Cohere’s first vision model Aya Vision is here with broad, multilingual understanding and open weights — but there’s a catch - Related to sales, catch, platform, agents, data
Cohere’s first vision model Aya Vision is here with broad, multilingual understanding and open weights — but there’s a catch
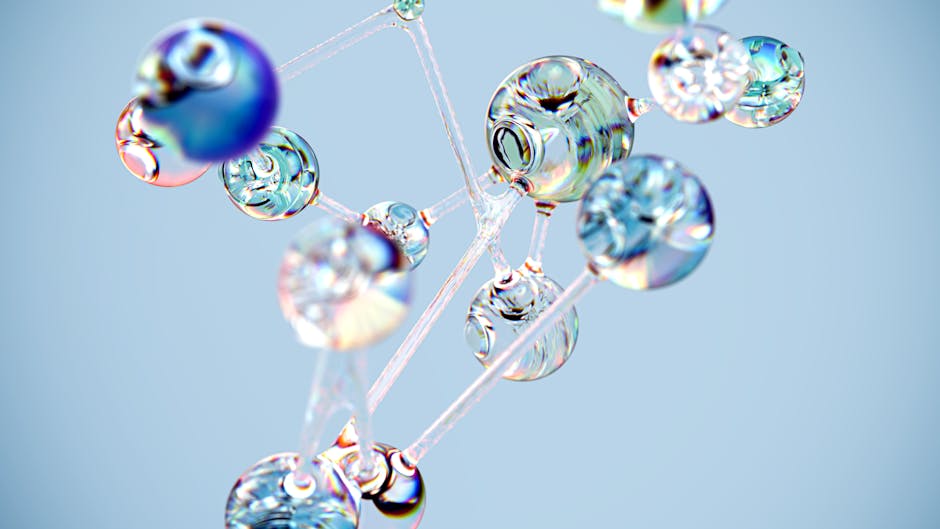
Canadian AI startup Cohere launched in 2019 specifically targeting the enterprise, but independent research has shown it has so far struggled to gain much of a market share among third-party developers compared to rival proprietary [website] model providers such as OpenAI and Anthropic, not to mention the rise of Chinese open-source competitor DeepSeek.
Yet Cohere continues to bolster its offerings: Today, its non-profit research division Cohere for AI presented the release of its first vision model, Aya Vision, a new open-weight multimodal AI model that integrates language and vision capabilities and boasts the differentiator of supporting inputs in 23 different languages spoken by what Cohere says in an official blog post is “half the world’s population,” making it appeal to a wide global audience.
Aya Vision is designed to enhance AI’s ability to interpret images, generate text, and translate visual content into natural language, making multilingual AI more accessible and effective. This would be especially helpful for enterprises and organizations operating in multiple markets around the world with different language preferences.
It’s available now on Cohere’s website and on AI code communities Hugging Face and Kaggle under a Creative Commons Attribution-NonCommercial [website] International (CC BY-NC [website] license, allowing researchers and developers to freely use, modify and share the model for non-commercial purposes as long as proper attribution is given.
In addition, Aya Vision is available through WhatsApp, allowing clients to interact with the model directly in a familiar environment.
This limits its use for enterprises and as an engine for paid apps or moneymaking workflows, unfortunately.
It comes in 8-billion and 32-billion parameter versions (parameters refer to the number of internal settings in an AI model, including its weights and biases, with more usually denoting a more powerful and performant model).
Even though leading AI models from rivals can understand text across multiple languages, extending this capability to vision-based tasks is a challenge.
But Aya Vision overcomes this by allowing clients to generate image captions, answer visual questions, translate images, and perform text-based language tasks in a diverse set of languages:
In its blog post, Cohere showed how Aya Vision can analyze imagery and text on product packaging and provide translations or explanations. It can also identify and describe art styles from different cultures, helping customers learn about objects and traditions through AI-powered visual understanding.
Aya Vision’s capabilities have broad implications across multiple fields:
• Language learning and education: consumers can translate and describe images in multiple languages, making educational content more accessible.
• Cultural preservation: The model can generate detailed descriptions of art, landmarks and historical artifacts, supporting cultural documentation in underrepresented languages.
• Accessibility tools: Vision-based AI can assist visually impaired clients by providing detailed image descriptions in their native language.
• Global communication: Real-time multimodal translation enables organizations and individuals to communicate across languages more effectively.
Strong performance and high efficiency across leading benchmarks.
One of Aya Vision’s standout elements is its efficiency and performance relative to model size. Despite being significantly smaller than some leading multimodal models, Aya Vision has outperformed much larger alternatives in several key benchmarks.
• Aya Vision 8B outperforms Llama 90B, which is 11 times larger.
• Aya Vision 32B outperforms Qwen 72B, Llama 90B and Molmo 72B, all of which are at least twice as large (or more).
• Benchmarking results on AyaVisionBench and m-WildVision show Aya Vision 8B achieving win rates of up to 79%, and Aya Vision 32B reaching 72% win rates in multilingual image understanding tasks.
A visual comparison of efficiency vs. performance highlights Aya Vision’s advantage. As shown in the efficiency vs. performance trade-off graph, Aya Vision 8B and 32B demonstrate best-in-class performance relative to their parameter size, outperforming much larger models while maintaining computational efficiency.
The tech innovations powering Aya Vision.
Cohere For AI attributes Aya Vision’s performance gains to several key innovations:
• Synthetic annotations: The model leverages synthetic data generation to enhance training on multimodal tasks.
• Multilingual data scaling: By translating and rephrasing data across languages, the model gains a broader understanding of multilingual contexts.
• Multimodal model merging: Advanced techniques combine insights from both vision and language models, improving overall performance.
These advancements allow Aya Vision to process images and text with greater accuracy while maintaining strong multilingual capabilities.
The step-by-step performance improvement chart showcases how incremental innovations, including synthetic fine-tuning (SFT), model merging, and scaling, contributed to Aya Vision’s high win rates.
Implications for enterprise decision-makers.
Despite Aya Vision’s ostensibly catering to the enterprise, businesses may have a hard time making much use of it given its restrictive non-commercial licensing terms.
Nonetheless, CEOs, CTOs, IT leaders and AI researchers may use the models to explore AI-driven multilingual and multimodal capabilities within their organizations — particularly in research, prototyping and benchmarking.
Enterprises can still use it for internal research and development, evaluating multilingual AI performance and experimenting with multimodal applications.
CTOs and AI teams will find Aya Vision valuable as a highly efficient, open-weight model that outperforms much larger alternatives while requiring fewer computational resources.
This makes it a useful tool for benchmarking against proprietary models, exploring potential AI-driven solutions, and testing multilingual multimodal interactions before committing to a commercial deployment strategy.
For data scientists and AI researchers, Aya Vision is much more useful.
Its open-source nature and rigorous benchmarks provide a transparent foundation for studying model behavior, fine-tuning in non-commercial settings, and contributing to open AI advancements.
Whether used for internal research, academic collaborations, or AI ethics evaluations, Aya Vision serves as a cutting-edge resource for enterprises looking to stay at the forefront of multilingual and multimodal AI — without the constraints of proprietary, closed-source models.
Aya Vision is part of Aya, a broader initiative by Cohere focused on making AI and related tech more multilingual.
Since its inception in February 2024, the Aya initiative has engaged a global research community of over 3,000 independent researchers across 119 countries, working together to improve language AI models.
To further its commitment to open science, Cohere has released the open weights for both Aya Vision 8B and 32B on Kaggle and Hugging Face, ensuring researchers worldwide can access and experiment with the models. In addition, Cohere For AI has introduced the AyaVisionBenchmark, a new multilingual vision evaluation set designed to provide a rigorous assessment framework for multimodal AI.
The availability of Aya Vision as an open-weight model marks an essential step in making multilingual AI research more inclusive and accessible.
Aya Vision builds on the success of Aya Expanse, another LLM family from Cohere For AI focused on multilingual AI. By expanding its focus to multimodal AI, Cohere For AI is positioning Aya Vision as a key tool for researchers, developers, and businesses looking to integrate multilingual AI into their workflows.
As the Aya initiative continues to evolve, Cohere For AI has also introduced plans to launch a new collaborative research effort in the coming weeks. Researchers and developers interested in contributing to multilingual AI advancements can join the open science community or apply for research grants.
For now, Aya Vision’s release represents a significant leap in multilingual multimodal AI, offering a high-performance, open-weight solution that challenges the dominance of larger, closed-source models. By making these advancements available to the broader research community, Cohere For AI continues to push the boundaries of what is possible in AI-driven multilingual communication.
AI coding tools have been both a boon and a bane for software engineers and developers. While many have managed to upskill themselves with AI and use ......
Ampere is accelerating its expansion from Arm-based server processors for AI processing into networking chips for the telecom market.
Accenture has strengthened its AI capabilities with the acquisition of Danish AI enterprise Halfspace and an investment in AI-driven analytics firm Aaru.......
MachineHack Launches Datalyze: A Simulation-Based Gamified Learning Platform for Data Analytics
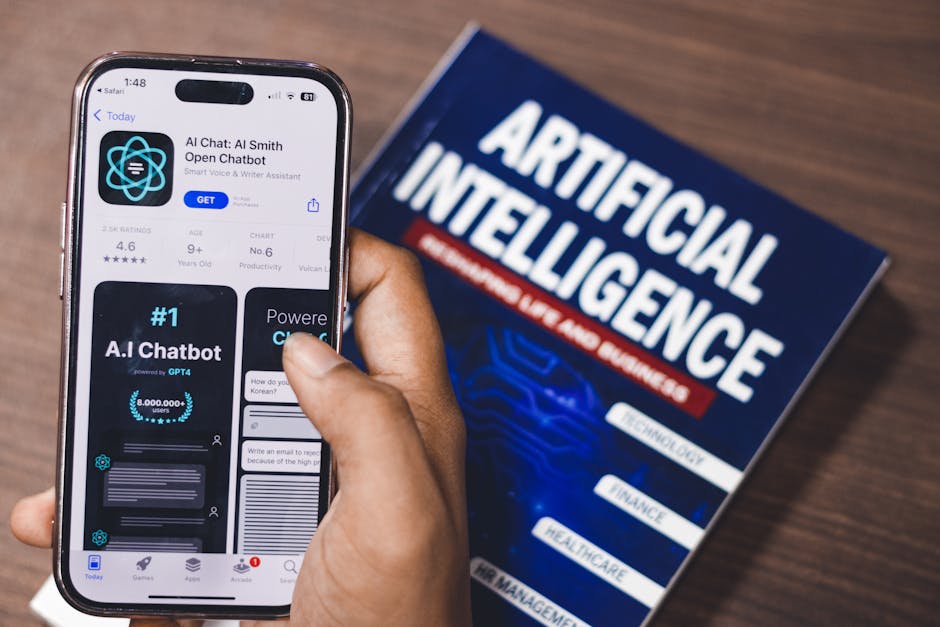
The ability to analyze and interpret data is no longer limited to data scientists or highly skilled analysts. With the launch of Datalyze, MachineHack is breaking down barriers, making data analytics accessible to everyone—from students and small business owners to non-technical professionals.
Datalyze is an AI-powered learning platform that provides simulation-based, gamified learning experiences. It simplifies data exploration, cleaning, and analysis, allowing people to derive actionable insights without requiring any coding knowledge or technical expertise.
For years, MachineHack has been known for its data science hackathons, helping professionals and aspiring data scientists sharpen their skills. With Datalyze, the enterprise is now focusing on education and skill-building, ensuring that data literacy becomes more accessible to a wider audience.
Datalyze eliminates the traditional hurdles of learning data analytics, such as complex programming languages and statistical jargon. Instead, it introduces a no-code, guided, and intuitive way to work with data.
Datalyze offers a structured, hands-on approach to learning data analytics. clients can engage in real-world data tasks while being guided through the entire process.
customers learn how to upload and structure data correctly. The platform simplifies the process of organizing, cleaning, and preparing datasets for analysis.
Datalyze teaches the fundamentals of data exploration, visualization, and interpretation. people can identify patterns, trends, and insights without needing to write SQL queries or Python scripts.
Interactive quizzes and challenges help individuals test their understanding and apply what they’ve learned in practical scenarios.
Datalyze follows a simple, step-by-step approach to ensure a smooth learning curve:
Upload Data – Users can import datasets from spreadsheets, databases, or preloaded sample data. Clean & Prepare – AI-powered suggestions help fix inconsistencies and standardize data. Explore Data – Users can visualize key patterns, trends, and correlations. Generate Insights – AI-based recommendations help users interpret data and find actionable insights. Assess & Improve – Users can take mini-assessments to reinforce their learning.
Unlike other analytics tools that require knowledge of SQL, Python, or R, Datalyze is entirely no-code. The platform uses an intuitive, drag-and-drop interface, making it easy for anyone to use.
The platform offers step-by-step guidance, ensuring customers don’t get stuck at any stage of the analysis process. AI-driven recommendations provide additional support.
Datalyze is accessible from anywhere without the need for expensive hardware or software. No installations, no costly licenses—just log in and start analyzing.
Why Datalyze Matters in Today’s Data-Driven World.
The demand for data literacy is skyrocketing. Whether it’s business decision-making, marketing strategy, or financial forecasting, companies rely on data to stay competitive.
But most people lack the tools and training to work with data effectively. Excel has limitations, traditional BI tools are expensive, and programming languages like Python and SQL can be intimidating.
Datalyze fills this gap by making data analytics easy, accessible, and practical for everyone.
MachineHack is constantly working on enhancing Datalyze with new elements and capabilities:
🔹 Industry-Specific Learning Tracks – Specialized modules for healthcare, finance, retail, and marketing analytics.
🔹 Collaboration elements – Allowing teams to analyze data together.
🔹 AI-Powered Insights – More advanced predictive analytics and forecasting tools.
🔹 More Gamification – Challenges, leaderboards, and certifications to make learning fun.
Datalyze is not just another data tool—it’s a movement towards democratizing data analytics. With MachineHack’s expertise in AI and data science competitions, this platform is set to transform the way people learn and apply data analytics skills.
Whether you’re a student, entrepreneur, or business professional, Datalyze empowers you to harness the power of data—easily, affordably, and effectively.
GitHub’s GHAS, a developer-first application testing solution built to improve code security in public and private repositories on GitHub, will now be......
I have been a data team manager for six months, and my team has grown from three to five.
I wrote about my initial manager experiences back in Novemb......
Food and grocery delivery platform Swiggy handles billions of orders annually across approximately 700 cities. Managing such a scale requires real-tim......
How Microsoft's new AI sales agents will help your team close deals faster
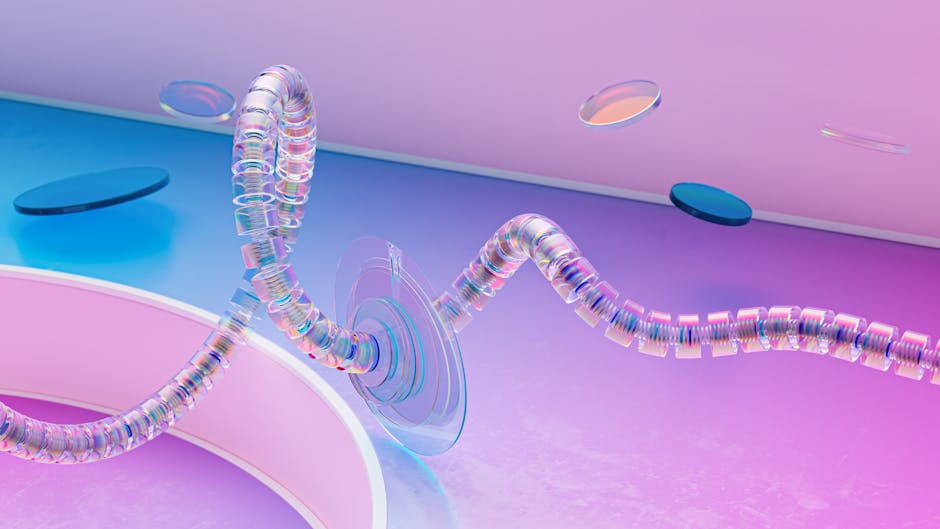
AI agents are revolutionizing the workplace by performing actions autonomously for workers and, in turn, streamlining operations. Microsoft has sprinkled agents throughout its offerings to help workers across different industries, and the newest addition includes sales.
On Wednesday, Microsoft unveiled Sales Agent, which can help sales teams close more deals faster by researching leads, setting up meetings, reaching out to end-consumers, and even closing sales for low-impact leads, 's blog post. The agent uses the corporation's CRM, corporation data, and Microsoft 365 data to inform its responses.
Also: The future of sales? These AI agents offer 24/7 ABC energy for SMBs.
Microsoft also launched Sales Chat which, as the name implies, is an AI-chat interface where the sales team can access all the information they need from CRM data, pitch decks, meetings, emails, the web, and more. Microsoft says this tool should help teams spend less time "digging" and more time selling.
, to access the information, clients can use simple conversational prompts, such as "Give me a list of deals that are at risk of falling through" or "What should I know going into tomorrow's meeting with this customer?"
Furthermore, clients can connect Sales Agent and Sales Chat to Dynamics 365 and Salesforce, two industry-leading CRMs, to close deals without accessing the CRM themselves. The agents will also integrate with Microsoft 365 Copilot Chat for a seamless experience.
clients can access the agents via public preview in Microsoft 365 Copilot and Copilot Chat starting in May.
Microsoft also unveiled Microsoft AI Accelerator for Sales, a program to help consumers optimize their sales operations. Microsoft describes it as "an , transform your sales organization, and migrate off legacy CRM vendors."
Also: Goodbye Gemini, hello Pixel Sense? What we know about Google's AI assistant for Pixel 10.
The program includes access to Microsoft 365 Copilot, pre-built and custom agents, Dynamics 365 Sales, and AI model fine-tuning. Perhaps the most useful tool is the ability to work with Microsoft's AI experts to aid the transition. The program will be available for end-people on April 1.
As far back as 2023, Google was reportedly working on an AI assistant for Pixel phones called.
Ongoing Trump administration cuts to government agencies risk creating new collateral damage: the future of AI research.
Market Impact Analysis
Market Growth Trend
2018 | 2019 | 2020 | 2021 | 2022 | 2023 | 2024 |
---|---|---|---|---|---|---|
23.1% | 27.8% | 29.2% | 32.4% | 34.2% | 35.2% | 35.6% |
Quarterly Growth Rate
Q1 2024 | Q2 2024 | Q3 2024 | Q4 2024 |
---|---|---|---|
32.5% | 34.8% | 36.2% | 35.6% |
Market Segments and Growth Drivers
Segment | Market Share | Growth Rate |
---|---|---|
Machine Learning | 29% | 38.4% |
Computer Vision | 18% | 35.7% |
Natural Language Processing | 24% | 41.5% |
Robotics | 15% | 22.3% |
Other AI Technologies | 14% | 31.8% |
Technology Maturity Curve
Different technologies within the ecosystem are at varying stages of maturity:
Competitive Landscape Analysis
Company | Market Share |
---|---|
Google AI | 18.3% |
Microsoft AI | 15.7% |
IBM Watson | 11.2% |
Amazon AI | 9.8% |
OpenAI | 8.4% |
Future Outlook and Predictions
The Vision Cohere First landscape is evolving rapidly, driven by technological advancements, changing threat vectors, and shifting business requirements. Based on current trends and expert analyses, we can anticipate several significant developments across different time horizons:
Year-by-Year Technology Evolution
Based on current trajectory and expert analyses, we can project the following development timeline:
Technology Maturity Curve
Different technologies within the ecosystem are at varying stages of maturity, influencing adoption timelines and investment priorities:
Innovation Trigger
- Generative AI for specialized domains
- Blockchain for supply chain verification
Peak of Inflated Expectations
- Digital twins for business processes
- Quantum-resistant cryptography
Trough of Disillusionment
- Consumer AR/VR applications
- General-purpose blockchain
Slope of Enlightenment
- AI-driven analytics
- Edge computing
Plateau of Productivity
- Cloud infrastructure
- Mobile applications
Technology Evolution Timeline
- Improved generative models
- specialized AI applications
- AI-human collaboration systems
- multimodal AI platforms
- General AI capabilities
- AI-driven scientific breakthroughs
Expert Perspectives
Leading experts in the ai tech sector provide diverse perspectives on how the landscape will evolve over the coming years:
"The next frontier is AI systems that can reason across modalities and domains with minimal human guidance."
— AI Researcher
"Organizations that develop effective AI governance frameworks will gain competitive advantage."
— Industry Analyst
"The AI talent gap remains a critical barrier to implementation for most enterprises."
— Chief AI Officer
Areas of Expert Consensus
- Acceleration of Innovation: The pace of technological evolution will continue to increase
- Practical Integration: Focus will shift from proof-of-concept to operational deployment
- Human-Technology Partnership: Most effective implementations will optimize human-machine collaboration
- Regulatory Influence: Regulatory frameworks will increasingly shape technology development
Short-Term Outlook (1-2 Years)
In the immediate future, organizations will focus on implementing and optimizing currently available technologies to address pressing ai tech challenges:
- Improved generative models
- specialized AI applications
- enhanced AI ethics frameworks
These developments will be characterized by incremental improvements to existing frameworks rather than revolutionary changes, with emphasis on practical deployment and measurable outcomes.
Mid-Term Outlook (3-5 Years)
As technologies mature and organizations adapt, more substantial transformations will emerge in how security is approached and implemented:
- AI-human collaboration systems
- multimodal AI platforms
- democratized AI development
This period will see significant changes in security architecture and operational models, with increasing automation and integration between previously siloed security functions. Organizations will shift from reactive to proactive security postures.
Long-Term Outlook (5+ Years)
Looking further ahead, more fundamental shifts will reshape how cybersecurity is conceptualized and implemented across digital ecosystems:
- General AI capabilities
- AI-driven scientific breakthroughs
- new computing paradigms
These long-term developments will likely require significant technical breakthroughs, new regulatory frameworks, and evolution in how organizations approach security as a fundamental business function rather than a technical discipline.
Key Risk Factors and Uncertainties
Several critical factors could significantly impact the trajectory of ai tech evolution:
Organizations should monitor these factors closely and develop contingency strategies to mitigate potential negative impacts on technology implementation timelines.
Alternative Future Scenarios
The evolution of technology can follow different paths depending on various factors including regulatory developments, investment trends, technological breakthroughs, and market adoption. We analyze three potential scenarios:
Optimistic Scenario
Responsible AI driving innovation while minimizing societal disruption
Key Drivers: Supportive regulatory environment, significant research breakthroughs, strong market incentives, and rapid user adoption.
Probability: 25-30%
Base Case Scenario
Incremental adoption with mixed societal impacts and ongoing ethical challenges
Key Drivers: Balanced regulatory approach, steady technological progress, and selective implementation based on clear ROI.
Probability: 50-60%
Conservative Scenario
Technical and ethical barriers creating significant implementation challenges
Key Drivers: Restrictive regulations, technical limitations, implementation challenges, and risk-averse organizational cultures.
Probability: 15-20%
Scenario Comparison Matrix
Factor | Optimistic | Base Case | Conservative |
---|---|---|---|
Implementation Timeline | Accelerated | Steady | Delayed |
Market Adoption | Widespread | Selective | Limited |
Technology Evolution | Rapid | Progressive | Incremental |
Regulatory Environment | Supportive | Balanced | Restrictive |
Business Impact | Transformative | Significant | Modest |
Transformational Impact
Redefinition of knowledge work, automation of creative processes. This evolution will necessitate significant changes in organizational structures, talent development, and strategic planning processes.
The convergence of multiple technological trends—including artificial intelligence, quantum computing, and ubiquitous connectivity—will create both unprecedented security challenges and innovative defensive capabilities.
Implementation Challenges
Ethical concerns, computing resource limitations, talent shortages. Organizations will need to develop comprehensive change management strategies to successfully navigate these transitions.
Regulatory uncertainty, particularly around emerging technologies like AI in security applications, will require flexible security architectures that can adapt to evolving compliance requirements.
Key Innovations to Watch
Multimodal learning, resource-efficient AI, transparent decision systems. Organizations should monitor these developments closely to maintain competitive advantages and effective security postures.
Strategic investments in research partnerships, technology pilots, and talent development will position forward-thinking organizations to leverage these innovations early in their development cycle.
Technical Glossary
Key technical terms and definitions to help understand the technologies discussed in this article.
Understanding the following technical concepts is essential for grasping the full implications of the security threats and defensive measures discussed in this article. These definitions provide context for both technical and non-technical readers.