New Robotics Method AnyPlace Achieves Object Placement Through VLMs, Synthetic Data - Related to data, vlms,, china’s, processor, literature
China’s Zuchongzhi 3.0 Quantum Processor ‘Outpaces’ Google Willow by Million Times
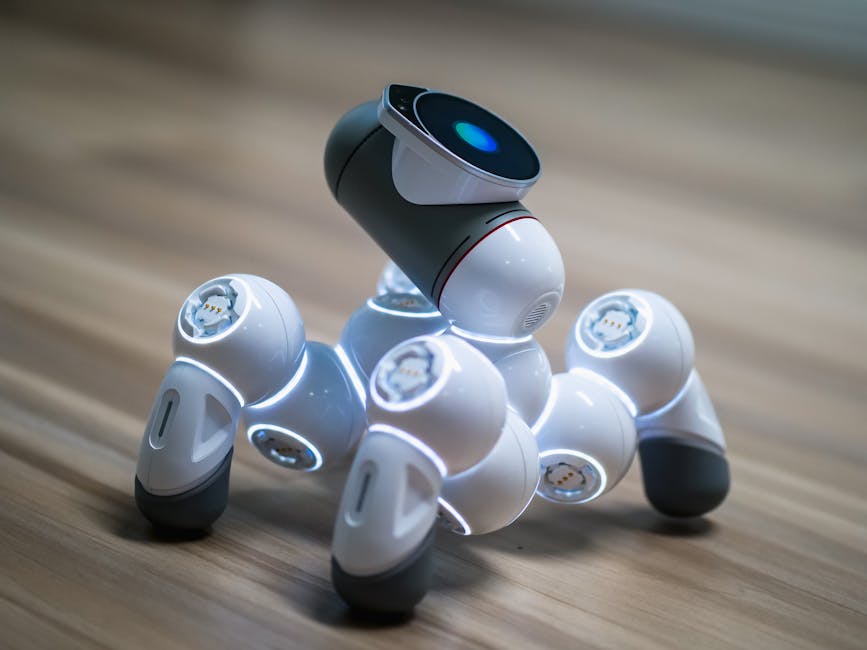
Chinese researchers from the University of Science and Technology of China (USTC) have unveiled the Zuchongzhi [website], a superconducting quantum processor with 105 qubits, marking a significant milestone in Chinese quantum computing.
The processor operates quadrillion times faster than the world’s fastest supercomputer and one million times faster than Google’s latest Willow, as per the findings .
The Zuchongzhi [website] was tested with an 83-qubit, 32-layer random circuit sampling task, claiming to achieve results that would take classical supercomputers over [website] billion years to replicate.
This achievement surpasses Google’s Sycamore processor, developed in 2019, by six orders of magnitude. The research team highlighted advancements in coherence time, gate fidelities, and readout accuracy.
The processor achieves a coherence time of 72 microseconds, single-qubit gate fidelity of [website], two-qubit gate fidelity of [website], and readout fidelity of [website] These improvements enable more complex operations and computations.
Building on previous successes with the Zuchongzhi-2 and Jiuzhang photonic systems, the team continues to push the boundaries of quantum error correction and scalability. They are researching surface codes for error correction and plan to expand integration capabilities to distances of 9 and 11.
The research team collaborated with institutions such as the Shanghai Research Center for Quantum Sciences and the Chinese Academy of Sciences.
In December last year, China also achieved a milestone in quantum computing with the launch of the ‘Tianyan-504’ superconducting quantum computer, equipped with the 504-qubit ‘Xiaohong’ chip.
The quantum computer, developed collaboratively by the China Telecom Quantum Group (CTQG), the Chinese Academy of Sciences (CAS), and QuantumCTek Co., Ltd., represented a leap in the field by surpassing the 500-qubit mark.
OpenAI has revealed NextGenAI, a new consortium with 15 leading research institutions to accelerate AI-driven breakthroughs in research and education......
American EV giant Tesla is hiring a software engineer in Pune, who is focused on front-end development.
The role involves working with a software tea......
“Conduct a comprehensive literature review on the state-of-the-art in Machine Learning and energy consumption. […]”.
Deep Research by OpenAI: A Practical Test of AI-Powered Literature Review
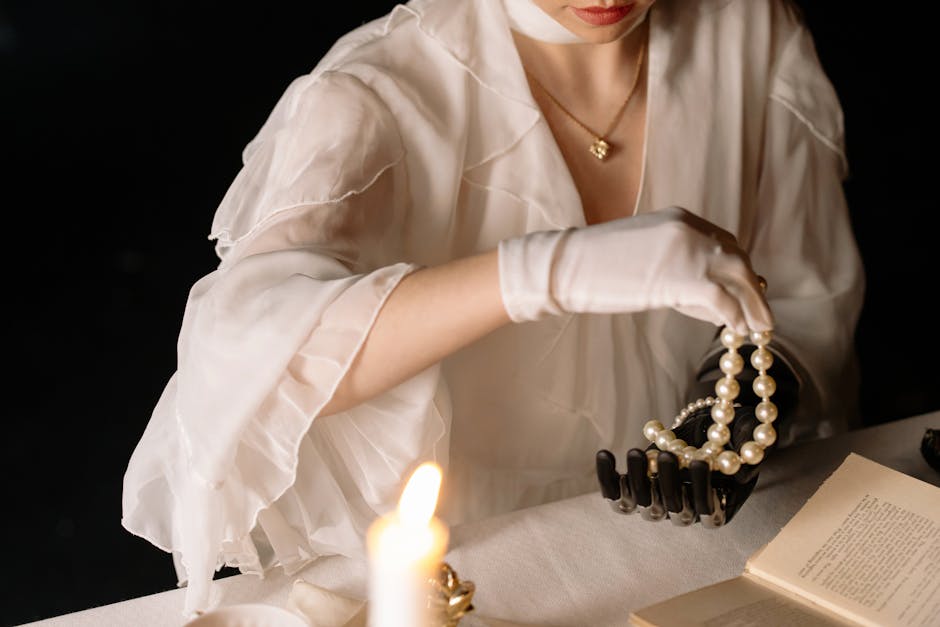
“Conduct a comprehensive literature review on the state-of-the-art in Machine Learning and energy consumption. […]”.
With this prompt, I tested the new Deep Research function, which has been integrated into the OpenAI o3 reasoning model since the end of February — and conducted a state-of-the-art literature review within 6 minutes.
This function goes beyond a normal web search (for example, with ChatGPT 4o): The research query is broken down & structured, the Internet is searched for information, which is then evaluated, and finally, a structured, comprehensive report is created.
1. What is Deep Research from OpenAI and what can you do with it?
If you have an OpenAI Plus account (the $20 per month plan), you have access to Deep Research. This gives you access to 10 queries per month. With the Pro subscription ($200 per month) you have extended access to Deep Research and access to the research preview of [website] with 120 queries per month.
OpenAI promises that we can perform multi-step research using data from the public web.
Duration: 5 to 30 minutes, depending on complexity.
Previously, such research usually took hours.
It is intended for complex tasks that require a deep search and thoroughness.
Conduct a literature review: Conduct a literature review on state-of-the-art machine learning and energy consumption.
Market analysis: Create a comparative findings on the best marketing automation platforms for companies in 2025 based on current market trends and evaluations.
Technology & software development: Investigate programming languages and frameworks for AI application development with performance and use case analysis.
Investment & financial analysis: Conduct research on the impact of AI-powered trading on the financial market based on recent reports and academic studies.
Legal research: Conduct an overview of data protection laws in Europe compared to the US, including relevant rulings and recent changes.
Deep Research uses various Deep Learning methods to carry out a systematic and detailed analysis of information. The entire process can be divided into four main phases:
1. Decomposition and structuring of the research question.
In the first step the tool processes the research question using natural language processing (NLP) methods. It identifies the most key key terms, concepts, and sub-questions.
This step ensures that the AI understands the question not only literally, but also in terms of content.
Once the tool has structured the research question, it searches specifically for information. Deep Research uses a mixture of internal databases, scientific publications, APIs, and web scraping. These can be open-access databases such as arXiv, PubMed, or Semantic Scholar, for example, but also public websites or news sites such as The Guardian, New York Times, or BBC. In the end, any content that can be accessed online and is publicly available.
3. Analysis & interpretation of the data.
The next step is for the AI model to summarize large amounts of text into compact and understandable answers. Transformers & Attention mechanisms ensure that the most important information is prioritized. This means that it does not simply create a summary of all the content found. Also, the quality and credibility of the sources is assessed. And cross-validation methods are normally used to identify incorrect or contradictory information. Here, the AI tool compares several sources with each other. However, it is not publicly known exactly how this is done in Deep Research or what criteria there are for this.
Finally, the final investigation is generated and displayed to us. This is done using Natural Language Generation (NLG) so that we see easily readable texts.
The AI system generates diagrams or tables if requested in the prompt and adapts the response to the user’s style. The primary data used are also listed at the end of the analysis.
3. How you can use Deep Research: A practical example.
In the first step, it is best to use one of the standard models to ask how you should optimize the prompt in order to conduct deep research. I have done this with the following prompt with ChatGPT 4o:
“Optimize this prompt to conduct a deep research:
Carrying out a literature search: Carry out a literature search on the state of the art on machine learning and energy consumption.”.
The 4o model suggested the following prompt for the Deep Research function:
The tool then asked me if I could clarify the scope and focus of the literature review. I have, therefore, provided some additional specifications:
ChatGPT then returned the clarification and started the research.
In the meantime, I could see the progress and how more reports were gradually added.
After 6 minutes, the state-of-the-art literature review was complete, and the study, including all information, was available to me.
4. Challenges and risks of the Deep Research feature.
Let’s take a look at two definitions of research:
“A detailed study of a subject, especially in order to discover new information or reach a new understanding.” Reference: Cambridge Dictionary.
“Research is creative and systematic work undertaken to increase the stock of knowledge. It involves the collection, organization, and analysis of evidence to increase understanding of a topic, characterized by a particular attentiveness to controlling information of bias and error.” Reference: Wikipedia Research.
The two definitions show that research is a detailed, systematic investigation of a topic — with the aim of discovering new information or achieving a deeper understanding.
Basically, the deep research function fulfills these definitions to a certain extent: it collects existing information, analyzes it, and presents it in a structured way.
However, I think we also need to be aware of some challenges and risks:
Danger of superficiality : Deep Research is primarily designed to efficiently search, summarize, and provide existing information in a structured form (at least at the current stage). Absolutely great for overview research. But what about digging deeper? Real scientific research goes beyond mere reproduction and takes a critical look at the insights. Science also thrives on generating new knowledge.
: Deep Research is primarily designed to efficiently search, summarize, and provide existing information in a structured form (at least at the current stage). Absolutely great for overview research. But what about digging deeper? Real scientific research goes beyond mere reproduction and takes a critical look at the sources. Science also thrives on generating new knowledge. Reinforcement of existing biases in research & publication : Papers are already more likely to be . “Non-significant” or contradictory results, on the other hand, are less likely to be published. This is known to us as publication bias. If the AI tool now primarily evaluates frequently cited papers, it reinforces this trend. Rare or less widespread but possibly important findings are lost. A possible solution here would be to implement a mechanism for weighted source evaluation that also takes into account less cited but relevant papers. If the AI methods primarily cite sources that are quoted frequently, less widespread but important findings may be lost. Presumably, this effect also applies to us humans.
: Papers are already more likely to be . “Non-significant” or contradictory results, on the other hand, are less likely to be published. This is known to us as publication bias. If the AI tool now primarily evaluates frequently cited papers, it reinforces this trend. Rare or less widespread but possibly critical findings are lost. A possible solution here would be to implement a mechanism for weighted source evaluation that also takes into account less cited but relevant papers. If the AI methods primarily cite information that are quoted frequently, less widespread but critical findings may be lost. Presumably, this effect also applies to us humans. Quality of research papers: While it is obvious that a bachelor’s, master’s, or doctoral thesis cannot be based solely on AI-generated research, the question I have is how universities or scientific institutions deal with this development. Students can get a solid research study with just a single prompt. Presumably, the solution here must be to adapt assessment criteria to give greater weight to in-depth reflection and methodology.
In addition to OpenAI, other companies and platforms have also integrated similar functions (even before OpenAI): For example, Perplexity AI has introduced a deep research function that independently conducts and analyzes searches. Also Gemini by Google has integrated such a deep research function.
The function gives you an incredibly quick overview of an initial research question. It remains to be seen how reliable the results are. Currently (beginning March 2025), OpenAI itself writes as limitations that the feature is still at an early stage, can sometimes hallucinate facts into answers or draw false conclusions, and has trouble distinguishing authoritative information from rumors. In addition, it is currently unable to accurately convey uncertainties.
But it can be assumed that this function will be expanded further and become a powerful tool for research. If you have simpler questions, it is improved to use the standard GPT-4o model (with or without search), where you get an immediate answer.
Want more tips & tricks about tech, Python, data science, data engineering, machine learning and AI? Then regularly receive a summary of my most-read articles on my Substack — curated and for free.
Ola is making a significant push into AI by developing Krutrim 3, a 700-billion parameter AI model, in partnership with Lenovo, the business showcased ......
Chinese researchers from the University of Science and Technology of China (USTC) have unveiled the Zuchongzhi [website], a superconducting quantum processo......
ZDNET's key takeaways The Aqara Camera Hub G5 Pro is available for $180 for the Wi-Fi version and $200 for the PoE version.
New Robotics Method AnyPlace Achieves Object Placement Through VLMs, Synthetic Data
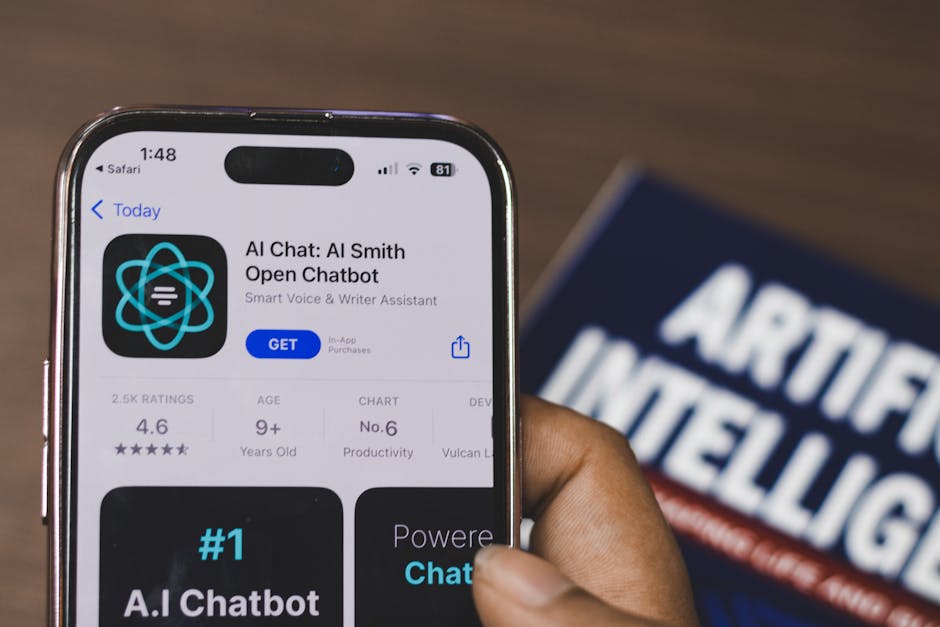
Researchers have introduced a new two-stage method for robotic object placement called AnyPlace, which demonstrates the ability to predict feasible placement poses. This advancement addresses the challenges of object placement, which is often difficult due to variations in object shapes and placement arrangements.
, one of the researchers from Georgia Institute of Technology, the work addresses the challenge of robot placement, focusing on the generalisability of solutions rather than domain-specific ones.
How can robots reliably place objects in diverse real-world tasks?
🤖🔍 Placement is tough—objects vary in shape and placement modes (such as stacking, hanging, and insertion), making it a challenging problem.
We introduce AnyPlace, a two-stage method trained purely on synthetic… [website] — Animesh Garg (@animesh_garg) February 24, 2025.
The system uses a vision language model (VLM) to produce potential placement locations, combined with depth-based models for geometric placement prediction.
“Our AnyPlace pipeline consists of two stages: high-level placement position prediction and low-level pose prediction,” the researcher paper stated.
The first stage uses Molmo, a VLM, and SAM 2, a large segmentation model, to segment objects and propose placement locations. Only the region around the proposed placement is fed into the low-level pose prediction model, which uses point clouds of objects to be placed and regions of placement locations.
Our key insight is that by leveraging a Vision-Language Model (VLM) to identify rough placement locations, we focus only on the relevant regions for local placement, which enables us to train the low-level placement-pose-prediction model to capture diverse placements efficiently. [website] — Animesh Garg (@animesh_garg) February 24, 2025.
The creators of AnyPlace have developed a fully synthetic dataset of 1,489 randomly generated objects, covering insertion, stacking, and hanging. In total, 13 categories were created, and 5,370 placement poses were generated, as per the paper.
This approach helps overcome limitations of real-world data collection, enabling the model to generalise across objects and scenarios.
Garg noted that for object placement, it is possible to generate highly effective synthetic data, allowing for the creation of a grasp predictor for any object using only synthetic data.
To generalize across objects & placements, we generate a fully synthetic dataset with:
✅ Diverse placement configurations (stacking, insertion, hanging) in IsaacSim.
This allows us to train our model without real-world data collection! 🚀 [website] — Animesh Garg (@animesh_garg) February 24, 2025.
“The use of depth data minimises the sim-to-real gap, making the model applicable in real-world scenarios with limited real-world data collection,” Garg noted. The synthetic data generation process creates variability in object shapes and sizes, improving the model’s robustness.
The model achieved an 80% success rate on the vial insertion task, showing robustness and generalisation. The simulation results outperform baselines in success rates, coverage of placement modes and fine-placement precision.
For real-world results, the method transfers directly from synthetic to real-world tasks, “succeeding where others struggle”.
🏆 Simulation results: Outperforms baselines in.
📌 Real-world results: Our method transfers directly from synthetic to real-world tasks, succeeding where others struggle! [website] — Animesh Garg (@animesh_garg) February 24, 2025.
Another lately released research introduces Phantom, a method to train robot policies without collecting any robot data and using only human video demonstrations.
Phantom turns human videos into “robot” demonstrations, making it significantly easier to scale up and diversify robotics data.
I have been a data team manager for six months, and my team has grown from three to five.
I wrote about my initial manager experiences back in Novemb......
As far back as 2023, Google was reportedly working on an AI assistant for Pixel phones called.
There are some Sql patterns that, once you know them, you start seeing them everywhere. The solutions to the puzzles that I will show you today are ac......
Market Impact Analysis
Market Growth Trend
2018 | 2019 | 2020 | 2021 | 2022 | 2023 | 2024 |
---|---|---|---|---|---|---|
23.1% | 27.8% | 29.2% | 32.4% | 34.2% | 35.2% | 35.6% |
Quarterly Growth Rate
Q1 2024 | Q2 2024 | Q3 2024 | Q4 2024 |
---|---|---|---|
32.5% | 34.8% | 36.2% | 35.6% |
Market Segments and Growth Drivers
Segment | Market Share | Growth Rate |
---|---|---|
Machine Learning | 29% | 38.4% |
Computer Vision | 18% | 35.7% |
Natural Language Processing | 24% | 41.5% |
Robotics | 15% | 22.3% |
Other AI Technologies | 14% | 31.8% |
Technology Maturity Curve
Different technologies within the ecosystem are at varying stages of maturity:
Competitive Landscape Analysis
Company | Market Share |
---|---|
Google AI | 18.3% |
Microsoft AI | 15.7% |
IBM Watson | 11.2% |
Amazon AI | 9.8% |
OpenAI | 8.4% |
Future Outlook and Predictions
The China Zuchongzhi Quantum landscape is evolving rapidly, driven by technological advancements, changing threat vectors, and shifting business requirements. Based on current trends and expert analyses, we can anticipate several significant developments across different time horizons:
Year-by-Year Technology Evolution
Based on current trajectory and expert analyses, we can project the following development timeline:
Technology Maturity Curve
Different technologies within the ecosystem are at varying stages of maturity, influencing adoption timelines and investment priorities:
Innovation Trigger
- Generative AI for specialized domains
- Blockchain for supply chain verification
Peak of Inflated Expectations
- Digital twins for business processes
- Quantum-resistant cryptography
Trough of Disillusionment
- Consumer AR/VR applications
- General-purpose blockchain
Slope of Enlightenment
- AI-driven analytics
- Edge computing
Plateau of Productivity
- Cloud infrastructure
- Mobile applications
Technology Evolution Timeline
- Improved generative models
- specialized AI applications
- AI-human collaboration systems
- multimodal AI platforms
- General AI capabilities
- AI-driven scientific breakthroughs
Expert Perspectives
Leading experts in the ai tech sector provide diverse perspectives on how the landscape will evolve over the coming years:
"The next frontier is AI systems that can reason across modalities and domains with minimal human guidance."
— AI Researcher
"Organizations that develop effective AI governance frameworks will gain competitive advantage."
— Industry Analyst
"The AI talent gap remains a critical barrier to implementation for most enterprises."
— Chief AI Officer
Areas of Expert Consensus
- Acceleration of Innovation: The pace of technological evolution will continue to increase
- Practical Integration: Focus will shift from proof-of-concept to operational deployment
- Human-Technology Partnership: Most effective implementations will optimize human-machine collaboration
- Regulatory Influence: Regulatory frameworks will increasingly shape technology development
Short-Term Outlook (1-2 Years)
In the immediate future, organizations will focus on implementing and optimizing currently available technologies to address pressing ai tech challenges:
- Improved generative models
- specialized AI applications
- enhanced AI ethics frameworks
These developments will be characterized by incremental improvements to existing frameworks rather than revolutionary changes, with emphasis on practical deployment and measurable outcomes.
Mid-Term Outlook (3-5 Years)
As technologies mature and organizations adapt, more substantial transformations will emerge in how security is approached and implemented:
- AI-human collaboration systems
- multimodal AI platforms
- democratized AI development
This period will see significant changes in security architecture and operational models, with increasing automation and integration between previously siloed security functions. Organizations will shift from reactive to proactive security postures.
Long-Term Outlook (5+ Years)
Looking further ahead, more fundamental shifts will reshape how cybersecurity is conceptualized and implemented across digital ecosystems:
- General AI capabilities
- AI-driven scientific breakthroughs
- new computing paradigms
These long-term developments will likely require significant technical breakthroughs, new regulatory frameworks, and evolution in how organizations approach security as a fundamental business function rather than a technical discipline.
Key Risk Factors and Uncertainties
Several critical factors could significantly impact the trajectory of ai tech evolution:
Organizations should monitor these factors closely and develop contingency strategies to mitigate potential negative impacts on technology implementation timelines.
Alternative Future Scenarios
The evolution of technology can follow different paths depending on various factors including regulatory developments, investment trends, technological breakthroughs, and market adoption. We analyze three potential scenarios:
Optimistic Scenario
Responsible AI driving innovation while minimizing societal disruption
Key Drivers: Supportive regulatory environment, significant research breakthroughs, strong market incentives, and rapid user adoption.
Probability: 25-30%
Base Case Scenario
Incremental adoption with mixed societal impacts and ongoing ethical challenges
Key Drivers: Balanced regulatory approach, steady technological progress, and selective implementation based on clear ROI.
Probability: 50-60%
Conservative Scenario
Technical and ethical barriers creating significant implementation challenges
Key Drivers: Restrictive regulations, technical limitations, implementation challenges, and risk-averse organizational cultures.
Probability: 15-20%
Scenario Comparison Matrix
Factor | Optimistic | Base Case | Conservative |
---|---|---|---|
Implementation Timeline | Accelerated | Steady | Delayed |
Market Adoption | Widespread | Selective | Limited |
Technology Evolution | Rapid | Progressive | Incremental |
Regulatory Environment | Supportive | Balanced | Restrictive |
Business Impact | Transformative | Significant | Modest |
Transformational Impact
Redefinition of knowledge work, automation of creative processes. This evolution will necessitate significant changes in organizational structures, talent development, and strategic planning processes.
The convergence of multiple technological trends—including artificial intelligence, quantum computing, and ubiquitous connectivity—will create both unprecedented security challenges and innovative defensive capabilities.
Implementation Challenges
Ethical concerns, computing resource limitations, talent shortages. Organizations will need to develop comprehensive change management strategies to successfully navigate these transitions.
Regulatory uncertainty, particularly around emerging technologies like AI in security applications, will require flexible security architectures that can adapt to evolving compliance requirements.
Key Innovations to Watch
Multimodal learning, resource-efficient AI, transparent decision systems. Organizations should monitor these developments closely to maintain competitive advantages and effective security postures.
Strategic investments in research partnerships, technology pilots, and talent development will position forward-thinking organizations to leverage these innovations early in their development cycle.
Technical Glossary
Key technical terms and definitions to help understand the technologies discussed in this article.
Understanding the following technical concepts is essential for grasping the full implications of the security threats and defensive measures discussed in this article. These definitions provide context for both technical and non-technical readers.