Advancing conservation with AI-based facial recognition of turtles - Related to best, programming, data, conservation, practices
Advancing conservation with AI-based facial recognition of turtles
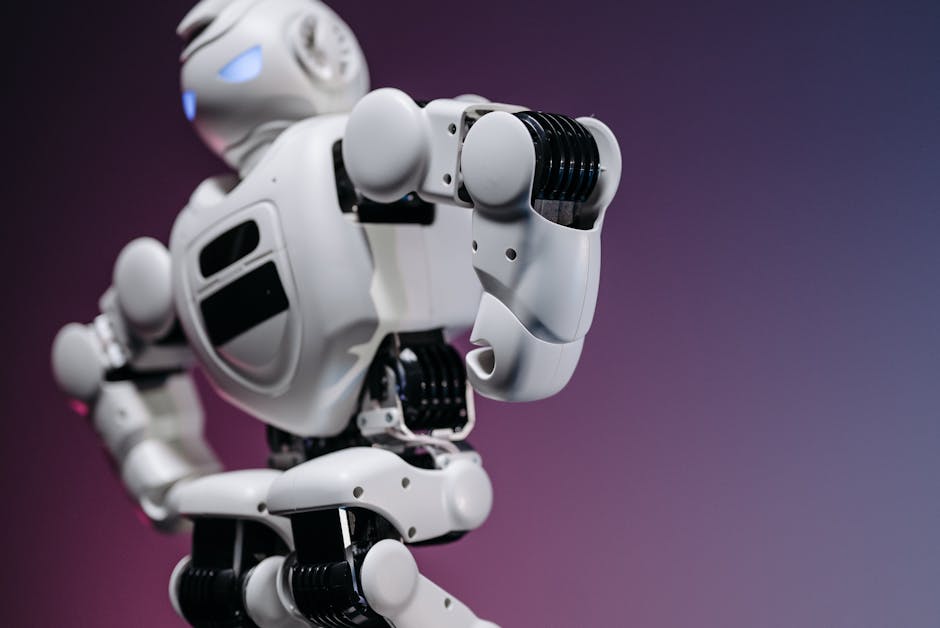
Finding solutions to improve turtle reidentification and supporting machine learning projects across Africa.
Protecting the ecosystems around us is critical to safeguarding the future of our planet and all its living citizens. Fortunately, new artificial intelligence (AI) systems are making progress in conservation efforts worldwide, helping tackle complex problems at scale – from studying the behaviour of animal communities in the Serengeti to help conserve the diminishing ecosystem, to spotting poachers and their wounded prey to prevent species going extinct.
As part of our mission to help benefit humanity with the technologies we develop, it's crucial we ensure diverse groups of people build the AI systems of the future so that it’s equitable and fair. This includes broadening the machine learning (ML) community and engaging with wider audiences on addressing crucial problems using AI.
Through investigation, we came across Zindi – a dedicated partner with complementary goals – who are the largest community of African data scientists and host competitions that focus on solving Africa’s most pressing problems.
Our Science team’s Diversity, Equity, and Inclusion (DE&I) team worked with Zindi to identify a scientific challenge that could help advance conservation efforts and grow involvement in AI. Inspired by Zindi’s bounding box turtle challenge, we landed on a project with the potential for real impact: turtle facial recognition.
Biologists consider turtles to be an indicator species. These are classes of organisms whose behaviour helps scientists understand the underlying welfare of their ecosystem. For example, the presence of otters in rivers has been considered a sign of a clean, healthy river, since a ban on chlorine pesticides in the 1970s brought the species back from the brink of extinction.
Turtles are another such species. By grazing on seagrass cover, they cultivate the ecosystem, providing a habitat for numerous fish and crustaceans. Traditionally, individual turtles have been identified and tracked by biologists with physical tags, though frequent loss or erosion of these tags in seawater has made this an unreliable method. To help solve some of these challenges, we launched an ML challenge called Turtle Recall.
Research Google DeepMind at ICLR 2024 Share.
Developing next-gen AI agents, exploring new modalities, and pioneering foundational l...
Impact AlphaDev discovers faster sorting algorithms Share.
New algorithms will transform the foundations of computing Digital socie...
With the help of AlphaFold, researchers are designing more effective drugs like never before.
Karen Akinsanya is President of R&D, Therapeutics, at Sc...
Competitive programming with AlphaCode
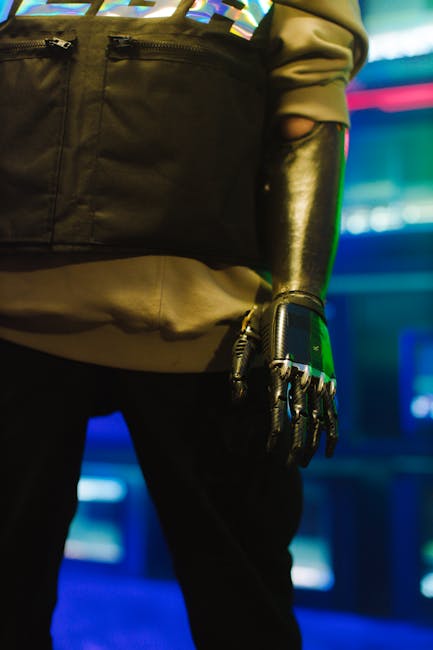
Note: This blog was first . Following the paper’s publication in Science on 8 Dec 2022, we’ve made minor updates to the text to reflect this.
Solving novel problems and setting a new milestone in competitive programming.
Creating solutions to unforeseen problems is second nature in human intelligence – a result of critical thinking informed by experience. The machine learning community has made tremendous progress in generating and understanding textual data, but advances in problem solving remain limited to relatively simple maths and programming problems, or else retrieving and copying existing solutions.
As part of DeepMind’s mission to solve intelligence, we created a system called AlphaCode that writes computer programs at a competitive level. AlphaCode achieved an estimated rank within the top 54% of participants in programming competitions by solving new problems that require a combination of critical thinking, logic, algorithms, coding, and natural language understanding.
, our paper details AlphaCode, which uses transformer-based language models to generate code at an unprecedented scale, and then smartly filters to a small set of promising programs.
We validated our performance using competitions hosted on Codeforces, a popular platform which hosts regular competitions that attract tens of thousands of participants from around the world who come to test their coding skills. We selected for evaluation 10 recent contests, each newer than our training data. AlphaCode placed at about the level of the median competitor, marking the first time an AI code generation system has reached a competitive level of performance in programming competitions.
To help others build on our results, we’ve released our dataset of competitive programming problems and solutions on GitHub, including extensive tests to ensure the programs that pass these tests are correct — a critical feature current datasets lack. We hope this benchmark will lead to further innovations in problem solving and code generation.
Responsibility & Safety Updating the Frontier Safety Framework Share.
Our next iteration of the FSF sets out stronger security prot...
Open-source AI is changing everything people thought they knew about artificial intelligence. Just look at DeepSeek...
Research Google DeepMind at ICLR 2024 Share.
Developing next-gen AI agents, exploring new modalities, and pioneering foundational l...
Best practices for data enrichment
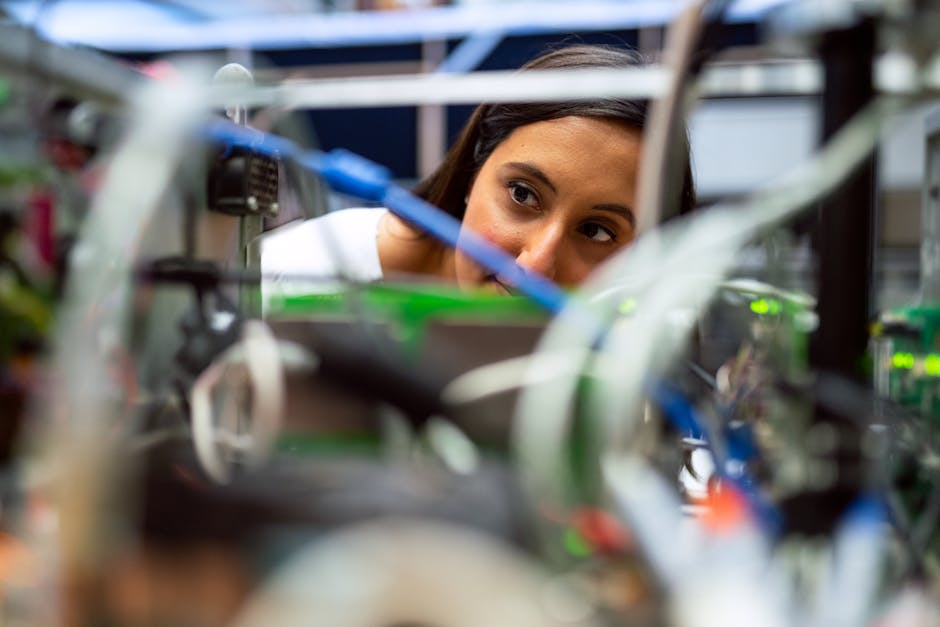
Building a responsible approach to data collection with the Partnership on AI.
At DeepMind, our goal is to make sure everything we do meets the highest standards of safety and ethics, in line with our Operating Principles. One of the most crucial places this starts with is how we collect our data. In the past 12 months, we’ve collaborated with Partnership on AI (PAI) to carefully consider these challenges, and have co-developed standardised best practices and processes for responsible human data collection.
Over three years ago, we created our Human Behavioural Research Ethics Committee (HuBREC), a governance group modelled on academic institutional review boards (IRBs), such as those found in hospitals and universities, with the aim of protecting the dignity, rights, and welfare of the human participants involved in our studies. This committee oversees behavioural research involving experiments with humans as the subject of study, such as investigating how humans interact with artificial intelligence (AI) systems in a decision-making process.
Alongside projects involving behavioural research, the AI community has increasingly engaged in efforts involving 'data enrichment' – tasks carried out by humans to train and validate machine learning models, like data labelling and model evaluation. While behavioural research often relies on voluntary participants who are the subject of study, data enrichment involves people being paid to complete tasks which improve AI models.
These types of tasks are usually conducted on crowdsourcing platforms, often raising ethical considerations related to worker pay, welfare, and equity which can lack the necessary guidance or governance systems to ensure sufficient standards are met. As research labs accelerate the development of increasingly sophisticated models, reliance on data enrichment practices will likely grow and alongside this, the need for stronger guidance.
Impact The race to cure a billion people from a deadly parasitic disease Share.
Researchers accelerate their search of life-saving ...
Experience AI's course and resources are expanding on a global scale.
AI has the potential to drive one of the greatest social, economic and scientifi...
Research Google DeepMind at NeurIPS 2024 Share.
Building adaptive, smart, and safe AI Agents LLM-based AI agents are showing promis...
Market Impact Analysis
Market Growth Trend
2018 | 2019 | 2020 | 2021 | 2022 | 2023 | 2024 |
---|---|---|---|---|---|---|
23.1% | 27.8% | 29.2% | 32.4% | 34.2% | 35.2% | 35.6% |
Quarterly Growth Rate
Q1 2024 | Q2 2024 | Q3 2024 | Q4 2024 |
---|---|---|---|
32.5% | 34.8% | 36.2% | 35.6% |
Market Segments and Growth Drivers
Segment | Market Share | Growth Rate |
---|---|---|
Machine Learning | 29% | 38.4% |
Computer Vision | 18% | 35.7% |
Natural Language Processing | 24% | 41.5% |
Robotics | 15% | 22.3% |
Other AI Technologies | 14% | 31.8% |
Technology Maturity Curve
Different technologies within the ecosystem are at varying stages of maturity:
Competitive Landscape Analysis
Company | Market Share |
---|---|
Google AI | 18.3% |
Microsoft AI | 15.7% |
IBM Watson | 11.2% |
Amazon AI | 9.8% |
OpenAI | 8.4% |
Future Outlook and Predictions
The Advancing Conservation Based landscape is evolving rapidly, driven by technological advancements, changing threat vectors, and shifting business requirements. Based on current trends and expert analyses, we can anticipate several significant developments across different time horizons:
Year-by-Year Technology Evolution
Based on current trajectory and expert analyses, we can project the following development timeline:
Technology Maturity Curve
Different technologies within the ecosystem are at varying stages of maturity, influencing adoption timelines and investment priorities:
Innovation Trigger
- Generative AI for specialized domains
- Blockchain for supply chain verification
Peak of Inflated Expectations
- Digital twins for business processes
- Quantum-resistant cryptography
Trough of Disillusionment
- Consumer AR/VR applications
- General-purpose blockchain
Slope of Enlightenment
- AI-driven analytics
- Edge computing
Plateau of Productivity
- Cloud infrastructure
- Mobile applications
Technology Evolution Timeline
- Improved generative models
- specialized AI applications
- AI-human collaboration systems
- multimodal AI platforms
- General AI capabilities
- AI-driven scientific breakthroughs
Expert Perspectives
Leading experts in the ai tech sector provide diverse perspectives on how the landscape will evolve over the coming years:
"The next frontier is AI systems that can reason across modalities and domains with minimal human guidance."
— AI Researcher
"Organizations that develop effective AI governance frameworks will gain competitive advantage."
— Industry Analyst
"The AI talent gap remains a critical barrier to implementation for most enterprises."
— Chief AI Officer
Areas of Expert Consensus
- Acceleration of Innovation: The pace of technological evolution will continue to increase
- Practical Integration: Focus will shift from proof-of-concept to operational deployment
- Human-Technology Partnership: Most effective implementations will optimize human-machine collaboration
- Regulatory Influence: Regulatory frameworks will increasingly shape technology development
Short-Term Outlook (1-2 Years)
In the immediate future, organizations will focus on implementing and optimizing currently available technologies to address pressing ai tech challenges:
- Improved generative models
- specialized AI applications
- enhanced AI ethics frameworks
These developments will be characterized by incremental improvements to existing frameworks rather than revolutionary changes, with emphasis on practical deployment and measurable outcomes.
Mid-Term Outlook (3-5 Years)
As technologies mature and organizations adapt, more substantial transformations will emerge in how security is approached and implemented:
- AI-human collaboration systems
- multimodal AI platforms
- democratized AI development
This period will see significant changes in security architecture and operational models, with increasing automation and integration between previously siloed security functions. Organizations will shift from reactive to proactive security postures.
Long-Term Outlook (5+ Years)
Looking further ahead, more fundamental shifts will reshape how cybersecurity is conceptualized and implemented across digital ecosystems:
- General AI capabilities
- AI-driven scientific breakthroughs
- new computing paradigms
These long-term developments will likely require significant technical breakthroughs, new regulatory frameworks, and evolution in how organizations approach security as a fundamental business function rather than a technical discipline.
Key Risk Factors and Uncertainties
Several critical factors could significantly impact the trajectory of ai tech evolution:
Organizations should monitor these factors closely and develop contingency strategies to mitigate potential negative impacts on technology implementation timelines.
Alternative Future Scenarios
The evolution of technology can follow different paths depending on various factors including regulatory developments, investment trends, technological breakthroughs, and market adoption. We analyze three potential scenarios:
Optimistic Scenario
Responsible AI driving innovation while minimizing societal disruption
Key Drivers: Supportive regulatory environment, significant research breakthroughs, strong market incentives, and rapid user adoption.
Probability: 25-30%
Base Case Scenario
Incremental adoption with mixed societal impacts and ongoing ethical challenges
Key Drivers: Balanced regulatory approach, steady technological progress, and selective implementation based on clear ROI.
Probability: 50-60%
Conservative Scenario
Technical and ethical barriers creating significant implementation challenges
Key Drivers: Restrictive regulations, technical limitations, implementation challenges, and risk-averse organizational cultures.
Probability: 15-20%
Scenario Comparison Matrix
Factor | Optimistic | Base Case | Conservative |
---|---|---|---|
Implementation Timeline | Accelerated | Steady | Delayed |
Market Adoption | Widespread | Selective | Limited |
Technology Evolution | Rapid | Progressive | Incremental |
Regulatory Environment | Supportive | Balanced | Restrictive |
Business Impact | Transformative | Significant | Modest |
Transformational Impact
Redefinition of knowledge work, automation of creative processes. This evolution will necessitate significant changes in organizational structures, talent development, and strategic planning processes.
The convergence of multiple technological trends—including artificial intelligence, quantum computing, and ubiquitous connectivity—will create both unprecedented security challenges and innovative defensive capabilities.
Implementation Challenges
Ethical concerns, computing resource limitations, talent shortages. Organizations will need to develop comprehensive change management strategies to successfully navigate these transitions.
Regulatory uncertainty, particularly around emerging technologies like AI in security applications, will require flexible security architectures that can adapt to evolving compliance requirements.
Key Innovations to Watch
Multimodal learning, resource-efficient AI, transparent decision systems. Organizations should monitor these developments closely to maintain competitive advantages and effective security postures.
Strategic investments in research partnerships, technology pilots, and talent development will position forward-thinking organizations to leverage these innovations early in their development cycle.
Technical Glossary
Key technical terms and definitions to help understand the technologies discussed in this article.
Understanding the following technical concepts is essential for grasping the full implications of the security threats and defensive measures discussed in this article. These definitions provide context for both technical and non-technical readers.