework and more time shipping actual capabilities that work.”.
Given these challenges, many developers are exploring alternatives that are. Admittedly, also not there. Even then, some prefer custom-built solutions over relying on an unstable framework.
For example, PydanticAI offers a more streamlined approach and is ‘Pythony’. This seems similar to what LangChain was known for — the PyTorch for building LLMs. However, just like LangChain, PydanticAI also faces similar issues.
Another emerging alternative is PocketFlow, which aims to provide a more modular and developer-friendly experience. Developers have also opted for LlamaIndex for a long time.
While LangChain has its proponents. The growing dissatisfaction hints at it must address key concerns to regain developer trust. Stability, superior documentation, and a focus on practical usability over unnecessary abstractions could help prevent further decline.
However, for many. The damage may already be done. While it may still be useful for rapid prototyping, many are moving to more stable and flexible alternatives. Whether LangChain can turn things around remains to be seen. For now, however, many developers are letting it go.
India continues to face a critical debate: Should the country invest in developing its own foundational LLM or focus on building applications on top o...
ZDNET's key takeaways Acer's Aspire 14 AI is available now at Costco for $699.
It has a fantastic battery and. Some of the latest hardware: all for a ...
Shenzhen-based UBTECH Robotics has successfully completed what is claimed to be the world’s first multi-humanoid robot collaborative training program ...
Market Impact Analysis
Market Growth Trend
2018 | 2019 | 2020 | 2021 | 2022 | 2023 | 2024 |
---|---|---|---|---|---|---|
23.1% | 27.8% | 29.2% | 32.4% | 34.2% | 35.2% | 35.6% |
Quarterly Growth Rate
Q1 2024 | Q2 2024 | Q3 2024 | Q4 2024 |
---|---|---|---|
32.5% | 34.8% | 36.2% | 35.6% |
Market Segments and Growth Drivers
Segment | Market Share | Growth Rate |
---|---|---|
Machine Learning | 29% | 38.4% |
Computer Vision | 18% | 35.7% |
Natural Language Processing | 24% | 41.5% |
Robotics | 15% | 22.3% |
Other AI Technologies | 14% | 31.8% |
Technology Maturity Curve
Different technologies within the ecosystem are at varying stages of maturity:
Competitive Landscape Analysis
Company | Market Share |
---|---|
Google AI | 18.3% |
Microsoft AI | 15.7% |
IBM Watson | 11.2% |
Amazon AI | 9.8% |
OpenAI | 8.4% |
Future Outlook and Predictions
The Generative Civic Institutions landscape is evolving rapidly, driven by technological advancements, changing threat vectors, and shifting business requirements. Based on current trends and expert analyses, we can anticipate several significant developments across different time horizons:
Year-by-Year Technology Evolution
Based on current trajectory and expert analyses, we can project the following development timeline:
Technology Maturity Curve
Different technologies within the ecosystem are at varying stages of maturity, influencing adoption timelines and investment priorities:
Innovation Trigger
- Generative AI for specialized domains
- Blockchain for supply chain verification
Peak of Inflated Expectations
- Digital twins for business processes
- Quantum-resistant cryptography
Trough of Disillusionment
- Consumer AR/VR applications
- General-purpose blockchain
Slope of Enlightenment
- AI-driven analytics
- Edge computing
Plateau of Productivity
- Cloud infrastructure
- Mobile applications
Technology Evolution Timeline
- Improved generative models
- specialized AI applications
- AI-human collaboration systems
- multimodal AI platforms
- General AI capabilities
- AI-driven scientific breakthroughs
Expert Perspectives
Leading experts in the ai tech sector provide diverse perspectives on how the landscape will evolve over the coming years:
"The next frontier is AI systems that can reason across modalities and domains with minimal human guidance."
— AI Researcher
"Organizations that develop effective AI governance frameworks will gain competitive advantage."
— Industry Analyst
"The AI talent gap remains a critical barrier to implementation for most enterprises."
— Chief AI Officer
Areas of Expert Consensus
- Acceleration of Innovation: The pace of technological evolution will continue to increase
- Practical Integration: Focus will shift from proof-of-concept to operational deployment
- Human-Technology Partnership: Most effective implementations will optimize human-machine collaboration
- Regulatory Influence: Regulatory frameworks will increasingly shape technology development
Short-Term Outlook (1-2 Years)
In the immediate future, organizations will focus on implementing and optimizing currently available technologies to address pressing ai tech challenges:
- Improved generative models
- specialized AI applications
- enhanced AI ethics frameworks
These developments will be characterized by incremental improvements to existing frameworks rather than revolutionary changes, with emphasis on practical deployment and measurable outcomes.
Mid-Term Outlook (3-5 Years)
As technologies mature and organizations adapt, more substantial transformations will emerge in how security is approached and implemented:
- AI-human collaboration systems
- multimodal AI platforms
- democratized AI development
This period will see significant changes in security architecture and operational models, with increasing automation and integration between previously siloed security functions. Organizations will shift from reactive to proactive security postures.
Long-Term Outlook (5+ Years)
Looking further ahead, more fundamental shifts will reshape how cybersecurity is conceptualized and implemented across digital ecosystems:
- General AI capabilities
- AI-driven scientific breakthroughs
- new computing paradigms
These long-term developments will likely require significant technical breakthroughs, new regulatory frameworks, and evolution in how organizations approach security as a fundamental business function rather than a technical discipline.
Key Risk Factors and Uncertainties
Several critical factors could significantly impact the trajectory of ai tech evolution:
Organizations should monitor these factors closely and develop contingency strategies to mitigate potential negative impacts on technology implementation timelines.
Alternative Future Scenarios
The evolution of technology can follow different paths depending on various factors including regulatory developments, investment trends, technological breakthroughs, and market adoption. We analyze three potential scenarios:
Optimistic Scenario
Responsible AI driving innovation while minimizing societal disruption
Key Drivers: Supportive regulatory environment, significant research breakthroughs, strong market incentives, and rapid user adoption.
Probability: 25-30%
Base Case Scenario
Incremental adoption with mixed societal impacts and ongoing ethical challenges
Key Drivers: Balanced regulatory approach, steady technological progress, and selective implementation based on clear ROI.
Probability: 50-60%
Conservative Scenario
Technical and ethical barriers creating significant implementation challenges
Key Drivers: Restrictive regulations, technical limitations, implementation challenges, and risk-averse organizational cultures.
Probability: 15-20%
Scenario Comparison Matrix
Factor | Optimistic | Base Case | Conservative |
---|---|---|---|
Implementation Timeline | Accelerated | Steady | Delayed |
Market Adoption | Widespread | Selective | Limited |
Technology Evolution | Rapid | Progressive | Incremental |
Regulatory Environment | Supportive | Balanced | Restrictive |
Business Impact | Transformative | Significant | Modest |
Transformational Impact
Redefinition of knowledge work, automation of creative processes. This evolution will necessitate significant changes in organizational structures, talent development, and strategic planning processes.
The convergence of multiple technological trends—including artificial intelligence, quantum computing, and ubiquitous connectivity—will create both unprecedented security challenges and innovative defensive capabilities.
Implementation Challenges
Ethical concerns, computing resource limitations, talent shortages. Organizations will need to develop comprehensive change management strategies to successfully navigate these transitions.
Regulatory uncertainty, particularly around emerging technologies like AI in security applications, will require flexible security architectures that can adapt to evolving compliance requirements.
Key Innovations to Watch
Multimodal learning, resource-efficient AI, transparent decision systems. Organizations should monitor these developments closely to maintain competitive advantages and effective security postures.
Strategic investments in research partnerships, technology pilots, and talent development will position forward-thinking organizations to leverage these innovations early in their development cycle.
Technical Glossary
Key technical terms and definitions to help understand the technologies discussed in this article.
Understanding the following technical concepts is essential for grasping the full implications of the security threats and defensive measures discussed in this article. These definitions provide context for both technical and non-technical readers.