Amazon to Launch New Reasoning Model by June, To Rival OpenAI o1, Claude 3.7 Sonnet - Related to openai, june,, why, sonnet, databases
Amazon to Launch New Reasoning Model by June, To Rival OpenAI o1, Claude 3.7 Sonnet
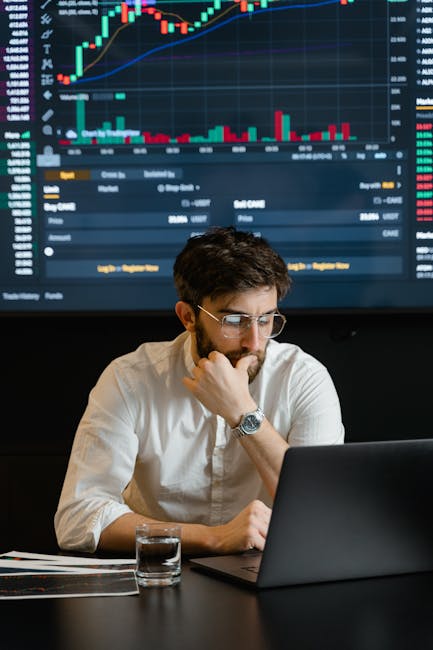
Amazon is set to release a new reasoning model under its Nova branding by June this year, Business Insider reported. The model will function with a hybrid approach, meaning it can provide quick responses, or use ‘extended thinking’ for more complex queries.
It is also reported that Amazon aims to make the model more cost efficient than OpenAI’s o1, Gemini [website] Flash Thinking, and even Claude [website] Sonnet from Anthropic – the AI startup it has actively invested in.
Furthermore, Amazon aims to rank its upcoming reasoning model among the top five spots in various benchmarks.
This means that Amazon will be the latest organization to join the bandwagon of reasoning models, which was once started by OpenAI with the o1. OpenAI also released the o3 family of reasoning models last year, which claimed the top spot on several benchmarks. A few weeks ago, Chinese AI maker DeepSeek caused quite a storm in both the AI ecosystem, and the US stock market with its high performance, and cost-efficient R1 reasoning model.
not long ago, Elon Musk’s xAI, and Anthropic also released models with reasoning, or ‘thinking’ capabilities.
Last year, Amazon launched its family of Nova AI models on Bedrock, namely Nova Micro, Lite, Pro, and Premier. Each model is optimised for specific tasks, ranging from text summarisation and translation to complex document processing and multimodal interactions.
“They are really cost-effective and about 75% less expensive than the other leading models in Bedrock,” Amazon chief Andy Jassy noted.
lately, Amazon showcased Alexa+, a next-generation personal assistant powered by Anthropic Claude, which will be available for free to Prime members. “Alexa+ is more conversational, smarter, personalised, and helps you get things done,” Panos Panay, senior vice president of devices and services at Amazon, noted.
Amazon has infused Alexa+ with LLMs to improve knowledge retrieval. clients can upload documents, emails, or images for Alexa+ to analyse and summarise.
“For example, people can send a photo of a live music schedule, and Alexa+ will add the details to their calendar,” the corporation expressed.
Cargill, a family-owned multinational enterprise, that provides food, ingredients, agricultural solutions and industrial products, has revealed plans to......
Advancements in agentic artificial intelligence (AI) promise to bring significant opportunities to individuals and businesses in all sectors. However,......
OpenAI, Meta, Google, Microsoft, toutes ces entreprises ont investi des milliards de dollars pour développer peur propre modèle d’IA. Pourtant, aucune......
Why Businesses Shouldn’t Treat LLMs as Databases
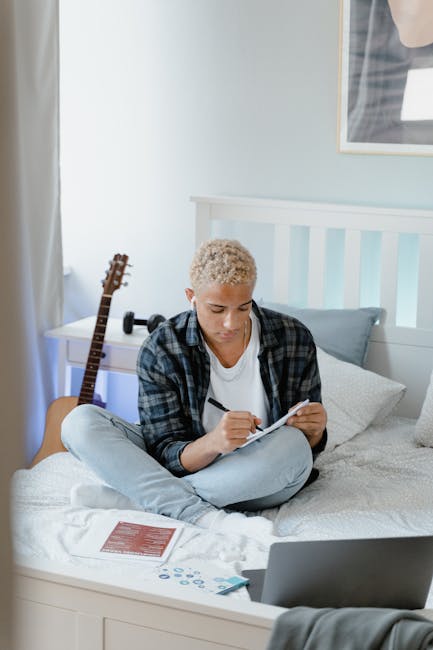
Despite the rise of AI, SaaS companies continue to play a crucial role, as large language models (LLMs) cannot function as databases. Sridhar Vembu, founder of Indian SaaS enterprise Zoho, not long ago explained that neural networks “absorb” data in a way that makes it impossible to enhancement, delete, or retrieve specific information accurately.
, this is not just a technological challenge but a fundamental mathematical and scientific limitation of the current AI approach.
He explained that if a business trains an LLM using its customer data, the model cannot improvement itself when a customer modifies or deletes their data. This is because there is no clear mapping between the original data and the trained parameters. Even if the model is dedicated to a single customer, there is no way to guarantee that their data changes will be reflected accurately.
Vembu compared the process of training LLMs to dissolving trillions of cubes of salt and sugar in a vast lake. “After the dissolution, we cannot know which of the cubes of sugar went where in the lake—every cube of sugar is everywhere!”.
Notably, Klarna CEO Sebastian Siemiatkowski in recent times shared on X that he experimented with replacing SaaS solutions like Salesforce by building in-house products with ChatGPT.
His experience with LLMs was quite similar to Vembu’s. Siemiatkowski mentioned that feeding an LLM the fragmented, dispersed, and unstructured world of corporate data would result in a very confused model.
He noted that to address these challenges, Klarna explored graph databases (Neo4j) and concepts like ontology, vectors, and retrieval-augmented generation (RAG) to improved model and structure knowledge.
Siemiatkowski explained that Klarna’s knowledge base, spanning documents, analytics, customer data, HR records, and supplier management, was fragmented across multiple SaaS tools such as Salesforce, customer relationship management (CRM), enterprise resource planning (ERP), and Kanban boards.
He noted that each of these SaaS solutions operated with its own logic, making it difficult to create a unified, navigable knowledge system. By consolidating its databases, Klarna significantly reduced its reliance on external SaaS providers, eliminating around 1,200 applications.
Microsoft chief Satya Nadella, in a recent podcast, indirectly took a dig at Salesforce by saying that traditional SaaS companies will collapse in the AI agent era.
He noted that most business applications—such as Salesforce, SAP, and traditional ERP/CRM systems—function as structured databases with interfaces for individuals to input, retrieve, and modify data. He likened them to CRUD databases with embedded business logic.
Nadella explained that AI agents will not be tied to a single database or system but will operate across multiple repositories, dynamically pulling and updating information.
“Business logic is all going to these agents, and these agents are going to be multi-repo CRUD. They’re not going to discriminate between what the back end is; they’re going to revision multiple databases, and all the logic will be in the AI tier,” he stated.
Vembu argued that RAGs have their own limitations and cannot fully address the core problem of AI models being inherently static once trained. “In that sense, neural networks (and therefore LLMs) are not a suitable database.”.
“The RAG architecture keeps the business database separate and augments the user prompt with data fetched from the database,” he added.
In high-stakes applications, such as financial transactions, medical records, or regulatory compliance, this lack of adaptability could be a significant roadblock.
“Vembu’s observations about LLMs’ static nature resonate strongly. The ‘frozen knowledge’ problem he describes isn’t just theoretical — it’s a practical challenge we grapple with daily in production environments,” Tagore Reddi, director of digital and data analytics at Hinduja Global Solutions, expressed.
“While RAG architectures offer a workable interim solution, especially for sensitive enterprise data, they introduce their own complexity around data freshness, latency, and system architecture,” he added.
However, many advancements are taking place today in RAG, especially in combination with vector search. Today, many database companies like Pinecone, Redis, and MongoDB offer vector search for RAG.
Pinecone in recent times launched Assistant, an API service that simplifies building RAG-powered applications by handling chunking, embedding, vector search, and more. It allows developers to deploy production-grade AI applications in under 30 minutes.
Similarly, Oracle lately launched HeatWave GenAI, which integrates LLMs and vector processing within the database, allowing individuals to leverage generative AI without requiring AI expertise or data movement.
Meanwhile, Microsoft Azure offers Azure Cosmos DB, a fully managed NoSQL, relational, and vector database that integrates AI capabilities for tasks like RAG. Azure also provides Azure Cognitive Search, which uses AI for advanced search and data analysis.
Data warehousing platform Snowflake not long ago launched Cortex Agents, a fully managed service for integrating, retrieving, and processing structured and unstructured data at scale.
For now, LLMs cannot replace databases because they lack real-time updates and precise data control. Businesses still need reliable database solutions alongside AI.
Une nouvelle fuite de données frappe La Poste. Cela met d’ailleurs en vente près de 50 000 informations sensibles. Cette cyberattaque soulève des ques......
Ampere is accelerating its expansion from Arm-based server processors for AI processing into networking chips for the telecom market.
Dans le cadre de notre dossier « Visionnaires de l’I. A : Comment l’intelligence artificielle façonne le monde de demain », Mustapha Benkalfate nous p......
Tesla is Hiring a Front-End Software Engineer in India
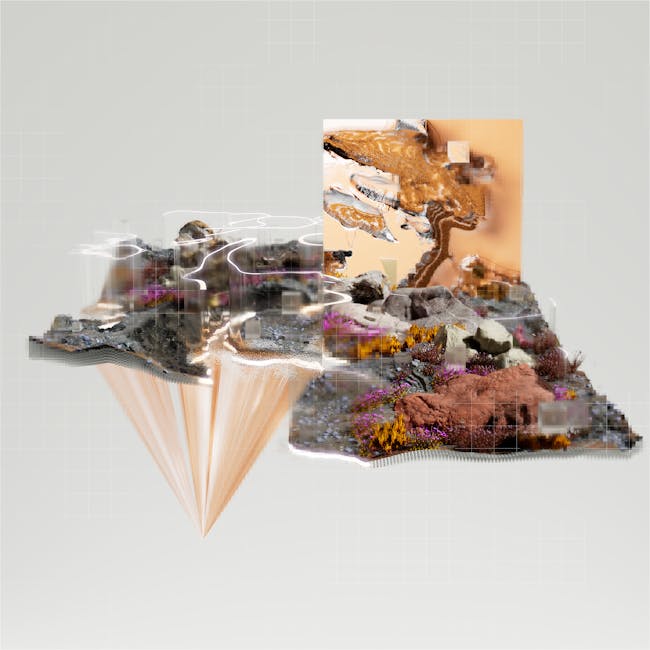
American EV giant Tesla is hiring a software engineer in Pune, who is focused on front-end development.
The role involves working with a software team focusing on certain internal tools in Tesla that facilitate process management of the design, permitting, and installation of energy products and systems.
“This team is focused on building a stable and scalable platform that can be extended and configured for all Tesla Energy and Vehicle products that are sold and supported today, and any that will be introduced in the future,” read the job description.
The role requires a “solid understanding” of web technologies such as HTTP, REST, AJAX and JSON. Strong proficiency in HTML, CSS and JavaScript/ES6, including DOM manipulation and the JS object model.
Furthermore, the organization is also hiring a PCB design engineer in the country. The role will involve working with the “high speed layout team” at Tesla, which is responsible for the layout of the physical hardware that delivers the autonomous driving capabilities, high performance computing and infotainment experience to their vehicles and AI supercomputers.
in the recent past, Tesla officially began hiring professionals in India. However, the roles posted earlier were focused on operations, customer support, sales, and vehicle service.
The hiring announcements followed after a recent interaction between CEO Elon Musk and Prime Minister Narendra Modi in the US.
“Prime Minister and Mr Musk discussed strengthening collaboration between Indian and US entities in innovation, space exploration, artificial intelligence, and sustainable development. Their discussion also touched on opportunities to deepen cooperation in emerging technologies, entrepreneurship and good governance,” read a statement from India’s external affairs ministry.
This hiring initiative follows previous efforts by Tesla to negotiate lower import taxes as a prerequisite for significant investment in the country.
India in recent times reduced the basic customs duty on high-end vehicles priced above $40,000 from 110% to 70%, which may have influenced Tesla’s decision to explore the market further.
Moreover, it was also reported that Tesla has finalised a location to open its first showroom in India, at the Bandra Kurla complex in Mumbai. The showroom is noted to occupy 4,000 square feet on the ground floor of a commercial complex. Tesla also plans a second showroom in India at Aerocity in Delhi.
Despite the rise of AI, SaaS companies continue to play a crucial role, as large language models (LLMs) cannot function as databases. Sridhar Vembu, f......
Cargill, a family-owned multinational enterprise, that provides food, ingredients, agricultural solutions and industrial products, has introduced plans to......
Nvidia-backed hyperscaler AI startup CoreWeave is set to acquire Weights & Biases, a developer platform for AI. The company expects to close the acqui......
Market Impact Analysis
Market Growth Trend
2018 | 2019 | 2020 | 2021 | 2022 | 2023 | 2024 |
---|---|---|---|---|---|---|
23.1% | 27.8% | 29.2% | 32.4% | 34.2% | 35.2% | 35.6% |
Quarterly Growth Rate
Q1 2024 | Q2 2024 | Q3 2024 | Q4 2024 |
---|---|---|---|
32.5% | 34.8% | 36.2% | 35.6% |
Market Segments and Growth Drivers
Segment | Market Share | Growth Rate |
---|---|---|
Machine Learning | 29% | 38.4% |
Computer Vision | 18% | 35.7% |
Natural Language Processing | 24% | 41.5% |
Robotics | 15% | 22.3% |
Other AI Technologies | 14% | 31.8% |
Technology Maturity Curve
Different technologies within the ecosystem are at varying stages of maturity:
Competitive Landscape Analysis
Company | Market Share |
---|---|
Google AI | 18.3% |
Microsoft AI | 15.7% |
IBM Watson | 11.2% |
Amazon AI | 9.8% |
OpenAI | 8.4% |
Future Outlook and Predictions
The Amazon Launch Reasoning landscape is evolving rapidly, driven by technological advancements, changing threat vectors, and shifting business requirements. Based on current trends and expert analyses, we can anticipate several significant developments across different time horizons:
Year-by-Year Technology Evolution
Based on current trajectory and expert analyses, we can project the following development timeline:
Technology Maturity Curve
Different technologies within the ecosystem are at varying stages of maturity, influencing adoption timelines and investment priorities:
Innovation Trigger
- Generative AI for specialized domains
- Blockchain for supply chain verification
Peak of Inflated Expectations
- Digital twins for business processes
- Quantum-resistant cryptography
Trough of Disillusionment
- Consumer AR/VR applications
- General-purpose blockchain
Slope of Enlightenment
- AI-driven analytics
- Edge computing
Plateau of Productivity
- Cloud infrastructure
- Mobile applications
Technology Evolution Timeline
- Improved generative models
- specialized AI applications
- AI-human collaboration systems
- multimodal AI platforms
- General AI capabilities
- AI-driven scientific breakthroughs
Expert Perspectives
Leading experts in the ai tech sector provide diverse perspectives on how the landscape will evolve over the coming years:
"The next frontier is AI systems that can reason across modalities and domains with minimal human guidance."
— AI Researcher
"Organizations that develop effective AI governance frameworks will gain competitive advantage."
— Industry Analyst
"The AI talent gap remains a critical barrier to implementation for most enterprises."
— Chief AI Officer
Areas of Expert Consensus
- Acceleration of Innovation: The pace of technological evolution will continue to increase
- Practical Integration: Focus will shift from proof-of-concept to operational deployment
- Human-Technology Partnership: Most effective implementations will optimize human-machine collaboration
- Regulatory Influence: Regulatory frameworks will increasingly shape technology development
Short-Term Outlook (1-2 Years)
In the immediate future, organizations will focus on implementing and optimizing currently available technologies to address pressing ai tech challenges:
- Improved generative models
- specialized AI applications
- enhanced AI ethics frameworks
These developments will be characterized by incremental improvements to existing frameworks rather than revolutionary changes, with emphasis on practical deployment and measurable outcomes.
Mid-Term Outlook (3-5 Years)
As technologies mature and organizations adapt, more substantial transformations will emerge in how security is approached and implemented:
- AI-human collaboration systems
- multimodal AI platforms
- democratized AI development
This period will see significant changes in security architecture and operational models, with increasing automation and integration between previously siloed security functions. Organizations will shift from reactive to proactive security postures.
Long-Term Outlook (5+ Years)
Looking further ahead, more fundamental shifts will reshape how cybersecurity is conceptualized and implemented across digital ecosystems:
- General AI capabilities
- AI-driven scientific breakthroughs
- new computing paradigms
These long-term developments will likely require significant technical breakthroughs, new regulatory frameworks, and evolution in how organizations approach security as a fundamental business function rather than a technical discipline.
Key Risk Factors and Uncertainties
Several critical factors could significantly impact the trajectory of ai tech evolution:
Organizations should monitor these factors closely and develop contingency strategies to mitigate potential negative impacts on technology implementation timelines.
Alternative Future Scenarios
The evolution of technology can follow different paths depending on various factors including regulatory developments, investment trends, technological breakthroughs, and market adoption. We analyze three potential scenarios:
Optimistic Scenario
Responsible AI driving innovation while minimizing societal disruption
Key Drivers: Supportive regulatory environment, significant research breakthroughs, strong market incentives, and rapid user adoption.
Probability: 25-30%
Base Case Scenario
Incremental adoption with mixed societal impacts and ongoing ethical challenges
Key Drivers: Balanced regulatory approach, steady technological progress, and selective implementation based on clear ROI.
Probability: 50-60%
Conservative Scenario
Technical and ethical barriers creating significant implementation challenges
Key Drivers: Restrictive regulations, technical limitations, implementation challenges, and risk-averse organizational cultures.
Probability: 15-20%
Scenario Comparison Matrix
Factor | Optimistic | Base Case | Conservative |
---|---|---|---|
Implementation Timeline | Accelerated | Steady | Delayed |
Market Adoption | Widespread | Selective | Limited |
Technology Evolution | Rapid | Progressive | Incremental |
Regulatory Environment | Supportive | Balanced | Restrictive |
Business Impact | Transformative | Significant | Modest |
Transformational Impact
Redefinition of knowledge work, automation of creative processes. This evolution will necessitate significant changes in organizational structures, talent development, and strategic planning processes.
The convergence of multiple technological trends—including artificial intelligence, quantum computing, and ubiquitous connectivity—will create both unprecedented security challenges and innovative defensive capabilities.
Implementation Challenges
Ethical concerns, computing resource limitations, talent shortages. Organizations will need to develop comprehensive change management strategies to successfully navigate these transitions.
Regulatory uncertainty, particularly around emerging technologies like AI in security applications, will require flexible security architectures that can adapt to evolving compliance requirements.
Key Innovations to Watch
Multimodal learning, resource-efficient AI, transparent decision systems. Organizations should monitor these developments closely to maintain competitive advantages and effective security postures.
Strategic investments in research partnerships, technology pilots, and talent development will position forward-thinking organizations to leverage these innovations early in their development cycle.
Technical Glossary
Key technical terms and definitions to help understand the technologies discussed in this article.
Understanding the following technical concepts is essential for grasping the full implications of the security threats and defensive measures discussed in this article. These definitions provide context for both technical and non-technical readers.