Salesforce’s AgentExchange launches with 200+ partners to automate your boring work tasks - Related to tasks, models, footage, teams, frame
Hugging Face Teams Up With JFrog To Hunt Down Malicious AI Models
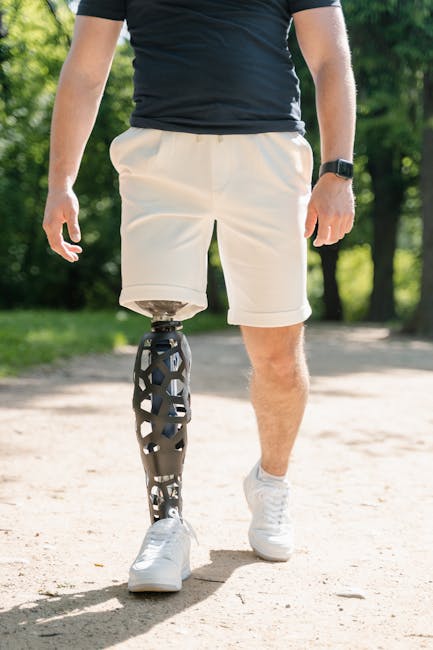
Hugging Face, one of the most sought-out platforms to host AI models, presented a partnership with software supply chain platform JFrog to improve security on the Hugging Face Hub.
Hugging Face explained that the model weights can contain code executed upon deserialisation and sometimes at inference time, depending on the format. To tackle this, it plans to integrate JFrog’s scanner into its platform, adding new scanning functionality to reduce false positives on the Model Hub.
“Through our integration with Hugging Face, we bring a powerful, methodology-driven approach that eliminates 96% of current false positives detected by scanners on the Hugging Face platform while also identifying threats that traditional scanners fail to detect,” JFrog stated. “Our unique approach dissects embedded code, extracts payloads, and normalises evidence to eliminate false positives while detecting more serious threats.”.
JFrog’s scanner aims to perform a deeper analysis and parse the code in model weights to check for potential malicious usage. The scanning is powered by its ‘file security scans’ interface.
It supports various models, including pickle-based models, TensorFlow models, GPT-Generated Unified Format (GGUF) models, Open Neural Network Exchange (ONNX) models, and more. Their documentation lists out all kinds of AI models supported by JFrog.
individuals do not need to do anything to benefit from the integration. All the public model repositories will be scanned by JFrog automatically as soon as they push files to the Model Hub.
Hugging Face has shared an example repository where individuals can check how the scanner flags malicious files.
With this integration to Hugging Face, people should get a more effective sense of security before using AI models to deploy for their use-cases.
Ongoing Trump administration cuts to government agencies risk creating new collateral damage: the future of AI research.
Dans le cadre de notre dossier « Visionnaires de l’I. A : Comment l’intelligence artificielle façonne le monde de demain », Daria Viktorova nous parta......
American EV giant Tesla is hiring a software engineer in Pune, who is focused on front-end development.
The role involves working with a software tea......
Salesforce’s AgentExchange launches with 200+ partners to automate your boring work tasks
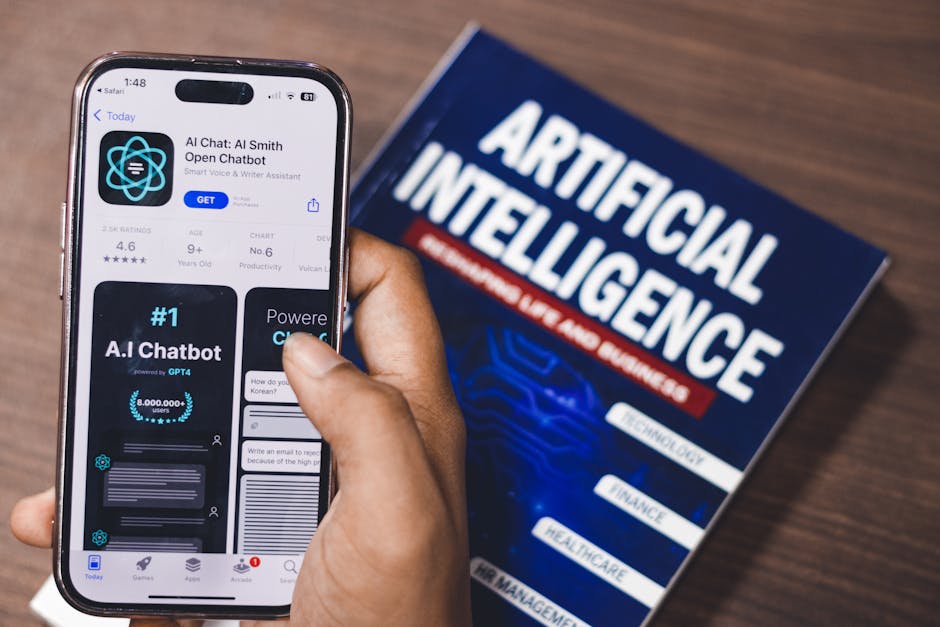
Salesforce has just unveiled a new marketplace called AgentExchange, creating what it describes as the first trusted marketplace for AI agents in enterprise software and positioning itself at the center of what it estimates will be a $6 trillion “digital labor” market.
The corporation’s push into AI agents — software that can perform complex tasks autonomously — represents one of Silicon Valley’s most ambitious attempts to transform how businesses operate.
“We’ve seen great adoption across clients like ZoomInfo, Remarkable, and Mimit Health who are using Agentforce in Slack to boost productivity,” presented Rob Seaman, SVP of product management at Salesforce, in an .
The new marketplace launches with more than 200 partners, including Google Cloud, DocuSign, Box and Workday, who are building pre-packaged agent solutions that businesses can implement without extensive technical expertise.
While much of the attention around artificial intelligence has focused on text-generating tools like ChatGPT, Salesforce is betting that specialized AI agents will deliver more immediate business value. These agents don’t just generate text — they take actions within business systems.
“If you look at the overall labor market, we don’t actually have enough people to do the jobs we currently need them to do,” Seaman explained. “This is a big transformation that will change many jobs, but it’s providing more labor capacity into the system.”.
The firm’s research points to significant demand for automation of administrative tasks. Amit Khanna, SVP and GM for Salesforce Health, cited research showing that “around 87% of people in healthcare say they work late each day to finish up administrative tasks.”.
Targeting healthcare’s administrative burden with specialized AI agents.
Healthcare presents a compelling use case for AI agents. The industry is administratively burdened, with clinicians spending significant time on documentation rather than patient care.
Khanna outlined several healthcare-specific applications: “We are looking at three areas: patient access — making appointments, finding providers, benefits verification; public health paperwork; and clinical trial matching.”.
For patient privacy, Salesforce has implemented multiple safeguards. “When we send data to language models for summarization, we remove all protected health information first,” Khanna explained. “It uses tags instead of names, generates a summary, and then replaces those tags with actual names before presenting to the user.”.
Salesforce’s no-code approach makes AI agent creation accessible to business teams.
A significant aspect of Salesforce’s approach is lowering the technical barriers to creating AI agents. , what has surprised him most is “the speed of creation and iteration.”.
“The number of people now that can create technology to solve problems has expanded greatly because it’s based on topics and instructions written in natural language, not programming languages,” Seaman showcased.
This simplification could enable business consumers to create their own automation solutions without depending on technical teams.
What early Salesforce end-individuals have learned about deploying AI agents successfully.
Early adopters have discovered critical considerations for effective AI agent deployment. Seaman noted that many organizations “don’t spend enough time thinking about dead ends or negative instructions.”.
“It’s just as essential to give topics and instructions as it is to provide guidance on what to do if the agent doesn’t know how to proceed,” he explained.
Remarkable, one early adopter, has deployed an IT help-desk agent that employees interact with directly in Slack. The agent handles routine tasks like password resets and helps new hires set up their equipment, while knowing when to involve human IT staff.
Salesforce’s vision for how AI agents will transform workplace roles and responsibilities.
As AI agents become more capable, questions about their impact on employment are inevitable. Seaman frames the technology not as a replacement for human workers but as a complement.
“I don’t think about it as replacing people. I think about it as augmenting them and helping them focus on the work that really matters,” he showcased.
In healthcare, Khanna believes AI agents will first tackle administrative tasks before gradually moving into clinical support roles. “The next wave will move toward the clinical side as doctors build trust in the technology,” he predicted.
The market for AI agents is still developing, but Salesforce is positioning itself as a platform firm rather than trying to build every possible agent itself. With AgentExchange, it’s creating an ecosystem where partners can build specialized agents for different industries.
Whether businesses embrace these AI agents as essential productivity tools or view them as interesting but not-yet-critical technology remains to be seen. For now, Salesforce is betting that the future workplace includes both human employees and digital ones.
Nvidia-backed hyperscaler AI startup CoreWeave is set to acquire Weights & Biases, a developer platform for AI. The company expects to close the acqui......
When OpenAI released its frontier AI models, the industry discourse quickly shifted to AI ‘eating’ startups, particularly SaaS companies. Despite thes......
GitHub’s GHAS, a developer-first application testing solution built to improve code security in public and private repositories on GitHub, will now be......
VOGIC AI Cuts Through Chaos, Scanning Your CCTV Footage Frame by Frame
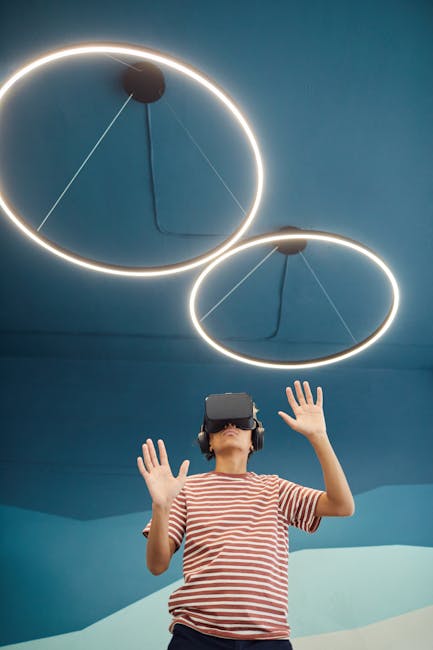
Rummaging through CCTV footage to find something specific is not just cumbersome but also extremely time-consuming. While it can be manageable for home individuals, it is a big undertaking for organisations, enterprises, government bodies, and public/private institutions.
Gurugram-based VOGIC AI decided to solve this real-world problem using AI.
AIM caught up with Arijit Biswas, the CEO and co-founder of VOGIC AI, for an ’re tackling the problem, some of its tech internals, and how they plan to make it secure.
Biswas explained that the CCTV footage is usually unstructured and in high volume, considering CCTVs run 24/7. It is tough to analyse a video or imagery data with so much going on. Citing Microsoft Power BI and Excel, which exist for numerical and text data, he stated there are no such popular tools helping the cause in the case of video data.
VOGIC AI empowers organisations with tools and pre-built modules through which one can extract information based on contexts like ‘a person walking close to a car’ or ‘a person trying to capture a photo of the car’.
He elaborated on this and noted, “The Army might have a different context, the law enforcement agencies might have a different context and a chain of retail stores might have a different context. There are different institutes and physical setups that have different contexts.”.
So, whether it is drone information or satellite information, with VOGIC AI, footages from such organisations can be analysed easily.
The firm’s name combines ‘video’ with ‘logic’. When asked how VOGIC AI integrates with the various CCTV platforms nationwide to provide its AI capabilities, Biswas explained that most CCTV vendors like CP Plus, Honeywell, Bosch, and others follow a standardised protocol called the Open Network Video Interface Forum (ONVIF). Their system is compatible with the same, enabling them to work with all kinds of OEMs.
In addition, some companies use a video management system like Milestone. They integrate their solution directly through the CCTV cameras or via the video management systems that the companies use.
So, what’s the tech stack (or the AI model) making it all this possible?
Biswas stated that they use a mix of conventional neural networks and large vision language models (VLMs), which are LLMs for videos, fine-tuned to the context of a CCTV camera. The VLM works with the concept of images and text pairs, which helps index the footage.
He further explained that the first layer of indexing (the heavy workload) is done by the neural networks, and the next layer of contextual information is added by the VLMs. The base model for the VLM is LLaVA, which was further trained using CCTV-specific videos to build VOGIC AI’s solution.
What Were The Challenges in Building This?
Biswas highlighted that the primary challenge was to acquire the video data for training, considering it is highly sensitive in nature. He also expressed that existing AI systems struggle to extract meaningful context from video footage, leading to false alerts.
Lastly, such VLMs are computationally intensive, which prompts the use of expensive GPUs, which may not be ideal.
While the platform has largely solved these challenges, it is taking further steps, such as making a crowdsourcing platform to encourage individuals to contribute video data, decluttering the VLM to a smaller model and adding contextual information to the footage.
For clients with a large CCTV infrastructure, the firm deploys most of its code based on the customer’s private cloud. The same applies for organisations with a data centre.
The firm also provides a GPU box, which connects to the host’s internet network, and processes data from within the network. However, the firm confirms that nowhere in the process is the data extracted or sent anywhere else.
In addition to this, it takes several safety measures, like data anonymisation with face blurring. Though the system can detect if the subject is a male or a female, it will not identify the person unless permitted to do so. end-clients can choose to toggle this feature as per the data privacy laws in their respective country.
“We have partnered with companies like Lenovo and Dell, and we are an NVIDIA Inception & Metropolis partner. A lot of innovations that NVIDIA carries out in security and safety are integrated into our systems too,” Biswas added.
The revenue model for VOGIC AI is straightforward, it includes charging clients per CCTV camera for continuous deployment. It charges a monthly license fee or as per the footage duration if it involves analysing a lot of archived data.
VOGIC AI is focused on businesses and organisations but also aims to integrate its solution for law enforcement and India’s national security, using a business-to-government (B2G) model. The firm has worked on projects like Varanasi Smart City and with a few drone surveillance companies. It is in the process of being tested by law enforcement agencies.
Biswas also mentioned his plans to explore working with security system integrators outside India, particularly in the Middle East.
Les forces de l’ordre du monde entier ont démantelé un réseau de diffusion de contenus pédopornographiques générés par l’IA. Cette opération a mené à ......
En seulement deux jours, le dernier modèle d’IA développé par Google a réussi un exploit inédit. Il a rattrapé une décennie entière de recherche humai......
Owners of the iPhone 15 Pro and Pro Max can now tap into a helpful AI-powered feature, courtesy of the latest iOS [website] developer beta.
Market Impact Analysis
Market Growth Trend
2018 | 2019 | 2020 | 2021 | 2022 | 2023 | 2024 |
---|---|---|---|---|---|---|
23.1% | 27.8% | 29.2% | 32.4% | 34.2% | 35.2% | 35.6% |
Quarterly Growth Rate
Q1 2024 | Q2 2024 | Q3 2024 | Q4 2024 |
---|---|---|---|
32.5% | 34.8% | 36.2% | 35.6% |
Market Segments and Growth Drivers
Segment | Market Share | Growth Rate |
---|---|---|
Machine Learning | 29% | 38.4% |
Computer Vision | 18% | 35.7% |
Natural Language Processing | 24% | 41.5% |
Robotics | 15% | 22.3% |
Other AI Technologies | 14% | 31.8% |
Technology Maturity Curve
Different technologies within the ecosystem are at varying stages of maturity:
Competitive Landscape Analysis
Company | Market Share |
---|---|
Google AI | 18.3% |
Microsoft AI | 15.7% |
IBM Watson | 11.2% |
Amazon AI | 9.8% |
OpenAI | 8.4% |
Future Outlook and Predictions
The Your Frame Hugging landscape is evolving rapidly, driven by technological advancements, changing threat vectors, and shifting business requirements. Based on current trends and expert analyses, we can anticipate several significant developments across different time horizons:
Year-by-Year Technology Evolution
Based on current trajectory and expert analyses, we can project the following development timeline:
Technology Maturity Curve
Different technologies within the ecosystem are at varying stages of maturity, influencing adoption timelines and investment priorities:
Innovation Trigger
- Generative AI for specialized domains
- Blockchain for supply chain verification
Peak of Inflated Expectations
- Digital twins for business processes
- Quantum-resistant cryptography
Trough of Disillusionment
- Consumer AR/VR applications
- General-purpose blockchain
Slope of Enlightenment
- AI-driven analytics
- Edge computing
Plateau of Productivity
- Cloud infrastructure
- Mobile applications
Technology Evolution Timeline
- Improved generative models
- specialized AI applications
- AI-human collaboration systems
- multimodal AI platforms
- General AI capabilities
- AI-driven scientific breakthroughs
Expert Perspectives
Leading experts in the ai tech sector provide diverse perspectives on how the landscape will evolve over the coming years:
"The next frontier is AI systems that can reason across modalities and domains with minimal human guidance."
— AI Researcher
"Organizations that develop effective AI governance frameworks will gain competitive advantage."
— Industry Analyst
"The AI talent gap remains a critical barrier to implementation for most enterprises."
— Chief AI Officer
Areas of Expert Consensus
- Acceleration of Innovation: The pace of technological evolution will continue to increase
- Practical Integration: Focus will shift from proof-of-concept to operational deployment
- Human-Technology Partnership: Most effective implementations will optimize human-machine collaboration
- Regulatory Influence: Regulatory frameworks will increasingly shape technology development
Short-Term Outlook (1-2 Years)
In the immediate future, organizations will focus on implementing and optimizing currently available technologies to address pressing ai tech challenges:
- Improved generative models
- specialized AI applications
- enhanced AI ethics frameworks
These developments will be characterized by incremental improvements to existing frameworks rather than revolutionary changes, with emphasis on practical deployment and measurable outcomes.
Mid-Term Outlook (3-5 Years)
As technologies mature and organizations adapt, more substantial transformations will emerge in how security is approached and implemented:
- AI-human collaboration systems
- multimodal AI platforms
- democratized AI development
This period will see significant changes in security architecture and operational models, with increasing automation and integration between previously siloed security functions. Organizations will shift from reactive to proactive security postures.
Long-Term Outlook (5+ Years)
Looking further ahead, more fundamental shifts will reshape how cybersecurity is conceptualized and implemented across digital ecosystems:
- General AI capabilities
- AI-driven scientific breakthroughs
- new computing paradigms
These long-term developments will likely require significant technical breakthroughs, new regulatory frameworks, and evolution in how organizations approach security as a fundamental business function rather than a technical discipline.
Key Risk Factors and Uncertainties
Several critical factors could significantly impact the trajectory of ai tech evolution:
Organizations should monitor these factors closely and develop contingency strategies to mitigate potential negative impacts on technology implementation timelines.
Alternative Future Scenarios
The evolution of technology can follow different paths depending on various factors including regulatory developments, investment trends, technological breakthroughs, and market adoption. We analyze three potential scenarios:
Optimistic Scenario
Responsible AI driving innovation while minimizing societal disruption
Key Drivers: Supportive regulatory environment, significant research breakthroughs, strong market incentives, and rapid user adoption.
Probability: 25-30%
Base Case Scenario
Incremental adoption with mixed societal impacts and ongoing ethical challenges
Key Drivers: Balanced regulatory approach, steady technological progress, and selective implementation based on clear ROI.
Probability: 50-60%
Conservative Scenario
Technical and ethical barriers creating significant implementation challenges
Key Drivers: Restrictive regulations, technical limitations, implementation challenges, and risk-averse organizational cultures.
Probability: 15-20%
Scenario Comparison Matrix
Factor | Optimistic | Base Case | Conservative |
---|---|---|---|
Implementation Timeline | Accelerated | Steady | Delayed |
Market Adoption | Widespread | Selective | Limited |
Technology Evolution | Rapid | Progressive | Incremental |
Regulatory Environment | Supportive | Balanced | Restrictive |
Business Impact | Transformative | Significant | Modest |
Transformational Impact
Redefinition of knowledge work, automation of creative processes. This evolution will necessitate significant changes in organizational structures, talent development, and strategic planning processes.
The convergence of multiple technological trends—including artificial intelligence, quantum computing, and ubiquitous connectivity—will create both unprecedented security challenges and innovative defensive capabilities.
Implementation Challenges
Ethical concerns, computing resource limitations, talent shortages. Organizations will need to develop comprehensive change management strategies to successfully navigate these transitions.
Regulatory uncertainty, particularly around emerging technologies like AI in security applications, will require flexible security architectures that can adapt to evolving compliance requirements.
Key Innovations to Watch
Multimodal learning, resource-efficient AI, transparent decision systems. Organizations should monitor these developments closely to maintain competitive advantages and effective security postures.
Strategic investments in research partnerships, technology pilots, and talent development will position forward-thinking organizations to leverage these innovations early in their development cycle.
Technical Glossary
Key technical terms and definitions to help understand the technologies discussed in this article.
Understanding the following technical concepts is essential for grasping the full implications of the security threats and defensive measures discussed in this article. These definitions provide context for both technical and non-technical readers.